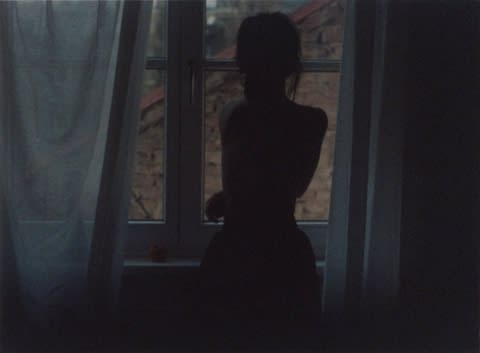
(Photo by Sina Kauri)

□ SAINT: automatic taxonomy embedding and categorization by Siamese triplet network
>> https://www.biorxiv.org/content/10.1101/2021.01.20.426920v1.full.pdf
SAINT is a weakly-supervised learning method where the embedding function is learned automatically from the easily-acquired data; SAINT utilizes the non-linear deep learning-based model which potentially better captures the complicated relationship among genome sequences.
SAINT encodes the phylogeny into a sequence triplets, each of which is represented as a k-mer frequency vector. Each layers are passed through a Siamese triplet network. The last layer learns a mapping directly from the hidden space to the embedding space of dimensionality d.
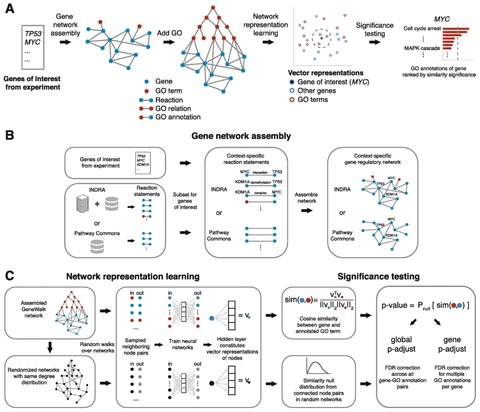
□ Polar sets: Sequence-specific minimizers
>> https://www.biorxiv.org/content/10.1101/2021.02.01.429246v1.full.pdf
Polar set is a new way to create sequence-specific minimizers that overcomes several shortcomings in previous approaches to optimize a minimizer sketch specifically for a given reference sequence.
Link energy measures how well spread out a polar set is. A context c is called an energy saver if E(c) less than 2/(w + 1), and its energy deficit is defined as 2/(w + 1) − E(c). The energy deficit of S, denoted D(S), is the total energy deficit across all energy savers: D(S) = Σc max(0, 2/(w + 1) − E(c)).
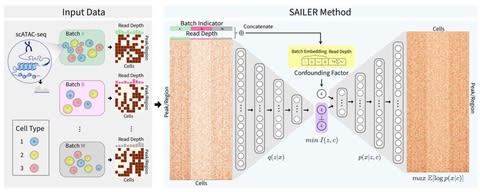
□ SAILER: Scalable and Accurate Invariant Representation Learning for Single-Cell ATAC-Seq Processing and Integration
>> https://www.biorxiv.org/content/10.1101/2021.01.28.428689v1.full.pdf
SAILER aims to learn a low-dimensional nonlinear latent representation of each cell that defines its intrinsic chromatin state, invariant to extrinsic confounding factors like read depth and batch effects.
SAILER adopts the conventional encoder-decoder framework ana imposes additional constraints to ensure the independence of the learned representations from the confounding factors. because no matrix factorization is involved, SAILER can easily scale to process millions of cells.
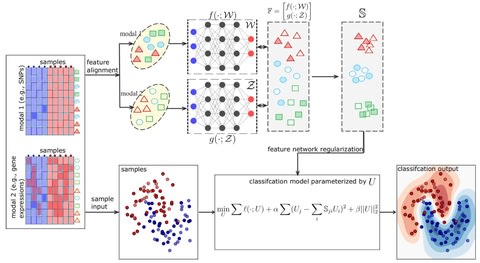
□ deepManReg: a deep manifold-regularized learning model for improving phenotype prediction from multi-modal data
>> https://www.biorxiv.org/content/10.1101/2021.01.28.428715v1.full.pdf
deepManReg conducts a deep manifold alignment between all features so that the features are aligned onto a common latent manifold space. The distances of various features b/n modalities on the space represent their nonlinear relationships identified by cross-modal manifolds.
deepManReg uses a novel optimization algorithm by backpropagating the Riemannian gradients on a Stiefel manifold. deepManReg solves the tradeoff between nonlinear and parametric manifold alignment.
