単一システムの無謬性は、その外層と作用する境界において、無謬であるがゆえに破滅的な瑕疵を引き起こす。
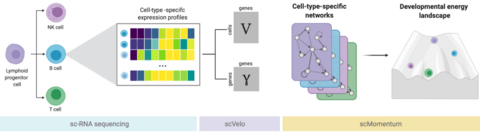
□ scMomentum: Inference of Cell-Type-Specific Regulatory Networks and Energy Landscapes
>> https://www.biorxiv.org/content/10.1101/2020.12.30.424887v1.full.pdf
scMomentum, a model-based data-driven formulation to predict gene regulatory networks and energy landscapes from single-cell transcriptomic data without requiring temporal or perturbation experiments.
scMomentum constructs two independent branching trajectories and uses scVelo. The inferred GRNs were used to derive the associated energy. The network distance matrix recovered trajectories on a Multidimensional Scaling projection (MDS) that resemble cell progressions.

□ SIGMA: Recovery of high-quality assembled genomes via single-cell genome-guided binning of metagenome assembly
>> https://www.biorxiv.org/content/10.1101/2021.01.11.425816v1.full.pdf
SIGMA (a single-cell genome-guided binning of metagenomic assemblies) can integrate SAG and MAG to reconstruct qualified microbial genomes and control their binning resolution based on the numbers and classification of SAGs.
SIGMA generates self-reference sequences from the same sample by single-cell sequencing and uses them as guides to reconstruct metagenomic bins.

□ scJoint: transfer learning for data integration of single-cell RNA-seq and ATAC-seq
>> https://www.biorxiv.org/content/10.1101/2020.12.31.424916v1.full.pdf
scJoint uses a neural network to simulta- neously train labelled and unlabelled data and embed cells from both modalities in a common lower dimensional space, enabling label transfer and joint visualisation in an integrative framework.
scJoint consistently provides meaningful joint visualisations and achieves significantly higher label transfer accuracy than existing methods using a complex cell atlas data and a biologically varying multi-modal data.

□ scMC learns biological variation through the alignment of multiple single-cell genomics datasets
>> https://genomebiology.biomedcentral.com/articles/10.1186/s13059-020-02238-2
scMC is particularly effective to address the over-alignment issue. Application of scMC to both simulated and real datasets from single-cell RNA-seq and ATAC-seq experiments demonstrates its capability of detecting context-specific biological signals via accurate alignment.
scMC algorithm integrates following steps: data preprocessing; feature matrix construction; inference of shared cell clusters b/n any pair of datasets; learning the confounding matrix; learning the correction vectors; and construction of corrected data for downstream analysis.

□ Inference of emergent spatio-temporal processes from single-cell sequencing reveals feedback between de novo DNA methylation and chromatin condensates
>> https://www.biorxiv.org/content/10.1101/2020.12.30.424823v1.full.pdf
how collective processes in physical space can be inferred from single-cell methylome sequencing measurements along the one-dimensional DNA sequence.
Combining single cell methylome data with a theoretical approach that transfers methods from quantum theory and statistical phyiscs (field theory, renormalization group theory) to genomics. The inference of the interaction kernel fully determined the dynamics in sequence space.
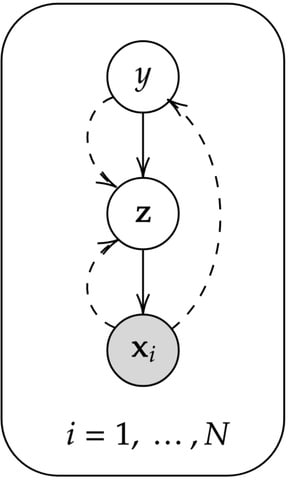
□ HaploNet: Haplotype and Population Structure Inference using Neural Networks in Whole-Genome Sequencing Data
>> https://www.biorxiv.org/content/10.1101/2020.12.28.424587v1.full.pdf
HaploNet utilizes a variational autoencoder (VAE) framework to learn mappings to and from a low-dimensional latent space in which we will perform indirect clustering of haplotypes with a Gaussian mixture prior.
z is a D-dimensional vector representing the latent haplotype encoding and C is the number of haplotype clusters. Ber(x; πθ(z)) is a vectorized notation of Bernoulli distributions and each of the L sites will have an independent probability mass function.
the covariance matrix of the multivariate Gaussian distribution is a diagonal matrix which will promote disentangled factors. the marginal posterior distribution and marginal approximate posterior distribution of z will both be a mixture of Gaussians.

