
□ Universal co-Extensions of torsion abelian groups
>> https://arxiv.org/pdf/2206.08857v1.pdf
An Ab3 abelian category which is Ext-small, satisfies the Ab4 condition if, and only if, each object of it is Ext-universal. In particular, this means that there are torsion abelian groups that are not co-Ext-universal in the category of torsion abelian groups.
Naturally the following question arises for non-Ab4 and Ab3 abelian categories: when an object V of such category admits a universal extension of V by every object? Characterizing all torsion abelian groups which are co-Ext-universal in such category.

□ Variational Bayes for high-dimensional proportional hazards models with applications within gene expression
>> https://academic.oup.com/bioinformatics/advance-article/doi/10.1093/bioinformatics/btac416/6617825
Using a sparsity-inducing spike-and-slab prior with Laplace slab and Dirac spike, referred to as sparse variational Bayes (SVB).
The method is based on a mean-field variational approximation, overcomes the computational cost of MCMC whilst retaining features, providing a posterior distribution for the parameters and offering a natural mechanism for variable selection via posterior inclusion probabilities.
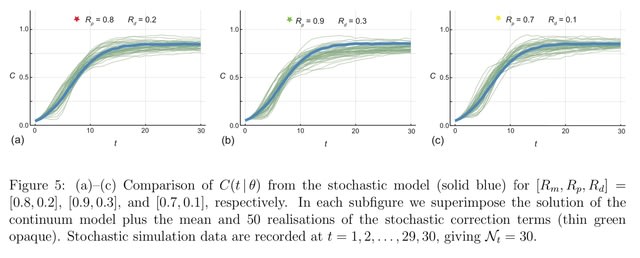
□ Reliable and efficient parameter estimation using approximate continuum limit descriptions of stochastic models
>> https://www.sciencedirect.com/science/article/abs/pii/S0022519322001990
Combining stochastic and continuum mathematical models in the context of lattice- based models of two-dimensional cell biology experiments by demonstrating how to simulate two commonly used experiments: cell proliferation assays and barrier assays.
Simulating a proliferation assay, where the continuum limit model is the logistic ordinary differential equation, as well as a barrier assay where the continuum limit model is closely related to the Fisher- Kolmogorov–Petrovsky–Piskunov equation partial differential equation.

□ Turing 次数から実効トポス上の Lawvere-Tierney 位相へ
>> https://researchmap.jp/cabinets/cabinet_files/download/815179/bdc501f2005d04fa6041239e58a67484
“探索問題のチューリング還元は,複雑性の低い ∀∃-型の 定理に関していえば,そこそこの精度で逆数学的結果と一致する ため,構成的逆数学の “予測” として利用可能である.”

□ SNVformer: An Attention-based Deep Neural Network for GWAS Data
>> https://www.biorxiv.org/content/10.1101/2022.07.07.499217v1.full.pdf
Sparse SNVs can be efficiently used by Transformer-based networks without expanding them to a full genome. It is able to achieve competitive initial performance, with an AUROC of 83% when classifying a balanced test set using genotype and demographic information.
A Transformer-based deep neural architecture for GWAS data, including a purpose-designed SNV encoder, that is capable of modelling gene-gene interactions and multidimensional phenotypes, and which scales to the whole-genome sequencing data standard for modern GWAS.

□ P-smoother: efficient PBWT smoothing of large haplotype panels
>> https://academic.oup.com/bioinformaticsadvances/article/2/1/vbac045/6611715
P-smoother, a Burrows-Wheeler transformation (PBWT) based smoothing algorithm to actively ‘correct’ occasional mismatches and thus ‘smooth’ the panel.
P-smoother runs a bidirectional PBWT-based panel scanning that flips mismatching alleles based on the overall haplotype matching context, the IBD (identical-by-descent) prior. P-smoother’s scalability is reinforced by benchmarks on panels ranging from 4000 - 1 million haplotypes.

