
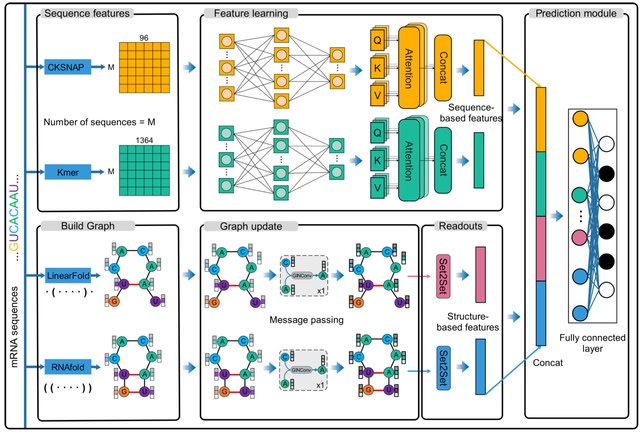
□ Allocator: A graph neural network-based framework for mRNA subcellular localization prediction
>> https://www.biorxiv.org/content/10.1101/2023.12.14.571762v1
Allocator is a multi-view parallel deep learning framework that is designed for mRNA multi-localization prediction. Allocator incorporates various network architectures, including multilayer perceptron (MLP), self-attention, and GIN (graph isomorphism network), to ensure reliable predictions.
Allocator employs two encodings, k-mer and CKSNAP (k-spaced nucleic acid pairs), for extracting primary sequence characteristics. These inputs undergo feature learning through two numerical extractors and two graph extractors.
Each node is denoted by a 10-dimensional feature vector that integrates four different encodings: one-hot, NCP: nucleotide chemical property, EIIP: electronion interaction pseudopotentials, and ANF: accumulated nucleotide frequency.

□ scInterpreter: a knowledge-regularized generative model for interpretably integrating scRNA-seq data
>> https://bmcbioinformatics.biomedcentral.com/articles/10.1186/s12859-023-05579-4
scinterpreter, an interpretable deep learning model that can learn the unified representation of cells in the embedding space. The encoder is designed to remove the batch effects, and the generator simulates this process.
scInterpreter can process vast data with mini-batch strategy. The embedding dimension is set to the number of pathways and constrain the decoder weights by prior knowledge, which allows for the explanation of cell function based on the amount of expression in each dimension.

□ SPDesign: protein sequence designer based on structural sequence profile using ultrafast shape recognition
>> https://www.biorxiv.org/content/10.1101/2023.12.14.571651v1
SPDesign, a method for protein sequence design based on structural sequence profile. SPDesign utilizes ultrafast shape recognition vectors to accelerate the search for similar protein structures, and then extracts the sequence profile from the analogs through structure alignment.
SPDesign can capture the intrinsic sequence-structure mapping. SPDesign utilizes the TM-align tool to perform a comprehensive alignment between the input backbone and all structures within the chosen k clusters. SPDesign performs very well on the overall fragment sequence.

□ BioEGRE: a linguistic topology enhanced method for biomedical relation extraction based on BioELECTRA and graph pointer neural network
>> https://bmcbioinformatics.biomedcentral.com/articles/10.1186/s12859-023-05601-9
BioEGRE (BioELECTRA and Graph pointer neural net-work for Relation Extraction), aimed at leveraging the linguistic topological features. First, the biomedical literature is preprocessed to retain sentences involving pre-defined entity pairs.
BioEGRE employs SciSpaCy to conduct dependency parsing; sentences are modeled as graphs based on the parsing results; BioELECTRA is utilized to generate token-level representations, which are modeled as attributes of nodes in the sentence graphs.
BioEGRE employs a graph pointer neural network layer to select the most relevant multi-hop neighbors to optimize representations; a fully-connected neural network layer is employed to generate the sentence-level representation.

□ Personalized Pangenome References
>> https://www.biorxiv.org/content/10.1101/2023.12.13.571553v1
A personalized pangenome reference by sampling haplotypes that are similar to the sequenced genome according to k-mer counts in the reads. It works directly with assembled haplotypes. Any alignments in the sampled graph are also valid in the original graph.
This approach is tailored for Giraffe, as the indexes it needs for read mapping can be built quickly. They assume a graph with a linear high-level structure, such as graphs built using the Minigraph-Cactus pipeline.
The structure of a bidirected sequence graph can be described hierarchically by its snarl decomposition. A snarl is a generalization of a bubble, and denotes a site of genomic variation. It is a subgraph separated by two node sides from the rest of the graph.
A graph can be decomposed into a set of chains, each of which is a sequence of nodes and snarls. A snarl may either be primitive, or it may be further decomposed into a set of chains.

