

□ First Things First: The Physics of Causality
>> https://fqxi.org/community/articles/display/236
Why do we remember the past and not the future? Untangling the connections between cause and effect, choice, and entropy.
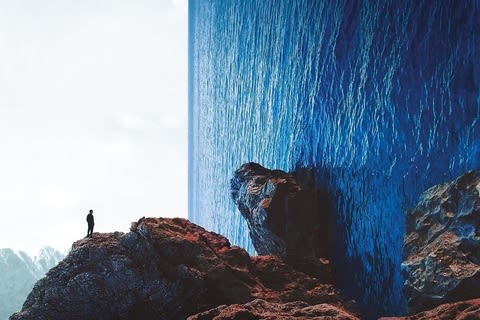
□ Is reality real? How evolution blinds us to the truth about the world
>> https://www.newscientist.com/article/mg24332410-300-is-reality-real-how-evolution-blinds-us-to-the-truth-about-the-world/
Our senses tell us only what we need to survive.

□ Evolutionary constraints in regulatory networks defined by partial order between phenotypes
>> https://www.biorxiv.org/content/biorxiv/early/2019/08/01/722520.full.pdf
the concept of partial order identifies the constraints, and test the predictions by experimentally evolving an engineered signal-integrating network in multiple environments.
expanding in fitness space along the Pareto-optimal front predicted by conflicts in regulatory demands, by fine-tuning binding affinities within the network.

□ Graph-based genome alignment and genotyping with HISAT2 and HISAT-genotype
>> https://www.nature.com/articles/s41587-019-0201-4
A true graph-based genome aligner: HISAT2 and HISAT-Genotype.
a method named HISAT2 (hierarchical indexing for spliced alignment of transcripts 2) that can align both DNA and RNA sequences using a graph Ferragina Manzini index.
Using HISAT2 to represent and search an expanded model of the human reference genome in which over 14.5 million genomic variants in combination with haplotypes are incorporated into the data structure used for searching and alignment.
□ CQF-deNoise: K-mer counting with low memory consumption enables fast clustering of single-cell sequencing data without read alignment
>> https://www.biorxiv.org/content/biorxiv/early/2019/08/02/723833.full.pdf
a fast k-mer counting method, CQF-deNoise, which has a novel component for dynamically identifying and removing false k-mers while preserving counting accuracy.
The k-mer counts from CQF-deNoise produced cell clusters from single-cell RNA-seq data highly consistent with CellRanger but required only 5% of the running time at the same memory consumption while the clusters produced remain highly similar.

□ BLANT - Fast Graphlet Sampling Tool
>> https://academic.oup.com/bioinformatics/advance-article-abstract/doi/10.1093/bioinformatics/btz603/5542947
BLANT, the Basic Local Alignment for Networks Tool is the analog of BLAST, but for networks: given an input graph, it samples small, induced, k-node subgraphs called k-graphlets.
Graphlets have been used to classify networks, quantify structure, align networks both locally and globally, identify topology-function relationships, and build taxonomic trees without the use of sequences.
BLANT offers sampled graphlets in various forms: distributions of graphlets or their orbits; graphlet degree or graphlet orbit degree vectors, the latter being compatible with ORCA.

□ Interpretability logics and generalized Veltman semantics
>> https://arxiv.org/pdf/1907.03849v1.pdf
obtaining modal completeness of the interpretability logics ILP0 and ILR w.r.t. generalized Veltman semantics.
a construction that might be useful for proofs of completeness of extensions of ILW w.r.t. generalized semantics in the future, and demonstrate its usage with ILW* = ILM0W.

