

□ OmegaFold: High-resolution de novo structure prediction from primary sequence
>> https://www.biorxiv.org/content/10.1101/2022.07.21.500999v1.full.pdf
OmegaFold enables accurate predictions on orphan proteins that do not belong to any functionally characterized protein family and antibodies that tend to have noisy MSAs due to fast evolution.
OmegaFold combines a large pretrained language model for sequence modeling and a geometry-inspired transformer. It learns single- and pairwise-residue embeddings. A stack of Geoformer layers then iteratively updates these embeddings to improve their geometric consistency.

□ HYFA: Hypergraph factorisation for multi-tissue gene expression imputation
>> https://www.biorxiv.org/content/10.1101/2022.07.31.502211v1.full.pdf
HYFA (Hypergraph Factorisation), a parameter-efficient graph representation learning approach for joint multi-tissue and cell-type GE imputation. Through transfer learning on a paired single-nucleus RNA-seq dataset (GTEx-v9), HYFA resolves cell-type signatures from bulk GE.
HYFA imputes tissue-specific GE via a specialised graph neural network operating on a hypergraph of metagenes. HYFA is genotype-agnostic, supports a variable number of collected tissues, and imposes strong inductive biases to leverage the shared regulatory architecture.

□ HiCoEx: Prediction of Gene Co-expression from Chromatin Contacts with Graph Attention Network
>> https://academic.oup.com/bioinformatics/advance-article/doi/10.1093/bioinformatics/btac535/6656345
HiCoEx, a novel machine learning framework based on graph neural network HiCoEx is able to automatically capture important patterns for the prediction of co-expression from chromosomal contacts between genes, and visualize the gene-gene interactions for mechanistic exploration.
HiCoEx calculates topological properties incl. Clustering Coefficient, Jaccard Index and Shortest path length. Pearson Correlation Coefficient (PCC) about each topological property is computed between the genes and their neighborhoods in the embedding space.

□ GIANT: A unified analysis of atlas single cell data
>> https://www.biorxiv.org/content/10.1101/2022.08.06.503038v1.full.pdf
GIANT integrates multi-modality and multi-tissue data. GIANT first converts datasets from different modalities into gene graphs, and then recursively embeds genes in the graphs into a latent space without additional alignment.
A dendrogram is then built to connect the gene graphs in a hierarchy. In recursive projection, a dendrogram is used to enforce similarity constraints across graphs while still allowing genes with multiple functions to be projected to different locations in the embedding space.

□ Exact polynomial-time isomorphism testing in directed graphs through comparison of vertex signatures in Krylov subspaces.
>> https://www.biorxiv.org/content/10.1101/2022.07.28.501884v1.full.pdf
Graph Krylov subspaces, which contain products of vectors and exponentiated adjacency matrices, are closely related to the tensor of eigenprojections, presenting an related avenue for isomorphism research.
Recursive exponentiation may also cause either vanishing or explosive growth of Krylov matrix elements. This problem may be addressed in some cases by normalising vectors.
A “vertex signature” is defined by initialising a Krylov matrix with a binary vector indicating the vertex position. the isomorphic mapping may be constructed iteratively o(n^5) time by building a set of vertex analogies sequentially.

□ Hierarchical Interleaved Bloom Filter: Enabling ultrafast, approximate sequence queries
>> https://www.biorxiv.org/content/10.1101/2022.08.01.502266v1.full.pdf
The HIBF data structure has enormous potential. It can be used on its own like in the tool Raptor, or can serve as a prefilter to distribute more advanced analyses such as read mapping.
Since the build time exceeds two orders of magnitude less than that of comparable tools like Mantis and Bifrost, the HIBF can easily be rebuilt even for huge data sets.
The HIBF builds an index up to 211 times faster, using up to 14 times less space and can answer approximate membership queries faster by a factor of up to 129. This can be considered a quantum leap that opens the door to indexing complete sequence.