□ Involutive Markov categories and the quantum de Finetti theorem
>> https://arxiv.org/abs/2312.09666
Involutive Markov categories are equivalent to Parzygnat's quantum Markov categories. Involutive Markov categories involves C*-algebras (of any dimension) as objects and completely positive unital maps as morphisms.
Prove a quantum de Finetti theorem for both the minimal and the maximal C*-tensor norms, and develop a categorical description of these quantum de Finetti theorems, a description which represents a universal property of state spaces.
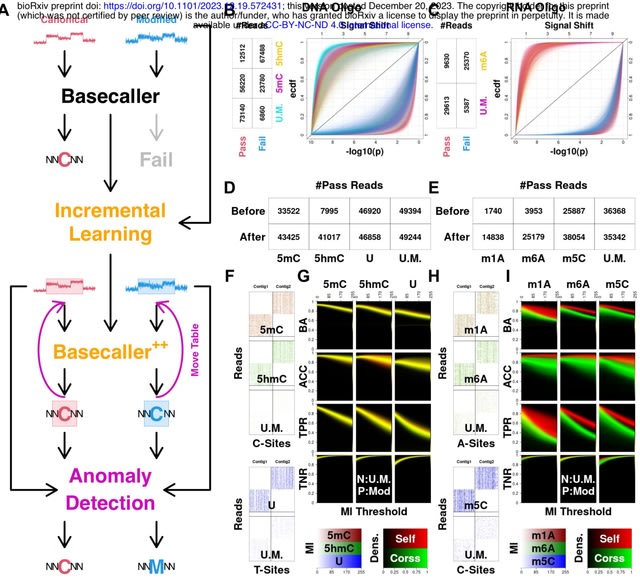
□ IL-AD: Adapting Nanopore Sequencing Basecalling Models for Modification Detection via Incremental Learning and Anomaly Detection
>> https://www.biorxiv.org/content/10.1101/2023.12.19.572431v1
Incremental learning (IL) generalizes basecallers to resolve sequence backbones for both canonical and modified nanopore sequencing readouts. IL-basecallers will therefore provide sequence backbones for each individual molecule, on top of which modifications could be analyzed.
Leverage anomaly detection (AD) techniques to scrutinize the modification status of individual nucleotides. AD summarizes a group of statistical approaches for identifying significantly deviated data observations, in this case modification-induced signals.

□ ESCHR: A hyperparameter-randomized ensemble approach for robust clustering across diverse datasets
>> https://www.biorxiv.org/content/10.1101/2023.12.18.571953v1
ESCHR, an ensemble clustering method with hyperparameter randomization that outperforms other methods across a broad range of single-cell and synthetic datasets, without the need for manual hyperparameter selection.
ESCHR characterizes continuum-like regions and per cell overlap scores to quantify the uncertainty in cluster assignment. ESCHR performs Leiden community detection on kNN graph using a randomly selected value for the required resolution-determining hyperparameter.

□ ENTRAIN: integrating trajectory inference and gene regulatory networks with spatial data to co-localize the receptor-ligand interactions that specify cell fate
>> https://academic.oup.com/bioinformatics/advance-article/doi/10.1093/bioinformatics/btad765/7479687
ENTRAIN (environment-aware trajectory inference), a computational method that integrates trajectory inference methods with ligand-receptor pair gene regulatory networks to identify extracellular signals and evaluate their relative contribution towards a differentiation trajectory.
The output from ENTRAIN can be superimposed on spatial data to co-localize cells and molecules in space and time to map cell fate potentials to cell-cell interactions.
ENTRAIN implements pseudotime analysis by using the Monocle3 workflow, which applies the SimplePPT tree algorithm to cells in reduced dimension space to calculate cell pseudotimes.
The ENTRAIN-Pseudotime module allows flexible input from any trajectory method provided that each input cell is assigned a pseudotime value and a trajectory branch in the Seurat object metadata.
ENTRAIN generalizes to other trajectory inference techniques, including UnIT Velo, VeloVI, and Diffusion Pseudotime methods with high similarity as measured by the rank-based overlap.

