
(Art by Nikita Kolbovskiy )

□ scPRINT: pre-training on 50 million cells allows robust gene network predictions
>> https://www.biorxiv.org/content/10.1101/2024.07.29.605556v1
sPRINT, a foundation model designed for gene network inference. scPRINT outputs cell type-specific genome-wide gene networks but also generates predictions on many related tasks, such as cell annotations, batch effect correction, and denoising, without fine-tuning.
scPRINT is trained with a novel weighted random sampling method3 over 40 million cells from the cellgene database from multiple species, diseases, and ethnicities, representing around 80 billion tokens.

□ biVI: Biophysical modeling with variational autoencoders for bimodal, single-cell RNA sequencing data
>> https://www.nature.com/articles/s41592-024-02365-9
biVI combines the variational autoencoder framework of scVI with biophysical models describing the transcription and splicing kinetics of RNA molecules. biVI successfully fits single-cell neuron data and suggests the biophysical basis for expression differences.
biVI retains the variational autoencoder’s ability to capture cell type structure in a low-dimensional space while further enabling genome-wide exploration of the biophysical mechanisms, such as system burst sizes and degradation rates, that underlie observations.
biVI consists of the three generative models (bursty, constitutive, and extrinsic) and scVI with negative binomial likelihoods. biVI models can be instantiated with single-layer linear decoders to directly link latent variables with gene mean parameters via layer weights.
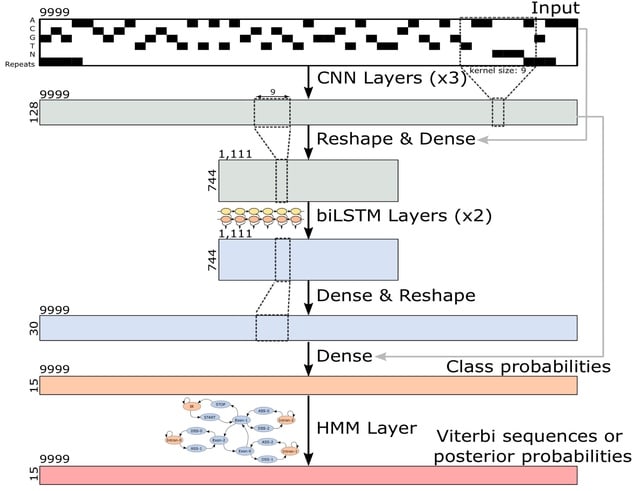
□ Tiberius: End-to-End Deep Learning with an HMM for Gene Prediction
>> https://www.biorxiv.org/content/10.1101/2024.07.21.604459v1
Tiberius, a novel deep learning-based ab initio gene structure prediction tool that end-to-end integrates convolutional and long short-term memory layers with a differentiable HMM layer. The HMM layer computes posterior probabilities or complete gene structures.
Tiberius employs a parallel variant of Viterbi, which can run in parallel on segments of a sequence. The Tiberius model has approximately eight million trainable parameters and it was trained with sequences of length T = 9999 and a length of T = 500,004 was used for inference.

□ WarpDemuX: Demultiplexing and barcode-specific adaptive sampling for nanopore direct RNA sequencing
>> https://www.biorxiv.org/content/10.1101/2024.07.22.604276v1
WarpDemuX, an ultra-fast and highly accurate adapter-barcoding and demultiplexing approach. WarpDemuX operates directly on the raw signal and does not require basecalling. It uses novel signal preprocessing and a fast machine learning algorithm for barcode classification.
WarpDemuX integrates a Dynamic Time Warping Distance (DTWD) kernel into a Support Vector Machine (SVM) classifier. This DTWD-based kernel function captures the essential spatial and temporal signal information by quantifying how similar an unknown barcode is to known patterns.

