
(Artwork by Viktor Blinnikov)

□ GPN-MSA: an alignment-based DNA language model for genome-wide variant effect prediction
>> https://www.biorxiv.org/content/10.1101/2023.10.10.561776v1
GPN-MSA, a novel DNA language model which is designed for genome wide variant effect prediction and is based on the biologically-motivated integration of a multiple-sequence alignment (MSA) across diverse species using the flexible Transformer architecture.
GPN-MSA is trained with a weighted cross-entropy loss, designed to downweight repetitive elements and up-weight conserved elements. As data augmentation in non-conserved regions, prior to computing the loss, the reference is sometimes replaced by a random nucleotide.

□ DEMINING: A deep learning model embedded framework to distinguish DNA and RNA mutations directly from RNA-seq
>> https://www.biorxiv.org/content/10.1101/2023.10.17.562625v1
DEMINING incorporated a deep learning model named DeepDDR, which achieved the differentiation of expressed DMs from RMs directly from aligned RNA-seq reads. DEMINING uncovered previously-underappreciated DMs and RMs in unannotated AML-associated gene loci.
DEMINING employs the Light Gradient Boosting Machine (LightGBM), Logistic Regression and Random Forest, RNN and a hybrid of CNN+RNN. DeepDDR with two layers of CNN and the CNN+RNN hybrid model demonstrated comparable performance.

□ scIBD: a self-supervised iterative-optimizing model for boosting the detection of heterotypic doublets in single-cell chromatin accessibility data
>> https://genomebiology.biomedcentral.com/articles/10.1186/s13059-023-03072-y
scIBD, a scCAS-specific self-supervised iterative-optimizing method to boost the detection of heterotypic doublets. As a simulation-based method, scIBD discards the routine random selection strategy that may yield excessive homotypic doublets in the simulation process.
scIBD uses an adaptive strategy to simulate high-confident heterotypic doublets and self-supervise for doublet-detection. scIBD adopts an iterative-optimizing strategy to detect the heterotypic doublets iteratively and finally outputs doublet scores based on an ensemble strategy.

□ CellContrast: Reconstructing Spatial Relationships in Single-Cell RNA Sequencing Data via Deep Contrastive Learning
>> https://www.biorxiv.org/content/10.1101/2023.10.12.562026v1
cellContrast, a deep-learning method that employs a contrastive learning framework for spatial relationship reconstruction. The fundamental assumption is that GE profiles can be projected into a latent space, where physically proximate cells demonstrate higher similarities.
cellContrast employs a contrastive framework of an encoder-projector. During inference, cellContrast discards the projector and uses the output of the encoder for spatial reconstruction, based on the principle that higher cosine similarity indicates shorter spatial distance.

□ sharp: Automated calibration of consensus weighted distance-based clustering approaches
>> https://academic.oup.com/bioinformatics/advance-article/doi/10.1093/bioinformatics/btad635/7320014
The proposed consensus weighted clustering is controlled by two hyper-parameters, including the regularisation parameter and the number of clusters.
Calibrate jointly these two hyper-parameters in a grid search maximising the sharp score, a novel score measuring clustering stability from (weighted) consensus clustering outputs.
The assumption that co-membership probabilities are the same for all pairs of items within a given consensus cluster or between a given pair of consensus clusters, respectively, constitutes a potential limitation of the sharp score.

□ Assessing the limits of zero-shot foundation models in single-cell biology
>> https://www.biorxiv.org/content/10.1101/2023.10.16.561085v1
Geneformer and scGPT exhibit limited reliability in zero-shot settings and often underperform compared to simpler methods. These findings serve as a cautionary note for the deployment of proposed single-cell foundation models.
scGPT defaults to predicting the median bin when only given access to gene embeddings. Masked language modeling (MLM) are not effective at learning gene embeddings, which would also impact Geneformer, given that it produces a cell embedding by averaging over gene embeddings.

□ Relational Composition of Physical Systems: A Categorical Approach
>> https://arxiv.org/abs/2310.06088
The fact that each quadratic form has a unique signature despite the diagonalizing basis non-unique is analogous to how each finite-dimensional vector space has a unique dimension, although the basis that proves that the vector space has a given dimension is non-unique.
Dirac diagrams, a novel notation inspired by both bond graphs and string diagrams. They describe the syntax and semantics of Dirac diagrams. We can construct a category of vector spaces with quadratic forms using the Grothendieck construction.