□ ChIP-DIP: A multiplexed method for mapping hundreds of proteins to DNA uncovers diverse regulatory elements controlling gene expression
>> https://www.biorxiv.org/content/10.1101/2023.12.14.571730v1
ChIP-DIP (ChIP Done In Parallel), a split-pool based method that enables simultaneous, genome-wide mapping of hundreds of diverse regulatory proteins in a single experiment.
ChIP-DIP generates highly accurate maps for all classes of DNA-associated proteins, including histone modifications, chromatin regulators, transcription factors, and RNA Polymerases.

□ MisFit: A probabilistic graphical model for estimating selection coefficient of nonsynonymous variants from human population sequence data
>> https://www.medrxiv.org/content/10.1101/2023.12.11.23299809v1
MisFit, a new method to jointly predict molecular effect and human fitness effect of missense variants through a probabilistic graphical model. MisFit can estimate selection coefficient for variants under moderate to strong negative selection.
MisFit uses Poisson-Inverse-Gaussian distribution to model allele counts in human populations. MisFit generates probability of amino acid in orthologues. Heterozygous is linear in logit scale, with gene-level maximum from a global prior.

□ ATOM-1: A Foundation Model for RNA Structure and Function Built on Chemical Mapping Data \
>> https://www.biorxiv.org/content/10.1101/2023.12.13.571579v1
ATOM-1, a foundation model trained on large quantities of chemical mapping data collected in-house across different experimental conditions, chemical reagents, and sequence libraries. Using probe networks, ATOM-1 has developed rich and accessible internal representations of RNA.
ATOM-1 has an understanding of secondary structure, Probe networks using ATOM-1 embeddings are considered. Since base pairing is a property of each pair of nucleotides, it is natural to apply these probes to the pair representation independently along the last dimension.
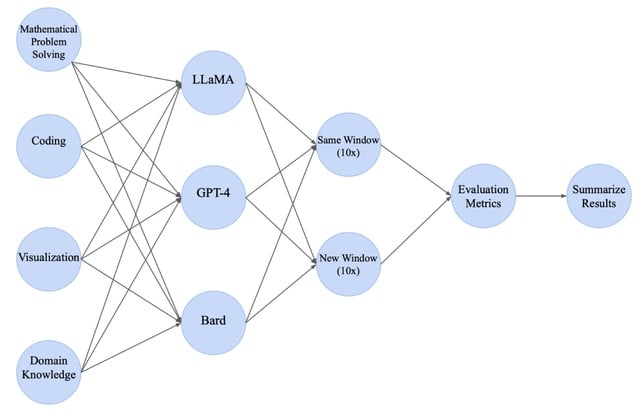
□ BioLLMBench: A Comprehensive Benchmarking of Large Language Models in Bioinformatics
>> https://www.biorxiv.org/content/10.1101/2023.12.19.572483v1
BioLLMBench, a benchmarking framework coupled with a comprehensive scoring metric scheme designed to evaluate the 3 most widely used LLMs, namely GPT-4, Bard and LLaMA in solving bioinformatics tasks.
The ROUGE (Recall-Oriented Understudy for Gisting Evaluation) scores were low across all models. GPT-4 provided more fluent summaries, but none of the models were able to fully capture the grammatical structure and context of the original texts.

□ LncLocFormer: a Transformer-based deep learning model for multi-label lncRNA subcellular localization prediction by using localization-specific attention mechanism
>> https://academic.oup.com/bioinformatics/advance-article/doi/10.1093/bioinformatics/btad752/7477673
LncLocFormer, a Transformer-based deep learning model using a localization-specific attention mechanism. LncLocFormer utilizes 8 Transformer blocks to model long-range dependencies within the lncRNA sequence and share information across the lncRNA sequence.
LncLocFormer can predict multiple subcellular localizations simultaneously for each IncRNA sequence. LncLocFormer learns different attention weights for different subcellular localizations, which can provide valuable information about the relationship between different labels.