□ GBZ File Format for Pangenome Graphs
>> https://www.biorxiv.org/content/10.1101/2022.07.12.499787v1.full.pdf
As the GBWTGraph uses a GBWT index for graph topology, it only needs to store a header and node labels. While the in-memory data structure used in Giraffe stores the labels in both orientations for faster access, serializing the reverse orientation is clearly unnecessary.
The libraries use Elias–Fano encoded bitvectors. While GFA graphs have segments with string names, bidirected sequence graphs have nodes w/ integer identifiers. And while the original graph may have segments w/ long labels, it often makes sense to limit the length of the labels.

□ LRBinner: Binning long reads in metagenomics datasets using composition and coverage information
>> https://almob.biomedcentral.com/articles/10.1186/s13015-022-00221-z
LRBinner, a reference-free binning approach that combines composition and coverage information of complete long-read datasets. LRBinner also uses a distance-histogram-based clustering algorithm to extract clusters with varying sizes.
LRBinner uses a variational auto-encoder to obtain lower dimensional representations by simultaneously incorporating both composition and coverage information of the complete dataset. LRBinner assigns unclustered reads to obtained clusters using their statistical profiles.
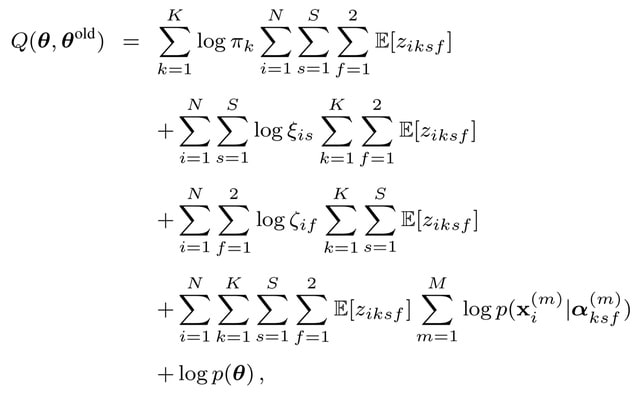
□ ChromDMM: A Dirichlet-Multinomial Mixture Model For Clustering Heterogeneous Epigenetic Data
>> https://academic.oup.com/bioinformatics/advance-article/doi/10.1093/bioinformatics/btac444/6628584
ChromDMM, a product Dirichlet-multinomial mixture model that provides a probabilistic method to cluster multiple chromatin-feature coverage signals extracted from the same locus.
ChromDMM learns the shift and flip states more accurately compared to ChIP-Partitioning and SPar-K. Owing to hyper-parameter optimisation, ChromDMM can also regularise the smoothness of the epigenetic profiles across the consecutive genomic regions.

□ Slow5tools: Flexible and efficient handling of nanopore sequencing signal data
>> https://www.biorxiv.org/content/10.1101/2022.06.19.496732v1.full.pdf
SLOW5 was developed to overcome inherent limitations in the standard FAST5 signal data format that prevent efficient, scalable analysis. SLOW5 can be encoded in human-readable ASCII format, or a more compact and efficient binary format (BLOW5).
Slow5tools uses multi-threading, multi-processing and other engineering strategies to achieve fast data conversion and manipulation, including live FAST5-to-SLOW5 conversion during sequencing.

□ RResolver: efficient short-read repeat resolution within ABySS
>> https://bmcbioinformatics.biomedcentral.com/articles/10.1186/s12859-022-04790-z
RResolver, a scalable algorithm that takes a short-read de Bruijn graph assembly with a starting k as input and uses a k value closer to that of the read length to resolve repeats. This larger k step bypasses multiple short k increments.
RResolver builds a Bloom filter of sequencing reads which is used to evaluate the assembly graph path support at branching points and removes paths w/ insufficient support. Any unambiguous paths have their nodes merged, with each path getting its own copy of the repeat sequence.

