
□ d-PBWT: dynamic positional Burrows-Wheeler transform
>> https://academic.oup.com/bioinformatics/advance-article-abstract/doi/10.1093/bioinformatics/btab117/6149123
Durbin’s positional Burrows-Wheeler transform (PBWT) is a scalable data structure for haplotype matching. It has been successfully applied to identical by descent (IBD) segment identification and genotype imputation.
d-PBWT, a dynamic data structure where the reverse prefix sorting at each position is stored with linked lists. And systematically investigated variations of set maximal match and long match query algorithms: while they all have average case time complexity.
□ Graviton2: A generalized approach to benchmarking genomics workloads in the cloud: Running the BWA read aligner
>> https://aws.amazon.com/blogs/publicsector/generalized-approach-benchmarking-genomics-workloads-cloud-bwa-read-aligner-graviton2/
The most cost-effective instance type turns out to be the m6g.8xlarge with a mean runtime of 258 sec / run cost of $0.88. The most cost-effective x86_64 instance type was the r5dn.8xlarge with a mean runtime of 237 sec. the arm64 architecture provides optimal performance.
Graviton2 utilizes 64-bit Arm Neoverse cores and deliver up to 40 percent better price performance over comparable current generation x86-based instances. And recompiled the Burrows-Wheeler Aligner (BWA) application for Arm-based chips and evaluated their cost effectiveness.
□ Chronos: a CRISPR cell population dynamics model
>> https://www.biorxiv.org/content/10.1101/2021.02.25.432728v1.full.pdf
Chronos, an algorithm for inferring gene knockout fitness effects based on an explicit model of the dynamics of cell proliferation after CRISPR gene knockout.
Chronos addresses sgRNA efficacy, variable screen quality and cell growth rate, and heterogeneous DNA cutting outcomes through a mechanistic model of the experiment.
Chronos also directly models the readcount level data using a more rigorous negative binomial noise model, rather than modeling log-fold change values with a Gaussian distribution as is typically done.

□ FICT: Cell Type Assignments for Spatial Transcriptomics Data
>> https://www.biorxiv.org/content/10.1101/2021.02.25.432887v1.full.pdf
FICT (FISH Iterative Cell Type assignment) maximizes a joint probabilistic likelihood function that takes into account both the expression of the genes in each cell and the joint multi-variate spatial distribution of cell types.
FICT can correctly determine both expression and neighborhood parameters for different cell types improving on methods that rely only on expression levels or do not take into account the complete neighborhood of each cell.
FICT can also identify cell sub-types that are similar in terms of their expression while differ in their spatial organization.

□ MIGNON: A versatile workflow to integrate RNA-seq genomic and transcriptomic data into mechanistic models of signaling pathways
>> https://journals.plos.org/ploscompbiol/article?id=10.1371/journal.pcbi.1008748
MIGNON, a complete and versatile workflow able to exploit all the information contained in RNA-Seq data and producing not only the conventional normalized gene expression matrix, but also an annotated VCF file per sample with the corresponding mutational profile.
Gene expression and LoF variants are integrated by doing an in-silico knockdown of genes that present a LoF variant. MIGNON can combine both files to model signaling pathway activities through an integrative functional analysis using the mechanistic Hipathia algorithm.

□ Triku: a feature selection method based on nearest neighbors for single-cell data
>> https://www.biorxiv.org/content/10.1101/2021.02.12.430764v1.full.pdf
triku, a FS method that selects genes that show an unexpected distribution of zero counts and whose expression is localized in cells that are transcriptomically similar.
Triku identifies genes that are locally overexpressed in groups of neighboring cells by inferring the distribution of counts in the vicinity of a cell and computing the expected distribution of counts.
the Wasserstein distance between the observed and the expected distributions is computed. Higher distances imply that the gene is locally expressed in a subset of transcriptionally similar cells. a subset of relevant features is selected using a cutoff value for the distance.

□ kmtricks: Efficient construction of Bloom filters for large sequencing data collections
>> https://www.biorxiv.org/content/10.1101/2021.02.16.429304v1.full.pdf
kmtricks, a novel approach for generating Bloom filters from terabase-sized sequencing data. Kmtricks is an efficient method for jointly counting k-mers across multiple samples, incl. a streamlined Bloom filter construction by directly counting hashes instead of k-mers.
Kmtricks takes advantage of joint counting to preserve low-abundant k-mers present in several samples, improving the recovery of non-erroneous k-mers. HowDe-SBT/kmtricks is 1-1.5x faster to construct than HowDe-SBT/KMC, 3-4x faster than HowDe-SBT/Jellyfish, 2x faster than Mantis.

