
(Created with Midjourney v6 ALPHA)
□ ÆSTRAL / “Freedom”
ÆSTRALはドイツのIDM・Trap Musicクリエイターで、シネマティックで重厚なトラックメイキングとエレクトロニカを融合させたスタイル。Hans Zimmerの同名曲のカバー、”Freedom”は、Lisa Gerrardのコーラスが天上に響き渡るような壮大なスケールを感じさせる

□ scHolography: a computational method for single-cell spatial neighborhood reconstruction and analysis
>> https://genomebiology.biomedcentral.com/articles/10.1186/s13059-024-03299-3
scHolography trains neural networks to perform the high-dimensional transcriptome-to-space (T2S) projection. scHolography utilizes post-integration ST expression data as training input and SIC values as training targets for generating the T2S projection model.
scHolography learns inter-pixel spatial affinity and reconstructs single-cell tissue spatial neighborhoods. scHolography determines spatial dynamics of gene expression. The spatial gradient is defined as gene expression changes along the Stable-Matching Neighbors (SMN) distances.

□ G4-DNABERT: Analysis of live cell data with G-DNABERT supports a role for G-quadruplexes in chromatin looping
>> https://www.biorxiv.org/content/10.1101/2024.06.21.599985v1
G4-DNABERT employs fine-tuning DNABERT model trained on 6-mers representation of DNA sequence and used 512 bp context length. It learns not only regular sequence pattern but implicit patterns in loops and implicit patterns of adjacent flanks as one can see in attention maps.
G4-DNABERT revealed statistically significant enrichment of G4s in proximal (8.6-fold) and distal (1.9-fold) enhancers. G4-DNABERT revealed statistically significant enrichment of G4s in proximal (8.6-fold) and distal (1.9-fold) enhancers.

□ Φ-Space: Continuous phenotyping of single-cell multi-omics data
>> https://www.biorxiv.org/content/10.1101/2024.06.19.599787v1
Φ-Space, a computational framework for the continuous phenotyping of single-cell multi-omics data. Φ-Space adopts a highly versatile modelling strategy to continuously characterise query cell identity in a low-dimensional phenotype space, defined by reference phenotypes.
Φ-Space characterises developing and out-of-reference cell states; Φ-Space is robust against batch effects in both reference and query; Φ-Space adapts to annotation tasks involving multiple omics types; Φ-Space overcomes technical differences between reference and query.
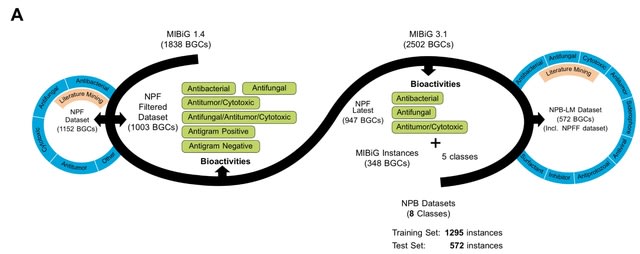

□ NPBdetect: Predicting biological activity from biosynthetic gene clusters using neural networks
>> https://www.biorxiv.org/content/10.1101/2024.06.20.599829v1
NPBdetect is built through rigorous experiments. NPBdetect improves data standardization by composing two datasets, one training and one test set which is inspired by contemporary datasets in Al. Minimum Information about a Biosynthetic Gene Cluster is utilized.
NPBdetect includes assessing the Natural Product Function (NPF) descriptors to select the best one(s) to build the model, using the latest antiSMASH tool for annotations, and integrating new sequence-based descriptors.

□ singletCode: Synthetic DNA barcodes identify singlets in scRNA-seq datasets and evaluate doublet algorithms
>> https://www.cell.com/cell-genomics/fulltext/S2666-979X(24)00176-9
singletCode, a DNA barcode analysis approach for a new application: identifying “true” singlets in scRNA-seq datasets. Since DNA barcoding allows for individual cells to have a unique identifier prior to scRNA-seq protocols, these barcodes could help identify “true” singlets.
singletCode provides a framework to identify ground-truth singlets for downstream analysis. Alternatively, singletCode itself can be leveraged to systematically test the performance of different doublet detection methods in scRNA-seq and other modalities.

