

□ Retrotransposons hijack alt-EJ for DNA replication and eccDNA biogenesis
>> https://www.nature.com/articles/s41586-023-06327-7
Retrotransposons hijack the alternative end-joining (alt-EJ) DNA repair process of the host for a circularization step to synthesize their second-strand DNA. Using Nanopore sequencing to examine the fates of replicated retrotransposon DNA.
Using extrachromosomal circular DNA production as a readout, further genetic screens identified factors from alt-EJ as essential for retrotransposon replication. alt-EJ drives the second-strand synthesis of the long terminal repeat retrotransposon DNA through a circularization.

□ fortuna: Counting pseudoalignments to novel splicing events
>> https://academic.oup.com/bioinformatics/advance-article/doi/10.1093/bioinformatics/btad419/7222626
Using pairing information during mapping could potentially further improve mapping accuracy, but in contrast to genomic mappings the unknown structure of the originating transcript would only impose weak constraints on mapping locations.
fortuna creates a set of sequence fragments of guessed novel transcripts that contain all possible combinations of unspliced exonic segments. fortuna pseudoaligns reads to fragments using kallisto and derives counts of the most elementary splicing units from equivalence classes.

□ Distinguishing word identity and sequence context in DNA language models
>> https://www.biorxiv.org/content/10.1101/2023.07.11.548593v1
To build a framework to extract information content from foundation DNA language models, they used DNABERT a transformer model1 with a Bidirectional Encoder Representations from Transformers (BERT) architecture.
DNABERT struggled to predict next-k-mers of the same size that it managed to predict when masked. Evaluation for contextualized learning w/ maximum explainable variance also showed that average embedding of the tokens explains more maximum variance than the static W2V embedding.

□ TFvelo: gene regulation inspired RNA velocity estimation
>> https://www.biorxiv.org/content/10.1101/2023.07.12.548785v1
The insight behind TFvelo that the clockwise curve on the joint plot between two variables indicates the potential causality with time-delay, can provide a new perspective to infer the regulation relationship from single cell data.
TFvelo can be used to infer the pseudo time, cell trajectory and detect key TF-target regulation. TFvelo relies on a generalized EM algorithm, which iteratively updates the weights of the TFs, the latent time of cells, and the parameters in the dynamic equation.

□ Cytocipher determines significantly different populations of cells in single cell RNA-seq data
>> https://academic.oup.com/bioinformatics/advance-article/doi/10.1093/bioinformatics/btad435/7224247
Cytocipher refers back to the original gene expression measurements, and performs per-cell enrichment scoring for cluster marker genes and a bi-directional statistical test to infer significantly different clusters.
Cytocipher would be sensitive to transcriptionally distinct intermediate states, potentially allowing for identification of fine-grained branch points that represent lineage decisions toward terminal cell fates.

□ Mellon: Quantifying Cell-State Densities in Single-Cell Phenotypic Landscapes
>> https://www.biorxiv.org/content/10.1101/2023.07.09.548272v1
Mellon is a non-parametric cell-state density estimator based on a nearest-neighbors-distance distribution. It uses a sparse gaussian process to produce a differntiable density function that can be evaluated out of sample.
Mellon connects densities between highly similar cell-states using Gaussian processes to accurately and robustly compute cell-state densities that characterize single-cell phenotypic landscapes.
Mellon infers a continuous density function across the high-dimensional cell-state space, capturing the essential characteristics of the cell population in its entirety. The density function can also be used to determine cell-state densities at single-cell resolution.

□ mapquik: Efficient mapping of accurate long reads in minimizer space
>> https://genome.cshlp.org/content/early/2023/06/29/gr.277679.123
mapquik, a novel strategy that creates accurate longer seeds by anchoring alignments through matches of k consecutively-sampled minimizers (k-min-mers) and only indexing k-min-mers that occur once in the reference genome, thereby unlocking ultra-fast mapping.
mapquik significantly accelerates the seeding and chaining steps. These accelerations are enabled not only from minimizer-space seeding but also a novel heuristic O(n) pseudo-chaining algorithm, which improves upon the long-standing O(n log n) bound.

