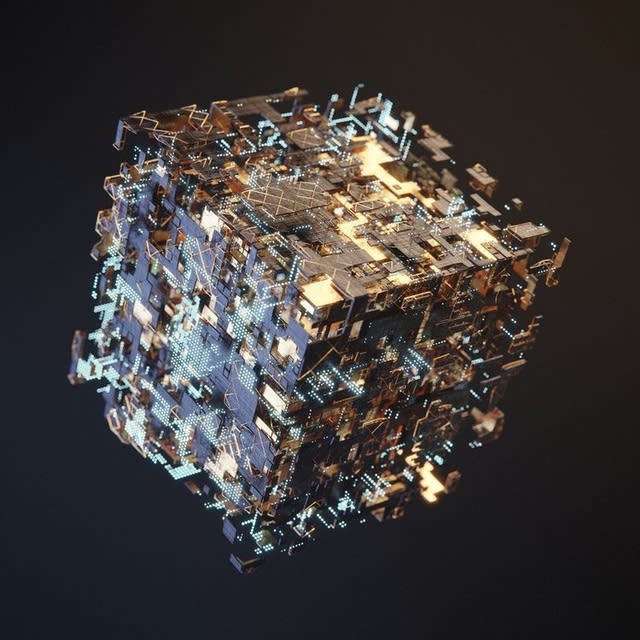
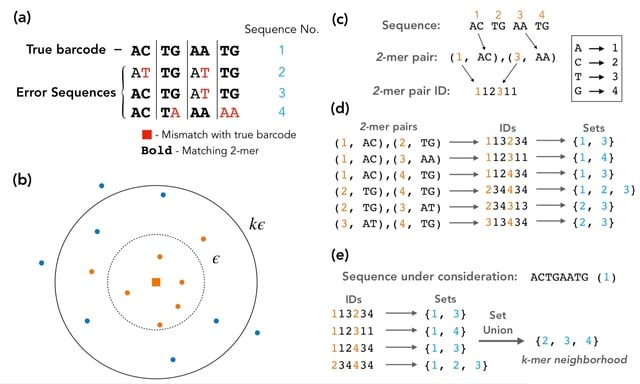
□ Shepherd: Accurate Clustering for Correcting DNA Barcode Errors
>> https://academic.oup.com/bioinformatics/advance-article/doi/10.1093/bioinformatics/btac395/6609174
Shepherd, a novel clustering method that is based on an indexing system of barcode sequences using k-mers, and a Bayesian statistical test incorporating a substitution error rate to distinguish true from error sequences.
Shepherd provides barcode count estimates that are significantly more accurate, producing 10-150 times fewer spurious lineages. Shepherd introduces the novel capability of tracking lineages that are undetectable in the first time point but emerge at later time points.
Shepherd exploits the pigeonhole principle to efficiently find neighborhoods for each sequence using the k-mer indexing system. It enables identification of sequence neighborhoods, and can be applied to any neighborhood identification task involving the Hamming distance.
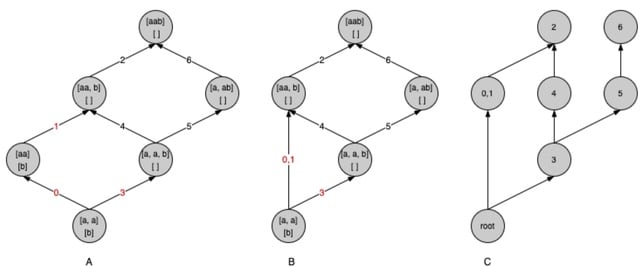
□ Graph-based algorithms for Laplace transformed coalescence time distributions.
>> https://www.biorxiv.org/content/10.1101/2022.05.20.492768v1.full.pdf
Using the Laplace transform, this distribution can be generated with a simple recursive procedure, regardless of model complexity.
Assuming an infinite-sites mutation model, the probability of observing specific configurations of linked variants within small haplotype blocks can be recovered from the Laplace transform of the joint distribution of branch lengths.
The state space diagram can be turned into a computational graph, allowing efficient evaluation of the Laplace transform by means of a graph traversal algorithm. This algorithm can be applied to tabulate the likelihoods of mutational configurations in non-recombining blocks.