□ Figbird: A probabilistic method for filling gaps in genome assemblies
>> https://academic.oup.com/bioinformatics/advance-article/doi/10.1093/bioinformatics/btac404/6613135
Figbird, a probabilistic method for filling gaps in draft genome assemblies using second generation reads based on a generative model for sequencing that takes into account information on insert sizes and sequencing errors.
Figbird is based on a generative model for sequencing proposed in CGAL and subsequently used to develop a scaffolding tool SWALO, and uses an iterative approach based on the expectation-maximization (EM) algorithm for a range of gap lengths.
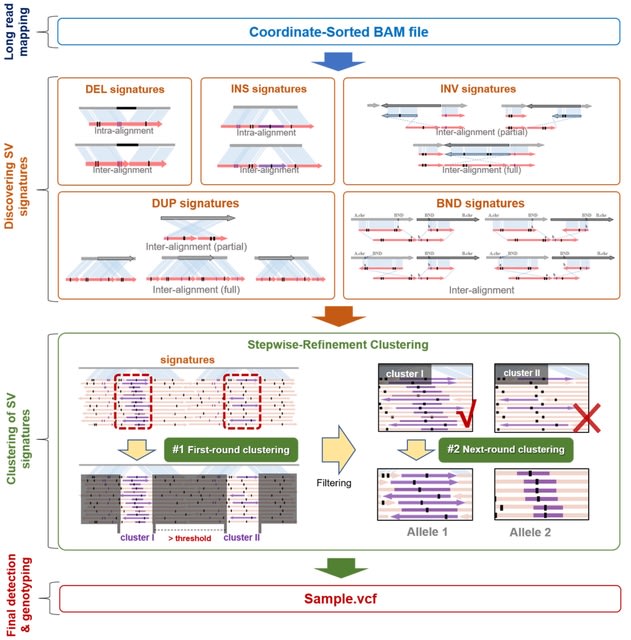
□ CuteSV: Structural Variant Detection from Long-Read Sequencing Data https://link.springer.com/protocol/10.1007/978-1-0716-2293-3_9
cuteSV, a sensitive, fast, and scalable alignment-based SV detection approach to complete comprehensive discovery of diverse SVs. cuteSV is suitable for large-scale genome project since its excellent SV yields and ultra-fast speed.
cuteSV employs a stepwise refinement clustering algorithm to process the comprehensive signatures from inter- and intra-alignment, construct and screen all possible alleles thus completes high-quality SV calling.

□ HoloNet: Decoding functional cell-cell communication events by multi-view graph learning on spatial transcriptomics
>> https://www.biorxiv.org/content/10.1101/2022.06.22.496105v1.full.pdf
HoloNet models CEs in spatial data as a multi-view network using the ligand and receptor expression profiles, developed a graph neural network model to predict the expressions of specific genes.
HoloNet reveals the holographic cell–cell communication networks which could help to find specific cells and ligand– receptor pairs that affect the alteration of gene expression and phenotypes. HoloNet interpretes the trained neural networks to decode FCEs.
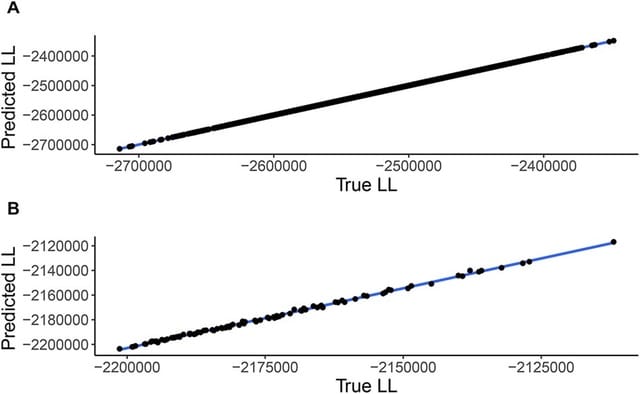
□ A LASSO-based approach to sample sites for phylogenetic tree search
>> https://academic.oup.com/bioinformatics/article/38/Supplement_1/i118/6617489
An artificial-intelligence-based approach, which provides means to select the optimal subset of sites and a formula by which one can compute the log-likelihood of the entire data based on this subset.
The grid of penalty parameters is chosen, by default, such that the maximum value in the grid is the minimal penalty which forces all coefficients to equal exactly zero. Iterating over the penalty grid until finding a solution matching this criterion.