□ LTMG: a novel statistical modeling of transcriptional expression states in single-cell RNA-Seq data
>> https://academic.oup.com/nar/advance-article/doi/10.1093/nar/gkz655/5542876
a left truncated mixture Gaussian (LTMG) model, from the kinetic relationships of the transcriptional regulatory inputs, mRNA metabolism and abundance in single cells.
This biological assumption of the low non-zero expressions, rationality of the multimodality setting, and the capability of LTMG in extracting expression states specific to cell types or functions, are validated on independent experimental data sets.
□ DNA Rchitect: An R based visualizer for network analysis of chromatin interaction data
>> https://academic.oup.com/bioinformatics/advance-article-abstract/doi/10.1093/bioinformatics/btz608/5543099
DNA Rchitect is a Shiny App for visualizing genomic data (HiC, mRNA, ChIP, ATAC etc) in bed, bedgraph, and bedpe formats. HiC (bedpe format) data is visualized with both bezier curves coupled with network statistics and graphs (using an R port of igraph).
Using DNA Rchitect, the uploaded data allows the user to visualize different interactions of their sample, perform simple network analyses, while also offering visualization of other genomic data types.
□ circMeta: a unified computational framework for genomic feature annotation and differential expression analysis of circular RNAs
>> https://academic.oup.com/bioinformatics/advance-article-abstract/doi/10.1093/bioinformatics/btz606/5543088
circMeta has three primarily functional modules: (i) a pipeline for comprehensive genomic feature annotation related to circRNA biogenesis, incl length of introns flanking circularized exons, repetitive elements such as Alu elements and SINEs.
(ii) a two-stage DE approach of circRNAs based on circular junction reads to quantitatively compare circRNA levels.
(iii) a Bayesian hierarchical model for DE analysis of circRNAs based on the ratio of circular reads to linear reads in back-splicing sites to study spatial and temporal regulation of circRNA production.
□ scRNABatchQC: Multi-samples quality control for single cell RNA-seq data
>> https://academic.oup.com/bioinformatics/advance-article-abstract/doi/10.1093/bioinformatics/btz601/5542946
scRNABatchQC, an R package to compare multiple sample sets simultaneously over numerous technical and biological features, which gives valuable hints to distinguish technical artifact from biological variations.
scRNABatchQC supports multiple types of inputs, including gene-cell count matrices, 10x genomics, SingleCellExperiment or Seurat v3 objects.
□ ArtiFuse – Computational validation of fusion gene detection tools without relying on simulated reads
>> https://academic.oup.com/bioinformatics/advance-article-abstract/doi/10.1093/bioinformatics/btz613/5543101
As ArtiFuse affords total control over involved genes and breakpoint position, and assessed performance with regard to gene-related properties, showing a drop in recall value for low expressed genes in high coverage samples and genes with co-expressed paralogues.
ArtiFuse provides a more realistic benchmark that can be used to develop more accurate fusion gene prediction tools for application in clinical settings.

□ Factored LT and Factored Raptor Codes for Large-Scale Distributed Matrix Multiplication
>> https://arxiv.org/pdf/1907.11018v1.pdf
These coding schemes is based on LT codes and Raptor code, referred to as factored LT (FLT) codes, which is better in terms of numerical stability as well as decoding complexity when compared to Polynomial codes.
a Raptor code based scheme, referred to as factored Raptor (FR) codes, which performs well when K is moderately large. the decoding complexity of FLT codes is O(rtlogK), whereas the decoding complexity of Polynomial code is O(rt log2 K log log K).
□ Observability Analysis for Large-Scale Power Systems Using Factor Graphs
>> https://arxiv.org/pdf/1907.10338v1.pdf
a novel observability analysis approach based on the factor graphs and Gaussian belief propagation (BP) algorithm.
the Gaussian Belief Propagation (BP) - based algorithm is numerically robust, because it does not include direct factorization or inversion of matrices, thereby avoiding inaccurate computation of zero pivots and incorrect choice of a zero threshold.

□ Phase Transition Unbiased Estimation in High Dimensional Settings
>> https://arxiv.org/abs/1907.11541v1
A new estimator for the logistic regression model, with and without random effects, that also enjoy other properties such as robustness to data contamination and are also not affected by the problem of separability.
This estimator can be computed using a suitable simulation based algorithm, namely the iterative bootstrap, which is shown to converge exponentially fast.
□ Bootstrapping Networks with Latent Space Structure
>> https://arxiv.org/pdf/1907.10821v1.pdf
The first method generates bootstrap replicates of network statistics that can be represented as U-statistics in the latent positions, and avoids actually constructing new bootstrapped networks.
The second method generates bootstrap replicates of whole networks, and thus can be used for bootstrapping any network function.
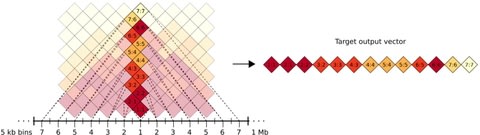
□ DeepC: Predicting chromatin interactions using megabase scaled deep neural networks and transfer learning.
>> https://www.biorxiv.org/content/biorxiv/early/2019/08/04/724005.full.pdf
DeepC integrates DNA sequence context on an unprecedented scale, bridging the different levels of resolution from base pairs to TADs.
DeepC is the first sequence based deep learning model that predicts chromatin interactions from DNA sequence within the context of the megabase scale.
□ CODC: A copula based model to identify differential coexpression
>> https://www.biorxiv.org/content/biorxiv/early/2019/08/05/725887.1.full.pdf
the proposed method performs well because of the popular scale-invariant property of copula.
The Copula is used to model the dependency between expression profiles of a gene pair.

