
□ OPERA: Joint analysis of GWAS and multi-omics QTL summary statistics reveals a large fraction of GWAS signals shared with molecular phenotypes
>> https://www.cell.com/cell-genomics/fulltext/S2666-979X(23)00119-2
OPERA (Omics PlEiotRopic Association), a method that jointly analyzes GWAS and multi-omics xQTL summary statistics to enhance the identification of molecular phenotypes associated with complex traits through shared causal variants.
OPERA computes the posterior probabilities of associations at all xQTLs. Further analysis to distinguish causality (i.e., vertical pleiotropy) from horizontal pleiotropy requires multiple independent trans-xQTLs for a single molecular phenotype.

□ GeoDock: Flexible Protein-Protein Docking with a Multi-Track Iterative Transformer
>> https://www.biorxiv.org/content/10.1101/2023.06.29.547134v1
GeoDock, a multi-track iterative transformer network to predict a docked structure from separate docking partners. Unlike deep learning models for protein structure prediction that input multiple sequence alignments.
GeoDock inputs just the sequences and structures of the docking partners, which suits the tasks when the individual structures are given. GeoDock is flexible at the protein residue level, allowing the prediction of conformational changes upon binding.

□ GRAPE for fast and scalable graph processing and random-walk-based embedding
>> https://www.nature.com/articles/s43588-023-00465-8
GRAPE (Graph Representation Learning, Prediction and Evaluation), a software resource for graph processing and embedding that is able to scale with big graphs by using smart data structures, algorithms, and a fast parallel implementation of random-walk-based methods.
GRAPE comprises approximately 1.7 million well-documented lines of Python and Rust code and provides 69 node-embedding methods, 25 inference models, a collection of efficient graph-processing utilities, and over 80,000 graphs from the literature and other sources.

□ PyWGCNA: A Python package for weighted gene co-expression network analysis
>> https://academic.oup.com/bioinformatics/advance-article/doi/10.1093/bioinformatics/btad415/7218311
PyWGCNA stores user-specified network parameters such as the network type and major outputs such as the adjacency matrix. PyWGCNA removes overly sparse genes/transcripts or samples and lowly-expressed genes/transcripts, as well as outlier samples based on hierarchical clustering.
PyWGCNA can perform module-trait correlation, compute and summarize module eigengene expression across sample metadata categories, detecting hug genes in each module, and perform functional enrichment analysis in each module.

□ Sequence basis of transcription initiation in human genome
>> https://www.biorxiv.org/content/10.1101/2023.06.27.546584v1
Basepair resolution transcription initiation signal patterns contain signatures of underlying sequence-based transcription initiation mechanisms. Therefore, capturing how transcription initiation patterns depend on sequence patterns may allow deconvolution of such mechanisms.
Puffin computes basepair-resolution activation scores for all sequence patterns it learned. All sequence pattern activations' position-specific effects on transcription initiation are combined in log scale, which is equivalent to multiplicative combination in count scale.

□ Deep TDA: A New Algorithm for Uncovering Insights from Complex Data
>> https://mem.ai/p/vhzFdDXsmAhiDeYU5oZi
>> https://datarefiner.com/feed/why-tda
Deep TDA, a new self-supervised learning algorithm, has been developed to overcome the limitations of traditional dimensionality reduction algorithms such as t-SNE and UMAP. It is more robust to noise and outliers, can scale to complex and high-dimensional datasets.
DeepTDA can capture and represent the bigger picture of the dataset. Deep TDA consistently maintains fine-grained structure, detects and represents global structures, and groups similar data points together.
□ NimwegenLab
>> https://twitter.com/nimwegenlab/status/1676574559796101120
Perfect example of what is so terribly wrong with this field. No explanation at all of how it works or why it is better. We know it's mathematically impossible to capture all structure in an arbitrary high-dim dataset in 2D. So Q is: what structure does 'deep TDA' decide to keep?

