
□ Mapping Cell Fate Transition in Space and Time
>> https://www.biorxiv.org/content/10.1101/2024.02.12.579941v1
TopoVelo (Topological Velocity inference) jointly infers the dynamics of cell fate transition over time and space. TopoVelo extends the RNA velocity framework to model single-cell gene expression dynamics of an entire tissue with spatially coupled differential equations.
TopoVelo models the differentiation of all cells using spatially coupled differential equations, formulates a principled Bayesian latent variable model that describes the data generation process, and derives an approximate Bayesian estimation using autoencoding variational Bayes.


□ NuPose: Genome-wide Nucleosome Positioning and Associated Features uncovered with Interpretable Deep Residual Networks
>> https://www.biorxiv.org/content/10.1101/2024.02.09.579668v1
NuPose is an interpretable framework based on the concepts of deep residual networks. NuPose able to learn sequence and structural patterns and their dependencies associated with nucleosome organization in human genome.
NuPoSe can be used to identify nucleosomal regions, not covered by experiments, and be applied to unseen data from different cell types. Their findings point to 43 informative DNA sequence features, most of them constitute tri-nucleotides, di-nucleotides and one tetra-nucleotide.

□ Scywalker: scalable end-to-end data analysis workflow for nanopore single-cell transcriptome sequencing
>> https://www.biorxiv.org/content/10.1101/2024.02.22.581508v1
Scywalker is an integrated workflow for analyzing nanopore long-read single-cell sequencing data, currently tailored to the 10x Genomics platform. Scywalker orchestrates a complete workflow from FASTQ to cell-type demultiplexed gene and isoform discovery and quantification.
Scywalker supports scalable parallelization. Most steps are subdivided into smaller jobs, which are efficiently distributed over different processing cores, either on the same computer or over different computers in a cluster.

□ ConvNet-VAE: Integrating single-cell multimodal epigenomic data using 1D-convolutional neural networks
>> https://www.biorxiv.org/content/10.1101/2024.02.16.580655v1
ConvNet-VAE is a convolutional variational autoencoder based upon a Bayesian generative model. To apply Conv1D, the input multimodal data are transformed into 3-dimensional arrays (cell x modality x bin), following window-based genome binning at 10 kilobase resolution.
The encoder efficiently extracts latent factors, which are then mapped back to the input feature space by the decoder network. ConvNet-VAE uses a discrete data likelihood (Poisson distribution) to directly model the observed raw counts.
In this model, the categorical variables (e.g., batch information) are one-hot encoded and then concatenated with the flattened convolutional layer outputs, instead of being combined directly with the multimodal fragment count data over the sorted genomic bins.

□ Discrete Probabilistic Inference as Control in Multi-path Environments
>> https://arxiv.org/abs/2402.10309
Maximum Entropy Reinforcement Learning (MaxEnt RL) to solve this problem for some distributions, it has been shown that in general, the distribution over states induced by the optimal policy may be biased in cases where there are multiple ways to generate the same object.
Generative Flow Networks (GFlowNets) learn a stochastic policy that samples objects proportionally to their reward by approximately enforcing a conservation of flows across the a finite-horizon Markov Decision Process.
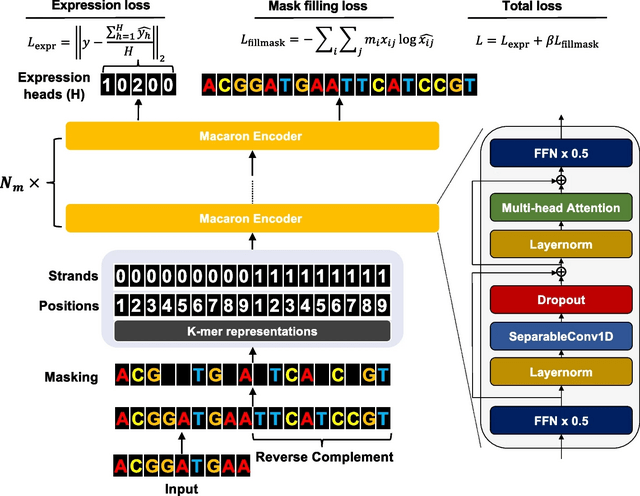
□ Proformer: a hybrid macaron transformer model predicts expression values from promoter sequences
>> https://bmcbioinformatics.biomedcentral.com/articles/10.1186/s12859-024-05645-5
Proformer, an over-parametrized Transformer architecture for large scale regression task on DNA sequences. Proformer includes a new design named multiple expression heads (MEH) to stabilize the convergence, compared with the conventional average pooling heads.
Proformer has two half-step feed forward (FFN) layers were placed at the beginning and the end of each encoder block, and a separable 1D convolution layer was inserted after the first FFN layer and in front of the multi-head attention layer.
The sliding k-mers from one-hot encoded sequences were mapped onto a continuous embedding, combined with the learned positional embedding and strand embedding (forward strand vs. reverse complemented strand) as the sequence input.