□ ZetaSuite: computational analysis of two-dimensional high-throughput data from multi-target screens and single-cell transcriptomics
>> https://genomebiology.biomedcentral.com/articles/10.1186/s13059-022-02729-4
Zeta is Z-based estimation of global splicing regulators. Zeta statistics can maximally segregate high-quality cells from damaged ones while minimize unwanted artifacts. ZetaSuite is a computational framework initially developed to process the data from a siRNA screen.
ZetaSuite generates a Z-score for each AS event against each targeting RNA in the data matrix and then computes the number of hits at each Z-score cutoff from low to high and in both directions to separately quantify induced exon skipping or inclusion events.

□ Tensor Decomposition Discriminates Tissues Using scATAC-seq
>> https://www.biorxiv.org/content/10.1101/2022.08.04.502875v1.full.pdf
Tensor Decomposition to an scATAC-seq data set and the obtained embedding can be used for UMAP, following which the embedded material obtained by UMAP can differentiate tissues from which the scATAC sequence was retrieved.
Applying UPGMA (unweighted pair group method with arithmetic mean) to negatively signed correlation coefficients. TD can deal with large sparse data sets generated by approximately 200 bp intervals and this number can be as high as 13,627,618, as these can be stored in a sparse matrix format.

□ CIARA: a cluster-independent algorithm for the identification of markers of rare cell types from single-cell RNA seq data
>> https://www.biorxiv.org/content/10.1101/2022.08.01.501965v1.full.pdf
CIARA (Cluster Independent Algorithm for the identification of markers of RAre cell types) identifies potential marker genes of rare cell types by exploiting their property of being highly expressed in a small number of cells with similar transcriptomic signatures.
CIARA ranks genes based on their enrichment in local neighborhoods defined from a K-nearest neighbors (KNN) graph. The top-ranked genes have, thus, the property of being “highly localized” in the gene expression space.

□ ASURAT: Functional annotation-driven unsupervised clustering of single-cell transcriptomes
>> https://academic.oup.com/bioinformatics/advance-article/doi/10.1093/bioinformatics/btac541/6655687
ASURAT, a computational tool for simultaneously performing unsupervised clustering and functional annotation of biological process, and signaling pathway activity for transcriptomic data, using a correlation graph decomposition for genes in database-derived functional terms.
ASURAT creates sign-by-sample matrices (SSMs). SSM is analogous to a read count table, where the rows represent signs with biological meaning instead of individual genes and the values contained are “sign scores” instead of read counts.
Since ASURAT can create multivariate data (i.e., SSMs) from multiple signs, ranging from cell types to biological functions, it will be valuable to consider graphical models of signs.
A non-Gaussian Markov random field theory is one of the most promising approaches to address this problem, although requires a large number of samples for achieving true graph edges.

□ Metheor: Ultrafast DNA methylation heterogeneity calculation from bisulfite read alignments
>> https://www.biorxiv.org/content/10.1101/2022.07.20.500893v1.full.pdf
The main algorithmic advantage of Metheor comes from the fact that it only reads through the entire BAM file only once. Reduced representation bisulfite sequencing (RRBS) predominantly targets the CpG-dense regions. This read-centric approach iterates through aligned reads.
Metheor produces methylation heterogeneity levels accurately. Metheor supports Computation of local pairwise methylation discordance (LPMD). LPMD is defined as a fraction of CpG pairs within a given range of genomic distance. LPMD does not depend on length of sequencing read.

□ Asteroid: a new minimum balanced evolution supertree algorithm robust to missing data
>> https://www.biorxiv.org/content/10.1101/2022.07.22.501101v1.full.pdf
Asteroid, a novel supertree method that infers an unrooted species tree from a set of unrooted gene trees. Asteroid is more robust to missing data than ASTRAL and ASTRID, while being several orders of magnitude faster than ASTRAL for datasets that contain thousands of genes.
Asteroid computes for each input gene tree a distance matrix based on the gene internode distance. Then, it computes a species tree from this set of distance matrices under the minimum balanced evolution principle.

