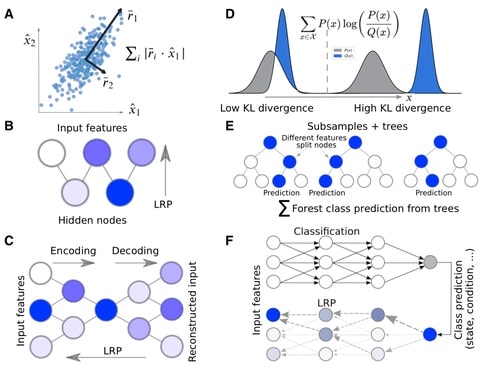
□ Molecular Insights from Conformational Ensembles via Machine Learning
>> https://www.cell.com/biophysj/pdfExtended/S0006-3495(19)34401-7
Learning ensemble properties from molecular simulations and provide easily interpretable metrics of important features with prominent ML methods of varying complexity, incl. PCA, RFs, autoencoders, restricted Boltzmann machines, and multilayer perceptrons (MLPs).
MLP, which has the ability to approximate nonlinear classification functions because of its multilayer architecture and use of activation functions, successfully identified the majority of the important features from unaligned Cartesian coordinates.
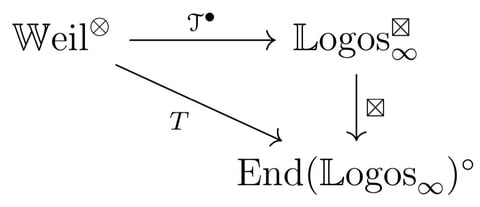
□ Dual tangent structures for infinity-toposes
>> https://arxiv.org/pdf/2101.08805v1.pdf
the tangent structure on the ∞-category of differentiable ∞-categories. That tangent structure encodes the ideas of Goodwillie’s calculus of functors and highlights the analogy between that theory and the ordinary differential calculus of smooth manifolds.
Topos∞, the ∞-category of ∞-toposes and geometric morphisms, and the opposite ∞-category Topos. The ‘algebraic’ morphisms between two ∞-toposes are those that preserve colimits and finite limits; i.e. the left adjoints of the geometric morphisms.
□ The Linear Dynamics of Wave Functions in Causal Fermion Systems
>> https://arxiv.org/pdf/2101.08673v1.pdf
The dynamics of spinorial wave functions in a causal fermion system, so-called dynamical wave equation is derived. Its solutions form a Hilbert space, whose scalar product is represented by a conserved surface layer integral.
In order to obtain a space which can be thought of as being a generalization of the Hilbert space of all Dirac solutions, and extending H only by those physical wave functions obtained when the physical system is varied while preserving the Euler-Lagrange equations.
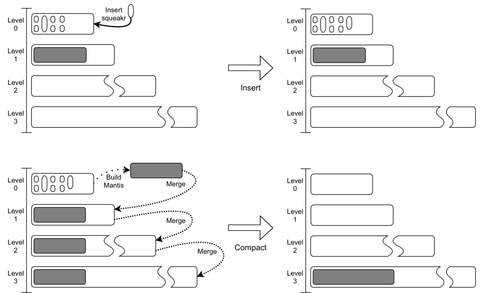
□ Dynamic Mantis: An Incrementally Updatable and Scalable System for Large-Scale Sequence Search using LSM Trees
>> https://www.biorxiv.org/content/10.1101/2021.02.05.429839v1.full.pdf
Minimum Spanning Tree-based Mantis using the Bentley-Saxe transformation to support efficient updates. Mantis’s scalability by constructing an index of ≈40K samples from SRA by adding samples one at a time to an initial index of 10K samples.
VariMerge and Bifrost scaled to only 5K and 80 samples, respectively, while Mantis scaled to more than 39K samples. Queries were over 24× faster in Mantis than in Bifrost. Mantis indexes were about 2.5× smaller than Bifrost’s indexes and about half as big as VariMerge’s indexes.
Assuming the merging algorithm runs in linear time, then Bentley-Saxes increases the costs of insertions by a factor of O(rlogrN/M) and the cost of queries by a factor of O(logrN/M). Querying for a k-mer in Squeakr takes O(1) time, so queries in the Dynamic Mantis cost O(M +Q(N)logr N/M).