□ NmTHC: a hybrid error correction method based on a generative neural machine translation model with transfer learning
>> https://bmcgenomics.biomedcentral.com/articles/10.1186/s12864-024-10446-4
NmTHC reasonably adopts the generative Neural Machine Translation (NMT) model to transform hybrid error correction tasks into machine translation problems and provides a perspective for solving long-read error correction problems with the ideas of Natural Language Processing.
NmTHC employs a seq2seq-based generative framework to address the bottleneck of unequal input and output lengths. Consequently, NmTHC breaks through the finite state space of HMMs and capture context to fix those unaligned regions.

□ DDN3.0: Determining significant rewiring of biological network structure with differential dependency networks
>> https://academic.oup.com/bioinformatics/advance-article/doi/10.1093/bioinformatics/btae376/7696711
DDN3.0 (Differential Dependency Network) uses fused Lasso regression to jointly learn the common and rewired network structures. DDN3.0 replaces the inner products among data vectors w/ the pre-calculated equivalent and corresponding correlation coefficients, termed BCD-CorrMtx.
DDN3.0 employs unbiased model estimation with a weighted error-measure applicable to imbalanced sample groups, multiple acceleration strategies to improve learning efficiency, and data-driven determination of proper hyperparameters.
DDN3.0 reformulates the original objective function by assigning a sample-size-dependent normalization factor to the error measure on each group, which effectively equalizes the contributions of different groups to the overall error-measure.

□ TransfoRNA: Navigating the Uncertainties of Small RNA Annotation with an Adaptive Machine Learning Strategy
>> https://www.biorxiv.org/content/10.1101/2024.06.19.599329v1
TransfoRNA is a machine learning framework based on Transformers that explores an alternative strategy. It uses common annotation tools to generate a small seed of high-confidence training labels, while then expanding upon those labels iteratively.
TranstoRNA learns sequence-specific representations of all RNAs to construct a similarity network which can be interrogated as new RNAs are annotated, allowing to rank RNAs based on their familiarity.
TransfoRNA encodes input RNA sequences (or structures) into a vector representation (i.e. embedding) that is then used to classify the sequence as an RNA class. Each RNA sequence is encoded into a fixed-length vectorized form, which involves a tokenization step.

□ OM2Seq: Learning retrieval embeddings for optical genome mapping
>> https://academic.oup.com/bioinformaticsadvances/advance-article/doi/10.1093/bioadv/vbae079/7688356
OM2Seq, a new approach for accurate mapping of DNA fragment images to a reference genome. Based on a Transformer-encoder architecture, OM2Seq is trained on acquired OGM data to efficiently encode DNA fragment images and reference genome segments into a unified embedding space.
OM2Seq is composed of two Transformer-encoders: one dubbed the Image Encoder, tasked with encoding DNA molecule images into embedding vectors, and another called the Genome Encoder, devoted to transforming genome sequence segments into their embedding vector counterparts.

□ node2vec2rank: Large Scale and Stable Graph Differential Analysis via Multi-Layer Node Embeddings and Ranking
>> https://www.biorxiv.org/content/10.1101/2024.06.16.599201v1
node2vec2rank, a method for graph differential analysis that ranks nodes according to the disparities of their representations in joint latent embedding spaces. Node2vec2rank uses a multi-layer node embedding algorithm to create two sets of vector representations for all genes.
For every gene, n2v2r computes the disparity between its two representations, which is then used to rank the genes in descending order of disparities. The process is repeated multiple times, producing different embedding spaces and ranking based on different distance metrics.

□ BiomiX: a User-Friendly Bioinformatic Tool for Automatized Multiomics Data Analysis and Integration
>> https://www.biorxiv.org/content/10.1101/2024.06.14.599059v1
BiomiX provides robust, validated pipelines in single omics with additional functions, such as sample subgrouping analysis, gene ontology, annotation, and summary figures. BiomiX implements MOFA, allowing for an automatic selection of the total number of factors and the identification of the biological processes behind the factors of interest through clinical data correlation and pathway analysis.
BiomiX implemented, for the first time, the factor identification through an automatic bibliography research on Pubmed, underlining the importance of integrating literature knowledge in the interpretation of MOFA factors.

□ Squigulator: Simulation of nanopore sequencing signal data with tunable parameters
>> https://genome.cshlp.org/content/34/5/778.full
Squigulator (squiggle simulator), a fast and simple tool for in silico generation of nanopore current signal data that emulates the properties of real data from a nanopore device.
Squigulator uses existing ONT pore models, which model the expected current level as a given DNA/RNA subsequence occupies a nanopore, and applies empirically determined noise functions to generate realistic signal data from a reference sequence/s.
Squigulator can adjust the noise parameters; DNA translocation speed, data acquisition rate; and pseudoexperimental variables. This capacity for deterministic parameter control is an important advantage of Squigulator, enabling parameter exploration during algorithm development.