□ Supervised biomedical semantic similarity
>> https://www.biorxiv.org/content/10.1101/2021.02.16.431402v1.full.pdf
This approach is independent of the semantic aspects, the specific implementation of knowledge graph-based similarity and the ML algorithm employed in regression.
This approach is able to learn a supervised semantic similarity that outperforms static semantic similarity both using KG embeddings and standard taxonomic SSMs, obtaining more accurate similarity values.

□ MQF and buffered MQF: quotient filters for efficient storage of k-mers with their counts and metadata
>> https://bmcbioinformatics.biomedcentral.com/articles/10.1186/s12859-021-03996-x
the mixed-counters quotient filter (MQF) as a new variant of the CQF with novel counting and labeling systems. MQF adapts to a wider range of data distributions for increased space efficiency and is faster than the CQF for insertions and queries in most of the tested scenarios.
MQF comes with a novel labeling system that supports associating each k-mer w/ multiple values to avoid redundant duplication of k-mers' keys in separate data structures. MQF needs just an extra O(N) operation to update the block labels where N is the number of its unique k-mers.

□ MUFFIN: Metagenomics workflow for hybrid assembly, differential coverage binning, metatranscriptomics and pathway analysis
>> https://journals.plos.org/ploscompbiol/article?id=10.1371/journal.pcbi.1008716
MUFFIN utilizes the advantages of both sequencing technologies. Short-reads provide a better representation of low abundant species due to their higher coverage based on read count. Long-reads are utilized to resolve repeats for better genome continuity.
MUFFIN is capable of enhancing the pathway results present by incorporating the data as well as the general expression level of the genes. MUFFIN executes a de novo assembly of the RNA-seq reads instead of a mapping of the reads against the MAGs to avoid bias during the mapping.

□ kLDM: Inferring Multiple Metagenomic Association Networks based on the Variation of Environmental Factors
>> https://www.sciencedirect.com/science/article/pii/S1672022921000206
the k-Lognormal-Dirichlet-Multinomial (kLDM) model, which estimates multiple association networks that correspond to specific environmental conditions, and simultaneously infers microbe-microbe and environmental factor-microbe associations for each network.
kLDM adopts a split-merge algorithm to estimate the number of environmental conditions and sparse OTU-OTU and EF-OTU associations under each environmental condition.
□ Variance Penalized On-Policy and Off-Policy Actor-Critic
>> https://arxiv.org/pdf/2102.01985.pdf
an on- and off-policy actor-critic algorithm for variance penalized objective which leverages multi- timescale stochastic approximations, where both value and variance critics are estimated in TD style.
the convergence of the algorithm to locally optimal policies for finite state action Markov decision processes. And result in trajectories with much lower variance as compared to the risk-neutral and existing indirect variance-penalized counterparts.
□ scSorter: assigning cells to known cell types according to marker genes
>> https://genomebiology.biomedcentral.com/articles/10.1186/s13059-021-02281-7
scSorter is based on the observation that marker genes, which are expected to express in higher levels in the corresponding cell types, may in practice express at a very low level in many of those cells.
scSorter takes full use of such feature and allows cells to express either at an elevated level or a base level, without a direct penalty.
□ BiSEK: a platform for a reliable differential expression analysis
>> https://www.biorxiv.org/content/10.1101/2021.02.22.432271v1.full.pdf
Biological Sequence Expression Kit (BiSEK), a graphical user interface-based platform for DEA, dedicated to a reliable inquiry. BiSEK is based on a novel algorithm to track discrepancies between the data and the statistical model design.
PaDETO (Partition Distance Explanation Tree Optimizer) tracks discrepancies in the data, alerts about problems and offers the best solutions considering the user setup, to increase reliability of the DEA output.
BiSEK enables differential-expression analysis of groups of genes, to identify affected pathways, without relying on the significance of genes comprising them.
□ WLasso: A variable selection approach for highly correlated predictors in high-dimensional genomic data
>> https://academic.oup.com/bioinformatics/advance-article-abstract/doi/10.1093/bioinformatics/btab114/6146520
Regularized approaches are classically used to perform variable selection in high-dimensional linear models. However, these methods can fail in highly correlated settings.
WLasso consists in rewriting the initial high-dimensional linear model to remove the correlation between the biomarkers (predictors) and in applying the generalized Lasso criterion.