□ scTite: Entropy-based inference of transition states and cellular trajectory for single-cell transcriptomics
>> https://academic.oup.com/bib/advance-article-abstract/doi/10.1093/bib/bbac225/6607748
scTite uses a new metric called transition entropy met to measure the uncertainty of a cell belonging to different cell clusters, and then identify cell states and transition cells.
scTite utilizes the Wasserstein distance on the probability distribution, and construct the minimum spanning tree. It adopts the signaling entropy / partial correlation coefficient to determine transition paths, which contain a group of transition cells w/ the largest similarity.

□ A survey of mapping algorithms in the long-reads era
>> https://www.biorxiv.org/content/10.1101/2022.05.21.492932v1.full.pdf
The unprecedented characteristics of this new type of sequencing data created a shift, and methods moved on from the seed-and-extend framework previously used for short reads to a seed-and-chain framework due to the abundance of seeds in each read.
The long-read mapping algorithms are based on alternative seed constructs or chaining formulations. The usage of diagonal-transition algorithms which was initially define for edit distance has been reactivated for the gap-affine model with the wavefront alignment algorithm.

□ DNAscope: High accuracy small variant calling using machine learning
>> https://www.biorxiv.org/content/10.1101/2022.05.20.492556v1.full.pdf
As a successor to GATK HaplotypeCaller, DNAscope uses a similar logical architecture, but introduces improvements to active region detection and local assembly for improved sensitivity and robustness, especially across high-complexity regions.
DNAscope can be used with a Bayesian genotyping model, allowing users to benefit from DNAscope’s improved active region detection and local assembly when resequencing diverse organisms.
Sequence reads aligned across active regions undergo local assembly using de Bruijn graphs and read-haplotype likelihoods are calculated through PairHMM.
Gradient Boosting Machines (GBMs) build trees in succession to train sequential ensembles of weak, base learners, reducing residuals in a stepwise fashion.
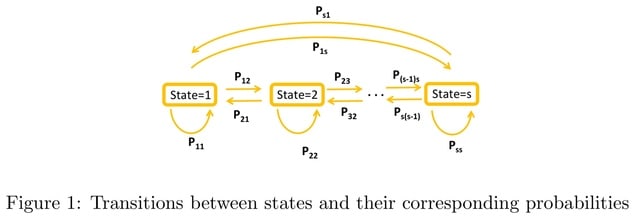
□ A scalable approach for continuous time Markov models with covariates
>> https://www.biorxiv.org/content/10.1101/2022.06.06.494953v1.full.pdf
Using a mini-batch stochastic gradient descent algorithm which uses a smaller random subset of the dataset at each iteration, making it practical to fit large scale data.
An optimization technique for continuous time Markov models (CTMM) which uses a stochastic gradient descent algorithm combined with differentiation of the matrix exponential using a Pad ́e approximation.

□ DeepLUCIA: predicting tissue-specific chromatin loops using Deep Learning-based Universal Chromatin Interaction Annotator
>> https://academic.oup.com/bioinformatics/advance-article-abstract/doi/10.1093/bioinformatics/btac373/6596048
DeepLUCIA (Deep Learning-based Universal Chromatin Interaction Annotator) does not use TF binding profile data which previous TF binding-dependent methods critically rely on, its prediction accuracies are comparable to those of the previous TF binding-dependent methods.
DeepLUCIA enables the tissue-specific chromatin loop predictions from tissue-specific epigenomes that cannot be handled by genomic variation-based approach.