□ SeqScreen: accurate and sensitive functional screening of pathogenic sequences via ensemble learning
>> https://genomebiology.biomedcentral.com/articles/10.1186/s13059-022-02695-x
SeqScreen uses a multimodal approach combining conventional alignment-based tools, machine learning, and expert sequence curation to produce a new paradigm for novel pathogen detection tools, which is beneficial to synthetic DNA manufacturers.
SeqScreen provides an advantage in that it also reports the most likely taxonomic assignments and protein-specific functional information for each sequence, incl. GO terms / FunSoCs, to identify pathogenic sequences in each sequence without relying solely on taxonomic markers.

□ AvP: a software package for automatic phylogenetic detection of candidate horizontal gene transfers.
>> https://www.biorxiv.org/content/10.1101/2022.06.23.497291v1.full.pdf
AvP (Alienness vs Predictor) to automate the robust identification of HGTs at high-throughput. AvP facilitates the identification and evaluation of candidate HGTs in sequenced genomes across multiple branches of the tree of life.
AvP extracts all the information needed to produce input files to perform phylogenetic reconstruction, evaluate HGTs from the phylogenetic trees, and combine multiple external information.

□ LowKi: Moment estimators of relatedness from low-depth whole-genome sequencing data
>> https://bmcbioinformatics.biomedcentral.com/articles/10.1186/s12859-022-04795-8
Both the kinship coefficient φ and the fraternity coefficient ψ for all pairs of individuals are of interest. However, when dealing with low-depth sequencing or imputation data, individual level genotypes cannot be confidently called.
LowKi (Low-depth Kinship), a new method-of-moment estimators of both the coefficients φ and ψ calculated directly from genotype likelihoods. LowKi is able to recover the structure of the Full GRM kinship and fraternity matrices.

□ Comprehensive benchmarking of CITE-seq versus DOGMA-seq single cell multimodal omics
>> https://genomebiology.biomedcentral.com/articles/10.1186/s13059-022-02698-8
DOGMA-seq provides unprecedented opportunities to study complex cellular and molecular processes at single cell resolution, but a comprehensive independent evaluation is needed to compare these new trimodal assays to existing single modal and bimodal assays.
Single cell trimodal omics measurements were generally better than after an alternative “low-loss lysis”. DOGMA-seq with optimized DIG permeabilization and its ATAC library provides more information, although its mRNA libraries have slightly inferior quality compared to CITE-seq.

□ ClearCNV: CNV calling from NGS panel data in the presence of ambiguity and noise
>> https://academic.oup.com/bioinformatics/advance-article-abstract/doi/10.1093/bioinformatics/btac418/6617832
CNV calling has not been established in all laboratories performing panel sequencing. At the same time such laboratories have accumulated large data sets and thus have the need to identify copy number variants on their data to close the diagnostic gap.
clearCNV identifies CNVs affecting the targeted regions. clearCNV can cope relatively well with the wide variety of panel types, panel versions and vendor technologies present in typical heterogenous panel data collections found in rare disease research.
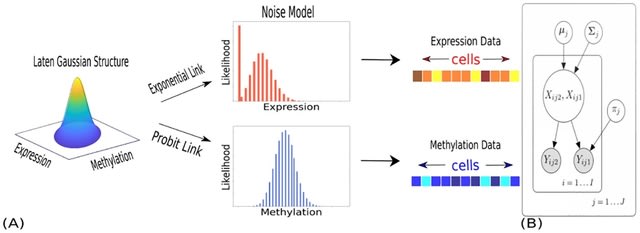
□ SCRaPL: A Bayesian hierarchical framework for detecting technical associates in single cell multiomics data
>> https://journals.plos.org/ploscompbiol/article?id=10.1371/journal.pcbi.1010163
SCRaPL Single Cell Regulatory Pattern Learning) achieves higher sensitivity and better robustness in identifying correlations, while maintaining a similar level of false positives as standard analyses based on Pearson and Spearman correlation.
SCRaPL aims become a useful tool in the hands of practitioners seeking to understand the role of particular genomic regions in the epigenetic landscape. SCRaPL can increase detection rates up to five times compared to standard practices.