□ G-Tric: generating three-way synthetic datasets with triclustering solutions
>> https://bmcbioinformatics.biomedcentral.com/articles/10.1186/s12859-020-03925-4
G-Tric can replicate real-world datasets and create new ones that match researchers needs across several properties, including data type (numeric or symbolic), dimensions, and background distribution.
triclustering, a new subspace clustering, proposed to enable the search for patterns that correlate subsets of observations, shows similarities on a specific subset of features, and whose values are repeated or evolve coherently across a third dimension, generally time or space.

□ scReQTL: an approach to correlate SNVs to gene expression from individual scRNA-seq datasets
>> https://bmcgenomics.biomedcentral.com/articles/10.1186/s12864-020-07334-y
scReQTL analysis includes approximately 4 billion scRNA-seq reads. ScReQTL analysis was performed after classification of the cells by cell type, and only SNVs covered by a minimum of 10 unique sequencing reads per cell were included in the analysis.
scReQTL can be applied on genomic positions of interest from external sources, for example sets of somatic mutations from the COSMIC database, or known RNA-edited loci from the REDI portal; the selected sets of loci can be used as input for STAR-WASP alignment for.
□ Evaluating collapsed misassembly with asmgene
>> http://lh3.github.io/2020/12/25/evaluating-assembly-quality-with-asmgene
Percent MMC is a new metric to measure the quality of an assembly. It takes minutes to compute, is gene focused and is robust to structural variations in comparison to evaluations based on assembly-to-reference alignment.
MMC = 1 - |{MCinASM} ∩ {MCinREF}| / |{MCinREF}|
In the ideal case of a perfect assembly, %MMC should be zero. A higher fraction suggests more collapsed assemblies.
□ Hifiasm_meta: de novo metagenome assembler, based on hifiasm, a haplotype-resolved de novo assembler for PacBio Hifi reads
>> https://github.com/xfengnefx/hifiasm-meta
Hifiasm_meta handles chimeric read detection and contained reads etc more carefully in the metagenome assembly context, which, in some cases, could benefit the less represented species in the sample.
Hifiasm_meta comes with a read selection module, which enables the assembly of dataset of high redundancy without compromising overall assembly quality, and meta-centric graph cleaning modules.

□ scFusion: Single cell gene fusion detection
>> https://www.biorxiv.org/content/10.1101/2020.12.27.424506v1.full.pdf
scFusion is computationally more efficient, has far less false discoveries while achieves similar detection power compared to fusion detection tools developed for bulk data.
scFusion models the background noise as zero inflated negative binomial and uses a statistical testing to control for false positives. The deep learning model is trained to recognize technical chimeric artefacts and filter false fusion candidates generated by these artefacts.

□ LongGF: computational algorithm and software tool for fast and accurate detection of gene fusions by long-read transcriptome sequencing
>> https://bmcgenomics.biomedcentral.com/articles/10.1186/s12864-020-07207-4
LongGF has several steps to detect gene fusions from the BAM file: get multiple mapped long reads, obtain candidate gene pairs, find gene pairs with non-random supporting long reads, and output prioritized list of candidate gene fusions ranked by the number of supporting reads.
LongGF is implemented in C++ and is very fast to run, and it only takes several minutes and 3GB memory on 50,000 long reads from a transcriptome for gene fusion detection.
□ Convex hulls in hamming space enable efficient search for similarity and clustering of genomic sequences
>> https://bmcbioinformatics.biomedcentral.com/articles/10.1186/s12859-020-03811-z
The convex hull in hamming space is a data structure that provides information on average hamming distance within the set, average hamming distance between two sets; closeness centrality of each sequence; and lower/upper bound of all the pairwise distances.
The convex hull distance algorithm is a fast and efficient strategy for massively reducing the computational burden of pairwise comparison among large samples of sequences. And aiding the calculation of transmission links among infected individuals using threshold-based methods.

□ Automated Isoform Diversity Detector (AIDD): a pipeline for investigating transcriptome diversity of RNA-seq data
>> https://bmcbioinformatics.biomedcentral.com/articles/10.1186/s12859-020-03888-6
Automated Isoform Diversity Detector, AIDD contains open source tools for various tasks needed to map transcriptome diversity, including RNA editing events.
AIDD is designed to run automatically with limited user input through a customizable bash script that controls multiple computational tools, including HISAT2 and GATK, among others, to comprehensively analyse RNA-seq datasets.

