
"I remember lying out in my bed and looking at the vast, quiet sky. Up above my head, there were three stars in a row, & I remember thinking, 'Well, I'll have those three stars all my life, & wherever I am, they will be. They are my stars, and they belong to me" - Spike Milligan

□ HiCAGE: an R package for large-scale annotation and visualization of 3C-based genomic data:
>> https://www.biorxiv.org/content/biorxiv/early/2018/05/05/315234.full.pdf
HiCAGE provides 3C-based data integrated with gene expression analysis, as well as graphical summaries of integrations and gene-ontology enrichment of candidate genes based on proximity. Additionally, HiCAGE will increase our understanding of the functional consequences of changes to the nuclear architecture by linking gene expression with chromatin state interactions.
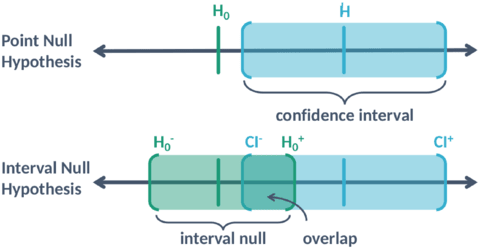
□ Second-generation p-values: Improved rigor, reproducibility, & transparency in statistical analyses
>> http://journals.plos.org/plosone/article?id=10.1371/journal.pone.0188299
The second-generation p-value is the proportion of data-supported hypotheses that are also null hypotheses, and indicate when the data are compatible with null hypotheses (pδ = 1), or with alternative hypotheses (pδ = 0), or when the data are inconclusive (0

□ On the networked architecture of genotype spaces and its critical effects on molecular evolution:
>> https://arxiv.org/pdf/1804.06835.pdf
when the complex architecture of genotype spaces is taken into account, the evolutionary dynamics of molecular populations becomes intrinsically non-uniform, sharing deep qualitative and quantitative similarities with slowly driven physical systems. Furthermore, the phenotypic plasticity inherent to genotypes transforms classical fitness landscapes into multiscapes where adaptation in response to an environmental change may be very fast. building a mesoscopic description in which phenotypes, rather than genotypes, are the basic elements of our dynamical framework, and in which microscopic details are subsumed in an effective, possibly non-Markovian stochastic dynamics.
□ Machine Learning’s ‘Amazing’ Ability to Predict Chaos
>> https://www.quantamagazine.org/machine-learnings-amazing-ability-to-predict-chaos-20180418/
the flamelike system would continue to evolve out to eight “Lyapunov times”. The Lyapunov time represents how long it takes for two almost-identical states of a chaotic system to exponentially diverge. it typically sets the horizon of predictability. “In order to have this exponential divergence of trajectories you need this stretching, and in order not to run away to infinity you need some folding.” The stretching and compressing in the different dimensions correspond to a system’s positive and negative “Lyapunov exponents,” respectively.
□ Using machine learning to replicate chaotic attractors and calculate Lyapunov exponents from data:
>> https://aip.scitation.org/doi/pdf/10.1063/1.5010300
a limited time series of measurements as input to a high-dimensional dynamical system called a “reservoir.” After the reservoir's response to the data is recorded, linear regression is used to learn a large set of parameters, called the “output weights.” The learned output weights are then used to form a modified autonomous reservoir designed to be capable of producing an arbitrarily long time series whose ergodic properties approximate those of the input signal. Since the reservoir equations and output weights are known, we can compute the derivatives needed to determine the Lyapunov exponents of the autonomous reservoir, which we then use as estimates of the Lyapunov exponents for the original input generating system.

