
(Created with Midjourney v5.2)


□ scDiffEq: drift-diffusion modeling of single-cell dynamics with neural stochastic differential equations
>> https://www.biorxiv.org/content/10.1101/2023.12.06.570508v1
scDiffEq, a drift-diffusion framework for learning the deterministic dynamics. scDiffEq utilizes the metric of Sinkhorn divergence, an unbiased entropically regularized Wasserstein distance. Using multi-time point lineage-traced data, scDiffEq improves prediction of cell fate.
scDiffEq is based on neural Stochastic Differential Equations (SDEs) and is designed to accept cell input of any dimension. scDiffEq requires the annotation of an initial position from which it solves an IVP, to fitting the neural SDE describing the dynamics of the cell manifold.

□ CellHorizon: Probabilistic clustering of cells using single-cell RNA-seq data
>> https://www.biorxiv.org/content/10.1101/2023.12.12.571199v1
CellHorizon a probabilistic method for clustering scRNA-seq data that is based on a generative model. CellHorizon relies on CellAssign that does not require any prior marker gene information and models the expression data using negative binomial distribution.
CellHorizon captures the uncertainty associated with each cell's assignment to a cluster. It also takes dropout into account by associating a dropout rate with each gene so that, dropout and actual zero value in the expression can be differentiated.

□ CytoSimplex: Visualizing Single-cell Fates and Transitions on a Simplex
>> https://www.biorxiv.org/content/10.1101/2023.12.07.570655v1
CytoSimplex quantifies the current state and future differentiation of cells undergoing fate transition. Before cells reach their final fates, they often pass through intermediate multipotent states where they have characteristics and potential to generate multiple lineages.
CytoSimplex models the space of lineage differentiation as a simplex with vertices representing potential terminal fates.
A simplex extends a triangle into any dimension; w/ a point is a OD simplex, a line segment is a 1D simplex, a triangle is a 2D simplex, and a tetrahedron is a 3D simplex. The variables cannot change independently, resulting in K-1 degrees of freedom for a K-dimensional simplex.


□ Lokatt: a hybrid DNA nanopore basecaller with an explicit duration hidden Markov model and a residual LSTM network
>> https://bmcbioinformatics.biomedcentral.com/articles/10.1186/s12859-023-05580-x
Lokatt, a HMM-DNN nanopore DNA basecaller that uses an explicit duration Hidden Markov model (EDHMM) with an additional duration state that models the dwell time of the dominating k-mer.
Lokatt integrates an EDHMM modelling the dynamic of the ratcheting enzyme, and is tasked to learn the complete characteristics of the ion current measurements.
Lokatt adopts residual blocks w/ convolution layers, followed by bi-directional LSTM and an EDHMM layer, totaling 15.3 million parameters. It is used for a sample-to-k-mer level alignment assumes the Gaussian observation probabilities and trained with the Baum-Welch algorithm.

□ Towards explainable interaction prediction: Embedding biological hierarchies into hyperbolic interaction space
>> https://www.biorxiv.org/content/10.1101/2023.12.05.568518v1
Comparing Euclidean and non-Euclidean models, incorporating various prior hierarchies and latent dimensions. Using a pairwise model, Euclidean versions perform similarly or even slightly better according to the binary classification task and are computationally more efficient.
The input sequences are converted to 300-dimensional vectors using Mol2vec and ProtVec embeddings. Subsequently, these encoders, coupled with an embedding clip and exponential map, generate latent representations within a shared hyperbolic manifold using Poincaré maps.
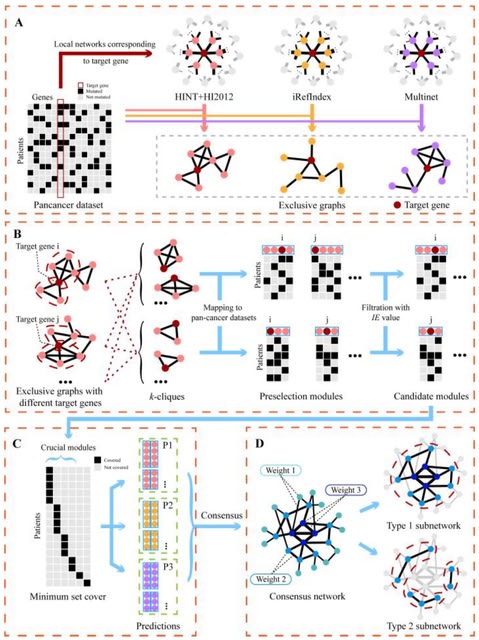
□ MaxCLK: discovery of cancer driver genes via maximal clique and information entropy of modules
>> https://academic.oup.com/bioinformatics/advance-article/doi/10.1093/bioinformatics/btad737/7462770
MaxCLK, an algorithm for identifying cancer driver genes, which was developed by an integrated analysis of somatic mutation data and protein‒protein interaction (PPI) networks and further improved by an information entropy (IE) index.
MaxCLK uses a modified maximal clique algorithm to find all feasible solutions, which is much more efficient than Binary linear programming (BLP). MaxCLK seeks out all the k-cliques. All predictions are consolidated into a weighted undirected network.