□ STORIES: Learning cell fate landscapes from spatial transcriptomics using Fused Gromov-Wasserstein
>> https://www.biorxiv.org/content/10.1101/2024.07.26.605241v1
STORIES (SpatioTemporal Omics eneRglES), a novel trajectory inference method capable of learning a causal model of cellular differentiation from spatial transcriptomics through time using Fused Gromov-Wasserstein (FGW).
STORIES learns a potential function that defines each cell's stage of differentiation. STORIES allows one to predict the evolution of cells at future time points. Indeed, STORIES learns a continuous model of differentiation, while Moscot uses FGW to connect adjacent time points.

□ MultiMIL: Multimodal weakly supervised learning to identify disease-specific changes in single-cell atlases
>> https://www.biorxiv.org/content/10.1101/2024.07.29.605625v1
Multi-MIL employs a multiomic data integration strategy using a product-of-expert generative model, providing a comprehensive multimodal representation of cells.
MultiMIL accepts paired or partially overlapping single-cell multimodal data across samples with varying phenotypes and consists of pairs of encoders and de-coders, where each pair corresponds to a modality.
Each encoder outputs a unimodal representation for each cell, and the joint cell representation is calculated from the unimodal representations. The joint latent representations are then fed into the decoders to reconstruct the input data.
Cells from the same sample are combined with the multiple-instance learning (MIL) attention pooling layer, where cell weights are learned with the attention mechanism, and the sample representations are calculated as a weighted sum of cell representations.

□ scCross: a deep generative model for unifying single-cell multi-omics with seamless integration, cross-modal generation, and in silico exploration
>> https://genomebiology.biomedcentral.com/articles/10.1186/s13059-024-03338-z
sCross employs modality-specific variational autoencoders to capture cell latent embeddings for each omics type. sCross leverages biological priors by integrating gene set matrices as additional features for each cell.
sCross harmonizes these enriched embeddings into shared embeddings z using further variational autoencoders and critically, bidirectional aligners. Bidirectional aligners are pivotal for the cross-modal generation.
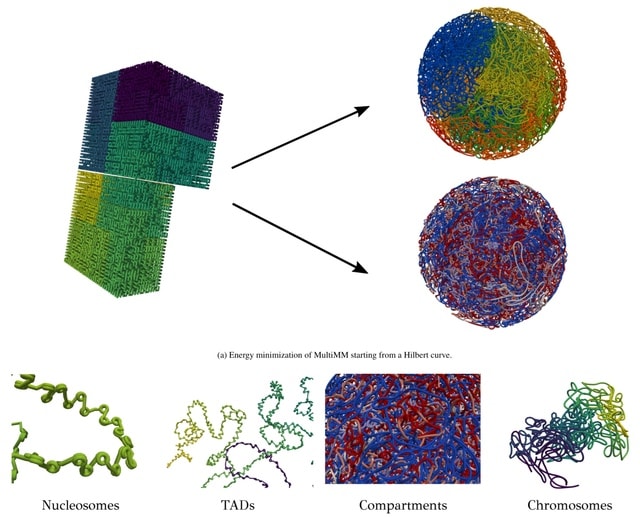
□ MultiMM: Multiscale Molecular Modelling of Chromatin: From Nucleosomes to the Whole Genome
>> https://www.biorxiv.org/content/10.1101/2024.07.26.605260v1
MultiMM (Multiscale Molecular Modelling) employs a multi-scale energy minimization strategy with a large choice of numerical integrators. MultiMM adapts the provided loop data to match the simulation's granularity, downgrading the data accordingly.
MultiMM consolidates loop strengths by summing those associated with the same loop after downgrading and retains only statistically significant ones, applying a threshold value. Loop strengths are then transformed to equilibrium distances.
MultiMM constructs a Hilbert curve structure. MultiMM employs a multi-scale molecular force-field. It encompasses strong harmonic bond and angle forces between adjacent beads, along with harmonic spring forces of variable strength to model the imported long-range loops.