Deepalignomic requires a non-trivial hyperparameter optimization includes a large combination of parameters. Another potential issue for aligning such large datasets in deepManReg which may be computational intensive is the large joint Laplacian matrix.
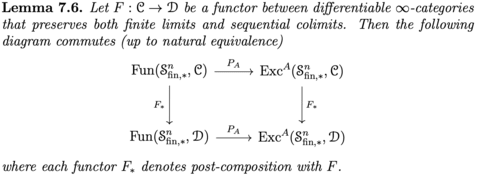
□ TANGENT ∞-CATEGORIES AND GOODWILLIE CALCULUS:
>> https://arxiv.org/pdf/2101.07819v1.pdf
A tangent structure on an infinity-category X consists of an endofunctor on X, which plays the role of the tangent bundle construction, together with various natural transformations that mimic structure possessed by the ordinary tangent bundles of smooth manifolds.
The characterization of differential objects as stable ∞-categories confirms the intuition, promoted by Goodwillie, that in the analogy between functor calculus and the ordinary calculus of manifolds one should view the category of spectra as playing the role of Euclidean space.
Lurie's construction admits the additional structure maps and satisfies the conditions needed to form a tangent infinity-category, which refers to as the Goodwillie tangent structure on the infinity-category of infinity-categories.
□ Hausdorff dimension and infinitesimal similitudes on complete metric spaces
>> https://arxiv.org/pdf/2101.07520v1.pdf
the Hausdorff dimension and box dimension of the attractor generated by a finite set of contractive infinitesimal similitudes are the same.
The concept of infinitesimal similitude introduced in generalizes not only the similitudes on general metric spaces but also the concept of conformal maps from Euclidean domain to general metric spaces.
the continuity of Hausdorff dimension of the attractor of generalized graph-directed constructions under certain conditions. Estimating the lower bound for Hausdorff dimension of a set of complex continued fractions.
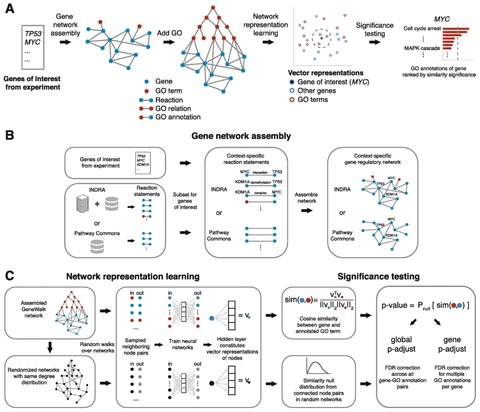
□ GeneWalk identifies relevant gene functions for a biological context using network representation learning
>> https://genomebiology.biomedcentral.com/articles/10.1186/s13059-021-02264-8
GeneWalk first automatically assembles a biological network from a knowledge base INDRA and the GO ontology starting with a list of genes of interest (e.g., differentially expressed genes or hits from a genetic screen) as input.
GeneWalk quantifies the similarity between vector representations of a gene and GO terms through representation learning with random walks on a condition-specific gene regulatory network. Similarity significance is determined with node similarities from randomized networks.
□ MultiNanopolish: Refined grouping method for reducing redundant calculations in nanopolish
>> https://academic.oup.com/bioinformatics/advance-article-abstract/doi/10.1093/bioinformatics/btab078/6126805
Multithreading Nanopolish (MultiNanopolish), which decomposes the whole process of iterative calculation in Nanopolish into small independent calculation tasks, making it possible to run this process in the parallel mode.