□ scMTNI: Inference of cell type-specific gene regulatory networks on cell lineages from single cell omic datasets
>> https://www.biorxiv.org/content/10.1101/2022.07.25.501350v1.full.pdf
scMTNI (single-cell Multi-Task Network Inference), a multi-task learning framework that integrates the cell lineage structure, scRNA-seq and scATAC-seq measurements to enable joint inference of cell type-specific GRNs.
scMTNI uses a novel probabilistic prior to incorporate the lineage structure and outputs GRNs for each cell type on a cell lineage. The output networks of scMTNI are analyzed using two dynamic network analysis methods: edge-based k-means clustering and topic models.

□ HAlign 3: fast multiple alignment of ultra-large numbers of similar DNA/RNA sequences
>> https://academic.oup.com/mbe/advance-article/doi/10.1093/molbev/msac166/6653123
HAlign 3 improves the time efficiency and the alignment quality. The suffix tree data structure is specifically modified to fit the nucleotide sequence: Left-child right-sibling is replaced by a K-ary tree to build the suffix tree to reach a higher common substring searching efficiency.
A global substring selection algorithm combining directed acyclic graphs with dynamic programming is adopted to screen out the unsatisfactory common substrings. These improvements make HAlign 3 a specialized program to deal with ultra-large numbers of similar DNA/RNA sequences.

□ MGREML: Multivariate estimation of factor structures of complex traits using SNP-based genomic relationships
>> https://bmcbioinformatics.biomedcentral.com/articles/10.1186/s12859-022-04835-3
MGREML estimates multivariate factor structures and perform inferences on factor models at low computational cost. It enables simple structural equation modeling using MGREML, allowing to specify, estimate, and compare genetic factor models of their choosing using SNP data.
MGREML calculates the contribution of any given block in O(T^2) time. MGREML transforms the data, and reorders the variance matrix is block diagonal. Using a Broyden–Fletcher–Goldfarb–Shanno algorithm, it balances computational complexity & rate of convergence across iterations.

□ GE-Impute: graph embedding-based imputation for single-cell RNA-seq data
>> https://academic.oup.com/bib/advance-article-abstract/doi/10.1093/bib/bbac313/6651303
GE-Impute learns the neural graph representation for each cell and reconstructs the cell–cell similarity network accordingly, which enables better imputation of dropout zeros based on the more accurately allocated neighbors in the similarity network.
GE-Impute constructs a raw cell-cell similarity network based on Euclidean distance. For each cell, it simulates a random walk of fixed length using BFS and DFS strategy.
Next, graph embedding-based neural network was employed to train the embedding matrix for each cell based on sampling walks. The similarity among cells could be re-calculated from embedding matrix to predict new link-neighbors and reconstruct cell-cell similarity network.

□ DeepST: A versatile graph contrastive learning framework for spatially informed clustering, integration, and deconvolution of spatial transcriptomics
>> https://www.biorxiv.org/content/10.1101/2022.08.02.502407v1.full.pdf
Spatial contrastive self-supervised learning enables the learned spatial spot representation to be more informative and discriminative by minimizing the embedding distance between spatially adjacent spots and vice versa.
DeepST learns a mapping matrix to project the scRNA-seq data into the ST space based on their learned features via a contrastive learning mechanism where the similarities of spatially neighboring spots are maximized and those of spatially non-neighboring spots are minimized.

□ Exploring Phylogenetic Classification and Further Applications of Codon Usage Frequencies
>> https://www.biorxiv.org/content/10.1101/2022.07.20.500846v1.full.pdf
GridSearchCV was used to search over hyperparameters. Using the sparse categorical crossentropy loss function, the adam optimizer, 5 fold CV, 15 epochs, a validation split of 0.1 the code chose the number of layers, neurons in each layer, and the l2 penalty for regularization.