□ stGCL: A versatile cross-modality fusion method based on multi-modal graph contrastive learning for spatial transcriptomics
>> https://www.biorxiv.org/content/10.1101/2023.12.10.571025v1
stGCL adopts a novel histology-based Vision Transformer (H-ViT) method to effectively encode histological features and combines multi-modal graph attention auto-encoder (GATE) with contrastive learning to fuse cross-modality features.
stGCL can generate effective embeddings for accurately identifying spatially coherent regions. stGCL combines reconstruction loss and contrastive loss to update the spot embedding.

□ DeconV: Probabilistic Cell Type Deconvolution from Bulk RNA-sequencing Data
>> https://www.biorxiv.org/content/10.1101/2023.12.07.570524v1
DeconV assumes a linear-sum-property between single-cell and bulk gene expression, implying that bulk gene expression is a sum of the components from single-cell gene expression. DeconV models cell-type-specific GE with probability distributions as opposed to point estimates.
DeconV consists of two models, a reference model and a deconvolution model. Reference model learns latent parameters from single-cell reference after which deconvolution model uses the learned parameters to infer optimal cell type composition of a bulk sample.
The reference model, is a probabilistic model consisting of a discrete distribution (zero-inflated Poisson or zero inflated negative-binomial) with cell-type-specific parameters for single-cell gene counts.
The Deconvolution model translates single-cell expression to pseudo-bulk or real bulk gene expression. This is motivated by the aggregation-property of Poisson distributions which states that the sum of two (or more) Poisson random variables has also a Poisson distribution.

□ TIGON: Reconstructing growth and dynamic trajectories from single-cell transcriptomics data
>> https://www.nature.com/articles/s42256-023-00763-w
TIGON (Trajectory Inference with Growth via Optimal transport and Neural network) that infers cell velocity, growth and cellular dynamics by connecting unpaired time-series single-cell transcriptomics data.
TIGON is a dynamic, unbalanced OT model. TIGON features a mesh-free, dimensionless formulation based on Wasserstein–Fisher–Rao (WFR) distance that is readily solvable by neural ODEs and inference of temporal, causal GRNs and growth-related genes.

□ invMap: a sensitive mapping tool for long noisy reads with inversion structural variants
>> https://academic.oup.com/bioinformatics/advance-article/doi/10.1093/bioinformatics/btad726/7460205
invMap, a two step long read alignment strategy (referred to as invMap) with prioritized chaining, which separately deals with the main chain and potential inversion-chain in the candidate aligned region.
By transforming the non-co-linear anchors to co-linear cases, invMap can find the inversion events even with small size. invMap modifies the nonlinear anchors occurring in the aligned region to linear ones and identifies small new chains to detect potential inversions.