□ GV-Rep: A Large-Scale Dataset for Genetic Variant Representation Learning
>> https://arxiv.org/abs/2407.16940
GV-Rep, a large-scale dataset of functionally annotated genomic variants (GVs), which could be used for deep learning models to learn meaningful genomic representations. GV-Rep aggregates data from seven leading public GV databases and a clinician-validated set.
The dataset organizes GV records into a standardized format, consisting of a (reference, alternative, annotation) triplet, and each record is tagged with a label that denotes attributes like pathogenicity, gene expression influence, or cell fitness impact.
These annotated records are utilized to fine-tune genomic foundation models (GFMs). These finetuned GMs generates meaningful vectorized representations, enabling the training of smaller models for classifying unknown GVs or for search and indexing within a vectorized space.

□ ChromBERT: Uncovering Chromatin State Motifs in the Human Genome Using a BERT-based Approach
>> https://www.biorxiv.org/content/10.1101/2024.07.25.605219v1
ChromBERT, a model specifically designed to detect distinctive patterns within chromatin state annotation data sequences. By adapting the BERT algorithm as utilized in DNABERT, They pretrained the model on the complete set of genic regions using 4-mer tokenization.
ChromBERT extends the concept fundamentally to the adaptation of chromatin state-annotated human genome sequences by combining it with Dynamic Time Warping.
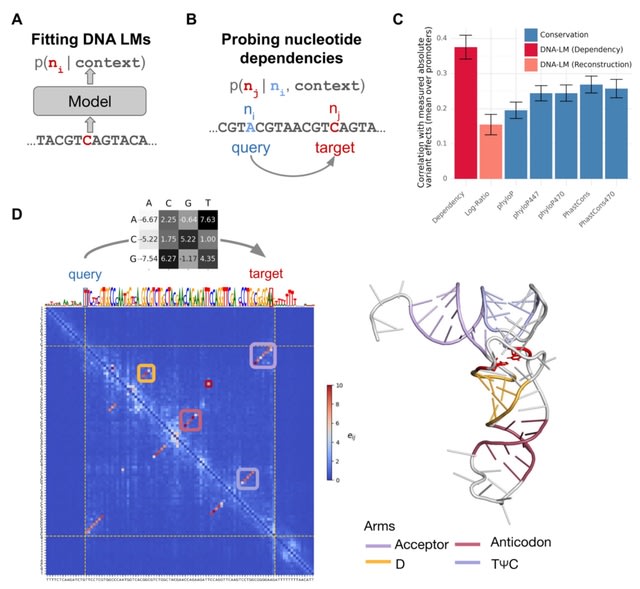
□ Nucleotide dependency analysis of DNA language models reveals genomic functional elements
>> https://www.biorxiv.org/content/10.1101/2024.07.27.605418v1
DNA language models are trained to reconstruct nucleotides, providing nucleotide probabilities given their surrounding sequence context. The probability of a particular nucleotide to be a guanine depends on whether it is intronic or located at the third base of a start codon.
Mutating a nucleotide in the sequence context (query nucleotide) into all three possible alternatives and record the change in predicted probabilities at a target nucleotide in terms of odds ratios.
This procedure, which can be repeated for all possible query-target combinations, quantifies the extent to which the language model prediction of the target nucleotide depends on the query nucleotide, all else equal.