□ iProL: identifying DNA promoters from sequence information based on Longformer pre-trained model
>> https://bmcbioinformatics.biomedcentral.com/articles/10.1186/s12859-024-05849-9
iProL utilizes the Longformer pre-trained model with attention mechanism as the embedding layer, then uses CNN and BiLSTM to extract sequence local features and long-term dependency information, and finally obtains the prediction results through two fully connected layers.
iProL receives 81-bp long DNA sequences, split into 2-mer nucleotide segments. iProL uses the pre-trained model named "longformer-base-4096", which supports text sequences up to a maximum length of 4096 and can embed each word into a vector of 768 dimensions.

□ STHD: probabilistic cell typing of single Spots in whole Transcriptome spatial data with High Definition
>> https://www.biorxiv.org/content/10.1101/2024.06.20.599803v1
The STHD model leverages cell type-specific gene expression from reference single-cell RNA-seq data, constructs a statistical model on spot gene counts, and employs regularization from neighbor similarity. STHD implements fast optimization enabled by efficient gradient descent. STHD outputs cell type probabilities and labels based on Maximum a Posterior.

□ FastHPOCR: Pragmatic, fast and accurate concept recognition using the Human Phenotype Ontology
>> https://academic.oup.com/bioinformatics/advance-article/doi/10.1093/bioinformatics/btae406/7698025
FastHPOCR is a phenotype concept recognition package using the Human Phenotype Ontology to extract concepts from free text. The solution relies on the fundamental pillars of concept recognition.
FastHPOCR relies on a collection of clusters of morphologically-equivalent tokens aimed at addressing lexical variability and on a closed-world assumption applied during concept recognition to find candidates and perform entity linking.

□ ESM3: A frontier language model for biology
>> https://www.evolutionaryscale.ai/blog/esm3-release
ESM3, the first generative model for biology that simultaneously reasons over the sequence, structure, and function of proteins. ESM3 is trained across the natural diversity of the Earth—billions of proteins.
ESM3 is a multi-track transformer that jointly reasons over protein sequence, structure, and function. ESM3 is trained with over 1x10^24 FLOPS and 98B parameters. ESM3 can be thought of as an evolutionary simulator.

一瞬なんでケネディ国際空港のターミナルでカンファレンスやってんのかなって思ったけど良く見たら違った…🫣
Showcase Event in San Francisco. It was an incredible evening of connecting with the biotech/techbio community, learning about the latest advances in the field from startups (including an ESM3 demo) to industry
>> https://x.com/shantenuagarwal/status/1806784991827014034

□ GENTANGLE: integrated computational design of gene entanglements
>> https://academic.oup.com/bioinformatics/advance-article/doi/10.1093/bioinformatics/btae380/7697098
GENTANGLE (Gene Tuples ArraNGed in overLapping Elements) is a high performance containerized pipeline for the computational design of two overlapping genes translated in different reading frames of the genome that can be used to design and test gene entanglements.
GENTANGLE uses CAMEOX, which is responsible for generating candidate entanglement solutions. CAMEOX introduces multi-thread parallelism and a dynamic stopping criterion. Each entanglement candidate sequence is modified for predicted fitness over different numbers of iterations.

□ SE3Set: Harnessing equivariant hypergraph neural networks for molecular representation learning
>> https://arxiv.org/abs/2405.16511
In computational chemistry, hypergraph algorithms simulate complex behaviors and optimize molecules through hypergraph grammar, providing multidimensional insights into molecular structures.
SESet, an innovative approach that enhances traditional GNNs by exploiting hypergraphs for modeling many-body interactions, while ensuring SE(3) equivariant representations that remain consistent regardless of molecular orientation.
SE3Set begins with node and hyperedge embeddings, cycles through V2E and E2V attention modules for iterative updates, and concludes with normalization and a feed-forward block. Atomic numbers and position vectors are transformed into initial embeddings for nodes and hyperedges.