□ MiGCN: Predicting Disease-gene Associations through Self-supervised Mutual Infomax Graph Convolution Network
>> https://www.biorxiv.org/content/10.1101/2023.07.13.548865v1
Self-Supervised Mutual Infomax GraphConvolution Network (MiGCN), a new method to predict disease-gene associations under the guidance of external disease-disease and gene-gene collaborative graphs.
MiGCN constructs two collaborative graphs from external gene-gene interactions and disease-disease associations information, which are individually input into a self-supervised mutual infomax module to learn the node embeddings by maximizing mutual information.

□ UNI-RNA: UNIVERSAL PRE-TRAINED MODELS REVOLUTIONIZE RNA RESEARCH
>> https://www.biorxiv.org/content/10.1101/2023.07.11.548588v1
Uni-RNA, a series of context-aware deep learning models. Based on the BERT architecture, advanced techniques such as rotary embedding, flash attention, and fused layernorm were integrated for optimal performance in terms of training efficiency and representational capabilities.
Uni-RNA models performed pre-training using 1 billion RNA sequences from different species and categories. To remove sequence redundancy, MMseqs2 clustering is employed. Uni-RNA enables direct prediction of modifications across full-length sequences.

□ CAJAL enables analysis and integration of single-cell morphological data using metric geometry
>> https://www.nature.com/articles/s41467-023-39424-2
CAJAL infers cell morphology latent spaces where distances between points indicate the amount of physical deformation required to change the morphology of one cell into that of another.
CAJAL enables the characterization of morphological cellular processes from a biophysical perspective and produces an actual mathematical distance upon which rigorous algebraic and statistical analytic approaches can be built.

□ scGPTHub: Single-Cell Foundation Models for Everyone
>> https://scgpthub.org/
scGPT Hub provides access to the scGPT model via a convenient user interface. The scGPT model is the first single-cell foundation model built through generative pre-training on over 33 million cells.
By adapting the transformer architecture, scGPT enables the simultaneous learning of cell and gene representations, facilitating a comprehensive understanding of cellular characteristics based on gene expression.

□ SPADE: Spatial pattern and differential expression analysis with spatial transcriptomic data
>> https://www.biorxiv.org/content/10.1101/2023.07.06.547967v1
SPADE for spatial pattern and differential expression analysis to identify SV genes in complex tissues using spatial transcriptomic data. SPADE employes a Gaussian process regression (GPR) model with a gene-specific Gaussian kernel to enable accurate detection of SV genes.
SPADE provides a framework for detecting SV genes between groups using a crossed likelihood-ratio test. SPADE estimates the optimal hyperparameter for kernel matrix in each group. For each gene, the log likelihood in each group can be easily calculated with its optimal kernel.
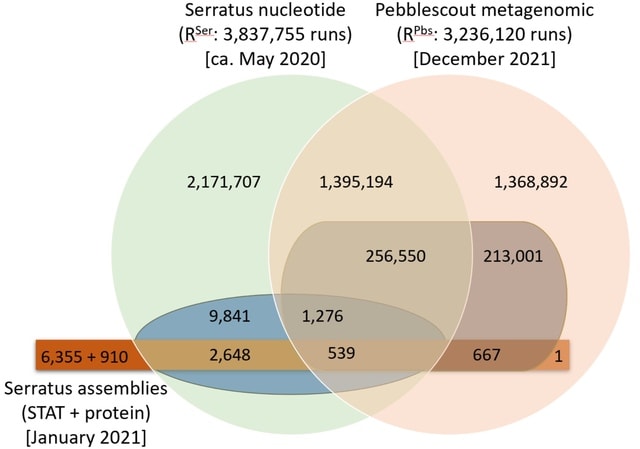
□ Pebblescout: Indexing and searching petabyte-scale nucleotide resources
>> https://www.biorxiv.org/content/10.1101/2023.07.09.547343v1
Pebblescout can be used for (i) indexing sequence data in a resource once and (ii) searching the index to produce a ranked list for the subset of the resource with matches to any user query; the guarantee on the match length is determined by the parameters used for indexing.
Pebblescout requires a network attached random access storage array. Pebblescout score considers only unmasked kmers sampled from the query. The score for a subject normalizes the sum of kmer scores for all kmers considered from the query that match the subject.