□ STACCato: Supervised Tensor Analysis tool for studying Cell-cell Communication using scRNA-seq data across multiple samples and conditions
>> https://www.biorxiv.org/content/10.1101/2023.12.15.571918v1
STACCato, the Supervised Tensor Analysis tool for studying Cell-cell Communication, that uses multi-sample multi-condition scRNA-seq dataset to identify CCC events significantly associated with conditions while adjusting for potential sample-level confounders.
STACCato considers the same 4-dimentional communication score tensor as the Tensor-cell2cell tool, with 4 dimensions corresponding to samples, ligand-receptor pairs, sender cell types, and receiver cell types.
STACCato employs supervised tensor decomposition to fit a regression model that considers the 4-dimensional communication score tensor as the outcome variable while treating the biological conditions and other sample-level covariates as independent variables.

□ SSEmb: A joint embedding of protein sequence and structure enables robust variant effect predictions
>> https://www.biorxiv.org/content/10.1101/2023.12.14.571755v1
SSEmb (Sequence Structure Embedding) combines a graph representation for the protein structure with a transformer model for processing multiple sequence alignments.
SSEmb obtains a variant effect prediction model that is more robust to cases where sequence information is scarce. Furthermore, SSEmb learns embeddings of the sequence and structural properties that are useful for other downstream tasks.

□ DeepPBS: Geometric deep learning for interpretable prediction of protein-DNA binding specificity
>> https://www.biorxiv.org/content/10.1101/2023.12.15.571942v1
Deep Predictor of Binding Specificity (DeepPBS), a geometric deep-learning model designed to predict binding specificity across protein families based on protein-DNA structures. The DeepPBS architecture allows investigation of different family-specific recognition patterns.
DeepPBS can be applied to predicted structures, and can aid in the modeling of protein-DNA complexes. DeepPBS is interpretable and can be used to calculate protein heavy atom-level importance scores, demonstrated as a case-study on p53-DNA interface.

□ Melon: metagenomic long-read-based taxonomic identification and quantification using marker genes
>> https://www.biorxiv.org/content/10.1101/2023.12.17.572079v1
Melon first extracts reads that cover at least one marker gene using a protein database, and then profiles the taxonomy of these marker-containing reads using a separate, nucleotide database. The use of two different databases is motivated by their distinct strengths.
The protein database is particularly well-suited for estimating the total number of genome copies because of its high conservation, whereas the nucleotide database has the potential to provide a greater taxonomic resolution for individual reads during profiling.

□ Smoother: a unified and modular framework for incorporating structural dependency in spatial omics data
>> https://genomebiology.biomedcentral.com/articles/10.1186/s13059-023-03138-x
By representing data as boundary-aware-weighted graphs and Markov random fields, Smoother explicitly characterizes the dependency structure, allowing information exchange between neighboring locations and facilitating scalable inference of cellular and cell-type activities.
Through the transformation between spatial prior and regularization loss, Smoother is highly modularized and ultra-efficient, enabling the seamless conversion of existing non-spatial single-cell-based models into spatially aware versions.

