
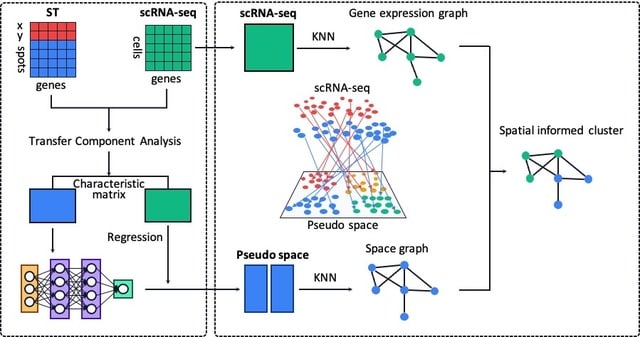
□ scSpace: Reconstruction of the cell pseudo-space from single-cell RNA sequencing data
>> https://www.biorxiv.org/content/10.1101/2022.05.07.491043v1.full.pdf
single-cell Spatial Position Associated Co-Embeddings (scSpace), an integrative algorithm to distinguish spatially variable cell subclusters by reconstructing cells onto a pseudo-space with spatial transcriptome references.
scSpace projects single cells into a pseudo-space via a Multi-layer Neural Network model, so that gene expression graph and spatial graph of cells can be embedded jointly for the further spatial reconstruction and space-informed cell clustering with higher accuracy and precision.

資源集約的な解析技術は、高スループットのbulk dataに対し体系的に適用することが出来ない。その為、データ駆動型のスケーリング因子を用いて事前に定義された疑似バルクデータをシミュレーションすることにより、現実のデータの統計的特徴を再現することが可能である。

□ SimBu: Bias-aware simulation of bulk RNA-seq data with variable cell type composition
>> https://www.biorxiv.org/content/10.1101/2022.05.06.490889v1.full.pdf
SimBu is a user-friendly and flexible tool for simulating realistic pseudo-bulk RNA-seq datasets serving as in silico gold-standard for assessing cell-type deconvolution methods.
A unique feature of SimBu is the modelling of cell-type-specific mRNA bias using experimentally or data-driven scaling factors. SimBu can use Smart-seq2 or 10x Genomics data to generate pseudo-bulk data that faithfully reflects the statistical features of true bulk RNA-seq data.

□ RECODE: Resolution of the curse of dimensionality in single-cell RNA sequencing data analysis
>> https://www.biorxiv.org/content/10.1101/2022.05.02.490246v1.full.pdf
RECODE (resolution of the curse of dimensionality) consistently eliminates COD in relevant scRNA-seq data with unique molecular identifiers. RECODE employs different principles and exhibits superior overall performance in cell-clustering and single-cell level analysis.
RECODE does not involve dimension reduction and recovers expression values for all genes, including lowly expressed genes, realizing precise delineation of cell-fate transitions and identification of rare cells with all gene information.

□ SMURF: embedding single-cell RNA-seq data with matrix factorization preserving selfconsistency
>> https://www.biorxiv.org/content/10.1101/2022.04.22.489140v1.full.pdf
SMURF embeds cells and genes into their latent space vectors utilizing matrix factorization with a mixture of Poisson-Gamma divergent as objective while preserving self-consistency. SMURF exhibited feasible cell subpopulation discovery efficacy with the latent vectors.
SMURF can embed the cell latent vectors into a 1D-oval and recover the time course of the cell cycle. SMURF paraded the most robust gene expression recovery power with low root mean square error and high Pearson correlation.

□ TopoGAN: Unsupervised manifold alignment of single-cell data
>> https://www.biorxiv.org/content/10.1101/2022.04.27.489829v1.full.pdf
TopoGAN, a topology-preserving multi-modal alignment of two single-cell modalities w/ non-overlapping cells or features. TopoGAN finds topology-preserving latent representations of the different modalities, which are then aligned in an unsupervised way using a topology-guided GAN.
The latent space representation of the two modalities are aligned in a topology-preserving manner. TopoGAN uses a topological autoencoder, which chooses point-pairs that are crucial in defining the topology of the manifold instead of trying to optimize all possible point-pairs.