□ BayesDeep: Reconstructing Spatial Transcriptomics at the Single-cell Resolution
>> https://www.biorxiv.org/content/10.1101/2023.12.07.570715v1
BayesDeep builds upon a Bayesian negative binomial regression model to recover gene expression at the single-cell resolution. BayesDeep deeply resolves gene expression for all "real" cells by integrating the molecular profile from SRT data and the morphological information.
The response variable is the spot-resolution gene expression measurements in terms of counts; and the explanatory variables are a range of cellular features extracted from the paired histology image, including cell type and nuclei-shape descriptors.
BayesDeep predicts the gene expression of all cells based on their cellular features, regardless of whether they are within or beyond spot regions. The model robustness is achieved by regularization using a spike-and-slab prior distribution to each regression coefficient.

□ DeepEnzyme: a robust deep learning model for improved enzyme turnover number prediction by utilizing features of protein 3D Structures
>> https://www.biorxiv.org/content/10.1101/2023.12.09.570923v1
DeepEnzyme integrates Transformer and Graph Convolutional Networks (GCN) models to distill features from both the enzyme and substrate for predicting kcat.
DeepEnzyme employs GCN to extract structural features based on protein 3D structures and substrate adjacency matrixes; Transformer is utilized to extract sequence features from protein sequences. ColabFold is employed to predict protein 3D structure.

□ scELMo: Embeddings from Language Models are Good Learners for Single-cell Data Analysis
>> https://www.biorxiv.org/content/10.1101/2023.12.07.569910v1
scELMo transfers the information of each cell from the sequencing data space to the LLM embedded space. It can finish this transformation by incorporating information from feature space or cell space.
scELMo with a fine-tuning framework performed better than the same settings but under the zero-short learning framework. scELMo + random emb represents fine-tuning scELMo with random numbers as meaningless gene embeddings.

□ Latent Dirichlet Allocation Mixture Models for Nucleotide Sequence Analysis
>> https://www.biorxiv.org/content/10.1101/2023.12.10.571018v1
LDA can identify subtypes of sequence, such as splice site subtypes enriched in long vs. short introns, and can reliably distinguish such properties as reading frame or species of origin.
LDA can analyze the building blocks from the input sequences (words or nucleotide k-mers) to recognize topics, which describe the features of the input sequences.
After summarizing the k-mer counts at each position in a matrix, LDA calculates k-mer matrices and transforms sequences into topic memberships. Sequence clustering can be achieved by analyzing the topic distributions and the interpretation of topics can reveal functional motifs.

□ H2G2: Generating realistic artificial Human genomes using adversarial autoencoders.
>> https://www.biorxiv.org/content/10.1101/2023.12.08.570767v1
H2G2 (the Haplotypic Human Genome Generator), a method to generate human genomic data on an increased scale using a generative neural network to simulate novel samples, while remaining coherent with the source dataset.
H2G2 uses a Generative Adversarial Network using Wasserstein loss (WGAN) on encoded subsections of genomic data spanning over 15000 mutations, equivalent to 1 megabase of DNA.

□ CellTICS: an explainable neural network for cell-type identification and interpretation based on single-cell RNA-seq data
>> https://academic.oup.com/bib/article-abstract/25/1/bbad449/7461884
CellTICS is a biologically interpretable neural network for (sub-) cell-type identification and interpretation based on single-cell RNA-seq data.
CellTICS prioritizes marker genes with cell-type-specific expression, using a hierarchy of biological pathways for neural network construction, and applying a multi-predictive-layer strategy to predict cell and sub-cell types.
The input of CellTICS are reference scRNA-seq data, reference label, and query data. Reference data and query data should be a gene-by-cell matrix. Reference label should be a two-column matrix representing cell type and sub-cell type of each cell.

□ scHiCyclePred: a deep learning framework for predicting cell cycle phases from single-cell Hi-C data using multi-scale interaction information
>> https://www.biorxiv.org/content/10.1101/2023.12.12.571388v1
scHiCyclePred integrates multiple feature sets extracted from single-cell Hi-C data and employs a fusion-prediction model based on deep learning methods to predict cell cycle phases.
scHiCyclePred uses two feature sets, the bin contact probability feature set, and a small intra-domain contact probability feature set, to improve the accuracy of cell cycle phase prediction.
In the fusion-prediction model, three feature vectors for each cell are input into the model, which generates three vectors in parallel after passing through two convolution modules composed of a Convld layer, BatchNorm layer, Maxpool layer, and Dropout layer. These three generated vectors are then merged into a single vector.