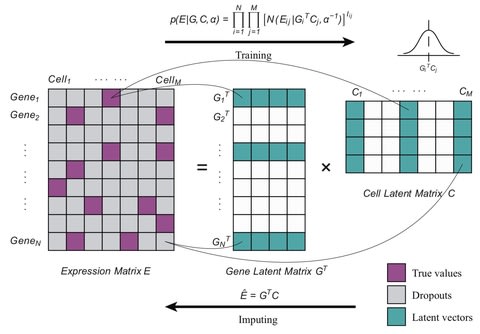
□ Bfimpute: A Bayesian factorization method to recover single-cell RNA sequencing data
>> https://www.biorxiv.org/content/10.1101/2021.02.10.430649v1.full.pdf
Bfimpute uses full Bayesian probabilistic matrix factorization to describe the latent information for genes and carries out a Markov chain Monte Carlo scheme which is able to easily incorporate any gene or cell related information to train the model and imputate.
Bfimpute performs better than the other imputation methods: scImpute, SAVER, VIPER, DrImpute, MAGIC, and SCRABBLE in scRNA-seq datasets on improving clustering and differential gene expression analyses and recovering gene expression temporal dynamics.
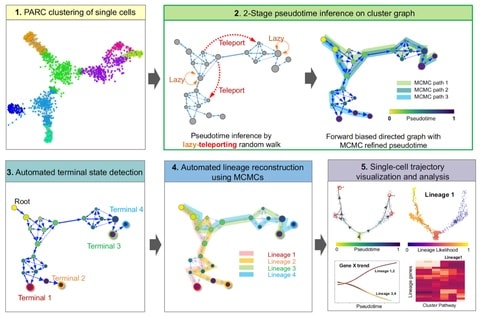
□ VIA: Generalized and scalable trajectory inference in single-cell omics data
>> https://www.biorxiv.org/content/10.1101/2021.02.10.430705v1.full.pdf
VIA, a graph-based trajectory inference (TI) algorithm that uses a new strategy to compute pseudotime, and reconstruct cell lineages based on lazy-teleporting random walks integrated with Markov chain Monte Carlo (MCMC) refinement.
VIA outperforms other TI algorithms in terms of capturing cellular trajectories not limited to multi-furcations, but also disconnected and cyclic topologies. By combining lazy-teleporting random walks and MCMC, VIA relaxes common constraints on graph traversal and causality.
□ FFW: Detecting differentially methylated regions using a fast wavelet-based approach to functional association analysis
>> https://bmcbioinformatics.biomedcentral.com/articles/10.1186/s12859-021-03979-y
FFW, Fast Functional Wavelet combines the WaveQTL framework with the theoretical null distribution of Bayes factors. The main difference between FFW and WaveQTL is that FFW requires regressing the trait of interest on the wavelet coefficients, regardless of the application.
Both WaveQTL and FFW offer a more flexible approach to modeling functions than conventional single-point testing. By keeping the design matrix constant across the screened regions and using simulations instead of permutations, FFW is faster than WaveQTL.

□ ChainX: Co-linear chaining with overlaps and gap costs
>> https://www.biorxiv.org/content/10.1101/2021.02.03.429492v1.full.pdf
ChainX computes optimal co-linear chaining cost between an input target and query sequences. It supports global and semi-global comparison modes, where the latter allows free end-gaps on a query sequence. It can serve as a faster alternative to computing edit distances.
ChainX is the the first subquadratic time algorithms, and solves the co-linear chaining problem with anchor overlaps and gap costs in ~O(n) time, where n denotes the count of anchors.
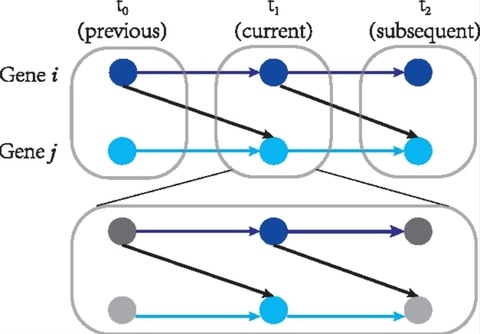
□ CITL: Inferring time-lagged causality using the derivative of single-cell expression
>> https://www.biorxiv.org/content/10.1101/2021.02.03.429525v1.full.pdf
CITL can infer non-time-lagged relationships, referred to as instant causal relationships. This assumes that the current expression level of a gene results from its previous expression level and the current expression level of its causes.