□ Hybrid Forecasting of Chaotic Processes: Using Machine Learning in Conjunction with a Knowledge-Based Model:
>> https://arxiv.org/pdf/1803.04779.pdf
Both the hybrid scheme and the reservoir-only model have the property of “training reusability", make any number of subsequent predictions by preceding each prediction with a short run in the configuration to resynchronize the reservoir dynamics with the dynamics to be predicted. A particularly dramatic example illustrating the effectiveness of the hybrid approach in which, when acting alone, both the knowledge-based predictor & the reservoir machine learning predictor give fairly worthless results (prediction time of only a fraction of a Lyapunov time). when the same two systems are combined in the hybrid scheme, good predictions are obtained for a substantial duration of about 4 Lyapunov times.
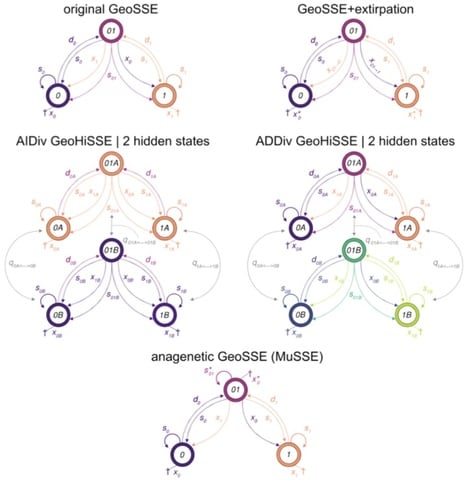
□ Hidden state models improve the adequacy of state-dependent diversification approaches using empirical trees, including biogeographical models:
>> https://www.biorxiv.org/content/biorxiv/early/2018/04/17/302729.full.pdf
HMM models in combination with a model-averaging approach naturally account for hidden traits when examining the meaningful impact of a suspected "driver" of diversification. they demonstrate the role of hidden state models as a general framework by expanding the original geographic state speciation and extinction model (GeoSSE).
For instance, when an asteroid impact throws up a dust cloud, or causes a catastrophic fire, every lineage alive at that time is affected simultaneously.Their ability to survive may come from heritable factors, but the sudden shift in diversification caused by an exogenous event like this appears suddenly across the tree, in a manner not yet incorporated in these models. Similarly, a secular trend affecting all species is not part of this model, but is in others, and they also do not incorporate factors like a species “memory” of time since last speciation, or even a global carrying capacity for a clade that affects the diversification rate. Most of these caveats are not limited to HMM models of diversification, but this reminder may serve to reduce overconfidence in results.

□ Cox-nnet: An artificial neural network method for prognosis prediction of high-throughput omics data:
>> http://journals.plos.org/ploscompbiol/article?id=10.1371/journal.pcbi.1006076
In 10 TCGA RNA-Seq data sets, Cox-nnet achieves the same or better predictive accuracy compared to other methods, including Cox-proportional hazards regression (with LASSO, ridge, and mimimax concave penalty), Random Forests Survival and CoxBoost. The outputs from the hidden layer node provide an alternative approach for survival-sensitive dimension reduction. it is possible to embed a priori biological pathway information into the architecture, by connecting genes in a pathway to a common node in the next hidden layer.