□ HGNNPIP: A Hybrid Graph Neural Network framework for Protein-protein Interaction Prediction
>> https://www.biorxiv.org/content/10.1101/2023.12.10.571021v1
HGNNPIP, as a hybrid supervised learning model, consists of sequence encoding and network embedding modules to comprehensively characterize the intrinsic relationship between two proteins.
IN HGNNPP, a random negative sampling strategy was designed for PPI prediction and compared with PopNS and SimNS. Random negative sampling refers to uniformly sampling negative instances from the space of all answers.

□ SPACE: Spatial Patterning Analysis of Cellular Ensembles enables statistically robust discovery of complex spatial organization at the cell and tissue level
>> https://www.biorxiv.org/content/10.1101/2023.12.08.570837v1
SPACE detects context-dependent associations, quantitative gradients and
orientations, and other organizational complexities. SPACE explores all possible ensembles – single entities, pairs, triplets, and so on – and ranks the strongest patterns of tissue organization.
SPACE compares all moments of any-dimensional distributions, even when the underlying data is compositional. SPACE operates on raw molecular expression data, classified pixels, spatial maps of cellular segmentation, and/or centroid data simultaneously.

□ Hyperedge prediction and the statistical mechanisms of higher-order and lower-order interactions in complex networks
>> https://www.pnas.org/doi/10.1073/pnas.2303887120
a group-based generative model for hypergraphs that does not impose an assortative mechanism to explain observed higher-order interactions, unlike current approaches. This model allows us to explore the validity of the assumptions.
The results indicate that the first assumption appears to hold true for real networks. However, the second assumption is not necessarily accurate; A combination of general statistical mechanisms can explain observed hyperedges.

□ A cross-attention transformer encoder for paired sequence data
>> https://www.biorxiv.org/content/10.1101/2023.12.11.571066v1
A new cross-attention layer that does produce a cross-attended embedding of both inputs as output. This layer can be used in combination with concatenated self-attention layers and parallel self-attention layers.
Transforming the cross-attention matrix to a matching shape. The projected cross-attention matrix has size len(s_a+s_b) × len(s_a+s_b), multiplying this with their Value vector results in a cross-attended embedding for both sequences.

□ Variant Graph Craft (VGC): A Comprehensive Tool for Analyzing Genetic Variation and Identifying Disease-Causing Variants.
>> https://www.biorxiv.org/content/10.1101/2023.12.12.571335v1
Variant Graph Craft (VGC), a VCF analysis tool offering a wide range of features for exploring genetic variations, incl. extraction of variant data, intuitive visualization of variants, and the provision of a graphical representation of samples, complete w/ genotype information.
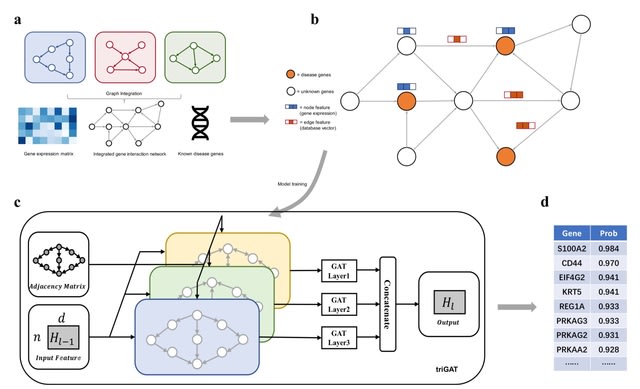
□ DGP-AMIO: Integration of multi-source gene interaction networks and omics data with graph attention networks to identify novel disease genes
>> https://www.biorxiv.org/content/10.1101/2023.12.03.569371v1
DGRP-AMIO (Disease Gene Predictor based on Attention Mechanism and Integration of multi-source gene interaction networks and Omics) merges gene interaction networks of different types and databases into a unified directed graph using triGAT framework.
DGRP-AMIO uses a a 0/1 vector on the edges to indicate the presence or absence of gene interactions in each database and incorporated this edge feature into the training of attention coefficients.