□ Flanker: a tool for comparative genomics of gene flanking regions
>> https://www.biorxiv.org/content/10.1101/2021.02.22.432255v1.full.pdf
Flanker performs alignment-free clustering of gene flanking sequences in a consistent format, allowing investigation of MGEs without prior knowledge of their structure.
Flanker clusters flanking sequences based on Mash distances, allowing for easy comparison of similarity and the extent of this similarity across sequences
Flanker can be flexibly parameterised to finetune outputs by characterising upstream and downstream regions separately and investigating variable lengths of flanking sequence.
□ ESCO: single cell expression simulation incorporating gene co-expression
>> https://academic.oup.com/bioinformatics/advance-article-abstract/doi/10.1093/bioinformatics/btab116/6149079
ESCO adopts the idea of the copula to impose gene co-expression, while preserving the highlights of available simulators, which perform well for simulation of gene expression marginally.
Using ESCO, they assess the performance of imputation methods on GCN recovery and find that imputation generally helps GCN recovery when the data are not too sparse, and the ensemble imputation method works best among leading methods.

□ CellWalkR: An R Package for integrating single-cell and bulk data to resolve regulatory elements
>> https://www.biorxiv.org/content/10.1101/2021.02.23.432593v1.full.pdf
CellWalkR implements and extends a previously introduced network-based model that relies on a random walk with restarts model of diffusion. CellWalkR can optionally run this step on a GPU using TensorFlow3 for a greater than 15-fold speedup.
The output is a large influence matrix, portions of which are used for cell labeling, determining label similarity, embedding cells into low dimensional space, and mapping regulatory regions to cell types.
□ DeTOKI identifies and characterizes the dynamics of chromatin topologically associating domains in a single cell
>> https://www.biorxiv.org/content/10.1101/2021.02.23.432401v1.full.pdf
decode TAD boundaries that keep chromatin interaction insulated (deTOKI) from ultra-sparse Hi-C data. By nonnegative matrix factorization, this novel algorithm seeks out for regions that insulate the genome into blocks with minimal chance of clustering.
deTOKI applies non-negative matrix factorization (NMF) to decompose the Hi-C contact matrix into genome domains that may be spatially segregated in 3D space. The alternative local optimal solutions in the structure ensemble are achieved by multiple random initiations.
□ REVA as a Well-curated Database for Human Expression-modulating Variants
>> https://www.biorxiv.org/content/10.1101/2021.02.24.432622v1.full.pdf
REVA, a manually curated database for over 11.8 million experimentally tested noncoding variants with expression-modulating potentials.
REVA provides high-qualify experimentally tested expression-modulating variants with extensive functional annotations, which will be useful for users in the noncoding variants community.

□ scMoC: Single-Cell Multi-omics clustering
>> https://www.biorxiv.org/content/10.1101/2021.02.24.432644v1.full.pdf
scMoC is designed to cluster paired multimodal datasets that measures both single-cell transcriptomics sequencing (scRNA-seq) and single-cell transposase accessibility chromatin sequencing.
scMOC encompasses an RNA-guided imputation strategy to leverage the higher data sparsity. scMOC builds on the idea that cell-cell similarities can be better estimated from the RNA profiles and then used to define a neighborhood to impute from it the ATAC data.
□ sweetD: An R package using Hoeffding's D statistic to visualise the dependence between M and A for large numbers of gene expression samples
>> https://www.biorxiv.org/content/10.1101/2021.02.24.432640v1.full.pdf
Using Hoeffding’s D statistic as a non-parametric measure of dependence between M and A, so that large numbers of MA plots need not be inspected. If a sample’s D statistic is high, this means there is a relationship between M and A. this relationship can be non-monotonic.
sweetD calculates Hoeffding's D statistic for all samples relative to the median or each other, which can take any log transformed gene expression matrix as an input, and which can simultaneously visualise changes in the distribution of Hoeffding's D statistic.