□ scHoML: Robust joint clustering of multi-omics single-cell data via multi-modal high-order neighborhood Laplacian Matrix optimization
>> https://academic.oup.com/bioinformatics/advance-article/doi/10.1093/bioinformatics/btad414/7210258
scHoML (a multimodal high-order neighborhood Laplacian Matrix optimization framework) can robustly represent the noisy, sparse multi-omics data in a unified low- dimensional embedding space.
The cluster number determination strategy with sample specific silhouette coefficient for small sample problems as well as variance based statistical measure offers a flexible way for accurately estimating the intrinsic clusters in the data.
The computational complexity of scHoML is mainly caused by Singular Value Decomposition. The complexity of solving the quadratic programming problem is O(ε^-1V). If the algorithm has been run for t iterations, the total complexity is O(t(n^3 + n+ε^-1V).

□ A Random Matrix Approach to Single Cell RNA-seq Analysis
>> https://www.biorxiv.org/content/10.1101/2023.06.28.546922v1
A statistical model for a gene module, define the module's signal and signal strength, and then exploit existing results in random matrix theory (RMT) to analyze clustering as signal strength varies.
RMT results provide explicit formulas for the PCA under the so-celled spiked model, which decomposes a matrix into a sum of a deterministic matrix - the spike - and a random matrix.
This statistical model decomposes the scaled expression matrix into a sum of a spike, which encodes the signal, and a random matrix, which encodes noise. Their formulas predict the fraction of cells that have the same cell state as their nearest neighbor in the knn graph.

□ RaptorX-Single: single-sequence protein structure prediction by integrating protein language models
>> https://www.biorxiv.org/content/10.1101/2023.04.24.538081v2
RaptorX-Single takes an individual protein sequence as input and then feed it into protein language models to produce sequence embedding, which is then fed into a modified Evoformer module and a structure generation module to predict atom coordinates.
RaptorX-Single uses a combination of three well-developed protein language models. ESM-1b is a Transformer of ~650M parameters that was trained on UniRef50 of 27.1 million protein sequences. For ProtTrans, they use the ProtT5-XL model of 3 billion parameters.
RaptorX-Single not only runs much faster than MSA-based AlphaFold2, but also outperforms it on antibody structure prediction, orphan protein structure prediction and single mutation effect prediction.
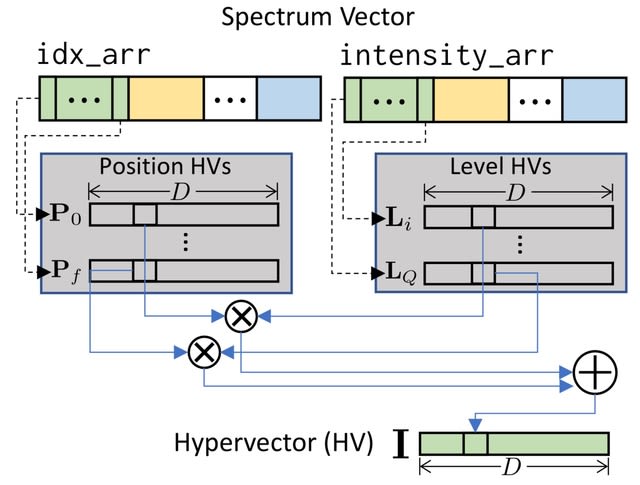
□ Accelerating Open Modification Spectral Library Searching on Tensor Core in High-dimensional Space
>> https://academic.oup.com/bioinformatics/advance-article/doi/10.1093/bioinformatics/btad404/7208862
HOMS-TC (Hyperdimensional Open Modification Search with Tensor Core acceleration) uses a new highly parallel encoding method based on the principle of hyperdimensional computing to encode mass spectral data to hypervectors while minimizing information loss.
The hypervector encoding captures spectral similarity by incorporating peak position and intensity and is tolerant to changes in peak intensity due to instrument errors or noise. HOMS-TC simplifies spectral library matching to efficient cosine similarity searching of hypervectors.