CITL estimates the changing expression levels of genes by “RNA velocity”. CITL infers different types of causality from previous methods that only used the current expression level of genes. Time-lagged causality may represent the relationships involving multi-modal variables.

□ ASIGNTF: AGNOSTIC SIGNATURE USING NTF: A UNIVERSAL AGNOSTIC STRATEGY TO ESTIMATE CELL-TYPES ABUNDANCE FROM TRANSCRIPTOMIC DATASETS
>> https://www.biorxiv.org/content/10.1101/2021.02.04.429589v1.full.pdf
ASigNTF: Agnostic Signature using Non-negative Tensor Factorization, to perform the deconvolution of cell types from transcriptomics data. NTF allows the grouping of closely related cell types without previous knowledge of cell biology to make them suitable for deconvolution.
ASigNTF, which is based on two complementary statistical/mathematical tools: non-negative tensor factorization (for dimensionality reduction) and the Herfindahl-Hirschman index.
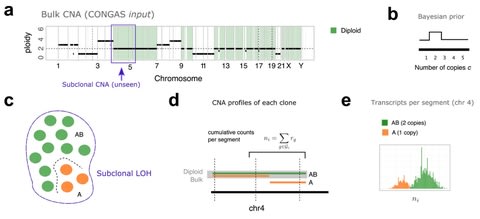
□ CONGAS: Genotyping Copy Number Alterations from single-cell RNA sequencing
>> https://www.biorxiv.org/content/10.1101/2021.02.02.429335v1.full.pdf
CONGAS, a Bayesian method to genotype CNA calls from single-cell RNAseq data, and cluster cells into subpopulations with the same CNA profile.
CONGAS is based on a mixture of Poisson distributions and uses, as input, absolute counts of transcripts from single-cell RNAseq. The model requires to know, in advance, also a segmentation of the genome and the ploidy of each segment.
The CONGAS model exists in both parametric and non-parametric form as a mixture of k ≥ 1 subclones with different CNA profiles. The model is then either a finite Dirichlet mixture with k clusters, or a Dirichlet Process with a stick-breaking construction.
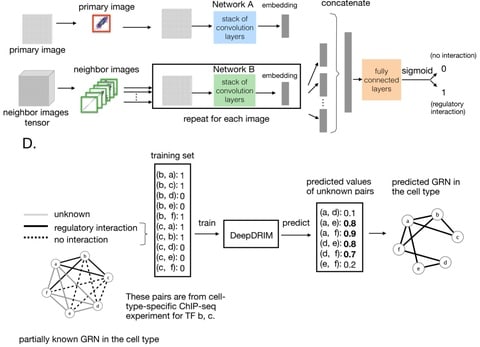
□ DeepDRIM: a deep neural network to reconstruct cell-type-specific gene regulatory network using single-cell RNA-seq data
>> https://www.biorxiv.org/content/10.1101/2021.02.03.429484v1.full.pdf
DeepDRIM a supervised deep neural network that represents gene pair joint expression as images and considers the neighborhood context to eliminate the transitive interactions.
DeepDRIM converts the numerical representation of TF-gene expression to an image and applies a CNN to embed it into a lower dimension. DeepDRIM requires validated TF-gene pairs for use as a training set to highlight the key areas in the embedding space.

□ RLZ-Graph: Constructing smaller genome graphs via string compression
>> https://www.biorxiv.org/content/10.1101/2021.02.08.430279v1.full.pdf
Defining a restricted genome graph and formalize the restricted genome graph optimization problem, which seeks to build a smallest restricted genome graph given a collection of strings.
RLZ-Graph, a genome graph constructed based on the relative Lempel-Ziv external pointer macro (EPM) algorithm. Among the approximation heuristics to solve the EPM compression problem, the relative Lempel-Ziv algorithm runs in linear time and achieves good compression ratios.