□ Tigmint: Correcting Assembly Errors Using Linked Reads From Large Molecules:
>> https://www.biorxiv.org/content/biorxiv/early/2018/04/20/304253.full.pdf
Tigmint identifies and corrects misassemblies using linked reads from 10x Genomics Chromium. The physical coverage of the large molecules is more consistent and less prone to coverage dropouts than that of the short read sequencing data. Each scaffold is scanned with a fixed window to identify areas where there are few spanning molecules, revealing possible misassemblies. Correcting the ABySS assembly of the human data set HG004 with Tigmint reduces the number of misassemblies identified by QUAST by 216, a reduction of 27%. The last assembly on the Pareto frontier is DISCOVARdenovo + BESST + Tigmint + ARCS, which strikes a good balance between both good contiguity and few misassemblies.
□ fast5seek
>> https://github.com/mbhall88/fast5seek
This program takes a directory (or multiple) of fast5 files along with any number of fastq, SAM, or BAM files. The output is the full paths for all fast5 files present in the fastq, BAM, or SAM files that are also in the provided fast5 directory(s). Sometimes there are additional characters in the fast5 names added on by albacore or MinKnow. They have variable length, so this attempts to clean the name to match what is stored by the fast5 files.
□ Clive_G_Brown:
MinKNOW 2.0 is coming ...
□ morgantaschuk:
Today's task is deleting 1TB of PhiX sequencing data from @illumina NovaSeq validation runs.
That's 3.479886103×10¹² bases
PhiX genome is 5386 bases long
So we theoretically sequenced 646098422 viral particles
The viral capsid is 250 Å wide
we sequenced 16 metres of PhiX
□ LCA robustly reveals subtle diversity in large-scale single-cell RNA-seq data:
>> https://www.biorxiv.org/content/biorxiv/early/2018/04/20/305581.full.pdf
Latent Cellular Analysis (LCA), a machine learning-based analytical pipeline that features a dual-space model search with inference of latent cellular states, control of technical variations, cosine similarity measurement, and spectral clustering. LCA provides mathematical formulae with which to project the remaining cells (testing cells) directly to the inferred low-dimensional LC space, after which individual cells are assigned to the subpopulation with the best similarity.
□ gmxapi: a high-level interface for advanced control and extension of molecular dynamics simulations:
>> https://www.biorxiv.org/content/biorxiv/early/2018/04/22/306043.full.pdf
This approach, originally published and implemented using CHARMM, is a common workflow in our group using GROMACS that requires custom code in three places: user-specified biasing forces in the core MD engine, analysis code to process predicted ensemble data and update the biasing forces, and parallelization scripts to manage execution, analysis, and data exchange between many ensemble members simultaneously.
□ Global Biobank Engine: enabling genotype-phenotype browsing for biobank summary statistics:
>> https://www.biorxiv.org/content/biorxiv/early/2018/04/22/304188.full.pdf
Genetic correlations have been estimated by applying the multivariate polygenic mixture model (MVPMM) to GWAS summary statistics for more than one million pairs of traits and can be visualized using the app. Users can filter the phenotypes and results that are displayed by the app.

□ Genomic SEM Provides Insights into the Multivariate Genetic Architecture of Complex Traits:
>> https://www.biorxiv.org/content/biorxiv/early/2018/04/21/305029.full.pdf
genomic structural equation modeling (Genomic SEM) can be used to identify variants with effects on general dimensions of cross-trait liability, boost power for discovery, and calculate more predictive polygenic scores. Genomic SEM is a Two-Stage Structural Equation Modeling approach. In Stage 1, the empirical genetic covariance matrix and its associated sampling covariance matrix are estimated. The diagonal elements of the sampling covariance matrix are squared standard errors.
□ Rethomics: an R framework to analyse high-throughput behavioural data:
>> https://www.biorxiv.org/content/biorxiv/early/2018/04/21/305664.full.pdf
At the core of rethomics lies the behavr table, a structure used to store large amounts of data (e.g. position and activity) and metadata (e.g. treatment and genotype) in a unique data.table-derived object. The metadata holds a single row for each of the n individuals. Its columns, the p metavariables, are one of two kinds: either required – and defined by the acquisition platform – or user-defined.
□ NanoDJ: a Jupyter notebook integration of tools for simplified manipulation and assembly of DNA sequences produced by ONT devices.
>> https://github.com/genomicsITER/NanoDJ
NanoDJ integrates basecalling, read trimming and quality control, simulation and plotting routines with a variety of widely used aligners and assemblers, including procedures for hybrid assembly.

□ Balancing Non-Equilibrium Driving with Nucleotide Selectivity at Kinetic Checkpoints in Polymerase Fidelity Control:
>> http://www.mdpi.com/1099-4300/20/4/306
the individual transitions serving as selection checkpoints need to proceed at moderate rates in order to sustain the necessary non-equilibrium drives as well as to allow nucleotide selections for an optimal error control. The accelerations on the backward transitions show similar but opposite trends: the accelerations lead to close-to-equilibrium with low speeds and high error rates; slowing down the transitions promotes far-from-equilibrium with high speeds and low error rates, except for the selection checkpoint at which the error rate rises for the too slow backward transition.