□ PepFlow: direct conformational sampling from peptide energy landscapes through hypernetwork-conditioned diffusion
>> https://www.biorxiv.org/content/10.1101/2023.06.25.546443v1
PepFlow, a hypernetwork-conditioned Boltzmann generator that enables direct all-atom sampling from the allowable conformational space of input peptide sequence.
PepFlow is trained on known molecular conformations as an score-based generative models (SGM) and is subsequently used as a probability flow ODE for sampling and training by energy.
PepFlow has a large capacity to predict both single-state structures and conformational ensembles. PepFlow can recapitulate structures found in experimentally generated ensembles of short linear motifs.

□ CARBonAra: Context-aware geometric deep learning for protein sequence design
>> https://www.biorxiv.org/content/10.1101/2023.06.19.545381v1
CARBonAra (Context-aware Amino acid Recovery from Backbone Atoms and heteroatoms), a new protein sequence generator model based on the Protein Structure Transformer (PeSTo), a geometric transformer architecture that operates on atom point clouds.
CARBonAra predicts the amino acid confidence per position from a backbone scaffold alone or complexed by any kind of non-protein molecules. CARBonAra uses geometrical transformers to encode the local neighbourhood of the atomic point cloud using the geometry and atomic elements.
CARBonAra encodes the interactions of the nearest neighbours and employs a transformer to decode and update the state of each atom. The model predicts multi-class residue-wise amino acid confidences. CARBonAra thus provides a potential sequence space.

□ CNETML: maximum likelihood inference of phylogeny from copy number profiles of multiple samples
>> https://genomebiology.biomedcentral.com/articles/10.1186/s13059-023-02983-0
CNETML, an approach based on a novel Markov model of duplication and deletion, to do maximum likelihood inference of single patient phylogeny from total copy numbers of multiple samples.
CNETS (Copy Number Evolutionary Tree Simulation), which was used to validate sample phylogeny inference methods. CNETML jointly infers the tree topology, node ages, and mutation rates of samples of different time points from (relative) total CNPs called from sWGS data.

□ Crafting a blueprint for single-cell RNA sequencing
>> https://www.cell.com/trends/plant-science/fulltext/S1360-1385(21)00247-8
Embarking on scRNA-Seq analysis in other species may require some unique protocol tweaks to isolate viable protoplasts and different thinking with regard to data annotation, but nothing insurmountable, and the richness of data will be a given.
To maximize the potential of scRNA-Seq, practical points require consideration. Principal among these are the optimization of cell-isolation procedures, accommodating biotic/abiotic stress responses, and discerning the number of cells and sequencing reads needed.

□ BioCypher: Democratizing knowledge representation
>> https://www.nature.com/articles/s41587-023-01848-y
Biomedical knowledge is fragmented across hundreds of resources. For instance, a clinical researcher may use protein information from UniProtKB genetic variants from COSMIC, protein interactions from IntAct, and information on clinical trials from ClinicalTrials.gov.
Combining these complementary datasets is a fundamental requirement for exhaustive biomedical research and thus has motivated a number of integration efforts to form harmonised knowledge graphs (i.e., knowledge representations based on a machine-readable graph structure).

□ UNRES-GPU for Physics-Based Coarse-Grained Simulations of Protein Systems at Biological Time- and Size-Scales
>> https://academic.oup.com/bioinformatics/advance-article/doi/10.1093/bioinformatics/btad391/7203798
An over 100-time speed up of the GPU code (run on an NVIDIA A100) with respect to the sequential code and an 8.5 speed-up with respect to the parallel (OpenMP) code (run on 32 cores of 2 AMD EPYC 7313 CPUs) has been achieved for large proteins (with size over 10,000 residues).
Due to the averaging over the fine-grain degrees of freedom, 1 time unit of UNRES simulations is equivalent to about 1,000 time units of laboratory time, therefore millisecond time scale of large protein systems can be reached with the UNRES-GPU code.

□ Predicting protein variants with equivariant graph neural networks
>> https://arxiv.org/abs/2306.12231
There is a research gap in comparing structure- and sequence-based methods for predicting protein variants that are better than the wildtype protein. Filling this gap by conducting a comparative study between the abilities of equivariant graph neural networks (EGNNs).
Passing the masked graph through a EGNN model to recover the score associated with each amino-acid. It generates meaningful mutations that have a higher chance of being bio-physically relevant, so they discard positions where the equivariant model makes the wrong prediction.