□ GreenHill: a de novo chromosome-level scaffolding and phasing tool using Hi-C https://genomebiology.biomedcentral.com/articles/10.1186/s13059-023-03006-8
GreenHill receives assembled contigs from other assembler as inputs. Any format of contigs is acceptable,such as paired-haplotype, pseudo-haplotype, and haplotype-ignorant styles.
GreenHill-based assemblies have greater phasing accuracy than FALCON-phase-based assemblies. Using a newly developed algorithm, long reads and Hi-C were synergistically used to improve the accuracy of the resulting haplotypes.

□ SCS: cell segmentation for high-resolution spatial transcriptomics
>> https://www.nature.com/articles/s41592-023-01939-3
Existing cell segmentation methods for this data only rely on the stained image, which do not fully utilize the information provided by the experiment leading to less accurate results.
SCS (subcellular spatial transcriptomics cell segmentation) combines imaging data with sequencing data to improve cell segmentation accuracy. SCS assigns spots to cells by adaptively learning the position of each spot relative to the center of its cell using a transformer.

□ kGWASflow: a modular, flexible, and reproducible Snakemake workflow for k-mers-based GWAS
>> https://www.biorxiv.org/content/10.1101/2023.07.10.548365v1
kGWASflow conducts k-mer-based GWAS while offering enhanced pre- and post-GWAS analysis capabilities. kGWASflow offers extensive customization, either via the command line or a configuration file, enabling users to modify the workflow to their specific requirements.
kGWASflow initially retrieves the source reads for each associated k-mer from the FASTQ files of samples containing those k-mers. kGWASflow also converts the alignment outputs into BAM and BED files for downstream analysis.
kGWASflow first performs a de-novo assembly of the source reads using SPADES. After the assembly step, kGWASflow runs minimap2 to map assembled contigs onto a reference genome FASTA file.

□ SEESAW: detecting isoform-level allelic imbalance accounting for inferential uncertainty
>> https://genomebiology.biomedcentral.com/articles/10.1186/s13059-023-03003-x
Statistical Estimation of Allelic Expression using Salmon and Swish (SEESAW), for allelic quantification and inference of AI patterns. Aggregating isoform-level expression estimates to the TSS level can have higher sensitivity than either gene- or isoform-level analysis.
SEESAW follows the general framework of mmseq and mmdiff for haplotype- and isoform-specific quantification and uncertainty-aware inference. SEESAW assumes that phased genotypes are available, and is designed for multiple replicates / conditions of organisms w/ the same genotype.

□ ENTRAIN: integrating trajectory inference and gene regulatory networks with spatial data to co-localize the receptor-ligand interactions that specify cell fate
>> https://www.biorxiv.org/content/10.1101/2023.07.09.548284v1
ENTRAIN (ENvironment-aware TRajectory INference), a computational method that integrates trajectory inference methods with ligand-receptor pair gene regulatory networks to identify extracellular signals and evaluate their relative contribution towards a differentiation trajectory.
ENTRAIN-Pseudotime, ENTRAIN-Velocity, and ENTRAIN-Spatial, which can be applied on the outputs of pseudotime-based methods, RNA velocity or paired single-cell and spatially resolved data. ENTRAIN determines driver ligands responsible for observed RNA velocity vectors.

□ RNAGEN: A generative adversarial network-based model to generate synthetic RNA sequences to target proteins
>> https://www.biorxiv.org/content/10.1101/2023.07.11.548246v1
The RNAGEN model is a deep generative adversarial network (GAN) that learns to generate piRNA sequences with similar characteristics to the natural ones. This model is a novel version of the WGAN-GP architecture for one-hot encoded RNA sequences.
RNAGEN provides improved training over the original Convolutional GAN models and is less prone to overfitting than the WGAN architecture. RNAGEN learns latent vectors that lead to the generation of optimized piRNA sequences with improved binding scores to the target protein.

□ Hyperparameter optimisation in differential evolution using Summed Local Difference Strings, a rugged but easily calculated landscape for combinatorial search problems
>> https://www.biorxiv.org/content/10.1101/2023.07.11.548503v1
A simple, related objective function in which the objective is not to maximise each element but to maximise the sum of the differences between adjacent elements. This is very easily calculated, allowing rapid assessment of different search algorithms.
The contribution to the overall fitness of any element of the string is absolutely context-sensitive. The objective function for the hyperparameter optimisation for summed local difference strings has been defined.