□ scCNC: A method based on Capsule Network for Clustering scRNA-seq Data
>> https://academic.oup.com/bioinformatics/advance-article-abstract/doi/10.1093/bioinformatics/btac393/6608086
When confronted by the high dimensionality and general dropout events of scRNA-seq data, purely unsupervised clustering methods may not produce biologically interpretable clusters, which complicates cell type assignment.
scCNC, a semi-supervised clustering method based on a capsule network, that integrates domain knowledge into the clustering step. A Semi-supervised Greedy Iterative Training (SGIT) method used to train the whole network.

□ MGMM: Fitting Gaussian mixture models on incomplete data
>> https://bmcbioinformatics.biomedcentral.com/articles/10.1186/s12859-022-04740-9
MGMM, missingness-aware Gaussian mixture models for fitting GMMs in the presence of missing data. Unlike existing GMM implementations that can accommodate missing data, MGMM places no restrictions on the form of the covariance matrix.
MGMM employs an Expectation Conditional Maximization algorithm, which accelerates estimation by breaking direct maximization of the EM objective function into a sequence of simpler conditional maximizations. It handles both missingness of the cluster assignments and of elements.

□ Biomarker identification by reversing the learning mechanism of an autoencoder and recursive feature elimination
>> https://pubs.rsc.org/en/content/articlelanding/2022/mo/d1mo00467k
An autoencoder-based biomarker identification method by reversing the learning mechanism.
By reversing the learning mechanism of the trained autoencoders, they devised an explainable post hoc methodology for identifying the influential genes with a high likelihood of becoming biomarkers.

□ kngMap: Sensitive and Fast Mapping Algorithm for Noisy Long Reads Based on the K-Mer Neighborhood Graph
>> https://www.frontiersin.org/articles/10.3389/fgene.2022.890651/full
kngMap, a k-mer neighborhood graph mapper which is specifically designed to improve mapping sensitivity and deal with SV events. kngMap constructs a searching index for the reference genome to quickly find matched k-mers for query reads.
Such matches are then used to construct a k-mer d-neighborhood graph where matched k-mers are viewed as vertices and each pair of matched k-mers is connected by a direct edge.
kngMap has superior ability in terms of base-level sensitivity and end-to-end alignment, which can produce consecutive alignments for the whole read.

□ PCAone: fast and accurate out-of-core PCA framework for large scale biobank data
>> https://www.biorxiv.org/content/10.1101/2022.05.25.493261v1.full.pdf
PCAone uses a window based optimization scheme based on blocks of data which allows the algorithm to converge within a few passes through the whole data.
PCAone implements 3 fast PCA algorithms for finding the top eigenvectors of large datasets, which are Implicitly Restarted Arnoldi Method (IRAM), single pass Randomized SVD (RSVD) and our own fancy RSVD method with window based power iterations.

□ scEFSC: Accurate single-cell RNA-seq data analysis via ensemble consensus clustering based on multiple feature selections
>> https://www.sciencedirect.com/science/article/pii/S2001037022001416
scEFSC, a single-cell consensus clustering algorithm based on ensemble feature selection for scRNA-seq data analysis in an ensemble manner. the algorithm employs several unsupervised feature selections to remove genes that do not contribute significantly to the scRNA-seq data.
scEFSC algorithm exhibited superior clustering performance on the 14 scRNA-seq datasets, indicating that using multiple unsupervised feature selection algorithms can strengthen the clustering ability of consensus clustering over a single unsupervised feature selection algorithm.

□ Cello scope: a probabilistic model for marker-gene-driven cell type deconvolution in spatial transcriptomics data
>> https://www.biorxiv.org/content/10.1101/2022.05.24.493193v1.full.pdf
Cello scope, a novel Bayesian probabilistic graphical model of gene expression in ST data, which deconvolutes cell type composition in ST spots, and a method to infer model parameters based on a MCMC algorithm.
Cello scope was developed to assign cell types, and as such it assumes that each observation refers to only one cell. Cello scope is fully independent of scRNA-seq data intrinsically mitigates risks encountered while integrating data from the two disparate platforms.