□ CELLULAR: Contrastive Learning for Robust Cell Annotation and Representation from Single-Cell Transcriptomics
>> https://www.biorxiv.org/content/10.1101/2024.06.20.599868v1
CELLULAR (CELLUlar contrastive Learning for Annotation and Representation) leverages single-cell RNA sequencing data to train a deep neural network to produce an efficient, lower-dimensional, generalizable embedding space.
CELLULAR consists of a feed-forward encoder w/ 2 linear layers, each followed by normalization and a ReLU activation. The encoder is designed to compress the input after each layer, ending w/ a final embedding space of dimension 100. CELLULAR contains 2,558,600 learnable weights.

□ kISS: Efficient Construction and Utilization of k-Ordered FM-indexe for Ultra-Fast Read Mapping in Large Genomes
>> https://academic.oup.com/bioinformatics/advance-article/doi/10.1093/bioinformatics/btae409/7696319
kISS represents a sophisticated solution specifically engineered to optimize both time and space efficiency during the construction of k-ordered suffix arrays. This method leverages the ability to efficiently identify short seed sequences within large reference genomes.
kISS facilitates the creation of k-ordered FM-indexes, as initially proposed by sBWT, by using k-ordered suffix arrays. kISS enables the effective integration of these k-ordered FM-indexes with the FMtree's location function.
kISS takes a direct approach by sorting all left-most S-type (LMS) suffixes. This enhances parallelism and takes advantage of the speed improvements inherent in k-ordered concepts.

□ BioKGC: Path-based reasoning in biomedical knowledge graphs
>> https://www.biorxiv.org/content/10.1101/2024.06.17.599219v1
BioKGC, a novel graph neural network framework which builds upon the Neural Bellman-Ford Network (NBFNet). BioKGC employs neural formulations, specifically message passing GNNs, to learn path representations.
BioKGC incorporates a background regulatory graph (BRG) that adds additional connections between genes. This supplementary knowledge is leveraged for message passing, enhancing the information flow beyond the edges used for supervised training.
BioKGC learns representations between nodes by considering all relations along paths. It enhances prediction accuracy and interpretability, allowing for the visualization of influential paths and facilitating the validation of biological plausibility.

□ Hapsolutely: a user-friendly tool integrating haplotype phasing, network construction, and haploweb calculation
>> https://academic.oup.com/bioinformaticsadvances/article/doi/10.1093/bioadv/vbae083/7688355
Hapsolutely integrates phasing and graphical reconstruction steps of haplotype networks, and calculates and visualizes haplowebs and fields for re-combination, thus allowing graphical comparison of allele distribution and allele sharing for the purpose of species delimitation.
Hapsolutely facilitates the exploration of molecular differentiation across species partitions. The program be helpful to inspect and visualize concordant differentiation of lineages across markers or discordance based, for instance, on incomplete lineage sorting.

□ DeEPsnap: human essential gene prediction by integrating multi-omics data
>> https://www.biorxiv.org/content/10.1101/2024.06.20.599958v1
DeEPsnap integrates features from 5 omics data, incl. features derived from nucleotide sequence and protein sequence data, features learned from the PPI network, features encoded using GO enrichment scores, features from protein complexes, and features from protein domain data.
DeEPsnap uses a new cyclic learning method for our essential gene prediction problem. DeEPsnap can accurately predict human essential genes. The enrichment score is calculated as -log10 for each GO term. In this way, DeEPsnap gets a 100-dimension feature vector for each gene.

□ Genopyc: a python library for investigating the functional effects of genomic variants associated to complex diseases
>> https://academic.oup.com/bioinformatics/advance-article/doi/10.1093/bioinformatics/btae379/7695869
Genopyc allows to perform various tasks such as retrieve the functional elements neighbouring genomic coordinates, investigating linkage disequilibrium (LD), annotate variants, retrieving genes affected by non coding variants and perform and visualize functional enrichment analysis.
Genopyc also queries the variant effect predictor (VEP) to obtain the consequences of the SNPs on the transcript and its effect on neighboring genes and functional elements. Therefore, it is possible to retrieve the eQTL related to variants through the eQTL Catalogue.
Genopyc integrates the locus to gene (L2G) pipeline from Open Target Genetics. Genopyc can retrieve a linkage-disequilibrium (LD) matrix for a set of SNPs by using LDlink, convert genome coordinates between genome versions and retrieve genes coordinates in the genome.

