
□ Goldmund / "Occasus"
♪ Terrarium

□ New Top Expressed Genes visualization shows how top genes in one tissue are expressed in other tissues.
>> https://bit.ly/2q4pxuV

□ Clairvoyante: a multi-task convolutional deep neural network for variant calling in Single Molecule Sequencing:
>> https://www.biorxiv.org/content/biorxiv/early/2018/04/28/310458.full.pdf
Clairvoyante is the first method for Single Molecule Sequencing to finish a whole genome variant calling in two hours on a 28 CPU-core machine, with top-tier accuracy and sensitivity. Future improvements to the base-calling algorithm or sequencing chemistry will lead to raw reads with higher accuracy at these troublesome genome regions and hence, further decrease the number of known variants undetected by Clairvoyante.
□ Skyhawk: An Artificial Neural Network-based discriminator for reviewing clinically significant genomic variants:
>> https://www.biorxiv.org/content/biorxiv/early/2018/05/01/311985.full.pdf
The multi-task ANN was designed for variant calling in Single Molecule Sequencing and the method is orthogonal to traditional variant callers using algorithms such as Bayesian or local-assembly. Skyhawk mimics how a human visually identifies genomic features comprising a variant and decides whether the evidence supports or contradicts the sequencing read alignments using the network architecture they developed in a previous study named "Clairvoyante".

□ Black-boxing and cause-effect power: causal properties of physical systems across different spatiotemporal scales.
>> http://journals.plos.org/ploscompbiol/article?id=10.1371/journal.pcbi.1006114
while local maxima reveal cause-effect properties to an investigator studying the system, the global maximum specifies the set of elements and spatiotemporal grain at which the system has most cause-effect power upon itself–from its own intrinsic perspective. According to integrated information theory, a set of elements at the spatial-temporal grain that defines the global maximum of intrinsic cause-effect power corresponds to a physical substrate of consciousness.
□ Machine learning quantum phases of matter beyond the fermion sign problem:
Using Deep Learning to Navigate Chaos in Many-Body Problems:
>> https://www.nature.com/articles/s41598-017-09098-0
Using auxiliary-field quantum Monte Carlo (QMC) simulations to sample the many-fermion system, the Green’s function holds sufficient information to allow for the distinction of different fermionic phases. QMC + machine learning approach works even for systems exhibiting a severe fermion sign problem where conventional approaches to extract information from the Green’s function, e.g. in the form of equal-time correlation functions, fail.
□ Haplotype-aware genotyping from noisy long reads:
>> https://www.biorxiv.org/content/biorxiv/early/2018/04/03/293944.full.pdf
formalize the computational problem in terms of a Hidden Markov Model and compute posterior genotype probabilities using the forward-backward algorithm. Genotype predictions can then be made by picking the most likely genotype at each site. Computing genotypes based on bipartitions of reads that represent possible haplotypes of the individual helps to reduce the number of genotyping errors, since it makes it easier to detect sequencing errors in the given reads.
□ bioLQM: a java library for the manipulation and conversion of Logical Qualitative Models of biological networks:
>> https://www.biorxiv.org/content/biorxiv/early/2018/04/05/287011.full.pdf
a multi-valued component with a maximal activity level m is replaced by m Boolean components, each denoting increasing activity. All possible states of the original model can then be associated to states of the Boolean model. The logical rules of the new model ensure that we obtain the same transitions between these states. Ongoing discussions should lead to extensions of the SED-ML format and the Kinetic Simulation Algorithm Ontology to describe model modifications and simulation parameters.
□ Multi-configuration time-dependent Hartree method for Efficient grid-based quantum dynamics without pre-computed potential energy surfaces:
>> https://aip.scitation.org/doi/10.1063/1.5024869
The simulations of proton transfer in salicylaldimine and non-adiabatic dynamics in pyrazine show that their method can be used to efficiently model quantum dynamics in many-dimensional systems. A database of energies was created on-the-fly during propagation, and the SVD procedure, described above, was used to generate the potential energy operator for the dynamics from the kernel ridge regression fit using the additive kernel.

□ DeFine: Deep learning based Functional impact of non-coding variants evaluator for accurately quantify intensities of transcription factor-DNA binding and facilitate evaluation of functional non-coding variants:
>> https://academic.oup.com/nar/advance-article/doi/10.1093/nar/gky215/4958204
DeFine provides a framework for constructing cell type-specific deep learning models to assess the functional impact of abundant non-coding variants across the whole human genome. DeFine integrates cell type specific three-dimensional genome contact maps.
□ Learning and Forgetting Using Reinforced Bayesian Change Detection:
>> https://www.biorxiv.org/content/biorxiv/early/2018/04/06/294959.full.pdf
This rich model encompasses many aspects of Reinforcement Learning (RL), such as Temporal Difference RL and counterfactual learning, and accounts for the reduced computational cost of automatic behaviour. Hierarchical Adaptive Forgetting Variational Filter (HAFVF) that achieves adaptive change detection by the use of Stabilized Forgetting, updating its current belief based on a mixture of fixed, initial priors and previous posterior beliefs.
□ Phase Transitions of the Moran Process and Algorithmic Consequences:
>> https://arxiv.org/pdf/1804.02293.pdf
The Moran process is a randomised algorithm that models the spread of genetic mutations through graphs. If the graph is connected, the process eventually reaches "fixation", where every vertex is a mutant, or "extinction", where no vertex is a mutant. For all epsilon > 0, the expected running time on an n-vertex graph is o(n^(3+epsilon)). In fact, it is at most n^3 * exp(O((log log n)^3)) and that there is a family of graphs where it is Omega(n^3).