□ DeepHisCoM: deep learning pathway analysis using hierarchical structural component models
>> https://academic.oup.com/bib/advance-article-abstract/doi/10.1093/bib/bbac171/6590446
Deep-learning pathway analysis using Hierarchical structured CoMponent models (DeepHisCoM) utilizes DL methods to consider a nonlinear complex contribution of biological factors to pathways by constructing a multilayered model which accounts for hierarchical biological structure.
DeepHisCoM was shown to have a higher power in the nonlinear pathway effect and comparable power for the linear pathway effect when compared to the conventional pathway methods.

□ Simultant: simultaneous curve fitting of functions and differential equations using analytical gradient calculations
>> https://bmcbioinformatics.biomedcentral.com/articles/10.1186/s12859-022-04728-5
Simultant, a software package that allows complex fitting setups to be easily defined using a simple graphical user interface. Fitting functions can be defined directly as mathematical expressions or indirectly as the solution to specified ordinary differential equations.
Simultant accelerates fitting using analytical gradient calculations, thus enabling large-scale fits to be performed. Simultant furthermore utilizes automatic gradient calculations which permits fast fitting even with many parameters.

□ Exploiting Large Datasets Improves Accuracy Estimation for Multiple Sequence Alignment
>> https://www.biorxiv.org/content/10.1101/2022.05.22.493004v1.full.pdf
Facet-NN and Facet-LR; two new scoring-function-based accuracy estimators which reimagine the original Facet estimator by us- ing modern machine learning techniques for optimization, rather than combinatorial optimization, to exploit the much larger datasets.
An advisor contains two key components: a set of candidate parameter vectors, called an advisor set; and an accuracy estimation tool used to choose from among those vectors, called an advisor estimator.

□ scPrivacy: Privacy-preserving integration of multiple institutional data for single-cell type identification
>> https://www.biorxiv.org/content/10.1101/2022.05.23.493074v1.full.pdf
scPrivacy is an efficient automatically single-cell type identification prototype to facilitate single cell annotations, by integrating multiple references data distributed in different institutions using an federated learning based deep metric learning framework.
scPrivacy extends Deep Metric Learning to a federated learning framework by aggregating model parameters of institutions which fully utilized the information contained in multiple institutional datasets to train the aggregated model while avoiding integrating datasets physically.

□ scPreGAN: a deep generative model for predicting the response of single cell expression to perturbation
>> https://academic.oup.com/bioinformatics/advance-article-abstract/doi/10.1093/bioinformatics/btac357/6593485
In many cases, it is hard to collect the perturbed cells, such as knowing the response of a cell type to the drug before actual medication to a patient. Prediction in silicon could alleviate the problem and save cost.
ScPreGAN integrates autoencoder and generative adversarial network, the former is to extract common information of the unperturbed data and the perturbed data, the latter is to predict the perturbed data.

□ ChromGene: Gene-Based Modeling of Epigenomic Data
>> https://www.biorxiv.org/content/10.1101/2022.05.24.493345v1.full.pdf
ChromGene uses a mixture of hidden Markov models to model the combinatorial and spatial information of epigenomics maps. ChromGene can learn a common model across multiple cell types and use it to generate per-gene annotations for each.
ChromGene annotations are less likely to directly reflect information about gene length compared to baseline methods that incorporate information from the whole gene.