□ MenDEL: automated search of BAC sets covering long DNA regions of interest
>> https://www.biorxiv.org/content/10.1101/2022.06.26.496179v1.full.pdf
MenDEL – a web-based DNA design application, that provides efficient tools for finding BACs that cover long regions of interest and allow for sorting results based on multiple user defined criteria.
Deploying BAC libraries as indexed database tables allows further speed up and automate parsing of these libraries. An important property of those N-ary trees is that their depth-first traversals provide a complete list of unique BAC solutions.
□ Improving Biomedical Named Entity Recognition by Dynamic Caching Inter-Sentence Information
>> https://academic.oup.com/bioinformatics/advance-article/doi/10.1093/bioinformatics/btac422/6618522
Specifically, the cache stores recent hidden representations constrained by predefined caching rules. And the model uses a query-and-read mechanism to retrieve similar historical records from the cache as the local context.
Then, an attention-based gated network is adopted to generate context-related features with BioBERT. To dynamically update the cache, we design a scoring function and implement a multi-task approach to jointly train the model.

□ WiNGS: Widely integrated NGS platform for federated genome analysis
>> https://www.biorxiv.org/content/10.1101/2022.06.23.497325v1.full.pdf
WiNGS sits at the crossroad of patient privacy rights and the need for highly performant / collaborative genetic variant interpretation platforms. It is a fast, fully interactive, and open source web-based platform to analyze DNA variants in both research / diagnostic settings.

□ Accuracy of haplotype estimation and whole genome imputation affects complex trait analyses in complex biobanks
>> https://www.biorxiv.org/content/10.1101/2022.06.27.497703v1.full.pdf
While phasing accuracy varied both by choice of method and data integration protocol, imputation accuracy varied mostly between data integration protocols. Finally, imputation errors can modestly bias association tests and reduce predictive utility of polygenic scores.

□ MaxHiC: A robust background correction model to identify biologically relevant chromatin interactions in Hi-C and capture Hi-C experiments
>> https://journals.plos.org/ploscompbiol/article?id=10.1371/journal.pcbi.1010241
MaxHiC (Maximum Likelihood estimation for Hi-C), a negative binomial model that uses a maximum likelihood technique to correct the complex combination of known and unknown biases in both Hi-C and capture Hi-C libraries.
In MaxHiC, distance is modelled by a function that decreases at increasing genomic distances to reach a constant non-zero value. All of the parameters of the model are learned by maximizing the logarithm of likelihood of the observed interactions using the ADAM algorithm.
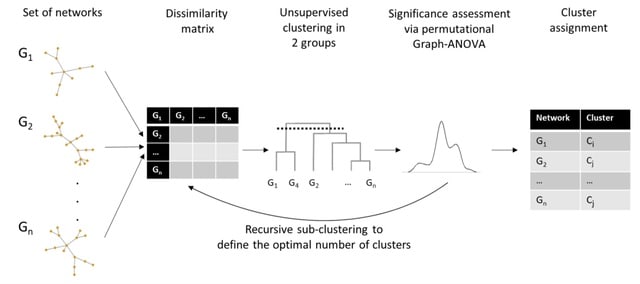
□ netANOVA: novel graph clustering technique with significance assessment via hierarchical ANOVA
>> https://www.biorxiv.org/content/10.1101/2022.06.28.497741v1.full.pdf
netANOVA analysis workflow we aim to exploit information about structural and dynamical properties of networks to identify significantly different groups of similar networks.
netANOVA workflow accommodates multiple distance measures: edge difference distance, a customized KNC version of k-step random walk kernel, DeltaCon, GTOM and the Gaussian kernel on the vectorized networks.