□ scUTRquant: Comprehensive annotation of 3′UTRs from primary cells and their quantification from scRNA-seq data
>> https://www.biorxiv.org/content/10.1101/2021.11.22.469635v2
Mapping mRNA 3′ end CS in more than 200 primary human and mouse cell types, resulting in a 40% increase of CS annotations relative to the GENCODE database.
scUTRquant quantifies a consistent set of 3'UTR isoforms, making it easier to integrate datasets. Coupled with scUTboot, significant differences in 3'UTRs across samples are identified, which allows the integration of 3'UTR quantification into standard scRNA-seq data analysis.
This data indicate that mRNA abundance and mRNA length are two independent axes of gene regulation that together determine the amount and spatial organization of protein synthesis.

□ CLOCI: Unveiling cryptic gene clusters with generalized detection
>> https://www.biorxiv.org/content/10.1101/2023.06.20.545441v1
CLOCI (Co-occurrence Locus and Orthologous Cluster Identifier), an algorithm that identifies gene clusters using multiple proxies of selection for coordinated gene evolution. CLOCI generalizes gene cluster detection and gene cluster family circumscription.
CLOCI improves detection of multiple known functional classes, and unveils noncanonical gene clusters. CLOCI is suitable for genome-enabled small molecule mining, and presents an easily tunable approach for delineating gene cluster families and homologous loci.

□ Modelling capture efficiency of single-cell RNA-sequencing data improves inference of transcriptome-wide burst kinetics
>> https://academic.oup.com/bioinformatics/advance-article/doi/10.1093/bioinformatics/btad395/7206880
A novel expression for the likelihood to be used for single-allele scRNA-seq data, which allows us to take cell-to-cell variation in cell size and capture efficiency correctly into account.
We show that numerical challenges can make maximum likelihood estimation (MLE) unreliable. To overcome this limitation, they introduce likelihood-free approaches, including a modified method of moments (MME) and two simulation-based inference methods.

□ Heuristics for the De Bruijn Graph Sequence Mapping Problem
>> https://www.biorxiv.org/content/10.1101/2023.02.05.527069v3
The Graph Sequence Mapping Problem - GSMP consists of finding a walk p in a sequence graph G that spells a sequence as similar as possible to a given sequence.
The De Bruin Graph Sequence Mapping Problem - BSMP was proved to be NP-complete considering the Hamming distance, leading to the development of a seed-and-extended heuristic.
Hirschberg reduces the quadratic space used to find an alignment for a pair of sequences using linear space by using the divide-and-conquer paradigm. De Brujin Sequance Mapping Tools can handle sequences with up to 7000 elements and graphs with with up 560,000 10-mers in 20 sec.

□ ESGq: Alternative Splicing events quantification across conditions based on Event Splicing Graphs
>> https://www.biorxiv.org/content/10.1101/2023.07.05.547757v1
ESGq, a novel approach for the quantification of AS events across conditions based on read alignment against Event Splicing Graphs. It takes as input a reference genome, a gene annotation, and a two conditions dataset with optional replicates, and computes the DE of annotated AS.
ESGq provides the Percent-Spliced In (PSI, W) with respect to each input replicate and the Ar, summarizing the differential expression of each event across the two conditions. ESGq retrieves the corresponding exons and adds them as nodes in the event splicing graph.

□ ABDS: tool suite for analyzing biologically diverse samples
>> https://www.biorxiv.org/content/10.1101/2023.07.05.547797v1
Mechanism-integrated group-wise imputation is developed to recruit signature genes involving informative missingness, cosine-based one-sample test is extended to detect enumerated signature genes, and unified heatmap is designed to comparably display complex expression patterns.
migImput imputes potentially informative missing values by considering both LLOD and MAR/MCAR mechanisms. Assessing imputation accuracy over masked values is intrinsically limited for real data because evaluation is not directly over authentic missing values.