□ LineageVAE: Reconstructing Historical Cell States and Transcriptomes toward Unobserved Progenitors
>> https://www.biorxiv.org/content/10.1101/2024.02.16.580598v1
LineageVAE utilizes deep learning based on the property that cells sharing barcodes have identical progenitors. LineageVAE transforms scRNA-seq observations with an identical lineage barcode into sequential trajectories toward a common progenitor in a latent cell state space.
LineageVAE depicts sequential cell state transitions from simple snapshots and infers cell states over time. Moreover, LineageVAE can generate transcriptomes at each time point using a decoder.

□ scGIST: gene panel design for spatial transcriptomics with prioritized gene sets
>> https://genomebiology.biomedcentral.com/articles/10.1186/s13059-024-03185-y
scGIST (single-cell Gene-panel Inference for Spatial Transcriptomics), a deep neural network with a custom loss function that casts sc-ST panel design as a constrained feature selection problem.
scGIST learns to classify the individual cells given their gene expression values. Its custom loss function aims at maximizing both cell type classification accuracy and the number of genes included from a given gene set of interest while staying w/in the panel’s size constraint.

□ CADECT: Evaluating the Benefits and Limits of Multiple Displacement Amplification with Whole-Genome Oxford Nanopore Sequencing
>> https://www.biorxiv.org/content/10.1101/2024.02.09.579537v1
CADECT (Concatemer Detection Tool) enables the identification and removal of putative inverted chimeric concatemers, thus improving the accuracy and contiguity of the genome assembly.
CADECT effectively mitigates the impact of concatemeric sequences, enabling the assembly of contiguous sequences even in cases where the input genomic DNA was degraded.
Annealing of random hexamer primers and addition of phi29-DNA polymerase leads to concatemers-mediated multiple displacement amplification from linear and circular concatemers respectively.

□ NUCLUSION: Scalable nonparametric clustering with unified marker gene selection for single-cell RNA-seq data
>> https://www.biorxiv.org/content/10.1101/2024.02.11.579839v1
NUCLUSION, an infinite mixture model that leverages Bayesian sparse priors to identify marker genes while simultaneously performing clustering on single-cell expression data. NCLUSION works directly on normalized count data, bypassing the need to perform dimensionality reduction.
Based on a sparse hierarchical Dirichlet process normal mixture model, NCLUSION learns the optimal number of clusters based on the variation observed b/n expression profiles and uses sparse prior distributions to identify genes that significantly influence cluster definitions.

□ Proteus: pioneering protein structure generation for enhanced designability and efficiency
>> https://www.biorxiv.org/content/10.1101/2024.02.10.579791v1
Proteus surpasses the designability of RFdiffusion by utilizing a graph-based triangle technique and a multi-track interaction network with great enhancement of the dataset.
The graph triangle block is applied to update the edge representation and employs a graph-based attention mechanism on edge representation with a sequence representation-gated structure bias.
Proteus transfers triangle techniques into the integration of latent representation of residue edges by the construction of KNN graph and building multi-track interaction networks, Proteus even largely surpasses RF diffusion on longer monomer (over 400 amino acids) generation.
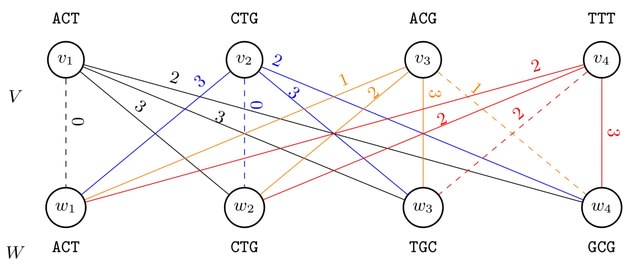

□ BMTC: The De Bruijn Mapping Problem with Changes in the Graph
>> https://www.biorxiv.org/content/10.1101/2024.02.15.580401v1
Reformulating the Graph Sequence Mapping Problem, this work introduced concepts such as the s-transformation of a De Bruijn graph and the Bipartition and matching between two sets of k-mers.
BMTC, an algorithm which utilizes the Hungarian algorithm to find a maximum-cost minimum matching in a bipartite graph, resulting in a modified set of vertices for the De Bruijn graph.
The theorem demonstrates that the cost of the maximum matching found in the bipartite graph is equal to the Hamming distance b/n the given sequence and the original graph. BMTC allows changes in the De Bruijn graph, proving advantageous for finding polynomial-time solutions.