□ The Genomic Code: The genome instantiates a generative model of the organism
>> https://arxiv.org/abs/2407.15908
The genome encodes a generative model of the organism. In this scheme, by analogy with variational autoencoders, the genome does not encode either organismal form or developmental processes directly, but comprises a compressed space of "latent variables".
These latent variables are the DNA sequences that specify the biochemical properties of encoded proteins and the relative affinities between trans-acting regulatory factors and their target sequence elements.
Collectively, these comprise a connectionist network, with weights that get encoded by the learning algorithm of evolution and decoded through the processes of development.
The latent variables collectively shape an energy landscape that constrains the self-organising processes of development so as to reliably produce a new individual of a certain type, providing a direct analogy to Waddington's famous epigenetic landscape.
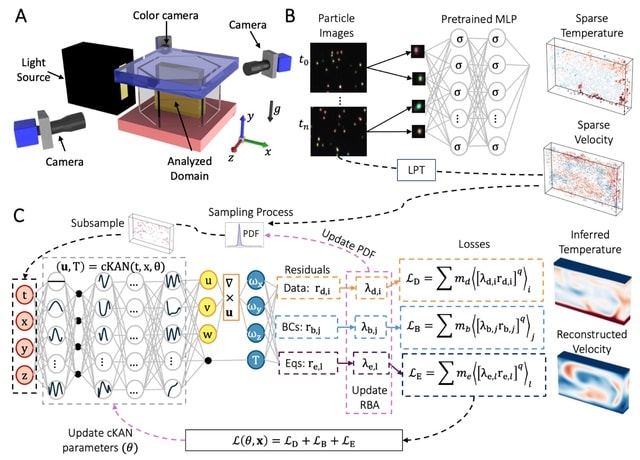
□ AIVT: Inferring turbulent velocity and temperature fields and their statistics from Lagrangian velocity measurements using physics-informed Kolmogorov-Arnold Networks
>> https://arxiv.org/abs/2407.15727
Artificial Intelligence Velocimetry-Thermometry (AIVT) method to infer hidden temperature fields from experimental turbulent velocity data. It enables us to infer continuous temperature fields using only sparse velocity data, hence eliminating the need for direct temperature measurements.
AIVT is based on physics-informed Kolmogorov-Arnold Networks (not neural networks) and is trained by optimizing a combined loss function that minimizes the residuals of the velocity data, boundary conditions, and the governing equations.
AIVT can be applied to a unique set of experimental volumetric and simultaneous temperature and velocity data of Rayleigh-Bénard convection (RBC) that we acquired by combining Particle Image Thermometry and Lagrangian Particle Tracking.

□ Stability Oracle: a structure-based graph-transformer framework for identifying stabilizing mutations
>> https://www.nature.com/articles/s41467-024-49780-2
Stability Oracle uses a graph-transformer architecture that treats atoms as tokens and utilizes their pairwise distances to inject a structural inductive bias into the attention mechanism. Stability Oracle also uses a data augmentation technique—thermodynamic permutations.
Stability Oracle consists of the local chemistry surrounding a residue w/ the residue deleted and two amino acid embeddings. Stability Oracle generates all possible point mutations from a single environment, circumventing the need for computationally generated mutant structures.

□ TEA-GCN: Constructing Ensemble Gene Functional Networks Capturing Tissue/condition-specific Co-expression from Unlabled Transcriptomic Data
>> https://www.biorxiv.org/content/10.1101/2024.07.22.604713v1
TEA-GCN (Two-Tier Ensemble Aggregation - GCN) leverages unsupervised partitioning of publicly derived transcriptomic data and utilizes three correlation coefficients to generate ensemble CGNs in a two-step aggregation process.
TEA-GCN uses of k-means clustering algorithm to divide gene expression data into partitions before gene co-expression determination. Expression data must be provided in the form of an expression matrix where expression abundances are in the form of Transcript per Million.

□ MultiOmicsAgent: Guided extreme gradient-boosted decision trees-based approaches for biomarker-candidate discovery in multi-omics data
>> https://www.biorxiv.org/cgi/content/short/2024.07.24.604727v1
MOAgent can directly handle molecular expression matrices - including proteomics, metabolomics, transcriptomics, as well as combinations thereof. The MOAgent-guided data analysis strategy is compatible with incomplete matrices and limited replicate studies.
The core functionality of MOAgent can be accessed via the "RFE++" section of the GUI. At its core, their selection algorithm has been implemented as a Monte-Carlo-like sampling of recursive feature elimination procedures.