□ SComatic: De novo detection of somatic mutations in high-throughput single-cell profiling data sets
>> https://www.nature.com/articles/s41587-023-01863-z
SComatic, an algorithm designed for the detection of somatic mutations in single-cell transcriptomic and ATAC-seq (assay for transposase-accessible chromatin sequence) data sets directly without requiring matched bulk or single-cell DNA sequencing data.
SComatic uses a panel of normals generated using a large collection of non-neoplastic samples to discount recurrent sequencing and mapping artefacts. For example, in 10× Genomics Chromium data, recurrent errors are enriched in LINE and SINE elements, such as Alu elements.

□ Genozip Deep: Deep FASTQ and BAM co-compression in Genozip 15
>> https://www.biorxiv.org/content/10.1101/2023.07.07.548069v1
The IGM acts as a long-term repository for off-machine raw sequencing data (FAST files) of internally and externally sequenced samples. Currently IGM has around 5 petabytes of storage of which the vast majority are FASTO files compressed with gzip and BAM/CRAM files.
Genozip Deep, a method for losslessly co-compressing FAST and BAM files. Improvements of 75% to 96% versus the already-compressed source files, translating to 2.3X to 6.8X better compression than current state-of-the-art algorithms that compress FAST and BAM separately.

□ SpaceANOVA: Spatial co-occurrence analysis of cell types in multiplex imaging data using point process and functional ANOVA
>> https://www.biorxiv.org/content/10.1101/2023.07.06.548034v1
SpaceANOVA, a highly powerful method to study differential spatial co-occurrence of cell types across multiple tissue or disease groups, based on the theories of the Poisson point process (PPP) and functional analysis of variance.
SpaceANOVA accommodates multiple images per subject and addresses the problem of missing tissue regions, commonly encountered in such a context due to the complex nature of the data-collection procedure.

□ STACAS: Semi-supervised integration of single-cell transcriptomics data
>> https://www.biorxiv.org/content/10.1101/2023.07.07.548105v1
STACAS v2, a semi-supervised scRNA-seq data integration method that leverages prior knowledge in the form of cell type annotations to preserve biological variance during integration.
STACAS v2 introduces the ability to use prior information, in terms of cell type labels, to refine the anchor set. STACAS outperforms popular unsupervised methods such as Harmony, FastMNN, Seurat v4, scVI, and Scanorama, as well as supervised methods such as scANVI and scGen.

□ Dromi: Python package for parallel computation of similarity measures among vector-encoded sequences
>> https://www.biorxiv.org/content/10.1101/2023.07.05.547866v1
Dromi, a simple python package that can compute different similarity measurements (i.e percent identity, cosine similarity, kmer similarities) across aligned vector-encoded sequences.
Dromi introduces the novel positional weights, meaning the cosine similarities as a measure of conservation across sequence elements such as residues in aligned biological sequences at the same position.

□ SPIN-CGNN: Improved fixed backbone protein design with contact map-based graph construction and contact graph neural network
>> https://www.biorxiv.org/content/10.1101/2023.07.07.548080v1
SPIN-CGNN, a deep graph neural network-based method for the fixed backbone design, in which a protein structure graph is constructed with a distance-based contact map. This graph construction enables GNN to handle a varied number of neighbors within a preset distance cutoff.
The symmetric edge information enabled information sharing inside an edge pair that connects two nodes. The information on second-order edges is expected to capture high-order interactions between two nodes from their shared neighbors.

□ LSMMD-MA: Scaling multimodal data integration for single-cell genomics data analysis
>> https://academic.oup.com/bioinformatics/advance-article/doi/10.1093/bioinformatics/btad420/7221538
MMD-MA maps each cell in each modality to a shared, low-dimensional space. A matching term based on the squared maximum mean discrepancy (MMD) w/ a Gaussian radial basis function (RBF) kernel ensures that the different modalities overlap in the representation space.
LSMMD-MA, a large-scale Python implementation of the MMD-MA method for multimodal data integration. LSMMD-MA reformulates the MMD-MA optimization problem using linear algebra and solve it with KeOps, a CUDA framework for symbolic matrix computation.
※コメント投稿者のブログIDはブログ作成者のみに通知されます