MultiNanopolish use a different iterative calculation strategy to reduce redundant calculations. MultiNanopolish reduces running time by 50% with read-uncorrected assembler (Miniasm) and 20% with read-corrected assembler (Canu and Flye) based on 40 threads mode.
□ s-aligner: a greedy algorithm for non-greedy de novo genome assembly
>> https://www.biorxiv.org/content/10.1101/2021.02.02.429443v1.full.pdf
Greedy algorithm assemblers are assemblers that find local optima in alignments of smaller reads.
s-aligner differs the most from a typical overlap-layout-consensus algorithm. Instead of looking for a Hamiltonian path in a graph connecting overlapped reads.
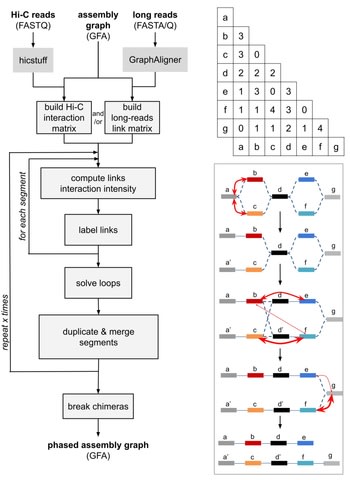
□ GraphUnzip: unzipping assembly graphs with long reads and Hi-C
>> https://www.biorxiv.org/content/10.1101/2021.01.29.428779v1.full.pdf
GraphUnzip implements a radically new approach to phasing that starts from an assembly graph instead of a collapsed linear sequence.
As GraphUnzip only connects sequences in the assembly graph that already had a potential link based on overlaps, it yields high-quality gap-less supercontigs.

□ DECODE: A Deep-learning Framework for Condensing Enhancers and Refining Boundaries with Large-scale Functional Assays
>> https://www.biorxiv.org/content/10.1101/2021.01.27.428477v2.full.pdf
DECODE uses Object boundary detection via weakly supervised learning framework (Grad-CAM), it extracts the implicit localization of the target from classification models and obtains a high-resolution subset of the image with the most informative content regarding the target.
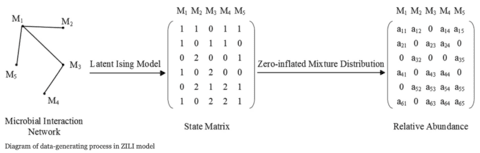
□ ZILI: Zero-Inflated Latent Ising model
>> https://biodatamining.biomedcentral.com/articles/10.1186/s13040-020-00226-7#Sec3
Conventional latent models, e.g state space model, typically assume the observed variables can be represented by a small number of latent variables and in this way the model dimensionality can be reduced.
ZILI, the zero-inflated latent Ising model is proposed which assumes the distribution of relative abundance relies only on finite latent states and provides a novel way to solve issues induced by the unit-sum and zero-inflation constrains.
□ gfabase: Graphical Fragment Assembly insert into GenomicSQLite
>> https://github.com/mlin/gfabase
gfabase is a command-line tool for indexed storage of Graphical Fragment Assembly (GFA1) data. It imports a .gfa file into a compressed .gfab file, from which it can later access subgraphs quickly (reading only the necessary parts), producing .gfa or .gfab.
.gfab is a new GFA-superset format with built-in compression and indexing. It is in fact a SQLite (+ Genomics Extension) database populated with a GFA1-like schema, which programmers have the option to access directly, without requiring gfabase nor even a low-level parser for .gfa/.gfab.
□ MBG: Minimizer-based Sparse de Bruijn Graph Construction
>> https://academic.oup.com/bioinformatics/advance-article/doi/10.1093/bioinformatics/btab004/6104877
MBG, Minimizer based sparse de Bruijn Graph constructor, a tool for building sparse de Bruijn graphs from HiFi reads. MBG outperforms existing tools for building dense de Bruijn graphs, and can build a graph of 50x coverage whole human genome HiFi reads in four hours on a single core.