□ AutoClass: A universal deep neural network for in-depth cleaning of single-cell RNA-Seq data
>> https://www.nature.com/articles/s41467-022-29576-y
AutoClass integrates two DNN components, Autoencoder / Classifier, as to maximize both noise removal and signal retention. AutoClass is distribution agnostic as it makes no assumption on specific data distributions, hence can effectively clean a wide range of noise and artifacts.
AutoClass is robust on key hyperparameter settings: i.e. bottleneck layer size, pre-clustering number and classifier weight. AutoClass does not presume any specific type or form of data distribution, hence has the potential to correct a wide range noises and non-signal variances.

□ TAMPA: interpretable analysis and visualization of metagenomics-based taxon abundance profiles
>> https://www.biorxiv.org/content/10.1101/2022.04.28.489926v1.full.pdf
TAMPA (Taxonomic metagenome profiling evaluation) , a robust and easy-to-use method that allows scientists to easily interpret and interact with taxonomic profiles produced by the many different taxonomic profiler methods beyond the standard metrics used by the scientific community.
TAMPA allows for users to choose among multiple graph layout formats, including pie, bar, circle and rectangular. TAMPA can illuminate important biological differences between the two tools and the ground truth at the phylum level, as well as at all other taxonomic ranks.
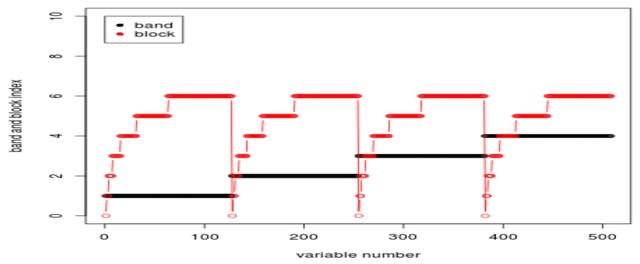
□ Threshold Values for the Gini Variable Importance: A Empirical Bayes Approach
>> https://www.biorxiv.org/content/10.1101/2022.04.06.487300v1.full.pdf
It is highly desirable that RF models be made more interpretable and a large part of that is a better understanding of the characteristics of the variable importance measures generated by the RF. Considering the mean decrease in node “impurity” (MDI) variable importance (VI).
Efron’s “local fdr” approach, calculated from an empirical Bayes estimate of the null distribution. the distribution may be multi-modal, which creates modelling difficulties; – the null distribution is not of an obvious form, as it is not symmetric.
□ Weighted Kernels Improve Multi-Environment Genomic Prediction
>> https://www.biorxiv.org/content/10.1101/2022.04.10.487783v1.full.pdf
A flexible GS framework capable of incorporating important genetic attributes to breeding populations and trait variability while addressing the shortcomings of conventional GS models.
Comparing to the existing Gaussian Kernel (GK) that assigns a uniform weight to every SNP, This Weighted Kernel (WK) captured more robust genetic relationship of individuals within and cross environments by differentiating the contribution of SNPs.

□ Pangolin: Predicting RNA splicing from DNA sequence
>> https://genomebiology.biomedcentral.com/articles/10.1186/s13059-022-02664-4
Pangolin can predict the usage of a splice site in addition to the probability that it is spliced. Pangolin improves prediction of the impact of genetic variants on RNA splicing, including common, rare, and lineage-specific genetic variation.
Pangolin’s architecture resembles that used in SpliceAI, which allows modeling of features from up to 5000 base pairs. Pangolin identifies loss-of-function mutations with high accuracy and recall, particularly for mutations that are not missense or nonsense.

□ MISTy: Explainable multiview framework for dissecting spatial relationships from highly multiplexed data
>> https://genomebiology.biomedcentral.com/articles/10.1186/s13059-022-02663-5
MISTy facilitates an in-depth understanding of marker interactions by profiling the intra- and intercellular relationships. MISTy builds multiple views focusing on different spatial or functional contexts to dissect different effects.
MISTy allows for a hypothesis-driven and composition of views that fit the application of interest. The views capture functional relationships, such as pathway activities and crosstalk, cell-type-specific relationships, or focus on relations b/n different anatomical regions.

□ wenda_gpu: fast domain adaptation for genomic data
>> https://www.biorxiv.org/content/10.1101/2022.04.09.487671v1.full.pdf
Weighted elastic net domain adaptation exploits the complex biological interactions that exist between genomic features to maximize transferability to a new context.
wenda_gpu uses GPyTorch, which provides efficient and modular Gaussian process inference. Using wenda_gpu, completing the whole prediction task on genome-wide datasets with tens of thousands of features is thus feasible in a single day on a single GPU-enabled computer.