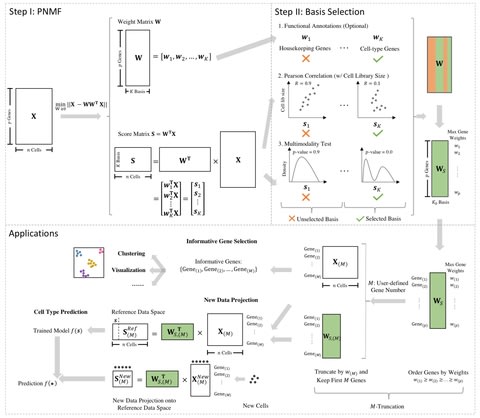
□ scPNMF: sparse gene encoding of single cells to facilitate gene selection for targeted gene profiling
>> https://www.biorxiv.org/content/10.1101/2021.02.09.430550v1.full.pdf
single-cell Projective Non-negative Matrix Factorization (scPNMF) combines the advantages of PCA and NMF by outputting a non-negative sparse weight matrix that can project cells in a high-dimensional scRNA-seq dataset onto a low-dimensional space.
The input of scPNMF is a log-transformed gene-by-cell count matrix. The output includes the selected weight matrix, a sparse and mutually exclusive encoding of genes as new, low dimensions, and the score matrix containing embeddings of input cells in the low dimensions.

□ ACE: Explaining cluster from an adversarial perspective
>>
Adversarial Clustering Explanation (ACE), projects scRNA-seq data to a latent space, clusters the cells in that space, and identifies sets of genes that succinctly explain the differences among the discovered clusters.
ACE first “neuralizes” the clustering procedure by reformulating it as a functionally equivalent multi-layer neural network. ACE is able to attribute the cell’s group assignments all the way back to the input genes by leveraging gradient-based neural network explanation methods.
□ Identity: rapid alignment-free prediction of sequence alignment identity scores using self-supervised general linear models
>> https://academic.oup.com/nargab/article/3/1/lqab001/6125549
Fast alternatives such as k-mer distances produce scores that do not have relevant biological meanings as the identity scores produced by alignment algorithms.
Identity, a novel method for generating sequences with known identity scores, allowing for alignment-free prediction of alignment identity scores. This is the first time identity scores are obtained in linear time O(n) using linear space.
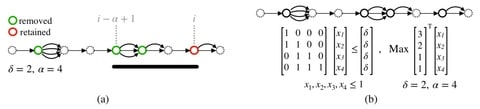
□ VF: A variant selection framework for genome graphs
>> https://www.biorxiv.org/content/10.1101/2021.02.02.429378v1.full.pdf
VF, a novel mathematical framework for variant selection, by casting it in terms of minimizing variation graph size subject to preserving paths of length α with at most δ differences.
This framework leads to a rich set of problems based on the types of variants (SNPs, indels), and whether the goal is to minimize the number of positions at which variants are listed or to minimize the total number of variants listed.
When VF algorithm is run with parameter settings amenable to long-read mapping (α = 10 kbp, δ = 1000), 99.99% SNPs and 73% indel structural variants can be safely excluded from human chromosome 1 variation graph.

□ GRAFIMO: variant and haplotype aware motif scanning on pangenome graphs
>> https://www.biorxiv.org/content/10.1101/2021.02.04.429752v1.full.pdf
GRAFIMO (GRAph-based Finding of Individual Motif Occurrences), a command-line tool for the scanning of known TF DNA motifs represented as Position Weight Matrices (PWMs) in VGs.
Given a reference genome and a set of genomic variants with respect to the reference, GRAFIMO interfaces with the VG software suite to build the main VG data structure, the XG graph index and the GBWT index used to track the haplotypes within the VG.
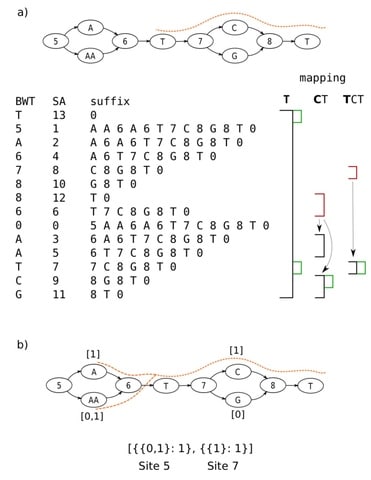
□ Enabling multiscale variation analysis with genome graphs
>> https://www.biorxiv.org/content/10.1101/2021.02.03.429603v1.full.pdf
Modeling the genome as a directed acyclic graph consisting of successive hierarchical subgraphs (“sites”) that naturally incorporate multiscale variation, and introduce an algorithm for genotyping, implemented in the software gramtools.