□ Strainberry: Automated strain separation in low-complexity metagenomes using long reads
>> https://www.biorxiv.org/content/10.1101/2021.02.24.429166v1.full.pdf
Strainberry combines a strain-oblivious assembler with the careful use of a long-read variant calling and haplotyping tool, followed by a novel component that performs long-read metagenome scaffolding.
Strainberry is able to accurately separate strains using long reads. An average depth of coverage of 60-80X suffices to assemble individual strains of low-complexity metagenomes with almost complete coverage and sequence identity exceeding 99.9%.
□ Lasso.TopX: Machine Learning Approaches Identify Genes Containing Spatial Information From Single-Cell Transcriptomics Data
>> https://www.frontiersin.org/articles/10.3389/fgene.2020.612840/full
The NN approach utilizes weak supervision for linear regression to accommodate for uncertain or probabilistic training labels. This is especially useful to take advantage of training data generated from DistMap’s probabilistic mapping output.
Lasso.TopX, leverages linear models using the least absolute shrinkage and selection operator (Lasso), which is applied to high-dimensional single-cell sequencing data in order to accurately identify genes that contain spatial information.

□ CINS: Cell Interaction Network inference from Single cell expression data
>> https://www.biorxiv.org/content/10.1101/2021.02.22.432206v1.full.pdf
CINS combines Bayesian network analysis with regression-based modeling to identify differential cell type interactions and the proteins that underlie them.
CINS learns a regression model with ligand-target interaction matrix that identifies the key ligands and targets that participate in the interactions between these cell types. CINS correctly identifies known interacting cell type pairs and ligands associated with these interactions.
□ MONTAGE: a new tool for high-throughput detection of mosaic copy number variation
>> https://bmcgenomics.biomedcentral.com/articles/10.1186/s12864-021-07395-7
Mosaicism describes a phenomenon where a mixture of genotypic states in certain genomic segments exists within the same individual. Mosaicism is a prevalent and impactful class of non-integer state copy number variation (CNV).
Montage directly interfaces with ParseCNV2 algorithm to establish disease phenotype genome-wide association and determine which genomic ranges had more or less than expected frequency of mosaic events.
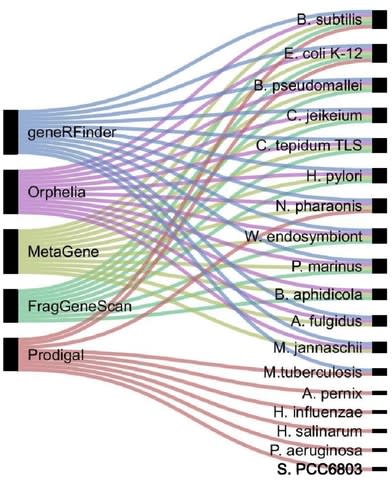
□ geneRFinder: gene finding in distinct metagenomic data complexities
>> https://bmcbioinformatics.biomedcentral.com/articles/10.1186/s12859-021-03997-w
geneRFinder, an ML-based gene predictor able to outperform state-of-the-art gene prediction tools across this benchmark by using only one pre-trained Random Forest model.
The geneRFinder is an ORF extraction based tool capable of identifying coding sequences and intergenic regions in metagenomic sequences, predicting based on the capture of signals from these regions.
□ Privacy-Preserving and Robust Watermarking on Sequential Genome Data using Belief Propagation and Local Differential Privacy
>> https://academic.oup.com/bioinformatics/advance-article-abstract/doi/10.1093/bioinformatics/btab128/6149476
a novel watermarking method on sequential genome data using belief propagation algorithm. Embedding robust watermarks so that the malicious adversaries can not temper the watermark by modification and are identified with high probability.
Achieving ε-local differential privacy in all data sharings with SPs. For the preservation of system robustness against single SP and collusion attacks. Considering publicly available genomic information like Minor Allele Frequency, Linkage Disequilibrium, Phenotype Information.
□ PICS2: Next-generation fine mapping via probabilistic identification of causal SNPs
>> https://academic.oup.com/bioinformatics/advance-article-abstract/doi/10.1093/bioinformatics/btab122/6149122
The Probabilistic Identification of Causal SNPs (PICS) algorithm and web application was developed as a fine-mapping tool to determine the likelihood that each single nucleotide polymorphism (SNP) in LD with a reported index SNP is a true causal polymorphism.
PICS2 enables performance of PICS analyses of large batches of index SNPs. And use of LD reference data generated from 1000 Genomes phase 3; annotation of variant consequences; annotation of GTEx eQTL genes and downloadable PICS SNPs from GTEx eQTLs.