□ chronODE: A framework to integrate time-series multi-omics data based on ordinary differential equations combined with machine learning
>> https://www.biorxiv.org/content/10.1101/2023.12.13.571513v1
chronODE, a mathematical framework based on ordinary differential equations that uniformly models the kinetics of temporal changes in gene expression and chromatin features.
chronODE is integrated with a neural-network architecture that can link and predict changes across different data modalities by solving multivariate time-series regressions.
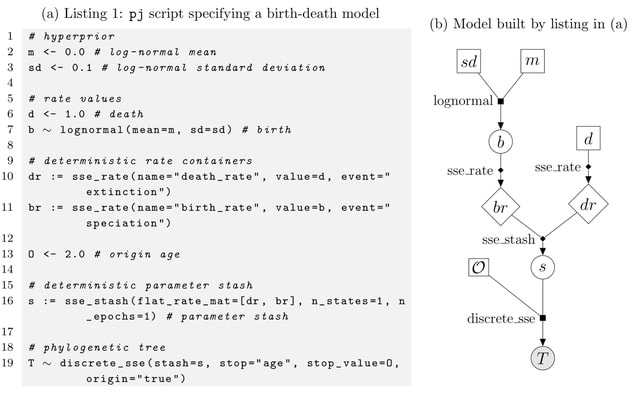
□ PhyloJunction: a computational framework for simulating, developing, and teaching evolutionary models
>> https://www.biorxiv.org/content/10.1101/2023.12.15.571907v1
PhyloJunction ships with a very general SSE (state-dependent speciation and extinction) model simulator and with additional functionalities for model validation and Bayesian analysis.
PhyloJunction has been designed with a graphical modeling architecture and equipped with a dedicated probabilistic programming language.
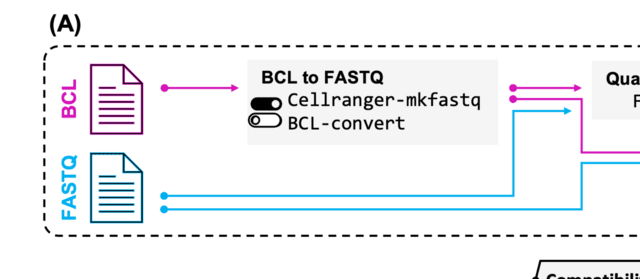
□ CellBridge: Scaling up Single-Cell RNA-seq Data Analysis
>> https://academic.oup.com/bioinformatics/advance-article/doi/10.1093/bioinformatics/btad760/7479685
CellBridge encompasses various crucial steps in scRNA-seq analysis, starting from the initial conversion of raw unaligned sequencing reads into the FASTQ format, followed by read alignment, gene expression quantification, normalization, batch correction, dimensionality reduction, etc.
CellBridge provides convenient parameterization of the workflow, while its Docker-based framework ensures reproducibility of results across diverse computing environments.
CellBridge accepts different types of input data for analysis. The first type is the widely used output of the 10X-Genomics Cell Ranger pipeline: the trio of the matrix of UMI counts, the list of cell barcodes, and the list of gene names.

□ ENGEP: advancing spatial transcriptomics with accurate unmeasured gene expression prediction
>> https://genomebiology.biomedcentral.com/articles/10.1186/s13059-023-03139-w
ENGEP integrates the results of different reference datasets and prediction methods, instead of relying on a single reference dataset. It not only avoids manual selection of the best reference dataset and prediction method but also results in a more consistent prediction.
ENGEP partitions each substantial reference dataset into smaller sub-reference datasets. ENGEP uses k-nearest-neighbor (k-NN) regression with ten different similarity measures and four different values of k (number of neighbors) to generate forty different base results.

□ PAPerFly: Partial Assembly-based Peak Finder for ab initio binding site reconstruction
>> https://bmcbioinformatics.biomedcentral.com/articles/10.1186/s12859-023-05613-5
PAPerFly takes in raw sequencing reads from a ChIP-seq experiment and the size of k-mer as input and outputs significantly enriched sequences with their respective significance. The reconstructed sequences are aligned and the peaks in the sequence enrichment are identified.
The PAPerFly algorithm traverses the sequencing reads with a sliding window of size k and identifies the sequences of k-mers and their respective numbers of observations. This is done for every replicate separately. The k-mer counts of the treatment replicates are then summed.
The k-mers with a low number of observations are pruned and a de Bruijn graph G is constructed from the remaining k-mers. The removal of the less frequent k-mers aims to eliminate sequencing errors, as well as to strengthen the signal of the studied binding site sequence.
Using a Gaussian hidden Markov model (GHMM), the reconstructed sequences are then broken down into segments corresponding to different GHMM states using the HMMlearn implementation.

□ Escort: Data-driven selection of analysis decisions in single-cell RNA-seq trajectory inference
>> https://www.biorxiv.org/content/10.1101/2023.12.18.572214v1
Escort is a framework for evaluating a single-cell RNA-seq dataset’s suitability for trajectory inference and for quantifying trajectory properties influenced by analysis decisions.
Escort is designed to guide users through the trajectory inference process by offering goodness-of-fit evaluations for embeddings that represent a range of analysis decisions such as feature selection, dimension reduction, and trajectory inference method-specific hyperparameters.

□ scResolve: Recovering single cell expression profiles from multi-cellular spatial transcriptomics
>> https://www.biorxiv.org/content/10.1101/2023.12.18.572269v1
scResolve generates subcellular resolution gene maps by combining spot-level expression profiles, and then from these maps segments individual cells and thereby produces their expression profiles.
A transformer model is trained to infer for each subcellular spot from gene expression whether it is part of a cell or part of the extracellular matrix, and its relative position with respect to the center of its nucleus.