□ SCIPIO-86: Beyond benchmarking and towards predictive models of dataset-specific single-cell RNA-seq pipeline performance
>> https://genomebiology.biomedcentral.com/articles/10.1186/s13059-024-03304-9
Single Cell pIpeline PredIctiOn (SCIPIO-86), represents the first dataset of single-cell pipeline performance comprising 4 corrected metrics across 24,768 dataset-pipeline pairs.
The performance of the analysis pipelines were dependent on the dataset, providing additional motivation to model pipeline performance as a function of dataset-specific characteristics and pipeline parameters.
Intriguingly, dataset-specific recommendations result in higher prediction accuracy when predicting the metrics themselves but not necessarily when considering whether predictions align with prior clustering results.

□ PxBLAT: an efficient python binding library for BLAT
>> https://bmcbioinformatics.biomedcentral.com/articles/10.1186/s12859-024-05844-0
PxBLAT, a Python-based framework designed to enhance the capabilities of BLAST-like alignment tool (BLAT). PxBLAT delivers its query results in alignment with the QueryResult class of Biopython, enabling seamless manipulation of query outputs. PxBLAT negates the necessity for intermediate files by conducting all operations in memory.

□ Phyloformer: Fast, accurate and versatile phylogenetic reconstruction with deep neural networks
>> https://www.biorxiv.org/content/10.1101/2024.06.17.599404v1
Phyloformer is a fast deep neural network-based method to infer evolutionary distance from a multiple sequence alignment. It can be used to infer alignments under a selection of evolutionary models: LG+GC, LG+GC with indels, CherryML co-evolution model and SelReg with selection.
Phyloformer is a learnable function for reconstructing a phylogenetic tree from an MSA representing a set of homologous sequences. It produces an estimate, under a chosen probabilistic model, of the distances between all pairs of sequences.

□ PathoLM: Identifying pathogenicity from the DNA sequence through the Genome Foundation Model
>> https://www.biorxiv.org/content/10.1101/2024.06.18.599629v1
PathoLM, a genome modeling tool that uses the pre-trained Nucleotide Transformer v2 50M for enhanced pathogen detection in bacterial and viral genomes, both improving accuracy and addressing data limitations.
Leveraging the strengths of pre-trained DNA models such as the Nucleotide Transformer, PathoLM requires minimal data for fine-tuning. It effectively captures a broader genomic context, significantly improving the identification of novel and divergent pathogens.
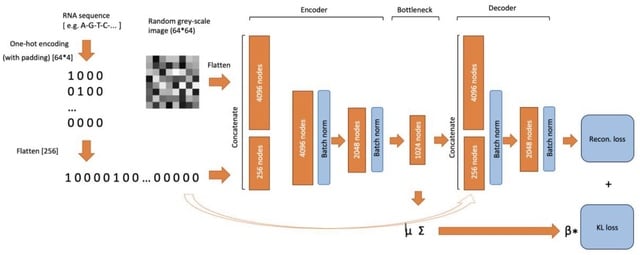
□ RNAfold: RNA tertiary structure prediction using variational autoencoder.
>> https://www.biorxiv.org/content/10.1101/2024.06.18.599511v1
RNAfold, a novel method for predicting of RNA tertiary structure using a Variational Autoencoder. Compared with traditional approaches (e.g., Dynamic Simulations), the method uses the complex non-linear relationship in the RNA sequences to perform the prediction.
RNAfold achieves the RMSE of approx. 3.3 Angstrom for predicting of the nucleotide positions. For some structures, sub-optimal conformations that could vary from the original tertiary structures are found. Diffusion models can enhance the prediction of the tertiary structure.

□ AEon: A global genetic ancestry estimation tool
>> https://www.biorxiv.org/content/10.1101/2024.06.18.599246v1
AEon, a probabilistic model-based global AE tool, ready for use on modern genomic data. AEon predicts fractional population membership of input samples given allele frequency data from known populations, accounting for possible admixture.

□ TarDis: Achieving Robust and Structured Disentanglement of Multiple Covariates
>> https://www.biorxiv.org/content/10.1101/2024.06.20.599903v1
TarDis employs covariate-specific loss functions through a self-supervision strategy, enabling the learning of disentangled representations that achieve accurate reconstructions and effectively preserve essential biological variations across diverse datasets.
TarDis handles both categorical and, notably, continuous variables, demonstrating its adaptability to diverse data characteristics and allowing for a granular understanding and representation of underlying data dynamics within a coherent and interpretable latent space.
※コメント投稿者のブログIDはブログ作成者のみに通知されます