□ LatentDAG: Representing core gene expression activity relationships using the latent structure implicit in bayesian networks
>> https://academic.oup.com/bioinformatics/advance-article/doi/10.1093/bioinformatics/btae463/7720781
LatentDAG, a Bayesian network can summarize the core relationships between gene expression activities. LatentDAG is substantially simpler than conventional co-expression network and ChiP-seq networks. It provides clearer clusters, without extraneous cross-cluster connections.
LatentDAG iterates all the genes in the network main component and selected the gene if the removal of the gene resulted in at least two separated components and each component having at least seven genes.

□ ASSMEOA: Adaptive Space Search-based Molecular Evolution Optimization Algorithm
>> https://academic.oup.com/bioinformatics/advance-article/doi/10.1093/bioinformatics/btae446/7718495
A strategy to construct a molecule-specific fragment search space to address the limited and inefficient exploration to chemical space.
Each molecule-specific fragment library are initially included the decomposition fragments of molecules with satisfactory properties in the database, and then are enlarged by adding the fragments from the new generated molecules with satisfactory properties in each iteration.
ASSMEOA is a molecule optimization algorithm to optimize molecules efficiently. They also propose a dynamic mutation strategy by replacing the fragments of a molecule with those in the molecule-specific fragment search space.


□ Gencube: Efficient retrieval, download, and unification of genomic data from leading biodiversity databases
>> https://www.biorxiv.org/content/10.1101/2024.07.18.604168v1
Gencube, a open-source command-line tool designed to streamline programmatic access to metadata and diverse types of genomic data from publicly accessible leading biodiversity repositories. gencube fetches metadata and Fasta format files for genome assemblies.
Gencube crossgenome fetches comparative genomics data, such as homology or codon / protein alignment of genes from different species. Gencube seqmeta generates a formal search query, retrieves the relevant metadata, and integrates it into experiment-level and study-level formats.

□ Pangene: Exploring gene content with pangene graphs
>> https://academic.oup.com/bioinformatics/advance-article/doi/10.1093/bioinformatics/btae456/7718494
Pangene takes a set of protein sequences and multiple genome assemblies as input, and outputs a graph in the GFA format. It aligns the set of protein sequences to each input assembly w/ miniprot, and derives a graph from the alignment with each contig encoded as a walk of genes.
Pangene provides utilities to classify genes into core genes that are present in most of the input genomes, or accessory genes. Pangene identifies generalized bubbles in the graph, which represent local gene order, gene copy-number or gene orientation variations.


□ QUILT2: Rapid and accurate genotype imputation from low coverage short read, long read, and cell free DNA sequence
>> https://www.biorxiv.org/content/10.1101/2024.07.18.604149v1
QUILT2, a novel scalable method for rapid phasing and imputation from 1c-WGS and fDNA using very large haplotype reference panels. QUILT2 uses a memory efficient version of the positional burrows wheeler transform (PBWT), which they call the multi-symbol PBWT (msPBWT).
QUILT2 uses msPBWT in the imputation process to find haplotypes in the haplotype reference panel that share long matches to imputed haplotypes with constant computational complexity, and with a very low memory footprint.
QUILT2 employs a two stage imputation process, where it first samples read labels and find an optimal subset of the haplotype reference panel using information at common SNPs, and then use these to initialize a final imputation at all SNPs.

□ MENTOR: Multiplex Embedding of Networks for Team-Based Omics Research
>> https://www.biorxiv.org/content/10.1101/2024.07.17.603821v1
MENTOR is a software extension to RWRtoolkit, which implements the random walk with restart (RWR) algorithm on multiplex networks. The RWR algorithm traverses a random walker across a monoplex / multiplex network using a single node, called the seed, as an initial starting point.
As an abstraction of the edge density of these networks, a topological distance matrix is created and hierarchical clustering used to create a dendrogram representation of the functional interactions. MENTOR can determine the topological relationships among all genes in the set.