□ iSpatial: Accurate inference of genome-wide spatial expression
>> https://www.biorxiv.org/content/10.1101/2022.05.23.493144v1.full.pdf
iSpatial uses two-rounds integration to reduce potential technology bias and batch effect on PCA space, allowing accurate integration of ST and scRNA-seq datasets. iSpatial outperforms existing approaches on its accuracy and it can reduce false-positive and false-negative signals.
iSpatial uses weighted KNN when performing expression inference: the neighbors close to the inquired cell will be assigned higher weights than neighbors far from the cell in expression imputation.
This should reduce the over-smoothing effect for rare cell types when relatively large K is used, as the neighbors relatively far away from the rare cell types will have less impact on the inferred expression.
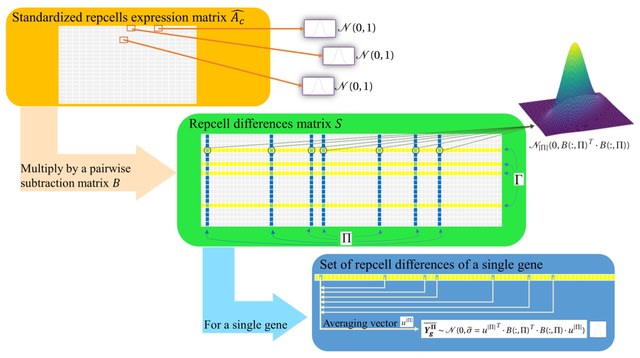
□ SPIRAL: Significant Process InfeRence ALgorithm for single cell RNA-sequencing and spatial transcriptomics
>> https://www.biorxiv.org/content/10.1101/2022.05.24.493189v1.full.pdf
SPIRAL is an algorithm that relies on a Gaussian statistical model to produce a comprehensive overview of significant processes in single cell RNA-seq, spatial transcriptomics or bulk RNA-seq.
SPIRAL detects structures combining selection on both gene and sample axes. SPIRAL provides a partitioning of the cells into layers based on the expression values. SPIRAL allows for the determination of statistically significant structures, distinguished from noise.

□ NeRFax: An efficient and scalable conversion from the internal representation to Cartesian space
>> https://www.biorxiv.org/content/10.1101/2022.05.25.493427v1.full.pdf
NeRFax, an efficient method for the conversion from internal to Cartesian coordinates that utilizes the platform-agnostic JAX Python library.
A single-CPU implementation of NeRFax algorithm consistently outperformed the state-of-the art NeRF code for every tested protein chain length in a range of 10 to 1,000 residues yielding 35 to 175 speedup.

□ ICAT: A Novel Algorithm to Robustly Identify Cell States Following Perturbations in Single Cell Transcriptomes
>> https://www.biorxiv.org/content/10.1101/2022.05.26.493603v1.full.pdf
Identify Cell states Across Treatments (ICAT) employes self-supervised feature weighting, followed by semi-supervised clustering, ICAT accurately identifies cell states across scRNA-seq perturbation experiments with high accuracy.
ICAT does not require prior knowledge of marker genes or extant cell states, is robust to perturbation severity, and identifies cell states with higher accuracy than leading integration workflows within both simulated and real scRNA-seq perturbation experiments.

□ Accelerating single-cell genomic analysis with GPUs
>> https://www.biorxiv.org/content/10.1101/2022.05.26.493607v1.full.pdf
RAPIDS K-Nearest Neighbors (KNN) graph construction, UMAP visualization, and Louvain clustering, had previously been integrated into the Scanpy framework.
RAPIDS can be used to load an scATAC-seq fragment file using the cuDF library and create sequencing coverage tracks for selected regions in each cluster, thus enabling interactive cluster-specific visualization alongside interactive clustering.

□ SCADIE: simultaneous estimation of cell type proportions and cell type-specific gene expressions using SCAD-based iterative estimating procedure
>> https://genomebiology.biomedcentral.com/articles/10.1186/s13059-022-02688-w
Unsupervised methods are useful in situations of cell type discovery or lack of supervising information, but as there is no guarantee that their inferred cell types have one-to-one mapping to actual cell types, annotating cell types remains a challenge.
SCADIE requires either bulk gene expression matrices and cell type proportions or bulk gene expression matrices and shared signature matrix as input; the cell type proportions can be obtained by any deconvolution method.

□ LoRTIS Software Suite: Transposon mutant analysis using long-read sequencing
>> https://www.biorxiv.org/content/10.1101/2022.05.26.493556v1.full.pdf
LoRTIS-SS uses the Snakemake framework to manage the workflow. The workflow uses long-read nucleotide sequence data such as those generated by the MinION sequencer.
The software workflow outputs data compatible with the established Bio-TraDIS analysis toolkit allowing for existing workflows to be easily upgraded to support long-read sequencing.