□ Chiron: Translating nanopore raw signal directly into nucleotide sequence using deep learning:
>> https://academic.oup.com/gigascience/advance-article/doi/10.1093/gigascience/giy037/4966989
Chiron has a novel architecture which couples a convolutional neural network with an RNN and a Connectionist Temporal Classification decoder. This enables it to model the raw signal data directly, without use of an event segmentation step. The convolutional layers discriminate local patterns in the raw input signal, whereas the recurrent layers integrate these patterns into basecall probabilities. At the top of the NN is a CTC decoder to provide the final DNA sequence according to the base probabilities.
□ Pushing the limits of de novo genome assembly for complex prokaryotic genomes harboring very long, near identical repeats:
>> https://www.biorxiv.org/content/biorxiv/early/2018/04/12/300186.full.pdf
Only by using very long reads from the ONT platform (mean read length 44 kb) was it possible to fully resolve this genome, indicating the presence of dark matter of genome assembly also in prokaryotes. Base called ONT reads were filtered (30 kb or longer), and assembled with Flye v.2.3 using standard parameters and performing three polishing iterations on Flye’s polishing stage (-i 3 --nano-raw [ont_reads.fastq] -g 7.7m).
□ Analysis on the Nonlinear Dynamics of Deep Neural Networks: Topological Entropy and Chaos:
>> https://arxiv.org/pdf/1804.03987.pdf
DNN is considered as a discrete-time dynamical system due to its layered structure. The complexity provided by the nonlinearity in the dynamics is analyzed in terms of topological entropy and chaos characterized by Lyapunov exponents. if the nonlinear dynamics provides too much complexity, the Vapnik-Chervonenkis (VC) dimension could be high and thus affect the generalization capability. there may exist chaotic behavior due to the nonlinearity, which explains why DNN can form complicated classification surfaces. we will calculate the topological entropy analytically in the high dimensional regime or numerically using the generating function of symbolic dynamics.

□ GNE: A deep learning framework for gene network inference by aggregating biological information:
>> https://www.biorxiv.org/content/biorxiv/early/2018/04/13/300996.full.pdf
The low-dimensional vector representation of the gene topological characteristics, which derive deeper insights into the structure of rapidly accumulating and diverse gene interaction networks, greatly simplify downstream modeling and ontology reconstruction.
□ Single cell RNA-seq denoising using a deep count autoencoder:
>> https://www.biorxiv.org/content/biorxiv/early/2018/04/13/300681.full.pdf
a deep count autoencoder network (DCA) takes the count distribution, overdispersion and sparsity of the data into account using a zero-inflated negative binomial noise model, and nonlinear gene-gene or gene-dispersion interactions are captured.
□ Tensorial Blind Source Separation for Improved Analysis of Multi-Omic Data:
>> https://www.biorxiv.org/content/biorxiv/early/2018/04/13/300277.full.pdf
For instance, one solution may be to first perform dimensional reduction using supervised feature selection on separate data-types, and subsequently applying the tensorial methods on a reduced feature space. "For Canonical Correlation Analysis (CCA) and sCCA, the maximum number of canonical vectors to search for was set to be equal to the RMT estimate of the concatanated matrix, i.e. equal to the dimension of joint variation."

□ DragoNN/DeepLIFT: Decoding non-coding regulatory elements and disease-associated variants with deep learning:
>> https://drive.google.com/file/d/1Uk9i2TykKOrQUO0HCy-_MrdVswQkKuky/view
The dragonn package implements Deep RegulAtory GenOmic Neural Networks (DragoNNs) for predictive modeling of regulatory genomics, nucleotide-resolution feature discovery, and simulations for systematic development and benchmarking.
□ emeraLD: Rapid Linkage Disequilibrium Estimation with Massive Data Sets:
>> https://www.biorxiv.org/content/biorxiv/early/2018/04/15/301366.full.pdf
In data sets with 10Ks of samples, emeraLD is 5-20x faster than PLINK-1.9 and 100s-Ks of times faster than existing tools for VCF files. For genotype formats, calculate the dot product using sparse-by-dense and sparse-by-sparse vector products. Using haplotype block format (M3VCF), calculate the dot product using within-block and between-block haplotype intersections.
□ Droplet scRNA-seq is not zero inflated
>> http://www.nxn.se/valent/2017/11/16/droplet-scrna-seq-is-not-zero-inflated
In high throughput variants of scRNA-seq assays cells are isolated in (reverse) droplets, within which several molecular reactions occur to eventually give rise to labeled cDNA from expressed genes from each cell. Part of what makes this possible is limiting the sequenced fragments to single tags from the 3' or 5' end of each transcript. It has recently been observed in statistical analysis that RNA tag counting versions of scRNA-seq data is explained without additional zero inflation.