MBG can construct graphs with arbitrarily high k-mer sizes, and k-mer sizes of thousands of base pairs are practical with real HiFi read data. the sparsity parameter w determines the sparseness of the resulting graph, with higher w leading to sparser graphs.

□ Strobemers: an alternative to k-mers for sequence comparison
>> https://www.biorxiv.org/content/10.1101/2021.01.28.428549v1.full.pdf
Under a certain minimizer sele tion technique, strobemers provide more evenly distributed se- quence matches than k-mers and are less sensitive to different mutation rates and distributions.
strobemers is inspired by strobe sequencing technology (an early Pacific Bio- sciences sequencing protocol), which would produce multiple subreads from a single contiguous fragment of DNA where the subreads are separated by ‘dark’ nucleotides whose identity is unknown.
□ tidybulk: an R tidy framework for modular transcriptomic data analysis
>> https://genomebiology.biomedcentral.com/articles/10.1186/s13059-020-02233-7
Tidybulk covers a wide variety of analysis procedures and integrates a large ecosystem of publicly available analysis algorithms under a common framework.
Tidybulk decreases coding burden, facilitates reproducibility, increases efficiency for expert users, lowers the learning curve for inexperienced users, and bridges transcriptional data analysis with the tidyverse.
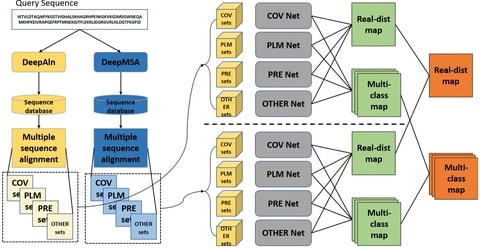
□ DeepDist: real-value inter-residue distance prediction with deep residual convolutional network
>> https://bmcbioinformatics.biomedcentral.com/articles/10.1186/s12859-021-03960-9
DeepDist, a multi-task deep learning distance predictor based on new residual convolutional network architectures to simultaneously predict real-value inter-residue distances and classify them into multiple distance intervals.
DeepDist can work well on some targets with shallow multiple sequence alignments. The MSE of DeepDist’s real-value distance prediction is 0.896 Å2 when filtering out the predicted distance ≥ 16 Å, which is lower than 1.003 Å2 of DeepDist’s multi-class distance prediction.
□ Overcoming the impacts of two-step batch effect correction on gene expression estimation and inference
>> https://www.biorxiv.org/content/10.1101/2021.01.24.428009v1.full.pdf
a basic theoretical explanation of the impacts of a na ̈ıve two-step batch correction strategy on downstream gene expression inference, and provide a heuristic demonstration and illustration of more complex scenarios using both simulated and real-data examples.
The ComBat approach, combined with an appropriate variance estimation approach that is built on the group-batch design matrix, proves to be effective in addressing the exaggerated and/or diminished significance problem in ComBat-adjusted data.

□ FILER: large-scale, harmonized FunctIonaL gEnomics Repository
>> https://www.biorxiv.org/content/10.1101/2021.01.22.427681v1.full.pdf
FunctIonaL gEnomics Repository (FILER), a large-scale, curated, integrated catalog of harmonized functional genomic and annotation data coupled with a scalable genomic search and querying interface to these data.
FILER provides a unified access to this rich functional and annotation data resource spanning >17 Billion records across genome with >2,700x total genomic coverage for both GRCh37/hg19 and GRCh38/hg38.

□ LanceOtron: a deep learning peak caller for ATAC-seq, ChIP-seq, and DNase-seq
>> https://www.biorxiv.org/content/10.1101/2021.01.25.428108v1.full.pdf
LanceOtron considers the patterns of the aligned sequence reads, and their enrichment levels, and returns a probability that a region is a true peak with signal arising from a biological event.
The core of LanceOtron’s peak scoring algorithm is a customized wide and deep model. First, local enrichment measurements are taken from the maximum number of overlapping reads. a multilayer perceptron combines the outputs from CNN and logistic regression model.