□ scTab: Scaling cross-tissue single-cell annotation models
>> https://www.biorxiv.org/content/10.1101/2023.10.07.561331v1
scTab, an automated, feature-attention-based cell type prediction model specific to tabular data, and train it using a novel data augmentation scheme across a large corpus of single-cell RNA-seq observations (22.2 million human cells in total).
scTab leverages deep ensembles for uncertainty quantification. Moreover, we account for ontological relationships between labels in the model evaluation to accommodate for differences in annotation granularity across datasets.
The adapted TabNet architecture for scTab consists of two key building blocks: The first building block is the feature transformer, which is a multi-layer perceptron with batch normalization (BN), skip connections, and a gated linear unit nonlinearity (GLU).

□ scPoli: Population-level integration of single-cell datasets enables multi-scale analysis across samples
>> https://www.nature.com/articles/s41592-023-02035-2
scPoli, an open-world learner that incorporates generative models to learn sample and cell representations for data integration, label transfer and reference mapping.
scPoli introduces two modifications to the CVAE architecture. These modifications are the replacement of OHE vectors with continuous vectors of fixed dimensionality to represent the conditional term, and the usage of cell type prototypes to enable label transfer.

□ Hifieval: Evaluation of haplotype-aware long-read error correction
>> https://academic.oup.com/bioinformatics/advance-article/doi/10.1093/bioinformatics/btad631/7321114
Hifieval compares the alignment of the raw read and the alignment of the corrected read. Hifieval evaluates phased assemblies and can distinguish under-corrections and over-corrections.
Hifieval calculates three metrics: correct corrections (CC), errors that are in raw reads but not in corrected reads; under-corrections (UC), errors present in both raw and corrected reads; and over-corrections (OC), new errors found in corrected reads but not in raw reads.
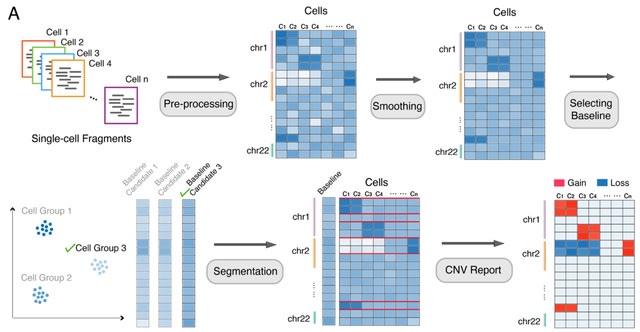
□ AtaCNV: Detecting copy number variations from single-cell chromatin sequencing data
>> https://www.biorxiv.org/content/10.1101/2023.10.15.562383v1
AtaCNV generates a single-cell read count matrix over genomic bins of 1 million base pairs. Cells and genomic bins are filtered according to bin mappability and number of zero entries. AtaCNV smooths the count matrix by fitting a one-order dynamic linear model for each cell.
AtaCNV normalizes the smoothed count data against those of normal cells to deconvolute copy number signals from other confounding factors. AtaCNV clusters the cells and identifies a group of high confidence normal cells and normalizes the data against their smoothed depth data.
AtaCNV applies the multi-sample BIC-seq algorithm to jointly segment all single cells and estimates the copy number ratios for each cell in each segment. CNV burden scores are also derived and cells with high CNV scores are regarded as malignant cells.
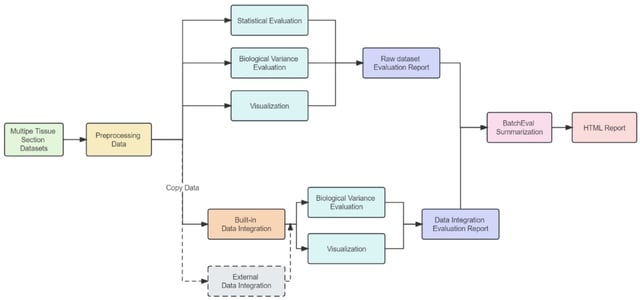
□ BatchEval Pipeline: Batch Effect Evaluation Workflow for Multiple Datasets Joint Analysis
>> https://www.biorxiv.org/content/10.1101/2023.10.08.561465v1
BatchEval Pipeline performs Min-Max normalization and logarithmic mapping preprocessing on each spot/cell gene expression levels and integrates multiple batches of gene expression data into low-dimensional representations.
BatchEval Pipeline employs the Kruskal-Wallis H test to evaluate the variation in the average level of gene expression across different tissue sections and performs variance analysis on gene expression total counts for each tissue section.