□ RUDEUS: a machine learning classification system to study DNA-Binding proteins
>> https://www.biorxiv.org/content/10.1101/2024.02.19.580825v1
RUDEUS, a Python library for DNA-binding classification systems and recognis-ing single-stranded and double-stranded interactions.
RUDEUS incorporates a generalizable pipeline that combines protein language models, supervised learning algorithms, and hyperparameter tuning guided by Bayesian approaches to train predictive models.
RUDEUS collects the protein sequences by incorporating length filters and removing non-canonical residues. Numerical representation strategies are applied to obtain encoded vectors through protein language, and all the different pre-trained models in the bio-embedding library.

□ scSemiGCN: boosting cell-type annotation from noise-resistant graph neural networks with extremely limited supervision
>> https://academic.oup.com/bioinformatics/advance-article/doi/10.1093/bioinformatics/btae091/7609673
scSemiGCN, a robust cell-type annotation method based on graph convolutional networks. Built upon a denoised network structure that characterizes reliable cell-to-cell connections, scSemiGCN generates pseudo labels for unannotated cells.
scSemiGCN projectins raw features onto a discriminative representation space by supervised contrastive learning. Finally, message passing with the refined features over the denoised network structure is conducted for semi-supervised cell-type annotation.

□ ChemGLaM: Chemical-Genomics Language Models for Compound-Protein Interaction Prediction
>> https://www.biorxiv.org/content/10.1101/2024.02.13.580100v1
ChemGLaM is based on the 2 independent language models, MoLFormer for compounds and ESM-2 for proteins, and fine-tuned for the CPI datasets using an interaction block with a cross-attention mechanism.
ChemGLaM is capable of predicting interactions between unknown compounds and proteins with higher accuracy.
ChemGLaM combines the independently pre-trained foundation models is effective for obtaining sophisticated representation of compound-protein interactions. Furthermore, ChemGLaM visualizes the learned cross-attention map.

□ SSBlazer: a genome-wide nucleotide-resolution model for predicting single-strand break sites
>> https://genomebiology.biomedcentral.com/articles/10.1186/s13059-024-03179-w
SSBlazer is a novel computational framework for predicting Single-strand breaks (SSB) sites within local genomic windows. This method utilizes advanced deep learning techniques such as residual blocks and self-attention mechanisms to enhance the accuracy of predictions.
SSBlazer is capable of quantifying the contribution of each nucleotide to the final prediction, thereby aiding in the identification of SSB-associated motifs, such as the GGC motif and regions with a high frequency of CpG sites.

□ HairSplitter: haplotype assembly from long, noisy reads
>> https://www.biorxiv.org/content/10.1101/2024.02.13.580067v1
HairSplitter first calls variants using a custom process to distinguish actual variants from alignment or sequencing artefacts, clusters the reads into an unspecified number of haplotypes, creates the new separated contigs and finally untangles the assembly graph.
Hairsplitter takes as input an assembly (obtained by any means) and the long reads (including high-error rate long reads) used to build this assembly. For each contig it checks if the contig was built using reads from different haplotypes/regions.
Hairsplitter separates the reads into as many groups as necessary and computes the different versions (e.g. alleles) of the contig actually present in the genome. It outputs a new assembly, where different versions of contigs are not collapsed into one but assembled separately.

□ DeepMod2: A signal processing and deep learning framework for methylation detection using Oxford Nanopore sequencing
>> https://www.nature.com/articles/s41467-024-45778-y
DeepMod2 takes ionic current signal from POD5/FAST5 files and read sequences from a BAM file as input and makes 5mC methylation prediction for each read independently using a BiLSTM or Transformer model.
DeepMod2 combines per-read predictions to estimate overall methylation level for each CpG site in the reference genome. It additionally provides haplotype-specific methylation counts if the input BAM file is phased.

□ Graphasing: Phasing Diploid Genome Assembly Graphs with Single-Cell Strand Sequencing
>> https://www.biorxiv.org/content/10.1101/2024.02.15.580432v1
Graphasing, a Strand-seq alignment-to-graph-based phasing and scaffolding workflow that assembles telomere-to-telomere (T2T) human haplotypes using data from a single sample.
Graphasing leverages a robust cosine similarity clustering approach to synthesize global phase signal from Strand-seq alignments with assembly graph topology, producing accurate haplotype calls and end-to-end scaffolds.