□ hybrid-LPA: Hybrid Clustering of Long and Short-read for Improved Metagenome Assembly
>> https://www.biorxiv.org/content/10.1101/2021.01.25.428115v1.full.pdf
hybrid-LPA, a new two-step Label Propagation Algorithm (LPA) that first forms clusters of long reads and then recruits short reads to solve the under-clustering problem with metagenomic short reads.
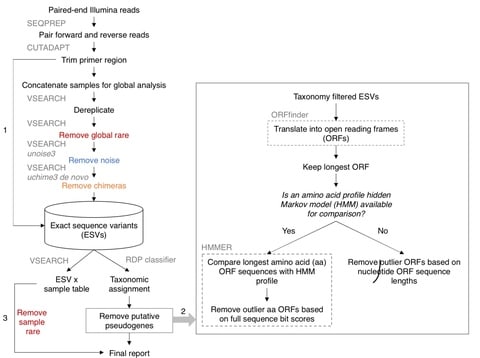
□ Profile hidden Markov model sequence analysis can help remove putative pseudogenes from DNA barcoding and metabarcoding datasets
>> https://www.biorxiv.org/content/10.1101/2021.01.24.427982v1.full.pdf
The combination of open reading frame length and hidden Markov model profile analysis can be used to effectively screen out obvious pseudogenes from large datasets.
This pseudogene removal methods cannot remove all pseudogenes, but remaining pseudogenes could still be useful for making higher level taxonomic assignments, though they may inflate richness at the species or haplotype level.
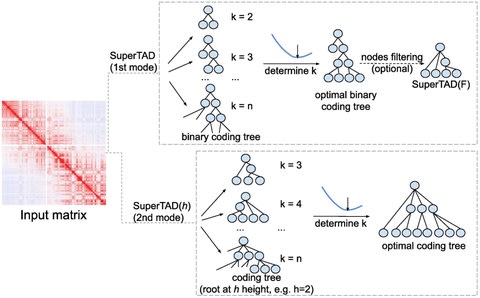
□ SuperTAD: robust detection of hierarchical topologically associated domains with optimized structural information
>> https://genomebiology.biomedcentral.com/articles/10.1186/s13059-020-02234-6
the problem is to find a partition with minimal structural information (entropy). They proposed a method which, through a top-down greedy recursion of partitioning and clustering, produces a hierarchical structure of TADs with the minimal structural entropy.
SuperTAD, an optimal algorithm using dynamic programming with polynomial time for computing the coding tree of a Hi-C contact map with minimal structural information.
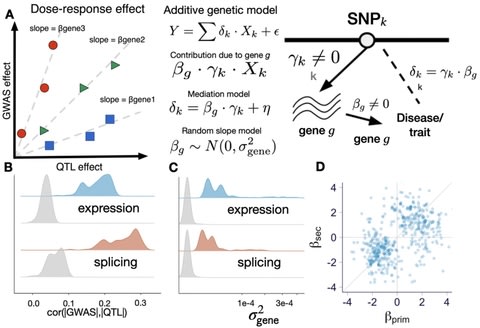
□ Exploiting the GTEx resources to decipher the mechanisms at GWAS loci
>> https://genomebiology.biomedcentral.com/articles/10.1186/s13059-020-02252-4
a systematic empirical demonstration of the widespread dose-dependent effect of expression and splicing on complex traits, i.e., variants with larger impact at the molecular level have larger impact at the trait level.
a database of optimal gene expression imputation models that were built on the fine-mapping probabilities for feature selection and that leverage the global patterns of tissue sharing of regulation to improve the weights.
Target genes in GWAS loci identified by enloc and PrediXcan were predictive of OMIM genes for matched traits, implying that for a proportion of the genes, the dose-response curve can be extrapolated to the rare and more severe end of the genotype-trait spectrum.