In gramtools, sequence search in genome graphs is supported using the compressed suffix array of a linearised representation of the graph, which we call variation-aware Burrows-Wheeler Transform (vBWT).
□ Practical selection of representative sets of RNA-seq samples using a hierarchical approach
>> https://www.biorxiv.org/content/10.1101/2021.02.04.429817v1.full.pdf
Hierarchical representative set selection is a divide-and-conquer-like algorithm that breaks the representative set selection into sub-selections and hierarchically selects representative samples through multiple levels.
Using the hierarchical selection (con-sidering one iteration of divide-and-merge with l chunks, chunk size m, and the final merged set size N′), the computational cost is reduced to O(lm2)+O(N′2) = O(N2/l)+O(N′2).
The seeded-chunking has an added computational cost O(Nl). So the total computational cost is O(N2/l) + O(N′2) + O(Nl). With multiple iterations, the computational cost is further reduced. Since m ≪ N, the memory requirement for computing the similarity matrix is greatly reduced.
□ LevioSAM: Fast lift-over of alternate reference alignments
>> https://www.biorxiv.org/content/10.1101/2021.02.05.429867v1.full.pdf
LevioSAM is a tool for lifting SAM/BAM alignments from one reference to another using a VCF file containing population variants. LevioSAM uses succinct data structures and scales efficiently to many threads.
When run downstream of a read aligner, levioSAM completes in less than 13% the time required by an aligner when both are run with 16 threads.
□ SamQL: A Structured Query Language and filtering tool for the SAM/BAM file format
>> https://www.biorxiv.org/content/10.1101/2021.02.03.429524v1.full.pdf
SamQL was developed in the Go programming language that has been designed for multicore and large-scale network servers and big distributed systems.
SamQL consists of a complete lexer that performs lexical analysis, and a parser, that together analyze the syntax of the provided query. SamQL builds an abstract syntax tree (AST) corresponding to the query.

□ HAST: Accurate Haplotype-Resolved Assembly Reveals The Origin Of Structural Variants For Human Trios
>> https://academic.oup.com/bioinformatics/advance-article/doi/10.1093/bioinformatics/btab068/6128392
HAST: Partition stLFR reads based on trio-binning algorithm using parentally unique markers. HAST is the first trio-binning- assembly-based haplotyping tool for co-barcoded reads.
Although the DNA fragment length and read coverage of each fragment vary for different co-barcoded datasets, HAST can cluster reads sharing the same barcodes and retain the long-range phased sequence information.
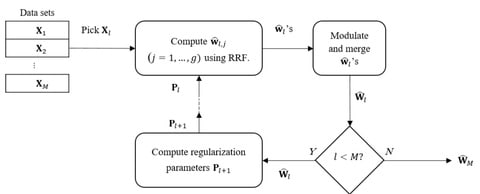
□ GENEREF: Reconstruction of Gene Regulatory Networks using Multiple Datasets
>> https://pubmed.ncbi.nlm.nih.gov/33539303/
GENEREF can accumulate information from multiple types of data sets in an iterative manner, with each iteration boosting the performance of the prediction results. The model is capable of using multiple types of data sets for the task of GRN reconstruction in arbitrary orders.
GENEREF uses a vector of regularization values for each sub-problem at each iteration. Similar to the AdaBoost algorithm, on the concep- tual level GENEREF can be thought of as machine learning meta algorithm that can exploit various regressors into a single model.
□ jSRC: a flexible and accurate joint learning algorithm for clustering of single-cell RNA-sequencing data
>> https://academic.oup.com/bib/advance-article/doi/10.1093/bib/bbaa433/6127146
Although great efforts have been devoted to clustering of scRNA-seq, the accuracy, scalability and interpretability of available algorithms are not desirable.
They solve these problems by developing a joint learning algorithm [a.k.a. joints sparse representation and clustering (jSRC)], where the dimension reduction (DR) and clustering are integrated.
□ CVTree: A Parallel Alignment-free Phylogeny and Taxonomy Tool based on Composition Vectors of Genomes
>> https://www.biorxiv.org/content/10.1101/2021.02.04.429726v1.full.pdf
CVTree stands for Composition Vector Tree which is the implementation of an alignment-free algorithm to generate a dissimilarity matrix from comparatively large collection of DNA sequences.