□ Tailored Graphical Lasso for Data Integration in Gene Network Reconstruction
>> https://www.biorxiv.org/content/10.1101/2020.12.29.424744v1.full.pdf
the graphical lasso and weighted graphical lasso can be considered special cases of the tailored graphical lasso, and a parameter determined by the data measures the usefulness of the prior information.
the tailored graphical lasso utilizes useful prior information more e↵ectively without involving any risk of loss of accuracy should the prior information be misleading.
□ Chromatin Interaction Neural Network (ChINN): A machine learning-based method for predicting chromatin interactions from DNA sequences
>> https://www.biorxiv.org/content/10.1101/2020.12.30.424817v1.full.pdf
Chromatin Interaction Neural Network (ChINN) predicts open chromatin interactions from DNA sequences. This model has been developed for RNA Polymerase II ChIA-PET interactions, CTCF ChIA-PET interactions and Hi-C interactions.
ChINN was able to identify convergent CTCF motifs, AP-1 transcription family member motifs such as FOS, and other transcription factors such as MYC as being important in predicting chromatin interactions.

□ eSPRESSO: a spatial self-organizing-map clustering method for single-cell transcriptomes of various tissue structures using graph-based networks
>> https://www.biorxiv.org/content/10.1101/2020.12.31.424948v1.full.pdf
eSPRESSO uses stochastic self-organizing map clustering, together with optimization of gene set by Markov chain Monte Carlo framework, to estimate the spatial domain structure of cells in any topology of tissues or organs from only their transcriptome profiles.
eSPRESSO, a graph-based SOM clustering to reconstruct any topology of domains or tissues as long as they can be drawn as some kind of connection graphs or network diagrams.

□ BERT-GT: Cross-sentence n-ary relation extraction with BERT and graph transformer
>> https://academic.oup.com/bioinformatics/advance-article/doi/10.1093/bioinformatics/btaa1087/6069538
Bidirectional Encoder Representations from Transformers with Graph Transformer (BERT-GT), through integrating a neighbor-attention mechanism into the BERT architecture.
In the self-attention mechanism, the whole sentence(s) to calculate the attention of the current token, the neighbor-attention mechanism in BERT-GT calculates its attention utilizing only its neighbor tokens.

□ SANS serif: alignment-free, whole-genome based phylogenetic reconstruction
>> https://www.biorxiv.org/content/10.1101/2020.12.31.424643v1.full.pdf
SANS serif accepts a list of multiple FASTA or FASTQ files containing complete genomes, assembled contigs, or raw reads as input. In addition, the program offers the option to import a colored de Bruijn graph generated with the software Bifrost.
SANS serif is capable of handling ambiguous IUPAC characters such as N’s, replacing these with the corresponding DNA bases, considering all possibilities.

□ A novel chromosome cluster types identification method using ResNeXt WSL model
>> https://www.sciencedirect.com/science/article/abs/pii/S1361841520303078
The proposed framework is based on ResNeXt weakly-supervised learning (WSL) pre-trained backbone and a task-specific network header.
The proposed framework is based on ResNeXt weakly-supervised learning (WSL) pre-trained backbone and a task-specific network header. A non-end-to-end paradigm to utilize existing chromosome cluster segmentation works.
□ Algorithm optimization for weighted gene co-expression network analysis: accelerating the calculation of Topology Overlap Matrices with OpenMP and SQLite
>> https://www.biorxiv.org/content/10.1101/2021.01.01.425026v1.full.pdf
If single-threaded algorithms can be changed to multi-threaded algorithms, it will be extremely improve the calculation speed.
the single-threaded algorithm of sequence comparison has been changed to the multi-threaded algorithm, and the algorithm of protein sequence search has been changed to the multi-threaded algorithm.


□ Accurate, scalable cohort variant calls using DeepVariant and GLnexus
>> https://academic.oup.com/bioinformatics/advance-article/doi/10.1093/bioinformatics/btaa1081/6064144
Adapting the scalable joint genotyper GLnexus to DeepVariant gVCFs and tune filtering and genotyping parameters to optimize performance for whole-genome sequences and whole-exome sequences across a range of sequence coverages and cohort sizes.
DeepVariant+GLnexus joint genotyping algorithms may be able to more accurately refine individual genotypes by DeepVariant since joint genotyping refine individuals’ variant calls based on observed allele frequencies in the rest of the cohort, using GQ as a prior on genotype.