□ DeepAccess: Discovering differential genome sequence activity with interpretable and efficient deep learning
>> https://www.biorxiv.org/content/10.1101/2021.02.26.433073v1.full.pdf
Differential Expected Pattern Effect (DEPE), a method to compare Expected Pattern Effects between two conditions or cell states.
DeepAccess was developed specifically for identifying cell type-specific sequence features from chromatin accessibility, Differential Expected Pattern Effect can be used to discover condition-specific sequence features from many types of experimental genome-wide sequencing data.
□ DENTIST – using long reads to close assembly gaps at high accuracy
>> https://www.biorxiv.org/content/10.1101/2021.02.26.432990v1.full.pdf
DENTIST uses uncorrected, long sequencing reads to close gaps in fragmented assemblies. DENTIST employs a reference-based consensus caller to generate high-quality consensus sequence for each closed assembly gap, maintaining a high base accuracy in the final assembly.
DENTIST is able to scaffold contigs using the given long reads. DENTIST provides a “free scaffolding mode”, where it scaffolds the given contigs just using long read alignments.
□ VarCA: Discovering single nucleotide variants and indels from bulk and single-cell ATAC-seq
>> https://www.biorxiv.org/content/10.1101/2021.02.26.433126v1.full.pdf
VarCA uses a random forest to predict indels and SNVs and achieves substantially better performance than any individual caller.
VarCA calculates the quality scores by their RF classification probabilities and fitting a linear model between the phred-scaled RF classification probabilities and empirical precision of each bin. And uses this model to calculate the final quality scores for every variant.

□ RWRF: Multi-dimensional data integration algorithm based on random walk with restart
>> https://bmcbioinformatics.biomedcentral.com/articles/10.1186/s12859-021-04029-3
RWRF (Random Walk with Restart for multi-dimensional data Fusion) uses similarity network of samples as the basis for integration. It constructs the similarity network for each data type and then connects corresponding samples of multiple similarity networks to to construct a multiplex network.
RWRF uses stationary probability distribution to fuse similarity networks. RWRF can automatically capture various structure information and make full use of topology information of the whole similarity network of each type of data.
□ Gene-Set Integrative Analysis of Multi-Omics Data Using Tensor-based Association Test
>> https://academic.oup.com/bioinformatics/advance-article-abstract/doi/10.1093/bioinformatics/btab125/6154849
A common strategy is to regress the outcomes on all omics variables in a gene set. However, this approach suffers from problems associated with high-dimensional inference.
TRinstruction, a tensor-based framework for variable-wise inference. By accounting for the matrix structure of an individual’s multi-omics data, tensor methods incorporate the relationship among omics effects, reduce the number of parameters, and boost the modeling efficiency.
□ ksrates: positioning whole-genome duplications relative to speciation events using rate-adjusted mixed paralog–ortholog KS distributions
>> https://www.biorxiv.org/content/10.1101/2021.02.28.433234v1.full.pdf
if the lineages involved exhibit different substitution rates, such direct naive comparison of paralog and ortholog KS estimates can be misleading and result in phylogenetic misinterpretation of WGD signatures.
ksrates estimates differences in synonymous substitution rates among the lineages involved and generates an adjusted mixed plot of paralog and ortholog KS distributions that allows to assess the relative phylogenetic positioning of presumed WGD and speciation events.

□ 2passtools: two-pass alignment using machine-learning-filtered splice junctions increases the accuracy of intron detection in long-read RNA sequencing
>> https://genomebiology.biomedcentral.com/articles/10.1186/s13059-021-02296-0
An alignment metrics and machine-learning-derived sequence information to filter spurious splice junctions from long-read alignments and use the remaining junctions to guide realignment in a two-pass approach.
2passtools, a method for filtered two-pass alignment of the relatively high-error long reads generated by techniques such as nanopore DRS. 2passtools, uses a rule-based approach to identify probable genuine and spurious splice junctions from first-pass read alignments.
□ GMSECT: Genome-Wide Massive Sequence Exhaustive Com-parison Tool for structural and copy number variations
>> https://www.biorxiv.org/content/10.1101/2021.03.01.433223v1.full.pdf
Most of the existing pair wise alignment tools are an extension to the dynamic programming algorithm, and though they are extensively fast in comparison to standard dynamic programming approach, they are not rapid and efficient to handle massive sequences.
The GMSECT algorithm can be implemented using other parallel application programming interfaces as well such as Posix-Threads or can even be implemented in a serial submission fashion.
※コメント投稿者のブログIDはブログ作成者のみに通知されます