□ Gene symbol recognition with GeneOCR
>> https://www.biorxiv.org/content/10.1101/2022.07.01.498459v1.full.pdf
GeneOCR (OCR=optical character recognition) employs a state- of-the-art character recognition system to recognize gene symbols.
The errors are mostly due to substitution of optically similar characters, e.g. 1 for I or O for 0. In summary, GeneOCR recognizes or suggests the correct gene symbol in >80% cases and the errors in the rest case involve mostly single characters.

□ SIDEREF: Shared Differential Expression-Based Distance Reflects Global Cell Type Relationships in Single-Cell RNA Sequencing Data
>> https://www.liebertpub.com/doi/10.1089/cmb.2021.0652
SIDEREF modifies a biologically motivated distance measure, SIDEseq, for use of aggregate comparisons of cell types in large single-cell assays. The distance matrix more consistently retains global cell type relationships than commonly used distance measures for scRNA seq clustering.
Exploring spectral dimension reduction of the SIDEREF distance matrix as a means of noise filtering, similar to principal components analysis applied directly to expression data.

□ Gfastats: conversion, evaluation and manipulation of genome sequences using assembly graphs
>> https://academic.oup.com/bioinformatics/advance-article/doi/10.1093/bioinformatics/btac460/6633308
Gfastats is a standalone tool to compute assembly summary statistics and manipulate assembly sequences in fasta, fastq, or gfa [.gz] format. Gfastats stores assembly sequences internally in a gfa-like format. This feature allows gfastats to seamlessly convert fast* to and from gfa [.gz] files.
Gfastats can also build an assembly graph that can in turn be used to manipulate the underlying sequences following instructions provided by the user, while simultaneously generating key metrics for the new sequences.

□ Fast-HBR: Fast hash based duplicate read remover
>> http://www.bioinformation.net/018/97320630018036.pdf
Fast-HBR, a fast and memory-efficient duplicate reads removing tool without a reference genome using de-novo principles. Fast-HBR is faster and has less memory footprint when compared with the state of the art De-novo duplicate removing tools.
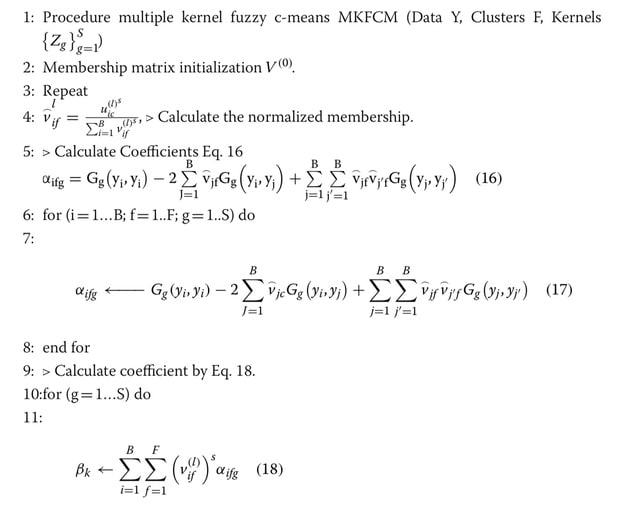
□ MKFTM: A novel multiple kernel fuzzy topic modeling technique for biomedical data
>> https://bmcbioinformatics.biomedcentral.com/articles/10.1186/s12859-022-04780-1
MKFTM technique uses fusion probabilistic inverse document frequency and multiple kernel fuzzy c-means clustering algorithm for biomedical text mining.
In detail, the proposed fusion probabilistic inverse document frequency method is used to estimate the weights of global terms while MKFTM generates frequencies of local and global terms with bag-of-words.