□ A probabilistic multi-omics data matching method for detecting sample errors in integrative analysis
>> https://academic.oup.com/gigascience/article/8/7/giz080/5530324
a sample-mapping procedure called MODMatcher (Multi-Omics Data matcher), which is not only able to identify mis-matched omics profile pairs but also to properly map them to correct samples based on other omics data.
a robust probabilistic multi-omics data-matching procedure, proMODMatcher, to curate data and identify and unambiguously correct data annotation and metadata attribute errors in large databases.

□ The Linked Selection Signature of Rapid Adaptation in Temporal Genomic Data
>> https://www.biorxiv.org/content/biorxiv/early/2019/08/02/559419.full.pdf
Temporal autocovariance is caused by the persistence over generations of the statistical associations (linkage disequilibria) between a neutral allele and the fitnesses of the random genetic backgrounds it finds itself on;
as long as some fraction of associations persist, the heritable variation for fitness in one generation is predictive of the change in later generations, as illustrated by the fact that Cov(∆p2, ∆p0) > 0.
Ultimately segregation and recombination break down haplotypes and shuffle alleles among chromosomes, leading to the decay of autocovariance with time.

□ Construction of two-input logic gates using Transcriptional Interference
>> https://www.biorxiv.org/content/biorxiv/early/2019/08/05/724278.full.pdf
The presence of TI in naturally occurring systems has brought interest in the modeling and engineering of this regulatory phenomenon.
This work also highlights the ability of TI to control RNAP traffic to create and tune logic behaviors for synthetic biology while also exploring fundamental regulatory dynamics of RNAP-transcription factor and RNAP-RNAP interactions.

□ Supervised-learning is an accurate method for network-based gene classification
>> https://www.biorxiv.org/content/biorxiv/early/2019/08/05/721423.full.pdf
a comprehensive benchmarking of supervised-learning for network-based gene classification, evaluating this approach and a state-of-the-art label-propagation technique on hundreds of diverse prediction tasks and multiple networks using stringent evaluation schemes.
The supervised-learning on a gene’s full network connectivity outperforms label-propagation and achieves high prediction accuracy by efficiently capturing local network properties, rivaling label-propagation’s appeal for naturally using network topology.
□ ViSEAGO: Clustering biological functions using Gene Ontology and semantic similarity
>> https://biodatamining.biomedcentral.com/articles/10.1186/s13040-019-0204-1
Visualization, Semantic similarity and Enrichment Analysis of Gene Ontology (ViSEAGO) analysis of complex experimental design with multiple comparisons.
ViSEAGO captures functional similarity based on GO annotations by respecting the topology of GO terms in the GO graph.

□ A Vector Representation of DNA Sequences Using Locality Sensitive Hashing
>> https://www.biorxiv.org/content/biorxiv/early/2019/08/06/726729.full.pdf
The embedding dimension is usually between 100 and 1000. Every row of the embedding matrix is a vector representing a word so every word is represented as a point in the d dimensional space.
Experiments on metagenomic datasets with labels demonstrated that Locality Sensitive Hashing (LSH) can not only accelerate training time and reduce the memory requirements to store the model, but also achieve higher accuracy than alternative methods.
□ projectR: An R/Bioconductor package for transfer learning via PCA, NMF, correlation, and clustering
>> https://www.biorxiv.org/content/biorxiv/early/2019/08/06/726547.full.pdf
projectR uses transfer learning (TL), a sub-domain of machine learning, for in silico validation, interpretation, and exploration of these spaces using independent but related datasets.
once the robustness of biological signal is established, these Trancefer Learning approaches can be used for multimodal data integration.
□ Switchable Normalization for Learning-to-Normalize Deep Representation
>> https://ieeexplore.ieee.org/document/8781758
Switchable Normalization (SN), which learns to select different normalizers for different normalization layers of a deep neural network. SN employs three distinct scopes to compute statistics (means and variances) including a channel, a layer, and a minibatch.
SN outperforms its counterparts on various challenging benchmarks, such as ImageNet, COCO, CityScapes, ADE20K, MegaFace and Kinetics.