□ Pasa: leveraging population pangenome graph to scaffold prokaryote genome assemblies
>> https://academic.oup.com/nar/article/52/3/e15/7469957
Pasa, a graph-based algorithm that utilizes the pangenome graph and the assembly graph information to impro v e scaff olding quality. Pasa is able to utilize the linkage information of the gene families of the species to resolve the contig graph of the assembly.
Pasa orients the gene-level genomes such that they have the most common consecutive gene pairs. The orientations of the gene-level genomes are determined by the following procedure: The algorithm begins with the first genome, and its orientation is chosen arbitrarily.
Pasa identifies an orientation of the second genome that maximizes the number of common pairs of consecutive genes with the first genome.
Similarly, Pasa finds an orientation of the third genome that has the largest number of common pairs of consecutive genes with the first two genomes, and the procedure is repeated for the remaining genomes.

□ TERRACE: Accurate Assembly of Circular RNAs
>> https://www.biorxiv.org/content/10.1101/2024.02.09.579380v1
TERRACE (accuraTe assEmbly of circRNAs using bRidging and mAChine lEarning), a new tool for assembling full-length circRNAs from paired-end total RNA-seq data. TERRACE stands out by assembling circRNAs accurately without relying on annotations.
TERRACE identifies back-spliced reads, which will be assembled into a set of candidate, full-length circular paths. The candidate paths, augmented by the annotated transcripts, are subjected to a selection process followed by a merging procedure to produce the resultant circRNAs.

□ TopoQual polishes circular consensus sequencing data and accurately predicts quality scores
>> https://www.biorxiv.org/content/10.1101/2024.02.08.579541v1
TopoQual, a tool utilizing partial order alignments (POA), topologically parallel bases, and deep learning to polish consensus sequences and more accurately predict base qualities.
TopoQual can find the alternative, or parallel, bases of the calling base in the POA graph. The parallel bases, in conjunction with the trinucleotide sequence of the read and the target base's quality score, are input to the deep learning model treating mismatch bases.


□ Motif Interactions Affect Post-Hoc Interpretability of Genomic Convolutional Neural Networks
>> https://www.biorxiv.org/content/10.1101/2024.02.15.580353v1
Since multiple regulatory elements can be involved in a regulatory mechanism, interactions between motifs complicate the prediction task. Motif interactions can occur in multiple forms, including additive effects as well as multiplicative interactions.
Genomic sequences have to be transformed into numerical matrices so they can be processed by CNNs. Each column of this matrix stands for one sequence position where the base at this position is represented by a one-hot-encoding vector.
They obtain real transcription factor binding motifs from the JASPAR database for the evaluation. They distinguish here between subsets of homologous and heterologous motif subsets to investigate if motif similarity influences interpretability.
Many approaches to interpreting genomic LLM models focus on the analysis of the attention scores or the output with post-hoc methods that mostly offer interpretations on the input token level.
One ongoing challenge is to uncover the grammar between interacting motifs so that interpreting genomic LLMs beyond those approaches could give better explanations of underlying biological processes.

□ SomaScan Bioinformatics: Normalization, Quality Control, and Assessment of Pre-Analytical Variation
>> https://www.biorxiv.org/content/10.1101/2024.02.09.579724v1
Pre-analytical variation (PAV) due to sample collection, handling, and storage is known to affect many analyses in molecular biology. By implementing data modeling techniques similar to those previously developed to find SomaScan signatures associated with clinical phenotypes.
SomaLogic has developed a novel set of so-called SomaSignal Tests (SSTs) to assess pre-analytical variation due to different sample processing factors, including fed-fasted time, number of freeze-thaw cycles, time-to-decant, time-to-spin, and time-to-freeze.

□ ELATUS: Uncovering functional lncRNAs by scRNA-seq
>> https://www.biorxiv.org/content/10.1101/2024.01.26.577344v2
ELATUS, a computational framework based on the pseudoaligner Kallisto that enhances the detection of functional lncRNAs previously undetected and exhibits higher concordance with the ATAC-seq profiles in single-cell multiome data.
ELATUS workflow to uncover biologically important IncRNAs. It started by importing the raw count matrices obtained after preprocessing with both Cell Ranger and Kallisto.
ATAC-seq data from the high-quality nuclei were normalized using a Latent Semantic Indexing approach. "Weighted nearest neighbour" (WNN) analysis was then performed to integrate the ATAC-seq with the gene expression obtained by Cell Ranger and Kallisto.