□ ATAC-DoubletDetector: A read count-based method to detect multiplets and their cellular origins from snATAC-seq data
>> https://www.biorxiv.org/content/10.1101/2021.01.04.425250v1.full.pdf
ATAC-DoubletDetector includes a novel clustering-based algorithm that accurately annotates the cellular origins of detected multiplets providing further data quality insights.
ATAC-DoubletDetector exploits read count distributions for a given nucleus to effectively detect and eliminate multiplets without requiring prior knowledge of cell-type information.

□ SCC: an accurate imputation method for scRNA-seq dropouts based on a mixture model
>> https://bmcbioinformatics.biomedcentral.com/articles/10.1186/s12859-020-03878-8
SCC gives competitive results compared to two existing methods while showing superiority in reducing the intra-class distance of cells and improving the clustering accuracy in both simulation and real data.
SCC replaces clustering similar cells with finding the nearest neighbor cells of each cell. In this way SCC can not only obtain the complete gene expression data but also preserve cell-to-cell heterogeneity.
SCC does not need the cell type as prior information and the scRNA-seq matrix is the only input. The output of SCC is a modified scRNA-seq matrix. Besides, SCC is memory-efficient because it only modifies one cell at a time.

□ MATHLA: a robust framework for HLA-peptide binding prediction integrating bidirectional LSTM and multiple head attention mechanism
>> https://bmcbioinformatics.biomedcentral.com/articles/10.1186/s12859-020-03946-z
MATHLA integrates bidirectional LSTM and multiple head attention mechanism has addressed these two questions by not only achieving prominent advantage in prediction accuracy for the HLA-C alleles but also attaining better prediction power for longer class I HLA ligands.
MATHLA allows input sequences with flexible lengths. The encoded matrix with dimension lseq*20, where lseq is the length of concatenated sequence of peptide and HLA pseudo-sequence, is then input into sequence learning layer.
□ KDS-Filt: Filtering Spatial Point Patterns Using Kernel Densities
>> https://linkinghub.elsevier.com/retrieve/pii/S2211675320300816
Kernel Density and Simulation based Filtering (KDS-Filt), showed superior performance to existing alternative approaches, especially when there is inhomogeneity in cluster sizes and density.
KDS-Filt estimates Estimate a data-driven kernel covariance matrix Σ for a bivariate normal smoothing density and evaluate the leave-one-out gˆi at the observed points xi ∈ X.

□ Partition Quantitative Assessment (PQA): A quantitative methodology to assess the embedded noise in clustered omics and systems biology data
>> https://www.biorxiv.org/content/10.1101/2021.01.08.425967v1.full.pdf
Many of the literature focuses on how well the clustering algorithm orders the data, with several measures regarding external and internal statistical measures; but none measure has been developed to statistically quantify the noise in an arranged vector posterior a clustering algorithm.
a relative score derived from an SC of the VP from the dendrogram of any clustering analysis and calculated Z- statistics as well as an extrapolation to deliver an estimation of noise in the Vector of Profiles.
□ xGAP: A python based efficient, modular, extensible and fault tolerant genomic analysis pipeline for variant discovery
>> https://academic.oup.com/bioinformatics/advance-article-abstract/doi/10.1093/bioinformatics/btaa1097/6069565
xGAP (extensible Genome Analysis Pipeline) implements massive parallelization of the GATK best practices pipeline by splitting a genome into many smaller regions with efficient load-balancing to achieve high scalability.
xGAP can process 30x coverage whole-genome sequencing (WGS) data in approximately 90 minutes. Compared to the Churchill pipeline, with similar parallelization, xGAP is 20% faster when analyzing 50X coverage WGS in AWS.