□ SGS: Empowering Integrative and Collaborative Exploration of Single-Cell and Spatial Multimodal Data
>> https://www.biorxiv.org/content/10.1101/2024.07.19.604227v1
SGS offer two modules: SC (single-cell and spatial visualization module) and SG (single-cell and genomics visualization module), w/ adaptable interface layouts and advanced capabilities.
Notably, the SG module incorporates a novel genome browser framework that significantly enhances the visualization of epigenomic modalities, including SCATAC, scMethylC, sc-eQTL, and scHiC etc.

□ Pseudovisium: Rapid and memory-efficient analysis and quality control of large spatial transcriptomics datasets
>> https://www.biorxiv.org/content/10.1101/2024.07.23.604776v1
Pseudovisium, a Python-based framework designed to facilitate the rapid and memory-efficient analysis, quality control and interoperability of high-resolution spatial transcriptomics data. This is achieved by mimicking the structure of 10x Visium through hexagonal binning of transcripts.
Pseudovisium increased data processing speed and reduced dataset size by more than an order of magnitude. At the same time, it preserved key biological signatures, such as spatially variable genes, enriched gene sets, cell populations, and gene-gene correlations.

□ SAVANA: reliable analysis of somatic structural variants and copy number aberrations in clinical samples using long-read sequencing
>> https://www.biorxiv.org/content/10.1101/2024.07.25.604944v1
SAVANA is a somatic SV caller for long-read data. It takes aligned tumour and normal BAM files, examines the reads for evidence of SVs, clusters adjacent potential SVs together, and finally calls consensus breakpoints, classifies somatic events, and outputs them in BEDPE and VCF.
SAVANA also identifies copy number abberations and predicts purity and ploidy. SAVANA provides functionalities to assign sequencing reads supporting each breakpoint to haplotype blocks when the input sequencing reads are phased.
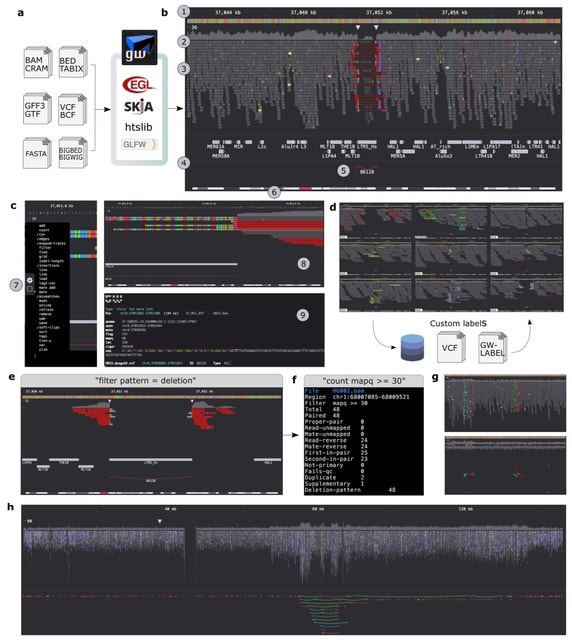
□ GW: ultra-fast chromosome-scale visualisation of genomics data
>> https://www.biorxiv.org/content/10.1101/2024.07.26.605272v1
Genome-Wide (GW) is an interactive genome browser that expedites analysis of aligned sequencing reads and data tracks, and introduces novel interfaces for exploring, annotating and quantifying data.
GW's high-performance design enables rapid rendering of data at speeds approaching the file reading rate, in addition to removing the memory constraints of visualizing large regions. GW explores massive genomic regions or chromosomes without requiring additional processing.
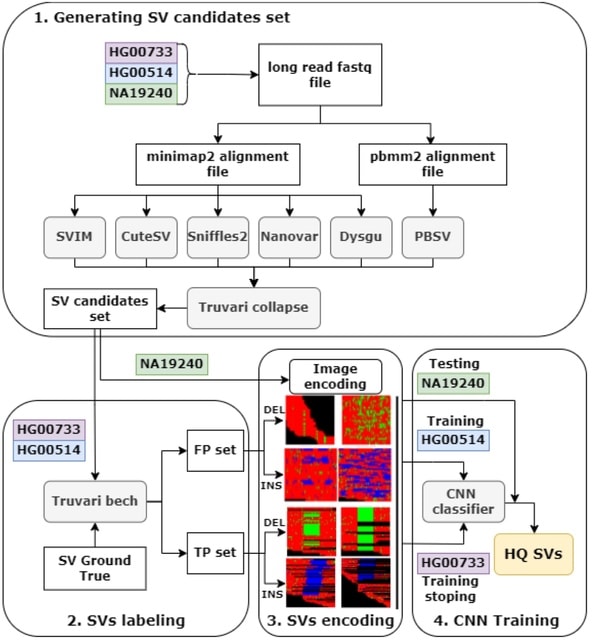
□ ConsensuSV-ONT - a modern method for accurate structural variant calling
>> https://www.biorxiv.org/content/10.1101/2024.07.26.605267v1
ConsensuSV-ONT, a novel meta-caller algorithm, along with a fully automated variant detection pipeline and a high-quality variant filtering algorithm based on variant encoding for images and convolutional neural network models.
ConsensuSV-ONT-core, is used for getting the consensus (by CNN model) out of the already-called SVs, taking as an input vof files, and returns a high-quality vof file. ConsensuSV-ONT-pipeline is the complete out-of-the-box solution using as the input raw ONT fast files.