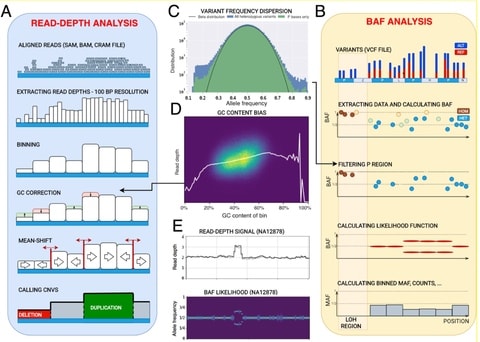
□ CNVpytor: a tool for CNV/CNA detection and analysis from read depth and allele imbalance in whole genome sequencing
>> https://www.biorxiv.org/content/10.1101/2021.01.27.428472v1.full.pdf
CNVpytor inherits the reimplemented core engine of CNVnator. it enables consideration of allele frequency of single nucleotide polymorphism (SNP) and small indels as an additional source of information for the analysis of CNV/CNA and copy number neutral variations.
CNVpytor calculates the likelihood function that describes an imbalance between haplotypes. Currently, BAF information is used when genotyping a specific genomic region where, along with estimated copy number, the output contains the average BAF level.
□ ICN: Extracting interconnected communities in gene Co-expression networks
>> https://academic.oup.com/bioinformatics/advance-article-abstract/doi/10.1093/bioinformatics/btab047/6122693
The interconnected community structure is more flexible and provides a better fit to the empirical co-expression matrix. ICN, an efficient algorithm by leveraging advanced graph norm shrinkage approach.
□ Long Reads Capture Simultaneous Enhancer-Promoter Methylation Status for Cell-type Deconvolution
>> https://www.biorxiv.org/content/10.1101/2021.01.28.428654v1.full.pdf
Despite focusing on Bionano Genomics reduced-representation optical methylation mapping (ROM), which currently provides the highest coverage of long reads, the principles are valid to other future datasets such as those produced by Oxford Nanopore ultralong-read sequencing protocol.
□ TARA: Data-driven biological network alignment that uses topological, sequence, and functional information
>> https://bmcbioinformatics.biomedcentral.com/articles/10.1186/s12859-021-03971-6
TARA-TS (TARA within-network Topology and across-network Sequence information) generalizes a prominent network embedding method that was proposed for within-a-single-network machine learning tasks such as node classification, clustering to the across-network of biological NA.
□ SOM-VN: Self-organizing maps with variable neighborhoods facilitate learning of chromatin accessibility signal shapes associated with regulatory elements
>> https://bmcbioinformatics.biomedcentral.com/articles/10.1186/s12859-021-03976-1
Self-Organizing Map with Variable Neighborhoods (SOM-VN) learns a set of representative shapes from a single, genome-wide, chromatin accessibility dataset to associate with a chromatin state assignment in which a particular RE is prevalent.
□ A dynamic recursive feature elimination framework (dRFE) to further refine a set of OMIC biomarkers
>> https://academic.oup.com/bioinformatics/advance-article-abstract/doi/10.1093/bioinformatics/btab055/6124282
a dynamic recursive feature elimination (dRFE) framework with more flexible feature elimination operations.
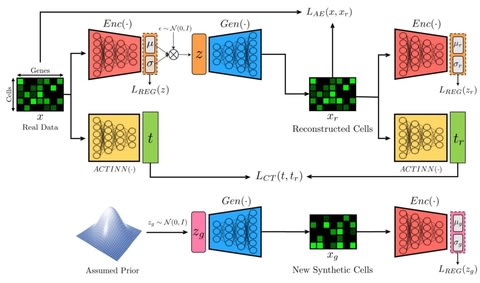
□ ACTIVA: realistic single-cell RNA-seq generation with automatic cell-type identification using introspective variational autoencoders
>> https://www.biorxiv.org/content/10.1101/2021.01.28.428725v1.full.pdf
ACTIVA (Automated Cell-Type-informed Introspective Variational Autoencoder) performs comparable to the state-of-the-art GAN models, scGAN and cscGAN, and trains significantly faster and maintains stability.
Deep investigation of the learned manifold of ACTIVA can further improve the interpretability, and also hypothesize that assuming a dierent prior such as a Zero Inflated Negative Binomial or a Poisson distribution could further improve the quality of generated data.