□ CHAPAO: Likelihood and hierarchical reference-based representation of biomolecular sequences and applications to compressing multiple sequence alignments
>> https://journals.plos.org/plosone/article?id=10.1371/journal.pone.0265360
CHAPAO (COmpressing Alignments using Hierarchical and Probabilistic Approach), a new lossless compression which is especially designed for multiple sequence alignments (MSAs) of biomolecular data.
CHAPAO combines likelihood based analyses of the sequence similarities and graph theoretic algorithms. CHAPAO has achieved more compression on the MSAs with less average pairwise hamming distance among the sequences.

□ Using topic modeling to detect cellular crosstalk in scRNA-seq
>> https://journals.plos.org/ploscompbiol/article?id=10.1371/journal.pcbi.1009975
A new method based on Latent Dirichlet Allocation (LDA) for detecting genes that change as a result of interaction. This method does not require prior information in the form of clustering or generation of synthetic reference profiles.
The model has been applied to two datasets of sequenced PICs and a dataset generated by standard 10x Chromium. Its approach assumes there is a reference population that can be used to fit the first LDA; for example this could be populations before an interaction has occurred.

□ DRUMMER—Rapid detection of RNA modifications through comparative nanopore sequencing
>> https://academic.oup.com/bioinformatics/advance-article/doi/10.1093/bioinformatics/btac274/6569078
DRUMMER (Detection of Ribonucleic acid Modifications Manifested in Error Rates) utilizes a range of statistical tests and background noise correction to identify modified nucleotides, operates w/ similar sensitivity to signal-level analysis, and correlates very well w/ orthogonal approaches.
DRUMMER can process both genome-level and transcriptome-level alignments. DRUMMER uses sequence read alignments against a genome to predict the location of putative RNA modifications in a genomic context.

□ Neural network approach to somatic SNP calling in WGS samples without a matched control.
>> https://www.biorxiv.org/content/10.1101/2022.04.14.488223v1.full.pdf
A neural network-based approach for calling somatic single nucleotide polymorphism (SNP) variants in tumor WGS samples without a matched normal.
The method relies on recent advances in artefact filtering as well as on state-of-the-art approaches to germline variant removal in single-sample calling. In the core of the method is a neural network classifier trained using 3D tensors consisting of piledup variant reads.

□ BioAct: Biomedical Knowledge Base Construction using Active Learning
>> https://www.biorxiv.org/content/10.1101/2022.04.14.488416v1.full.pdf
BioAct, is based on a partnership between automatic annotation methods (leveraging SciBERT with other machine learning models) and subject matter experts and uses active learning to create training datasets in the biological domain.
BioAct can be used to effectively increase the ability of a model to construct a correct knowledge base. The labels created using BioAct continuously improve the ability of a model to augment an existing seed knowledge base through many iterations of active learning.

□ Rye: genetic ancestry inference at biobank scale
>> https://www.biorxiv.org/content/10.1101/2022.04.15.488477v1.full.pdf
Rye (Rapid ancestrY Estimation) is a large scale global ancestry inference algorithm that works from principal component analysis (PCA) data. The PCA data (eigenvector and eigenvalue) reduces the massive genomic scale comparison to a much smaller matrix solving problem.
Rye infers GA based on PCA of genomic variant samples from ancestral reference populations and query individuals. The algorithm’s accuracy is powered by Metropolis-Hastings optimization and its speed is provided by non-negative least squares (NNLS) regression.

□ USAT: a Bioinformatic Toolkit to Facilitate Interpretation and Comparative Visualization of Tandem Repeat Sequences
>> https://www.biorxiv.org/content/10.1101/2022.04.15.488513v1.full.pdf
A conversion between sequence-based alleles and length-based alleles (i.e., the latter being the current allele designations in the CODIS system) is needed for backward compatibility purposes.
Universal STR Allele Toolkit (USAT) provides a comprehensive set of functions to analyze and visualize TR alleles, including the conversion between length-based alleles and sequence-based alleles, nucleotide comparison of TR haplotypes and an atlas of allele distributions.