□ A fast and simple approach to k-mer decomposition
>> https://www.biorxiv.org/content/10.1101/2024.07.26.605312v1
An intuitive integer representation of a k-mer, which at the same time acts as minimal perfect hash. This is accompanied by a minimal perfect hash function (MPHF) that decomposes a sequence into these hash values in constant time with respect to k.
It provides a simple way to give these k-mer hashes a pseudorandom ordering, a desirable property for certain k-mer based methods, such as minimizers and syncmers.

□ SCCNAInfer: a robust and accurate tool to infer the absolute copy number on scDNA-seq data
>> https://academic.oup.com/bioinformatics/advance-article/doi/10.1093/bioinformatics/btae454/7721932
SCCNAInfer calculates the pairwise distance among cells, and clusters the cells by a novel and sophisticated cell clustering algorithm that optimizes the selection of the cell cluster number.
SCCNAInfer automatically searches the optimal subclonal ploidy that minimizes an objective function that not only incorporates the integer copy number approximation algorithm, but also considers the intra-cluster distance and those in two different clusters.

□ scASfind: Mining alternative splicing patterns in scRNA-seq data
>> https://genomebiology.biomedcentral.com/articles/10.1186/s13059-024-03323-6
scASfind uses a similar data compression strategy as scfind to transform the cell pool-to-node differential PSI matrix into an index. This enables rapid access to cell type-specific splicing events and allows an exhaustive approach for pattern searches across the entire dataset.
scASfind does not involve any imputation or model fitting, instead cells are pooled to avoid the challenges presented by sparse coverage. Moreover, there is no restriction on the number of exons, or the inclusion/exclusion events involved in the pattern of interest.

□ HAVAC: An FPGA-based hardware accelerator supporting sensitive sequence homology filtering with profile hidden Markov models
>> https://bmcbioinformatics.biomedcentral.com/articles/10.1186/s12859-024-05879-3
HAVAC (The Hardware Accelerated single-segment Viterbi Additional Coprocessor), an FPGA-accellerated implementation of the Single-segment Ungapped Viterbi algorithm for use in nucleotide sequence with profile hidden Markov models.
HAVAC concatenates all sequences in a fasta file and all models in an hmm file before transferring the data to the accelerator for processing. The HAVAC kernel represents a 227× matrix calculation speedup over nhmmer with one thread and a 92× speedup over nhmmer with 4 threads.
※コメント投稿者のブログIDはブログ作成者のみに通知されます