□ A quaternion model for single cell transcriptomics
>> https://www.biorxiv.org/content/10.1101/2022.07.21.501020v1.full.pdf
Quaternions are four dimensional hypercomplex numbers that, along with real numbers, complex numbers and octonions, represent one of the four normed division algebras.
The quaternion associated with each cell represents a vector in R3 with vector length capturing sequencing depth and vector direction capturing the relative expression profile.
The proposed scRNA-seq quaternion model enables the spectral analysis scRNA-seq data relative to a single variable (e.g., pseudo-time) or two variables to be performed on a genome-wide basis by used a one or two-dimensional hypercomplex Fourier transformation.
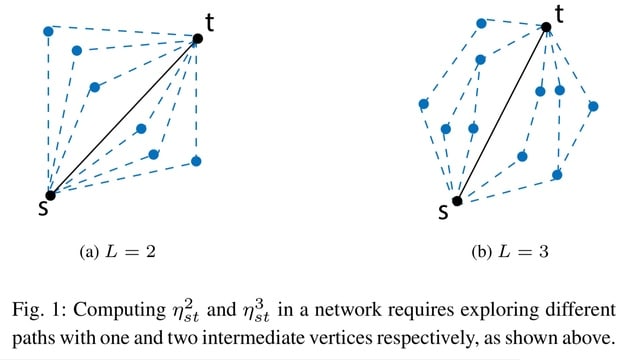
□ MCPNet : A parallel maximum capacity-based genome-scale gene network construction framework
>> https://www.biorxiv.org/content/10.1101/2022.07.19.500603v1.full.pdf
MCP Score, a novel maximum-capacity-path based metric to quantify the relative strengths of direct and indirect gene-gene interactions. MCPNet, an efficient, parallelized GRN reconstruction software that can scale to hundreds of cores.
The maximum capacity of all stlength-L paths can be computed via recursive path bisection. The recursive path bisection allows to be computed in O(|V| log2 L) for a single gene-gene pair, and the long range DPI scores for all gene pairs to be computed in O(|V |3log2 L) time.

□ LanceOtron: a deep learning peak caller for genome sequencing experiments
>> https://academic.oup.com/bioinformatics/advance-article/doi/10.1093/bioinformatics/btac525/6648462
LanceOtron combines deep learning for recognizing peak shape with multifaceted enrichment calculations for assessing significance. In benchmarking ATAC-seq, ChIP-seq, and DNase-seq, LanceOtron outperforms long-standing peak callers through its near perfect sensitivity.
LanceOtron uses the relationship b/n the number of overlapping reads and their relative positions at all 2,000 points, returning a shape score. A multilayer perceptron combines the CNN and logistic regression models, to produce an overall peak quality metric called Peak Score.

□ SpatialSort: A Bayesian Model for Clustering and Cell Population Annotation of Spatial Proteomics Data
>> https://www.biorxiv.org/content/10.1101/2022.07.27.499974v1.full.pdf
SpatialSort has the ability to accounts for the affinities of cells of different types to neighbour in space. By incorporating prior information about expected cell populations, SpatialSort is able to improve clustering accuracy and perform automated annotation of clusters.
SpatialSort models cell labels using an Hidden Markov Random Field (HMRF). SpatialSort takes the cell location and neighbour relations to construct sample-specific cell connectivity graphs that link cells that are spatially proximal.

□ Deep R-looper Discriminant: Cell-type-specific aberrant R-loop accumulation regulates target gene and confers cell-specificity
>> https://www.biorxiv.org/content/10.1101/2022.07.19.500727v1.full.pdf
Deep R-looper Discriminant, a deep neural network-based framework for extracting features automatically from epigenetic marks in genome bins around TSS and TTS and identifying aberrant R-loops against normal R-loops.
Deep R-looper Discriminant adoptes GridSearch CV to automate the tuning of hyperparameters for these baseline models and finally got optimized k-nearest neighbors (KNN), linear discriminant analysis (LDA), logistic regression (LR), naive bayes (NB), and random forests (RF).

□ HAT: Haplotype Assembly Tool using short and error-prone long reads
>> https://www.biorxiv.org/content/10.1101/2022.07.20.500775v1.full.pdf
HAT, a haplotype assembly tool that exploits short and long reads along with a reference genome to reconstruct haplotypes. HAT tries to take advantage of the accuracy of short reads and the length of the long reads to reconstruct haplotypes.
HAT comprises 3 components - initialization, iteration and assembly. Initialization creates the first phased blocks. The iteration expands the phased blocks and finds alleles of all haplotypes. Then, HAT clusters the reads, and assembles haplotypes using these clustered reads.