□ Generalised free energy and active inference: can the future cause the past?
>> https://www.biorxiv.org/content/biorxiv/early/2018/04/23/304782.full.pdf
Formally, the ensuing generalised free energy is a Hamiltonian Action, because it is a path or time integral of free energy at each time point. In other words, active inference is just a statement of Hamilton's Principle of Stationary Action. Generalised free energy minimisation replicates the epistemic and reward seeking behaviours induced in earlier active inference schemes, but prior preferences now induce an optimistic distortion of belief trajectories into the future. This allows beliefs about outcomes in the distal future to influence beliefs about states in the proximal future and present. That these beliefs then drive policy selection suggests that, under the generalised free energy formulation, the future can indeed cause the past. A prior belief about an outcome at a particular time point thus distorts the trajectory of hidden states at each time point reaching back to the present.
□ NiDelta: De novo protein structure prediction using ultra-fast molecular dynamics simulation:
>> https://www.biorxiv.org/content/biorxiv/early/2018/04/23/262188.full.pdf
NiDelta is built on a deep convolutional neural network and statistical potential enabling molecular dynamics simulation for modeling protein tertiary structure. Statistically determined residue-contacts from the MSAs and torsion angles (φ, ψ) predicted by deep learning method provide valuable structural constraints for the ultra-fast MD simulation (Upside).
□ GOcats: A tool for categorizing Gene Ontology into subgraphs of user-defined concepts:
>> https://www.biorxiv.org/content/biorxiv/early/2018/04/24/306936.full.pdf
discrepancies in the semantic granularity of gene annotations in knowledgebases represent a significant hurdle to overcome for researchers interested in mining genes based on a set of annotations used in experimental data. the topological distance between two terms in the ontology graph is not necessarily proportional to the semantic closeness in meaning between those terms, and semantic similarity reconciles potential inconsistencies between semantic closeness and graph distance.
□ HMMRATAC, The Hidden Markov ModeleR for ATAC-seq:
>> https://www.biorxiv.org/content/biorxiv/early/2018/04/24/306621.full.pdf
HMMRATAC splits a single ATAC-seq dataset into nucleosome- free and nucleosome-enriched signals, learns the unique chromatin structure around accessible regions, and then predicts accessible regions across the entire genome.

□ Discovery of Large Disjoint Motif in Biological Network using Dynamic Expansion Tree:
>> https://www.biorxiv.org/content/biorxiv/early/2018/04/25/308254.full.pdf
The dynamic expansion tree used in this algorithm is truncated when the frequency of the subgraph fails to cross the predefined threshold. This pruning criterion in DET reduces the space complexity significantly.

□ Identifying high-priority proteins across the human diseasome using semantic similarity:
>> https://www.biorxiv.org/content/biorxiv/early/2018/04/29/309203.full.pdf
a systematic collection of popular proteins across 10,129 human diseases as defined by the Disease Ontology, 10,642 disease phenotypes defined by Human Phenotype Ontology, and 2,370 cellular pathways defined by Pathway Ontology. This strategy allows instant retrieval of popular proteins across the human ”diseasome”, and further allows reverse queries from protein to disease, enabling functional analysis of experimental protein lists using bibliometric annotations.
□ Semantic Disease Gene Embeddings (SmuDGE): phenotype-based disease gene prioritization without phenotypes:
>> https://www.biorxiv.org/content/biorxiv/early/2018/04/30/311449.full.pdf
SmuDGE, a method that uses feature learning to generate vector-based representations of phenotypes associated with an entity. SmuDGE can match or outperform semantic similarity in phenotype-based disease gene prioritization, and furthermore significantly extends the coverage of phenotype-based methods to all genes in a connected interaction network.
※コメント投稿者のブログIDはブログ作成者のみに通知されます