□ STAIG: Spatial Transcriptomics Analysis via Image-Aided Graph Contrastive Learning for Domain Exploration and Alignment-Free Integration
>> https://www.biorxiv.org/content/10.1101/2023.12.18.572279v1
STAIG (Spatial Transcriptomics Analysis via Image-Aided Graph Contrastive Learning), a deep leaning framework based on the alignment-free integration of gene expression, spatial data, and histological images, to ensure refined spatial domain analyses.
STAIG extracts features from HE-stained images using a self-supervised model and builds a spatial graph with the features. The graph is further processed by contrastive learning via a graph neural network (GNN), which generates informative embeddings.
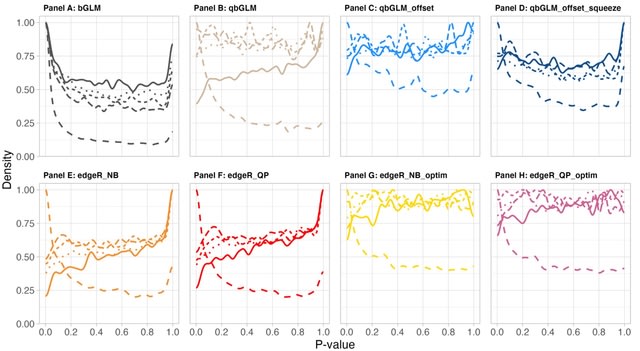
□ Differential detection workflows for multi-sample single-cell RNA-seq data
>> https://www.biorxiv.org/content/10.1101/2023.12.17.572043v1
A workflow for assessing differential detection (DD), which tests for differences in the average fraction of samples or cells in which a gene is detected. After benchmarking 8 different DD data analysis strategies, we provide a unified workflow for jointly assessing DE and DD.
DE and DD analysis provide complementary information, both in terms of the individual genes they report and in the functional interpretation of those genes.
Pseudobulking the binarized single cell counts is a natural strategy in the context of multi-sample/multi-cell datasets; it improves model performance, type I error control and tremendously decreases the computational complexity compared to a single-cell level analysis.

□ FURNA: a database for function annotations of RNA structures
>> https://www.biorxiv.org/content/10.1101/2023.12.19.572314v1
FURNA, the DB for experimental RNA structures that aims to provide a comprehensive repository of high-quality functional annotations. These include GO terms, Enzyme Commission numbers, ligand binding sites, RNA families, protein binding motifs, and cross-references to related DBs.
FURNA stands out in several ways. Firstly, it is the only database to utilize standard function vocabularies (GO terms and EC numbers) for the annotation of RNA tertiary structures.
Secondly, it outlines ligand-RNA interactions based on biological assembly, which enhances the investigational context of interactions within the complete RNA-containing complex.


□ Arctos: Community-driven innovations for managing biodiversity and cultural collections
>> https://www.biorxiv.org/content/10.1101/2023.12.15.571899v1
Arctos, a community solution for managing and accessing collections data for research and education. Specific goals to: Describe the core elements of Arctos for a broad audience with respect to the biodiversity informatics principles that enable high quality research;
Illustrate Arctos as a model for supporting and enhancing the Digital Extended Specimen; and Emphasize the role of the Arctos community for improving data discovery and enabling cross-disciplinary, integrative studies within a sustainable governance model.

□ Benchmarking splice variant prediction algorithms using massively parallel splicing assays
>> https://genomebiology.biomedcentral.com/articles/10.1186/s13059-023-03144-z
Massively parallel splicing assays (MPSAs) simultaneously assay many variants to nominate candidate splice-disruptive variants (SDVs).
Algorithms’ concordance with MPSA measurements, and with each other, is lower for exonic than intronic variants, underscoring the difficulty of identifying missense or synonymous SDVs.
Deep learning-based predictors trained on gene model annotations achieve the best overall performance at distinguishing disruptive and neutral variants, and controlling for overall call rate genome-wide, SpliceAI and Pangolin have superior sensitivity.
※コメント投稿者のブログIDはブログ作成者のみに通知されます