□ Reconstruction of private genomes through reference-based genotype imputation
>> https://genomebiology.biomedcentral.com/articles/10.1186/s13059-023-03105-6
Quantifying the risk of data leakage by developing a potential attack against existing imputation pipelines and then evaluating its effectiveness. The attack strategy resulting from the work consists of two parts: haplotype reconstruction and haplotype linking.
The haplotype reconstruction portion utilizes the output from imputation to reconstruct a set of reference panel haplotypes for each chromosome or for each chromosome “chunk” (i.e., non-overlapping segments within a chromosome).
The haplotype linking portion leverages any available genetic relatives to link across these genomic segments (chromosomes or chunks) to form sets of haplotypes and diplotypes predicted to belong to the same individual.
Reconstructed haplotypes from the same individual could be linked via their genetic relatives using our Bayesian linking algorithm, which allows a substantial portion of the individual’s diploid genome to be reassembled.
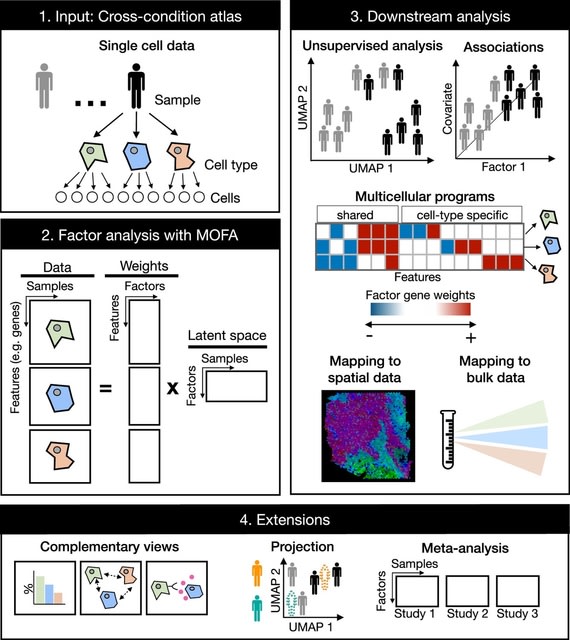
□ Multicellular factor analysis of single-cell data for a tissue-centric understanding of disease
>> https://elifesciences.org/articles/93161
Multicellular Factor Analysis is a fundamental advancement in the factor analysis of cross-condition single-cell atlases.
Multicellular factor analysis allows for the inclusion of structural or communication tissue-level views in the inference of multicellular programs, and the joint modeling of independent studies. Projection of new samples into an inferred multicellular space is also possible.

□ Enhancing Recognition and Interpretation of Functional Phenotypic Sequences through Fine-Tuning Pre-Trained Genomic Models
>> https://www.biorxiv.org/content/10.1101/2023.12.05.570173v1
The genomic diversity within HERV sequence-specific enriched motif regions of the human pangenome was assessed using Odgi Depth. Gene annotations that overlapped with these regions were categorized by chromosome and gene category using Bedtools Intersect.
The HERV & Regulatory phenotype datasets, maintaining the original interval lengths, allowed us to analyze the chromosomal distribution of the corresponding functional and nonfunctional random regions, confirming the uniformity of the constructed datasets across all chromosomes.
Currently, the commonly used pre-training BERT and GPT models have a maximum model input tokens limitation, possibly resulting in loss of spatial information of the genome and important regulatory elements, such as the long-distance Enhancer.
Despite DNA controlling complex life activities, research predominantly focuses on approximately 3% of protein-coding sequences. The fine-tuned HERV dataset reveals that hidden layer features enable the model to recognize phenotypic information in sequences and reduce noise.
To investigate how the model isolates phenotypic label-specific signals, they calculated local representation weight scores (ALRW) for phenotypic labels using average attention matrices.

□ QuadST: A Powerful and Robust Approach for Identifying Cell-Cell Interaction-Changed Genes on Spatially Resolved Transcriptomics
>> https://www.biorxiv.org/content/10.1101/2023.12.04.570019v1
QuadST is motivated by the idea that in the presence of cell-cell interaction, gene expression level can vary with cell-cell distance between cell type pairs, which can be particularly pronounced within and in the vicinity of cell-cell interaction distance.
QuadST infers interaction-changed genes (ICGs) in a specific cell type pair interaction based on a quantile regression model, which allows us to assess the strength of distance-expression association across entire distance quantiles conditioned on gene expression level.