□ DNA Storage Designer: A practical and holistic design platform for storing digital information in DNA sequence
>> https://www.biorxiv.org/content/10.1101/2023.07.11.548641v1
DNA Storage Designer, the first online platform to simulate the whole process of DNA storage experiments. This platform offers classical and novel technologies and experimental settings that simulate encoding, error simulation, and decoding for DNA storage system.
DNA Storage Designer enables not only to encode their files and simulate the entire process but also to upload FASTA files and solely simulate the sustaining process of sequences while mimicking the mutation errors along with distribution changes of sequences.

□ Sebastian Raschka
Gzip + kNN beats transformers on text classification.
(Gzip as in good old zip file compression)
“Low-Resource” Text Classification: A Parameter-Free Classification Method with Compressors
>> https://aclanthology.org/2023.findings-acl.426
>> https://twitter.com/rasbt/status/1679472364931670016
□ Rob Patro
>> https://twitter.com/nomad421/status/1679495774743216128
People seem really surprised by this result (it's cool!), but I think it's evidence of how wrapped up we are in the DL craze. There's a storied history of relative compression as a similarly measure. It's not surprising that it may capture something DL methods currently don't.
□ Halvar Flake RT
>> https://twitter.com/halvarflake/status/1679391941123792896
Understanding that every compressor is a machine learning predictor, and vice versa, was the single most important insight I learnt about between 2019 and now.

□ DeepRVAT: Integration of variant annotations using deep set networks boosts rare variant association genetics
>> https://www.biorxiv.org/content/10.1101/2023.07.12.548506v1
DeepRVAT is an end-to-end model that first accounts for nonlinear effects from rare variants on gene function (gene impairment module) to then model variation in one or multiple traits as linear functions of the estimated gene impairment scores.
DeepRVAT employs a deep set neural network architecture to aggregate the effects from multiple discrete and continuous annotations for an arbitrary number of rare variants. The gene impairment module can be used as input to train predictive models for phenotype from genotype.

□ SBOannotator: a Python Tool for the Automated Assignment of Systems Biology Ontology Terms
>> https://academic.oup.com/bioinformatics/advance-article/doi/10.1093/bioinformatics/btad437/7224245
The SBOannotator is the first standalone tool that automatically assigns SBO terms to multiple entities of a given SBML model, The main focus lies on the reactions, as the correct assignment of precise SBO annotations requires their extensive classification.
The SBOannotator can interpret this information and add a precise SBO term for "enzymatic catalyst". Without specifying the exact mechanism of this catalysis, the role of the modifier is now defined through an "is a"-relationship: This modifier is an enzymatic catalyst.

□ Nadavca: Precise Nanopore Signal Modeling Improves Unsupervised Single-Molecule Methylation Detection
>> https://www.biorxiv.org/content/10.1101/2023.07.13.548926v1
Nadavca, a nanopore signal aligner that incorporates several enhancements to the Dynamic Time Warping algorithm. Nadavca's output exhibits improved accuracy by eliminating length distribution artifacts and eliminating the need for event segmentation as a preliminary step.
The core part of Nadavca aligns a portion of nanopore signal to the corresponding part of the reference genome. The objective is to improve the accuracy of an approximate alignment, resulting from aligning base-called reads to the reference.
Nadavca considers a contribution of sub-optimal alignments. Many of these alignments can have scores very close to the optimum, representing uncertainty in the true alignment. Posterior decoding algorithms consider this uncertainty at each position of the alignment.

□ SANDSTORM / GARDN: Generative and predictive neural networks for the design of functional RNA molecules
>> https://www.biorxiv.org/content/10.1101/2023.07.14.549043v1
SANDSTORM, a generalized neural network architecture that utilizes the sequence and structure of RNA molecules to inform functional predictions. SANDSTORM achieves SOTA performance across several distinct RNA prediction tasks, while learning interpretable abstractions.
GARDN, a generative adversarial RNA design networks that allows the generative modelling of novel mRNA 5-prime untranslated regions and toehold switch riboregulators. These paired inputs are passed through parallel convolutional stacks that form an ensemble prediction.