□ scDEC-Hi-C: Deep generative modeling and clustering of single cell Hi-C data
>> https://www.biorxiv.org/content/10.1101/2022.07.19.500573v1.full.pdf
scDEC-Hi-C is a novel end-to-end deep learning framework for analyzing single cell Hi-C data using a multi-stage model. scDEC-Hi-C consists of a chromosome-wise autoencoder (AE) model and a cell-wise deep embedding and clustering model (scDEC).
Note that all baseline methods are only able to learn the embedding for each single cell and require additional clustering methods (e.g, K-means) while scDEC-Hi-C simultaneously learns cell embeddings and assigns clustering labels to each cell.
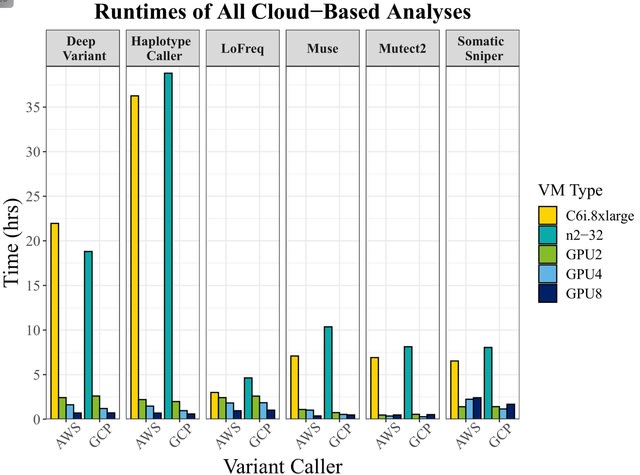
□ Accelerating genomic workflows using NVIDIA Parabricks
>> https://www.biorxiv.org/content/10.1101/2022.07.20.498972v1.full.pdf
Achieving up to 65x acceleration, bringing HaplotypeCaller runtime down from 36 hours to 33 minutes on AWS, 35 minutes on GCP, and 24 minutes on the NVIDIA DGX.
Alternatively, somatic variant callers achieved speedups up to 56.8x for the Mutect2 algorithm, but surprisingly, did not scale linearly with the number of GPUs, emphasizing the need for algorithmic benchmarking before embarking on large-scale projects.

□ BiGCARP: Deep self-supervised learning for biosynthetic gene cluster detection and product classification
>> https://www.biorxiv.org/content/10.1101/2022.07.22.500861v1.full.pdf
Biosynthetic Gene CARP (BiGCARP) represents BGCs as chains of functional protein domains, and uses ESM-1b, a protein masked language model, to obtain pretrained embeddings of functional protein domains with amino acid-level context.
A convolutional masked language model on these domains to develop meaningful learned representations of BGCs and their constituent domains. BiGCARP-random is initialized with a random Pfam embedding.

□ BWA-MEME: BWA-MEM emulated with a machine learning approach
>> https://academic.oup.com/bioinformatics/article-abstract/38/9/2404/6543607
BWA-MEME, the first full-fledged short read alignment software that leverages learned indices for solving the exact match search problem for efficient seeding.
BWA-MEME is a practical and efficient seeding algorithm based on a suffix array search algorithm that solves the challenges in utilizing learned indices for SMEM search which is extensively used in the seeding phase.