□ LAVASET: Latent variable stochastic ensemble of trees. An ensemble method for correlated datasets with spatial, spectral, and temporal dependencies
>> https://academic.oup.com/bioinformatics/advance-article/doi/10.1093/bioinformatics/btae101/7612229
LAVASET derives latent variables based on the distance characteristics of each feature and thereby incorporates the correlation factor in the splitting step. LAVASET inherently groups correlated features and ensures similar importance assignment for these.
LAVASET operates given a number of prerequisites and hyperparameters that can be optimized. LAVASET produces non-inferior performance results to traditional Random Forests in all but one of the examples, and in both simulated and real datasets.

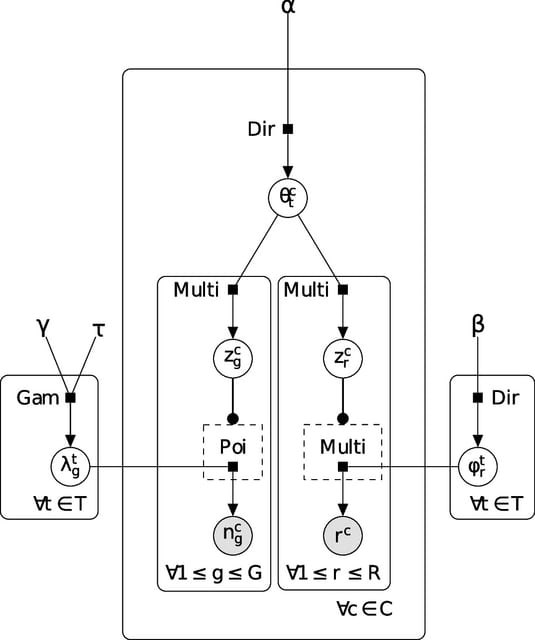
□ SHARE-Topic: Bayesian interpretable modeling of single-cell multi-omic data
>> https://genomebiology.biomedcentral.com/articles/10.1186/s13059-024-03180-3
SHARE-Topic extends the cisTopic model of single-cell chromatin accessibility by coupling the epigenomic state with gene expression through latent variables (topics) which are associated to regions and genes within an individual cell.
SHARE-Topic extracts a latent space representation of each cell informed by both the epigenome / transcriptome, but crucially also to model the joint variability of individual genes regions, providing an interpretable analysis tool which can help in generating novel hypotheses.

□ ScRAT: Phenotype prediction from single-cell RNA-seq data using Attention-Based neural networks
>> https://academic.oup.com/bioinformatics/advance-article/doi/10.1093/bioinformatics/btae067/7613064
ScRAT, a phenotype prediction framework that can learn from limited numbers of scRNA-seq samples with minimal dependence on cell-type annotations. ScRAT utilizes the attention mechanism to measure interactions between cells as their correlations, or attention weights.
ScRAT establishes the connection between the input (cells) and the output (phenotypes) of the Transformer model simply using the attention weights. ScRAT hence selects cells containing the most discriminative information to specific phenotypes, or critical cells.

□ SpaCCC: Large language model-based cell-cell communication inference for spatially resolved transcriptomic data
>> https://www.biorxiv.org/content/10.1101/2024.02.21.581369v1
spaCCC first relied on our fine-tuned single-cell LLM and functional gene interaction network to embed ligand and receptor genes expressed in interacting individual cells into a unified latent space.
Second, the ligand-receptor pairs with a significant closer distance in latent space were taken to be more likely to interact with each other.
Third, molecular diffusion and permutation test strategy were respectively employed to calculate the communication strength and filter out communications with low specificities.

□ Large-scale characterization of cell niches in spatial atlases using bio-inspired graph learning
>> https://www.biorxiv.org/content/10.1101/2024.02.21.581428v1
NicheCompass is a generative graph deep learning method designed based on the principles of cellular communication, enabling interpretable and scalable modeling of spatial omics data.
NicheCompass has a unique in-built capability for spatial reference mapping31 based on fine-tuning, thereby empowering computationally efficient integration and contextualization of a query dataset with a large-scale spatial reference atlas.

□ MaskGraphene: Advancing joint embedding, clustering, and batch correction for spatial transcriptomics using graph-based self-supervised learning
>> https://www.biorxiv.org/content/10.1101/2024.02.21.581387v1
MaskGraphene, a graph neural network with both self-supervised and self-contrastive training strategies designed for aligning and integrating ST data with gene expression and spatial location information while generating batch-corrected joint node embeddings.
MaskGraphene integrates node-to-node matching links from a local alignment algorithm. MaskGraphene selects spots across slices as triplets based on their embeddings, with the goal of bringing similar spots closer and pushing different spots further apart in an iterative manner.
※コメント投稿者のブログIDはブログ作成者のみに通知されます