□ TriTan: An efficient triple non-negative matrix factorisation method for integrative analysis of single-cell multiomics data
>> https://www.biorxiv.org/content/10.1101/2023.07.14.549059v1
TriTan (Triple inTegrative fast nonnegative matrix factorisation) decomposes the input single-cell multi-modal matrices into following low-dimensional matrices: a shared cell cluster matrix across all modalities, distinct feature-cluster matrices, and association matrices.
TriTan enables the simultaneous detection of latent cell clusters and feature clusters, as well as the exploration of associations between features, such as the links between genes and potential regulatory peaks.

□ BERLIN: Basic Explorer for single-cell RNAseq analysis and cell Lineage Determination.
>> https://www.biorxiv.org/content/10.1101/2023.07.13.548919v1
BERLIN, a basic analytical pipeline protocol, that outlines a workflow for analyzing scRNAseq data. This protocol encompasses crucial steps, including quality control, normalization, data scaling, dimensionality reduction, clustering, and automated cell annotation.
The output files generated by this protocol, including metadata, H5 Seurat files, cell subpopulation metadata, and ISCVA-compliant files, facilitate downstream analyses and enable integration with other analysis and visualization tools.
BERLIN performs clustering of the cells by constructing a shared nearest neighbor (SNN) graph, which connects cells based on their similarities in gene expression pattern. The Louvain algorithm is applied to optimize the modularity of a network by iteratively assigning nodes.

□ ChomActivity: Integrative epigenomic and functional characterization assay based annotation of regulatory activity across diverse human cell types
>> https://www.biorxiv.org/content/10.1101/2023.07.14.549056v1
ChromActivity, a computational framework that predicts gene regulatory element activity across diverse cell types by integrating information from chromatin marks and multiple functional characterization datasets.
ChromActivity produces two complementary integrative outputs for each cell type. One of them is ChromScoreHMM, which annotates the genome into states representing combinatorial and spatial patterns in the expert's regulatory activity track predictions.
The other is ChromScore, which is a cell type-specific continuous numerical score of predicted regulatory activity potential across the genome based on combining the individual expert predictions.

□ GeCoNet-Tool: a software package for gene co-expression network construction and analysis
>> https://bmcbioinformatics.biomedcentral.com/articles/10.1186/s12859-023-05382-1
In the network construction part, GeCoNet-Tool offers users various options for processing gene co-expression data derived from diverse technologies. The output of the tool is an edge list with the option of weights associated with each link.
In network analysis part, the user can produce a table that includes several network properties such as communities, cores, and centrality measures. With GeCoNet-Tool, users can explore and gain insights into the complex interactions between genes.

□ Huatuo: An analytical framework for decoding cell type-specific genetic variation of gene regulation
>> https://www.nature.com/articles/s41467-023-39538-7
Huatuo, a framework to decode genetic variation of gene regulation at cell type and single-nucleotide resolutions by integrating deep-learning-based variant predictions with population-based association analyses.
Huatuo sheds light on cell type-dependent cis-regulatory loci by investigating the interaction effects between genotypes and estimated cell type proportions with a linear regression model. Huatuo unravels the causal mechanisms underlying genetic variation of gene regulation.

□ RNA Strain-Match: A tool for matching single-nucleus, single-cell, or bulk RNA-sequencing alignment data to its corresponding genotype
>> https://www.biorxiv.org/content/10.1101/2023.07.14.548847v1
RNA Strain-Match, a quality control tool developed to match RNA data in the form of sequence alignment files (i.e. SAM or BAM files) to their corresponding genotype without the use of an RNA variant call format file.
RNA Strain-Match uses known genotyping information - specifically autosomal coding single nucleotide polymorphisms (SNPs) with a single alternative allele - to match RNA sequencing data to corresponding genotypic information.

□ MosaiCatcher v2: a single-cell structural variations detection and analysis reference framework based on Strand-seq
>> https://www.biorxiv.org/content/10.1101/2023.07.13.548805v1
MosaiCatcher v2, a standardised workflow and reference framework for single-cell SV detection using Strand-seq.
MosaiCatcher v2 incorporates a structural variation (S) functional analysis module, which uses nucleosome occupancy data measured directly from Strand-seq libraries (SNOVA) as well as a SV genotyper (ArbiGent).
※コメント投稿者のブログIDはブログ作成者のみに通知されます