□ EdgeScaping: Mapping the spatial distribution of pairwise gene expression intensities
>> https://journals.plos.org/plosone/article?id=10.1371/journal.pone.0220279
Using the learned embedded feature space implemented a fast, efficient algorithm to cluster the entire space of gene expression relationships while retaining gene expression intensity.
EdgeScaping efficiency: A core issue of clustering more than 1.7 billion edges within realistic computational and time constraints was the requirement that the algorithm be able to efficiently and quickly create the model as well as cluster the edges.
□ GEDIT: The Gene Expression Deconvolution Interactive Tool: Accurate Cell Type Quantification from Gene Expression Data
>> https://www.biorxiv.org/content/biorxiv/early/2019/08/07/728493.full.pdf
GEDIT requires as input two matrices of expression values. The first is expression data collected from a tissue sample; each column represents one mixture, and each row corresponds to a gene.
The second matrix contains the reference data, with each column representing a purified reference profile and each row corresponds to a gene.

□ Sequence tube maps: making graph genomes intuitive to commuters
>> https://academic.oup.com/bioinformatics/advance-article/doi/10.1093/bioinformatics/btz597/5542397
a graph layout approach for genomic graphs that focuses on maximizing the linearity of selected genomic paths.
In the second pass the algorithm passes over each horizontal slot from left to right and lays out its content (the nodes and all sequence paths traversing this slot, whether within a node or not) vertically.

□ scAEspy: a unifying tool based on autoencoders for the analysis of single-cell RNA sequencing data
>> https://www.biorxiv.org/content/biorxiv/early/2019/08/07/727867.full.pdf
Non-linear approaches for dimensionality reduction can be effectively used to capture the non-linearities among the gene interactions that may exist in the high-dimensional expres- sion space of scRNA-Seq data.
scAEspy allows the integration of data generated using different scRNA-Seq platforms.
In order to combine and analyse multiple datasets generated by using different scRNA-Seq platforms, the GMMMDVAE followed by BBKKNN and coupled with the constrained Poisson loss is the best solution.

□ Tersect: a set theoretical utility for exploring sequence variant data
>> https://academic.oup.com/bioinformatics/advance-article-abstract/doi/10.1093/bioinformatics/btz634/5544926
Tersect is a lightweight, command-line utility for conducting fast set theoretical operations and genetic distance estimation on biological sequence variant data.
Per-sample presence or absence of specific variants of a chromosome is encoded in bit arrays using a variant of the Word-Aligned Hybrid (WAH) compression algorithm.
Tersect encodes the presence or absence of each variant in specific samples and are directly parallel to the per-chromosome variant lists.
□ Graphical models for zero-inflated single cell gene expression
>> https://projecteuclid.org/euclid.aoas/1560758430
To infer gene coregulatory networks, using a multivariate Hurdle model. It is comprised of a mixture of singular Gaussian distributions.
Estimation and sampling for multi-dimensional Hurdle models on a Normal density with applications to single-cell co-expression.
These are distributions that are conditionally Normal, but with singularities along the coordinate axes, so generalize a univariate zero-inflated distribution.
□ SGTK: Scaffold Graph ToolKit, a tool for construction and interactive visualization of scaffold graph
>> https://github.com/olga24912/SGTK
Scaffold graph is a graph where vertices are contigs, and edges represent links between them.
Contigs can provided either in FASTA format or as the assembly graph in GFA/GFA2/FASTG format. Possible linkage information sources are:
* paired reads
* long reads
* paired and unpaired RNA-seq reads
* scaffolds
* assembly graph in GFA1, GFA2, FASTG formats
* reference sequences
□ Scalable probabilistic PCA for large-scale genetic variation data
>> https://www.biorxiv.org/content/biorxiv/early/2019/08/08/729202.full.pdf
SVD computations can leverage fast matrix-vector multiplication operations to obtain computational eciency is well known in the numerical linear algebra literature.
ProPCA is a scalable method for PCA on genotype data that relies on performing inference in a probabilistic model. Inference in ProPCA model consists of an iterative procedure that uses a fast matrix-vector multiplication algorithm.
□ DISSEQT-DIStribution-based modeling of SEQuence space Time dynamics
>> https://academic.oup.com/ve/article/5/2/vez028/5543652
DISSEQT pipeline (DIStribution-based SEQuence space Time dynamics) for analyzing, visualizing, and predicting the evolution of heterogeneous biological populations in multidimensional genetic space, suited for population-based modeling of deep sequencing and high-throughput data.
DISSEQT pipeline is centered around robust dimension and model reduction algorithms for analysis of genotypic data with additional capabilities for including phenotypic features to explore dynamic genotype–phenotype maps.
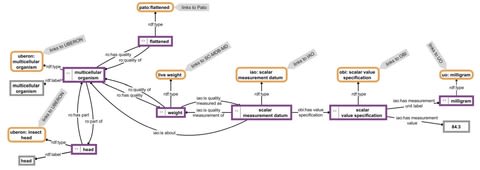
□ SOCCOMAS: a FAIR web content management system that uses knowledge graphs and that is based on semantic programming
>> https://academic.oup.com/database/article/doi/10.1093/database/baz067/5544589
Semantic Ontology-Controlled application for web Content Management Systems (SOCCOMAS), a development framework for FAIR (‘findable’, ‘accessible’, ‘interoperable’, ‘reusable’) Semantic Web Content Management Systems (S-WCMSs).
The source code of SOCCOMAS is written using the Semantic Programming Ontology (SPrO).
The provenance and versioning knowledge graph for a SOCCOMAS data document produced with semantic Morph·D·Base.
□ G3viz: an R package to interactively visualize genetic mutation data using a lollipop-diagram
>> https://academic.oup.com/bioinformatics/advance-article-abstract/doi/10.1093/bioinformatics/btz631/5545091