□ A Fast Lasso-based Method for Inferring Pairwise Interactions
>> https://www.biorxiv.org/content/10.1101/2021.01.28.428698v1.full.pdf
A method performs coordinate descent lasso-regression on a matrix containing all pairwise interactions present in the data. It drastically increased the scale of tractable data sets by compressing columns of the matrix using Simple-8b.
This approach to lasso regression is based on a cyclic coordinate descent algorithm. This method begins with βj = 0 for all j and updates the beta values sequentially, with each update attempting to minimise the current total error.

□ pmVAE: Learning Interpretable Single-Cell Representations with Pathway Modules
>> https://www.biorxiv.org/content/10.1101/2021.01.28.428664v1.full.pdf
Global reconstruction is achieved by summing over all pathway module outputs and a global latent representation of the input expression vector is achieved by concatenation of the latent representations from each pathway module.
The pathway modules within pmVAE construct a latent space factorized by pathways. This constructs a latent space factorized by pathway where sections of the embedding explicitly capture the effects of genes participating in the pathway.

□ McSplicer: a probabilistic model for estimating splice site usage from RNA-seq data
>> https://academic.oup.com/bioinformatics/advance-article/doi/10.1093/bioinformatics/btab050/6124273
McSplicer is a probabilistic model for estimating splice site usages, rather than modeling an individual outcome of a splicing process such as exon skipping. The potential splice sites partition a gene into a sequence of segments.
a sequence of hidden variables, each of which indicates whether a corresponding segment is part of a transcript. the splicing process by assuming that this sequence of hidden variables follows an inhomogeneous Markov chain, hence the name Markov chain Splicer.
□ HashSeq: A Simple, Scalable, and Conservative De Novo Variant Caller for 16S rRNA Gene Datasets
>> https://www.biorxiv.org/content/10.1101/2021.01.29.428714v1.full.pdf
HasgSeq, a very simple HashMap based algorithm to detect all sequence variants in a dataset. This resulted unsurprisingly in a large number of one-mismatch sequence variants.
HashSeq uses the normal distribution combined with LOESS regression to estimate background error rates as a function of sequencing depth for individual clusters.
□ Random rotation for identifying differentially expressed genes with linear models following batch effect correction
>> https://academic.oup.com/bioinformatics/advance-article-abstract/doi/10.1093/bioinformatics/btab063/6125383
The approach is based on generating simulated datasets by random rotation and thereby retains the dependence structure of genes adequately.
This allows estimating null distributions of dependent test statistics and thus the calculation of resampling based p-values and false discovery rates following batch effect correction while maintaining the alpha level.

□ LoopViz: A uLoop Assembly Clone Verification Tool for Nanopore Sequencing Reads
> https://www.biorxiv.org/content/10.1101/2021.02.01.427927v1.full.pdf
Loop assembly (uLOOP) is a recursive, Golden Gate-like assembly method that allows rapid cloning of domesticated DNA fragments to robustly refactor novel pathways.
LoopViz identifies full length reads originating from a single plasmid in the population, and visualizes them in terms of a user input DNA fragments file, and provides QC statistics.
□ sdcorGCN: Robust gene coexpression networks using signed distance correlation
>> https://academic.oup.com/bioinformatics/advance-article-abstract/doi/10.1093/bioinformatics/btab041/6125359
Distance correlation offers a more intuitive approach to network construction than commonly used methods such as Pearson correlation and mutual information.
sdcorGCN, a framework to generate self-consistent networks using signed distance correlation purely from gene expression data, with no additional information.
□ SpatialDWLS: accurate deconvolution of spatial transcriptomic data
>> https://www.biorxiv.org/content/10.1101/2021.02.02.429429v1.full.pdf
the cell type composition at each location is inferred by extending the dampened weighted least squares (DWLS) method, which was originally developed for deconvolving bulk RNAseq data.
In parallel, single-cell RNAseq analysis was carried out to identify cell-type specific gene signatures. The spatialDWLS method was applied to infer the distribution of different cell-types across developmental stages.
※コメント投稿者のブログIDはブログ作成者のみに通知されます