□ Distinguishing genetic correlation from causation across 52 diseases and complex traits:
>> https://www.biorxiv.org/content/biorxiv/early/2018/04/17/205435.full.pdf
a latent causal variable (LCV) model, under which the genetic correlation between two traits is mediated by a latent variable having a causal effect on each trait. the genetic causality proportion using mixed fourth moments E(α^21α1α2) & E(α^22α1α2) of marginal effect sizes, exploiting the fact that if trait 1 is causal for trait 2 then SNPs w/ large effects on trait 1 (large E(α^21)) will have correlated effects on trait 2 (large E(α1α2)).
□ STREAM: Single-cell Trajectories Reconstruction, Exploration And Mapping of omics data:
>> https://www.biorxiv.org/content/biorxiv/early/2018/04/18/302554.full.pdf
ElPiGraph (Elastic Principal Graph) is a completely redesigned algorithm for elastic principal graph optimization introducing the elastic matrix Laplacian, trimmed mean square error, explicit control for topological complexity and scalability to millions of points.
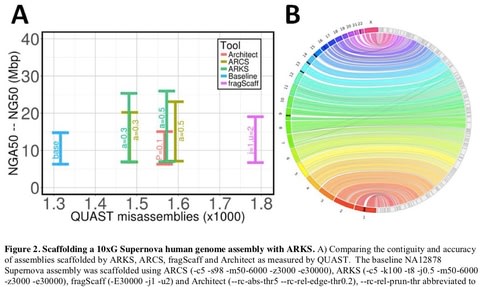
□ ARKS: chromosome-scale scaffolding of human genome drafts with linked read kmers:
>> https://www.biorxiv.org/content/biorxiv/early/2018/04/25/306902.full.pdf
demonstrate how the method provides further improvements of a megabase-scale Supernova human genome assembly, which itself exclusively uses linked read data for assembly, with an execution speed six to nine times faster than competitive linked read scaffolders. Following ARKS scaffolding of a human genome 10xG Supernova assembly, fewer than 9 scaffolds cover each chromosome, except the largest (chromosome 1, n=13).
□ Samplix: the Xdrop technology – for single molecule sample preparation: Information Integrity for Context in Genomics:
>> https://www.samplix.com
Samplix enables single DNA molecule sample preparation where today only multi-molecule sample preparation is possible. Among many applications, the Xdrop™ technology allows for targeted enrichment of very large DNA fragments.

□ A Monte Carlo method for in silico modeling and visualization of Waddington’s epigenetic landscape with intermediate details:
>> https://www.biorxiv.org/content/biorxiv/early/2018/04/29/310771.full.pdf
The method estimates the probability distribution of cellular states by collecting a large number of time-course simulations with random initial conditions. By projecting all the trajectories into a 2-dimensional plane of dimension i and j, we can approximately calculate the quasi-potential U(xi,xj) = −ln P(xi,xj), where P (xi , xj ) is the estimated probability of an equilibrium steady state or a non-equilibrium state.

□ DeepPVP: Deep PhenomeNET Variant Predictor: phenotype-based prioritization of causative variants using deep learning:
>> https://www.biorxiv.org/content/biorxiv/early/2018/05/02/311621.full.pdf
DeepPVP, an extension of the PVP system which uses deep learning and achieves significantly better performance in predicting disease-associated variants than the previous PVP system, as well as competing algorithms that combine pathogenicity and phenotype similarity. DeepPVP not only uses a deep artificial neural network to classify variants into causative and non-causative but also corrects for a common bias in variant prioritization methods in which gene-based features are repeated and potentially lead to overfitting.

□ Whale watching with BulkVis: A graphical viewer for Oxford Nanopore bulk fast5 files:
>> https://www.biorxiv.org/content/biorxiv/early/2018/05/03/312256.full.pdf
BulkVis can annotate signal features based on the metadata within the bulk fast5 file and provides a simple method to relate a base called read back to the channel and time in the data stream from which it originated.
long reads can be incorrectly divided by MinKNOW resulting in single DNA molecules being split into two or more reads. The longest seen to date is 2,272,580 bases in length and reported in eleven consecutive reads.

□ Causal gene regulatory network inference using enhancer activity as a causal anchor
>> https://www.biorxiv.org/content/biorxiv/early/2018/05/03/311167.full.pdf
a data-driven approach to classify enhancer expression as binary or continuous was sufficient to automatically select the best target prediction method, allowing parameter-free application of the method to organisms and cell types where validation data is not currently available.
□ SATurn: A modular bioinformatics framework for the design of robust maintainable web-based and standalone applications
>> https://ddamerell53.github.io/SATurn/
※コメント投稿者のブログIDはブログ作成者のみに通知されます