□ Using Machine Learning and Gene Nonhomology Features to Predict Gene Ontology
>> https://www.biorxiv.org/content/biorxiv/early/2019/08/09/730473.full.pdf
Non-homology-based functional annotation provides complementary strengths to homology-based annotation, with higher average performance in Biological Process GO terms,
the domain where homology-based functional annotation performs the worst, and weaker performance in Molecular Function GO terms, the domain where the accuracy of homology-based functional annotation is highest.
Non-homology-based functional annotation based on machine learning may ultimately prove useful both as a method to assign predicted functions to orphan genes, and to identify and correct functional annotation errors which were propagated through functional annotations.
□ MsPAC: A tool for haplotype-phased structural variant detection
>> https://academic.oup.com/bioinformatics/advance-article-abstract/doi/10.1093/bioinformatics/btz618/5545544
MsPAC, a tool that combines both technologies to partition reads, assemble haplotypes (via existing software), and convert assemblies into high-quality, phased SV predictions.
The output is a fasta file containing both haplotypes and VCF file with SVs.
MsPAC represents a framework for haplotype-resolved SV calls that moves one step closer to fully resolved.
□ SAPH-ire TFx: A Machine Learning Recommendation Method and Webtool for the Prediction of Functional Post-Translational Modifications
>> https://www.biorxiv.org/content/biorxiv/early/2019/08/09/731026.full.pdf
SAPH-ire TFx is optimized with both receiver operating characteristic (ROC) and recall metrics that maximally capture the range of diverse feature sets comprising the functional modified eukaryotic proteome.
SAPH-ire TFx – capable of predicting functional modification sites from large-scale datasets, and consequently focus experimental effort towards only those modifications that are likely to be biologically significant.
□ QS-Net: Reconstructing Phylogenetic Networks Based on Quartet and Sextet
>> https://www.frontiersin.org/articles/10.3389/fgene.2019.00607/full
QS-Net is a method generalizing Quartet-Net. the difficulty will be partially resolved with the development of high-speed computers and parallel algorithms.
Comparison with popular phylogenetic methods including Neighbor-Joining, Split-Decomposition and Neighbor-Net suggests that QS-Net is comparable with other methods in reconstructing tree-like evolutionary histories, while it outperforms them in reconstructing reticulate events.
QS-Net will be useful in identifying more complex reticulate events that will be ignored by other network reconstruction algorithms.
□ CrowdGO: a wisdom of the crowd-based Gene Ontology annotation tool
>> https://www.biorxiv.org/content/biorxiv/early/2019/08/10/731596.full.pdf
CrowdGO combines input predictions from any number of tools and combines them based on the Gene Ontology Directed Acyclic Graph. Using each GO terms information content, the semantic similarity between GO predictions of different tools, and a Support Vector Machine model.
※コメント投稿者のブログIDはブログ作成者のみに通知されます