And since the complexity of the CVTree algorithm is lower than linear complexity with the length of genome sequences, CVTree is efficient to handle huge whole genomes, and obtained the phylogenetic relationship.
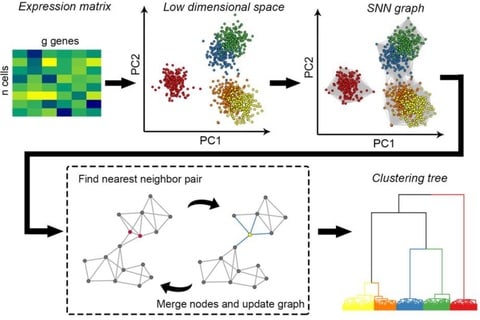
□ HGC: fast hierarchical clustering for large-scale single-cell data
>> https://www.biorxiv.org/content/10.1101/2021.02.07.430106v1.full.pdf
HGC combines the advantages of graph-based clustering and hierarchical clustering. On the shared nearest neighbor graph of cells, HGC constructs the hierarchical tree with linear time complexity.
HGC constructs SNN graph in the PC space, and a recursive procedure of finding the nearest-neighbor node pairs and updating the graph by merging the node pairs. HGC outputs a dendrogram like classical hierarchical clustering.
□ Sequencing DNA In Orbit
>> http://spaceref.com/onorbit/sequencing-dna-in-orbit.html
□ IUPACpal: efficient identification of inverted repeats in IUPAC-encoded DNA sequences
>> https://bmcbioinformatics.biomedcentral.com/articles/10.1186/s12859-021-03983-2
An inverted repeat (IR) is a single stranded sequence of nucleotides with a subsequent downstream sequence consisting of its reverse complement.
Any sequence of nucleotides appearing between the initial component and its reverse complement is referred to as the gap (or the spacer) of the IR. The gap’s size may be of any length, including zero.
IUPACPAL identifies many previously unidentified inverted repeats when compared with EMBOSS, and that this is also performed with orders of magnitude improved speed.
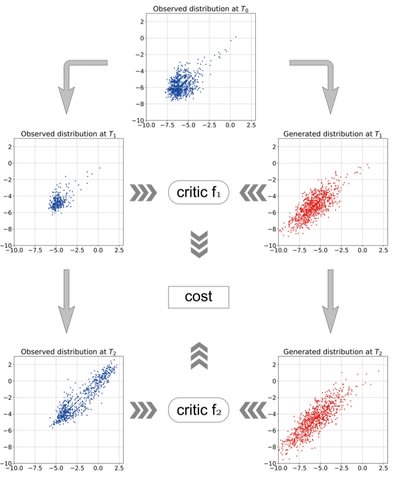
□ A data-driven method to learn a jump diffusion process from aggregate biological gene expression data
>> https://www.biorxiv.org/content/10.1101/2021.02.06.430082v1.full.pdf
The algorithm needs aggregate gene expression data as input and outputs the parameters of the jump diffusion process. The learned jump diffusion process can predict population distributions of GE at any developmental stage, achieve long-time trajectories for individual cells.
Gene expression data at a time point is treated as an empirical marginal distribution of a stochastic process. The Wasserstein distance between the empirical distribution and predicted distribution by the jump diffusion process is minimized to learn the dynamics.
□ Impact of concurrency on the performance of a whole exome sequencing pipeline
>> https://bmcbioinformatics.biomedcentral.com/articles/10.1186/s12859-020-03780-3
CES, concurrent execution strategy equally distributes the available processors across every sample’s pipeline.
CES implicitly tries to minimize the impact of sub-linearity of PaCo tasks on the overall total execution performance, which makes it even more suitable for pipelines that are heavily built around PaCo tasks. CES speedups over naive parallel strategy (NPS) up to 2–2.4.
□ deNOPA: Decoding nucleosome positions with ATAC-seq data at single-cell level
>> https://www.biorxiv.org/content/10.1101/2021.02.07.430096v1.full.pdf
deNOPA not only outperformed state-of-the-art tools, but it is the only tool able to predict nucleosome position precisely with ultrasparse ATAC-seq data.