□ Dug: A Semantic Search Engine Leveraging Peer-Reviewed Knowledge to Query Biomedical Data Repositories
>> https://academic.oup.com/bioinformatics/advance-article/doi/10.1093/bioinformatics/btac284/6571145
Dug applies semantic web and knowledge graph methods to improve the FAIR-ness of research data. A key obstacle to leveraging this knowledge is the lack of researcher tools to navigate from a set of concepts of interest towards relevant study variables. In a word, search.
Dug's ingest uses the Biolink upper ontology to annotate knowledge graphs and structure queries used to drive full text indexing and search. It uses Monarch Initiative APIs to perform named entity recognition on natural language prose to extract ontology identifiers.

□ Persistent Memory as an Effective Alternative to Random Access Memory in Metagenome Assembly
>> https://www.biorxiv.org/content/10.1101/2022.04.20.488965v1.full.pdf
PMem is a cost- effective option to extend the scalability of metagenome assemblers without requiring software refactoring, and this likely applies to similar memory-intensive bioinformatics solutions.

□ Fast and robust imputation for miRNA expression data using constrained least squares
>> https://bmcbioinformatics.biomedcentral.com/articles/10.1186/s12859-022-04656-4
A novel, fast method for data imputation using constrained Conjugate Gradient Least Squares (CGLS) borrowing ideas from the imaging and inverse problems literature.
The method will be denoted by Fast Linear Imputation. Reconstructing the missing data via nonnegative constrained regression, but with the further constraint that the regression weights sum to 1.

□ SCAMPP: Scaling Alignment-based Phylogenetic Placement to Large Trees
>> https://ieeexplore.ieee.org/document/9763324/
SCAMPP (SCAlable alignMent-based Phylogenetic Placement), a technique to extend the scalability of these likelihood-based placement methods to ultra-large backbone trees.

□ Spycone: Systematic analysis of alternative splicing in time course data
>> https://www.biorxiv.org/content/10.1101/2022.04.28.489857v1.full.pdf
Spycone uses gene or isoform expression as an input. Spycone features a novel method for IS detection and employs the sum of changes of all isoforms relative abundances (total isoform usage) across time points.
Spycone provides downstream analysis such as clustering by total isoform usage, i.e. grouping genes that are most likely to be coregulated, and network enrichment, i.e. extracting subnetworks or pathways that are over-represented by a list of genes.

□ scMOO: Imputing dropouts for single-cell RNA sequencing based on multi-objective optimization
>> https://academic.oup.com/bioinformatics/advance-article-abstract/doi/10.1093/bioinformatics/btac300/6575885
scMOO is different from existing ones, which assume that the underlying data has a preconceived structure and impute the dropouts according to the information learned from such structure.
the data combines three types of latent structures, including the horizontal structure (genes are similar to each other), the vertical structure (cells are similar to each other), and the low-rank structure.
The combination weights and latent structures are learned using multi-objective optimization. And, the weighted average of the observed data and the imputation results learned from the three types of structures are considered as the final result.

□ Improving the RNA velocity approach using long-read single cell sequencing
>> https://www.biorxiv.org/content/10.1101/2022.05.02.490352v1.full.pdf
Region velocity is a multi-platform and multi-model parameter to project cell state, which is based on long-read scRNA-seq.
Region velocity is primarily observed through the spindle-shaped relationship between the number of exons and introns in different genes, representing a steady-state model of the original RNA velocity parameter, and their correlation level varies in different genes.

□ GEMmaker: process massive RNA-seq datasets on heterogeneous computational infrastructure
>> https://bmcbioinformatics.biomedcentral.com/articles/10.1186/s12859-022-04629-7
GEMmaker, is a nf-core compliant, Nextflow workflow, that quantifies gene expression from small to massive RNA-seq datasets.
GEMmaker ensures results are highly reproducible through the use of versioned containerized software that can be executed on a single workstation, institutional compute cluster, Kubernetes platform or the cloud.

□ Hierarch: Analyzing nested experimental designs—A user-friendly resampling method to determine experimental significance
>> https://journals.plos.org/ploscompbiol/article?id=10.1371/journal.pcbi.1010061
Hierarch can be used to perform hypothesis tests that maintain nominal Type I error rates and generate confidence intervals that maintain the nominal coverage probability without making distributional assumptions about the dataset of interest.