□ Manipulating base quality scores enables variant calling from bisulfite sequencing alignments using conventional Bayesian approaches
>> https://www.biorxiv.org/content/10.1101/2021.01.11.425926v1.full.pdf
SNP data is desirable both for genotyping and for resolving the interaction between genetic and epigenetic effects when elucidating the DNA methylome. The confounding effect of bisulfite conversion can be resolved by observing differences in allele counts on a per-strand basis.
a computational pre-processing approach for adapting such data, thus enabling downstream analysis in this way using conventional variant calling software such as GATK or Freebayes.
The method involves a simple double-masking procedure which manipulates specific nucleotides and base quality (BQ) scores on alignments from bisulfite sequencing data, prior to variant calling.
□ Red Panda: a novel method for detecting variants in single-cell RNA sequencing
>> https://bmcgenomics.biomedcentral.com/articles/10.1186/s12864-020-07224-3
Red Panda takes two files as input: a tab-delimited file generated by sailfish containing a list of all isoforms and their expression levels in a VCF generated by samtools mpileup containing a pileup of all locations in the cell’s genome that differ from the reference.
Red Panda gains an advantage against other tools by intentionally separating variants into three separate classes and processing them differently: homozygous-looking, bimodally-distributed heterozygous, and non-bimodally-distributed heterozygous.
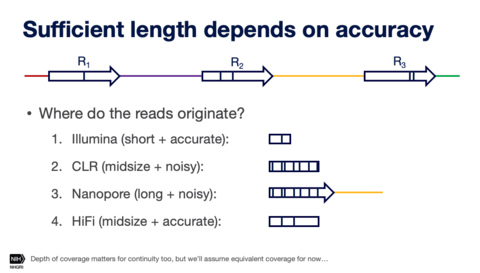
□ De novo assembly and haplotype phasing of diploid human genomes using long High-fidelity reads and non-trio phasing approaches
>> https://medium.com/dnanexus/towards-a-truly-personalized-genome-part-2-848d00116c8e
The higher accuracy of HiFi data enables the application of various algorithmic decision procedures used in new assemblers like Peregrine, Hifiasm, HiCanu, and IPA, which is not feasible with high error reads.
The phasing is performed on the de novo assembled genome rather than a typical reference-guided assembly.
The phasing algorithm is independent of the assembly algorithm. The lets the user choose which algorithms they want to use. They could even pick different algorithms for unphased and phased assemblies.
□ Minigraph as a multi-assembly SV caller
>> http://lh3.github.io/2021/01/11/minigraph-as-a-multi-assembly-sv-caller
The solution to these problems is multi-sequence alignment (MSA) which minigraph approximates. MSA naturally alleviates imprecise breakpoints because MSA effectively groups similar events first;
MSA also fully represents nested events because unlike mapping against a reference genome, MSA aligns inserted sequences not in the reference.
Minigraph is a fast and powerful multi-assembly SV caller. Although the calling is graph based, you can ignore the graph structure and focus on SVs only.

□ Bichrom: An interpretable bimodal neural network characterizes the sequence and preexisting chromatin predictors of induced transcription factor binding
>> https://genomebiology.biomedcentral.com/articles/10.1186/s13059-020-02218-6
Bichrom’s architecture embeds TF binding sites into a two-dimensional latent space, which can be used to estimate the relative contributions of DNA sequence and preexisting chromatin features at individual TF binding sites.
Bichrom’s bimodal neural network architecture hyper-parameters were chosen via a random grid-search. Each sub-network consists of a CNN layer that acts as a primary feature extractor, followed by a LSTM layer that can capture potential interactions between convolutional filters.
□ srnaMapper: an optimal mapping tool for sRNA-Seq reads
>> https://www.biorxiv.org/content/10.1101/2021.01.12.426326v1.full.pdf
srnaMapper mapps all the reads that the other map, and maps several reads that other do not. It also can map read with fewer errors, and find more loci per read.
srnaMapper manipulates the structure like a tree, and refer to this structure as the genome tree, even though it is, stricto sensu, an array. srnaMapper stores the reads into a radix tree, where each path from the root to a terminal node stores a sequence.
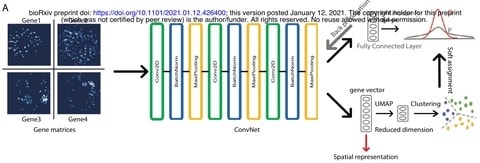
□ CoSTA: Unsupervised Convolutional Neural Network Learning for Spatial Transcriptomics Analysis
>> https://www.biorxiv.org/content/10.1101/2021.01.12.426400v1.full.pdf
CoSTA is inspired by computer vision and image classification to find relationships between spatial expression patterns of different genes while preserving the full spatial context.
CoSTA can optimize the model by minimizing bi-tempered logistic loss based on Bregman Divergences between the generated soft assignments and the probabilities from the fully connected layer.

□ HiDeF: identifying persistent structures in multiscale ‘omics data
>> https://genomebiology.biomedcentral.com/articles/10.1186/s13059-020-02228-4
HiDeF, the Hierarchical community Decoding Framework is an analysis framework to robustly resolve the hierarchical structures of networks based on multiscale community detection and the concepts of persistent homology.
HiDeF recognizes when a community is contained by multiple parent communities, which in the context of protein-protein networks suggests that the community participates in diverse pleiotropic biological functions.
※コメント投稿者のブログIDはブログ作成者のみに通知されます