The remarkable performance of deNOPA was fueled by the reads from short fragments, which compose nearly half of sequenced reads and are normally discarded from nucleosome position detection.
□ ldsep: Scalable Bias-corrected Linkage Disequilibrium Estimation Under Genotype Uncertainty
>> https://www.biorxiv.org/content/10.1101/2021.02.08.430270v1.full.pdf
ldsep: scalable moment-based adjustments to LD estimates based on the marginal posterior distributions of. these moment-based estimators are as accurate as maximum likelihood estimators, and are almost as fast as naive approaches based only on posterior mean genotypes.
the moment-based techniques which used in this manuscript, when applied to simple linear regression with an additive effects model (where the SNP effect is pro- portional to the dosage), result in the standard ordinary least squares estimates when using the posterior mean as a covariate.
< br />

□ S-conLSH: alignment-free gapped mapping of noisy long reads
>> https://bmcbioinformatics.biomedcentral.com/articles/10.1186/s12859-020-03918-3
S-conLSH utilizes the same hash function for computing the hash values and retrieves sequences of the reference genome that are hashed in the same position as the read. the locations of the sequences w/ the highest hits are chained as an alignment-free mapping of the query read.
S-conLSH uses Spaced context based Locality Sensitive Hashing. The spaced-context is especially suitable for extracting distant similarities. The variable-length spaced-seeds or patterns add flexibility to the algorithm by introducing gapped mapping of the noisy long reads.
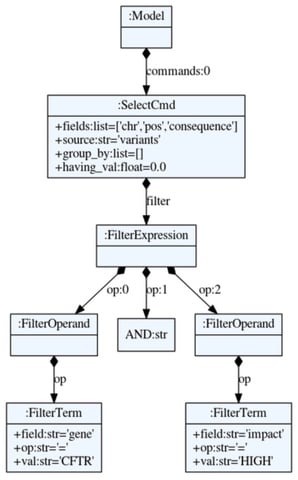
□ Cutevariant: a GUI-based desktop application to explore genetics variations
>> https://www.biorxiv.org/content/10.1101/2021.02.10.430619v1.full.pdf
The syntax of VQL makes use of the Python module textX which provides several tools to define a grammar and create parsers with an Abstract Syntax Tree.
Cutevariant is a cross-plateform application dedicated to maniupulate and filter variation from annotated VCF file. Cutevariant imports data into a local relational database wherefrom complex filter-queries can be built either from the intuitive GUI or using a Domain Specific Language (DSL).
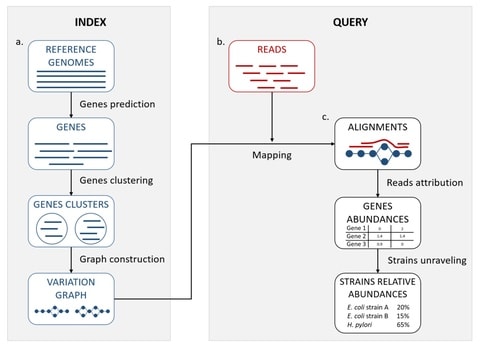
□ StrainFLAIR: Strain-level profiling of metagenomic samples using variation graphs
>> https://www.biorxiv.org/content/10.1101/2021.02.12.430979v1.full.pdf
StrainFLAIR is sub-divided into two main parts: first, an indexing step that stores clusters of reference genes into variation graphs, and then, a query step using mapping of metagenomic reads to infere strain-level abundances in the queried sample.
StrainFLAIR integrated a threshold on the proportion of specific genes detected that can be further explored to refine which strain abundances are set to zero.
□ CoDaCoRe: Learning Sparse Log-Ratios for High-Throughput Sequencing Data
>> https://www.biorxiv.org/content/10.1101/2021.02.11.430695v1.full.pdf
CoDaCoRe, a novel learning algorithm for Compositional Data Continuous Relaxations. Combinatorial optimization over the set of log-ratios (equivalent to the set of pairs of disjoint subsets of the covariates), by continuous relaxation that can be optimized using gradient descent.
CoDaCoRe ensembles multiple regressors in a stage-wise additive fashion, where each successive balance is fitted on the residual from the current model. CoDaCoRe identifies a sequence of balances, in decreasing order of importance, each of which is sparse and interpretable.
※コメント投稿者のブログIDはブログ作成者のみに通知されます