□ HGGA: hierarchical guided genome assembler
>> https://bmcbioinformatics.biomedcentral.com/articles/10.1186/s12859-022-04701-2
HGGA a method for assembling read data with the help of genetic linkage maps. HGGA produces more misassemblies than Kermit but less than miniasm, and produces a similar number of misassemblies as Kermit but less than miniasm.
HGGA does not do scaffolding, the process of ordering the contigs into scaffolds where contigs are separated by gaps. A scaffolding method could be run after HGGA to further increase the contiguity of the assembly. HGGA is inherently easy to parallelize beyond a single machine.

□ CSREP: A framework for summarizing chromatin state annotations within and identifying differential annotations across groups of samples
>> https://www.biorxiv.org/content/10.1101/2022.05.08.491094v1.full.pdf
CSREP takes as input chromatin state annotations for a group of samples and then probabilistically estimates the state at each genomic position and derives a representative chromatin state map for the group.
CSREP uses an ensemble of multi-class logistic regression classifiers to predict the chromatin state assignment of each sample given the state maps from all other samples.

□ Limited overlap of eQTLs and GWAS hits due to systematic differences in discovery
>> https://www.biorxiv.org/content/10.1101/2022.05.07.491045v1.full.pdf
eQTLs cluster strongly near transcription start sites, while GWAS hits do not. Genes near GWAS hits are enriched in numerous functional annotations, are under strong selective constraint and have a complex regulatory landscape across different tissue/cell types.

□ TT-Mars: structural variants assessment based on haplotype-resolved assemblies
>> https://genomebiology.biomedcentral.com/articles/10.1186/s13059-022-02666-2
TT-Mars takes advantage of the recent production of high-quality haplotype-resolved genome assemblies by providing false discovery rates for variant calls based on how well their call reflects the content of the assembly, rather than comparing calls themselves.
TT-Mars inherently provides a rough estimate of sensitivity because it does not fit into the paradigm of comparing inferred content, and requires variants to be called.
This estimate simply considers false negatives as variants detected by haplotype-resolved assemblies that are not within the vicinity of the validated calls, and one should consider a class of variant that may have multiple representations when reporting results.

□ MuSiC2: cell type deconvolution for multi-condition bulk RNA-seq data
>> https://www.biorxiv.org/content/10.1101/2022.05.08.491077v1.full.pdf
MuSiC2 is an iterative algorithm that aims to improve cell type deconvolution for bulk RNA-seq data using scRNA-seq data as reference when the bulk data are generated from samples with multiple clinical conditions where at least one condition is different from the scRNA-seq reference.
MuSiC2 takes two datasets as input, a scRNA-seq data generated from one clinical condition, and a bulk RNA-seq dataset collected from samples with multiple conditions in which one or more is different from the single-cell reference data.

□ Assembly-free discovery of human novel sequences using long reads
>> https://www.biorxiv.org/content/10.1101/2022.05.06.490971v1.full.pdf
An Assembly-Free Novel Sequence (AF-NS) performs quick identification of novel sequences without assembling processes. the AF-NS detected novel sequences covered over 90% of Illumina novel sequences and contained more DNA information missing from the Illumina data.
A single read can decipher large structural variations, which guarantees the feasibility of AF-NS to discover novel sequences at read level. All ONT long reads were aligned to references using minimap 2.17-r941, and reads with unmapped fragments longer than 300bp were selected.

□ MAGNETO: an automated workflow for genome-resolved metagenomics
>> https://www.biorxiv.org/content/10.1101/2022.05.06.490992v1.full.pdf
MAGNETO, an automated workflow dedicated to MAGs reconstruction, which includes a fully-automated co-assembly step informed by optimal clustering of metagenomic distances, and implements complementary genome binning strategies, for improving MAGs recovery.
□ The limitations of the theoretical analysis of applied algorithms
>> https://arxiv.org/pdf/2205.01785.pdf
Merge sort runs in O (n log n) worst-case time, which formally means that there exists a constant c such that for any large-enough input of n elements, merge sort takes at most cn log n time.
An inter-disciplinary field that uses algorithms to extract biological meaning from genome sequencing data. Demonstrating two concrete examples of how theoretical analysis has failed to achieve its goals but also give one encouraging example of success.
※コメント投稿者のブログIDはブログ作成者のみに通知されます