□ ATAC-STARR-seq reveals transcription factor-bound activators and silencers across the chromatin accessible human genome
>> https://genome.cshlp.org/content/early/2022/07/18/gr.276766.122
A new workflow that substantially expands the capabilities of ATAC- STARR-seq to extract and measure gene regulatory information. This workflow identifies both activators and silencers, as well as to simultaneously profile chromatin accessibility, and perform TF footprinting.
Adapting a modified tagmentation protocol (Omni-ATAC) to remove mitochondrial DNA from the DNA fragment pool.
The re-isolation of plasmid DNA recovers only the ATAC-STARR-seq plasmids that were successfully transfected, thus providing a more accurate representation of the “input” sample than sequencing without transfection.
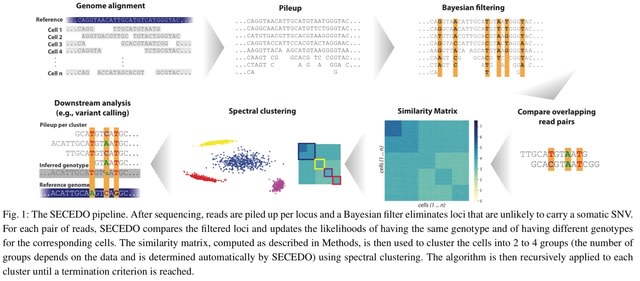
□ SECEDO: SNV-based subclone detection using ultra-low coverage single-cell DNA sequencing
>> https://academic.oup.com/bioinformatics/advance-article/doi/10.1093/bioinformatics/btac510/6651099
\
The pivotal blocks in the SECEDO pipeline are a Bayesian filtering strategy for efficient identification of relevant loci and derivation of a global cell-to-cell similarity matrix utilizing both the structure of reads and the haplotype phasing.
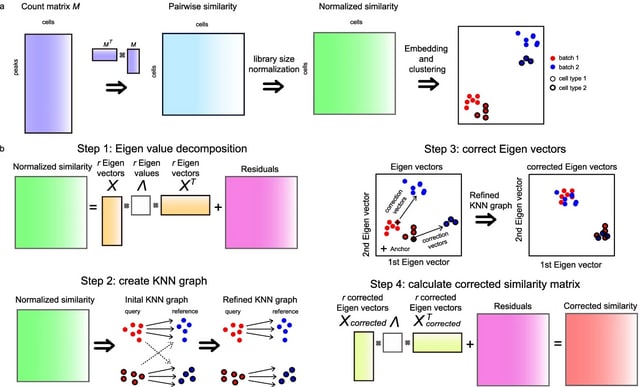
□ epiConv: Joint analysis of scATAC-seq datasets
>> https://bmcbioinformatics.biomedcentral.com/articles/10.1186/s12859-022-04858-w
epiConv is capable of aligning low-depth scATAC-Seq from co-assay data (simultaneous profiling of transcriptome and chromatin) onto high-quality ATAC-seq reference and increasing the resolution of chromatin profiles of co-assay data.
epiConv directly calculates the similarities between cells without embedding them into the latent feature space. epiConv can be used to integrate cells from different biological conditions, which reveals hidden cell populations that would otherwise be undetectable.

□ BMRF: Probabilistic Edge Inference of Gene Networks with Bayesian Markov Random Field Modelling
>> https://www.biorxiv.org/content/10.1101/2022.07.30.501645v1.full.pdf
This method combines the Bayesian Markov Random field model and conditional autoregressive model for the relationship between gene nodes. This analysis can evaluate the relative strength of the edges and further prioritize the edges of interest.
The proposed BMRF model was compared with M&B, Glasso, SPACE, and CLIME, as well as with the Bayesian approach BDgraph using the Bayesian model averaging procedure (denoted as BD_BMA) or the Maximum a posterior probability procedure.

□ HiCAT: A tool for automatic annotation of centromere structure
>> https://www.biorxiv.org/content/10.1101/2022.08.07.502881v1.full.pdf
HiCAT, a generalizable automatic centromere annotation tool, based on hierarchical tandem repeat mining and maximization of tandem repeat coverage to facilitate decoding of centromere architecture.
HiCAT transforms a centromere DNA sequence into a block list based on an input monomer template. HiCAT defines a similarity score based on the block edit distance to obtain a block similarity matrix. HiCAT detects LN-HORs using the Hierarchical Tandem Repeat Mining.
※コメント投稿者のブログIDはブログ作成者のみに通知されます