□ Trade-off between conservation of biological variation and batch effect removal in deep generative modeling for single-cell transcriptomics
>> https://www.biorxiv.org/content/10.1101/2022.07.14.500036v1.full.pdf
Using Pareto MTL for estimation of Pareto front in conjunction with MINE for measurement of batch effect to produce the trade-off curve between conservation of biological variation and removal of batch effect.
To control batch effect, the generative loss of scVI is penalized by the Hilbert-Schmidt Independence Criterion (HSIC). The generative loss of SAUCIE, a sparse autoencoder, is penalized by the Maximum Mean Discrepancy (MMD).
MINE is preferable to the more standard MMD measure in the sense that the former produces trade-off points that respect subproblem ordering and are interpretable in surrogate metric spaces.

□ RLSuite: An integrative R-loop bioinformatics framework
>> https://www.biorxiv.org/content/10.1101/2022.07.13.499820v1.full.pdf
R-loops are three-stranded nucleic acid structures containing RNA:DNA hybrids. While R-loop mapping via high-throughput sequencing can reveal novel insight into R-loop biology, the quality control of these data is a non-trivial task for which few bioinformatic tools exist.
RLSuite provides an integrative workflow for R-loop data analysis, including automated pre-processing of R-loop mapping data using a standard pipeline, multiple robust methods for quality control, and a range of tools for the initial exploration of R-loop data.

□ Clair3-trio: high-performance Nanopore long-read variant calling in family trios with trio-to-trio deep neural networks
>> https://academic.oup.com/bib/advance-article-abstract/doi/10.1093/bib/bbac301/6645484
Clair3-Trio employs a Trio-to-Trio deep neural network model, which allows it to input the trio sequencing information and output all of the trio’s predicted variants within a single model to improve variant calling.
MCVLoss is a novel loss function tailor-made for variant calling in trios, leveraging the explicit encoding of the Mendelian inheritance. Clair3-Trio uses independent dense layers to predict each individual’s genotype, zygosity and two INDEL lengths in the last layer.
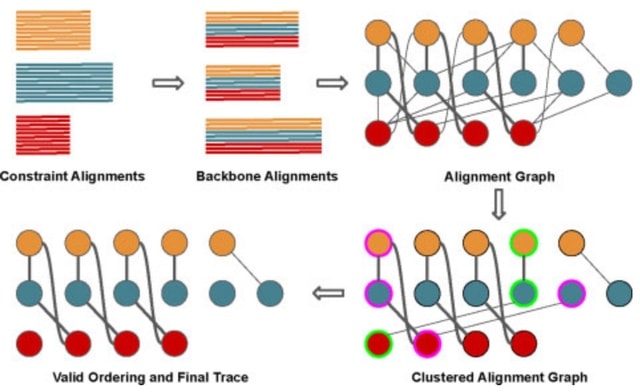
□ Large-Scale Multiple Sequence Alignment and the Maximum Weight Trace Alignment Merging Problem
>> https://ieeexplore.ieee.org/document/9832784/
MAGUS uses divide-and-conquer: it divides the sequences into disjoint sets, computes alignments on the disjoint sets, and then merges the alignments using a technique it calls the Graph Clustering Method (GCM).
GCM is a heuristic for the NP-hard Maximum Weight Trace, adapted to the Alignment Merging problem. The input to the MWT problem is a set of sequences and weights on pairs of letters from different sequences, and the objective is an MSA that has the maximum total possible weight.
※コメント投稿者のブログIDはブログ作成者のみに通知されます