□ CNpare: matching DNA copy number profiles
>> https://academic.oup.com/bioinformatics/advance-article/doi/10.1093/bioinformatics/btac371/6596047
CNpare identifies similar cell line models based on genome-wide DNA copy number. CNpare compares copy number profiles using four different similarity metrics, quantifies the extent of genome differences between pairs, and facilitates comparison based on copy number signatures.
CNpare can also be applied to other settings including: quality control - ensuring the sequenced copy number profile of a cell line matches the reference profile; assessing differences between cell line cultures - by etimating the percentage genome difference.

□ PRRR: A Poisson reduced-rank regression model for association mapping in sequencing data
>> https://www.biorxiv.org/content/10.1101/2022.05.31.494236v1.full.pdf
Poisson RRR (PRRR) and nonnegative Poisson RRR (nn-PRRR) — to jointly model associations within two high-dimensional paired sets of features where the response variables are counts.
PRRR is able to detect associations between a high-dimensional response matrix and a high- dimensional set of predictors by leveraging low-dimensional representations of the data.
PRRR is able to properly account for the count-based nature of single-cell RNA sequencing data using a Poisson likelihood. PRRR uses a Poisson likelihood to model the transcript counts for each cell as the response variables, conditional on observed cell-specific covariates.

□ Matilda: Multi-task learning from single-cell multimodal omics
>> https://www.biorxiv.org/content/10.1101/2022.06.01.494441v1.full.pdf
Matilda, a neural network-based multi-task learning method for integrative analysis of single-cell multimodal omics data. Matilda simultaneously performs data simulation, dimension reduction, cell type classification, and feature selection using a gradient descent procedure.
Matilda learns to combine and reduce the feature dimensions of single-cell multimodal omics data to a latent space using its VAE component in the framework.
The potential mismatch of cell types in the query datasets may have a significant impact on the performance of Matilda. A solution may be to utilise the prediction probability of the neural network for deciding whether a cell in a query dataset should be classified or not.

□ Markonv: a novel convolutional layer with inter-positional correlations modeled
>> https://www.biorxiv.org/content/10.1101/2022.06.09.495500v1.full.pdf
Markonv layer (Markov convolutional neural layer), a novel convolutional neural layer with Markov transition matrices as its filters, to model the intrinsic dependence in inputs as Markov processes.
Markonv-based networks could not only identify functional motifs with inter-positional correlations in large-scale omics sequence data effectively, but also decode complex electrical signals generated by Oxford Nanopore sequencing efficiently.

□ SNIKT: sequence-independent adapter identification and removal in long-read shotgun sequencing data
>> https://academic.oup.com/bioinformatics/advance-article-abstract/doi/10.1093/bioinformatics/btac389/6607583
Snikt (Slice Nucleotides Into Klassifiable Tequences) is a program that reports a visual confirmation of adapter or systemic contamination in whole-genome shotgun (WGS) or metagenomic sequencing DNA or RNA reads and based on user input, trims sequence ends to remove them.
Snikt works w/o prior information about the adapter sequence making it applilcable even when this information is unavailable. Its most suitable for long read. Because read end trimming for long reads does not have a significant impact on the overall read throughput post-cleaning.

□ DIVE: a reference-free statistical approach to diversity-generating and mobile genetic element discovery
>> https://www.biorxiv.org/content/10.1101/2022.06.13.495703v1.full.pdf
DIVE, a novel statistical, reference-free paradigm for de novo discovery of MGEs and DGMs by identifying k-mer sequences associated with high rates of sequence diversification.
DIVE generates a target dictionary with an online clustering method that collapses targets within “sequencing error" distance. It then models the number of clusters formed at each step using a Poisson-Binomial model.
※コメント投稿者のブログIDはブログ作成者のみに通知されます