□ GeneExt: a gene model extension tool for enhanced single-cell RNA-seq analysis
>> https://www.biorxiv.org/content/10.1101/2023.12.05.570120v1
GeneExt is a versatile tool to adjust existing gene annotations in order to improve scRNA-seq quantification across species. The software requires minimal input and can be used with minimal options, with default parameters optimized for most species.

□ RERconverge Expansion: Using Relative Evolutionary Rates to Study Complex Categorical Trait Evolution
>> https://www.biorxiv.org/content/10.1101/2023.12.06.570425v1
In this framework, a rate model places constraints on the rates inferred in the transition rate matrix of the Markov model. The rate model specifies which transition rates are zero, and which rates are equal.

□ wQFM-DISCO: DISCO-enabled wQFM improves phylogenomic analyses despite the presence of paralogs
>> https://www.biorxiv.org/content/10.1101/2023.12.05.570122v1
DISCO-R, a variant of DISCO with a refined and improved pruning strategy that provides more accurate and ro-bust results. They also propose wQFM-DISCO (wQFM paired with DISCO) as an adaptation of wQFM to handle multicopy gene trees resulting from GDL events.
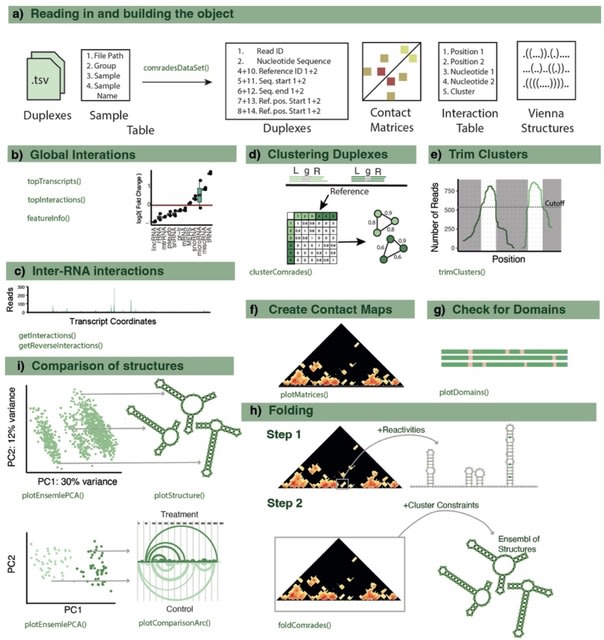
□ comrades-OO: An Object-Oriented R Package for Comprehensive Analysis of RNA Structure Generated using RNA crosslinking experiments
>> https://www.biorxiv.org/content/10.1101/2023.12.12.563348v1
COMRADES Object-Oriented (comrades00), a novel software package for the comprehensive analysis of data derived from the COMRADES (Crosslinking of Matched RNA and Deep Sequencing) method.
comrades00 offers a comprehensive pipeline from raw sequencing reads to the identification of RNA structural features. It includes read processing and alignment, clustering of duplexes, data exploration, folding and comparisons of RNA structures.

□ NestOR: Optimizing representations for integrative structural modeling using Bayesian model selection
>> https://www.biorxiv.org/content/10.1101/2023.12.12.571227v1
NestOR (Nested Sampling for Optimizing Representation), a fully automated, statistically rigorous method based on Bayesian model selection to identify the optimal coarse-grained representation for a given integrative modeling setup.
NestOR objectively determines the optimal coarse-grained representation for a given system and input information. NestOR obtains optimal representations for a system at a fraction of the cost required to assess each representation via full-length production sampling.

□ Oxford Nanopore
>> https://x.com/nanopore/status/1732544126262874346
What’s more, telomere-to-telomere (#t2t) assemblies now achievable with JUST simplex.
Q28 simplex data is accurate enough.
You do not need data from any other platform — paving the way for @nanopore T2T assembly, using just simplex data.
#nanoporeconf 1/2