□ TEclass2: Classification of transposable elements using Transformers
>> https://www.biorxiv.org/content/10.1101/2023.10.13.562246v1
TEclass2, a new architecture based on the Longformer model for the classification of selected TEs sequences, including various sequence specific aug-mentations, a k-mer specialized tokenizer, and implementing sliding window dilation.
TEclass2 is an all-in-one classifier that can be used to rapidly predict TE orders and superfamilies using TE models built upon the Transformer architecture. For TE DNA sequences, TEclass2 uses only the encoder-block, followed by a classification head as in a linear layer.

□ SPACO: Dimension Reduction by Spatial Components Analysis Improves Pattern Detection in Multivariate Spatial Data
>> https://www.biorxiv.org/content/10.1101/2023.10.12.562016v1
SPACO (Spatial Component Analysis), a proximity-aware kernel method for spatial data. By replacing PCA's global variance target with Moran's I, a measure of local (co)variance, SPACO constructs an ordered sequence of basis vectors, the spatial components (SpaC).
Orthogonal data projection onto the first k SpaCs maximises Moran's I, thereby pooling evidence of spatial dependence across genes with similar patterns. This enhances the sensitivity and spatial precision of the signal.
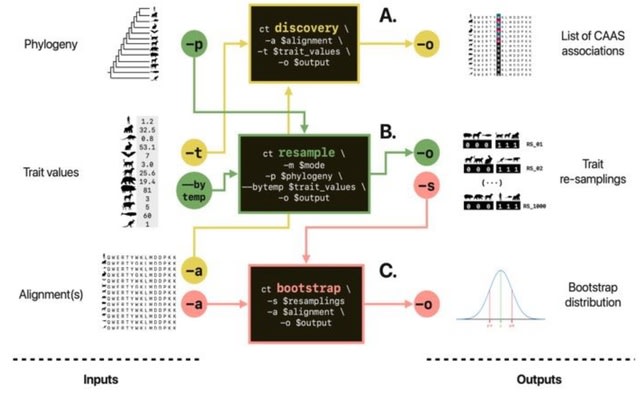
□ CAAStools: a toolbox to identify and test Convergent Amino Acid Substitutions
>> https://academic.oup.com/bioinformatics/advance-article/doi/10.1093/bioinformatics/btad623/7319365
CAAStools, a toolbox to identify and validate CAAS in a phylogenetic context. CAAStools implements different testing strategies through bootstrap analysis. CAAStools is designed to be included in parallel workflows and is optimized to allow scalability at proteome level.

□ Semla: A versatile toolkit for spatially resolved transcriptomics analysis and visualization
>> https://academic.oup.com/bioinformatics/advance-article/doi/10.1093/bioinformatics/btad626/7319366
semla, a toolbox for data processing, exploration, analysis, and visualization of spatial gene expression patterns in tissues. Semla takes advantage of the tidyverse framework for data handling and the patchwork framework for customizable visualization.
semla requires data generated with the Visium Gene Expression profiling platform, including expression matrices, histological images and spot coordinate files produced with the 10x Genomics Space Ranger pipeline.

□ Ggkegg: analysis and visualization of KEGG data utilizing grammar of graphics
>> https://academic.oup.com/bioinformatics/advance-article/doi/10.1093/bioinformatics/btad622/7319364
ggkegg to extend these packages. ggkegg retrieves information such as the KEGG PATHWAY and MODULE, formats them into a structure that is easy to analyze, and offers a series of functions for further analyses and visualization.
ggkegg can also be viewed as an extension of ggplot2, an R package that deconstructs graphical components and composes images as grammar of graphics and serves as the foundation for visualization in numerous publications on bioinformatics.

□ GeneSegNet: a deep learning framework for cell segmentation by integrating gene expression and imaging
>> https://genomebiology.biomedcentral.com/articles/10.1186/s13059-023-03054-0
GeneSegNet makes a joint use of gene spatial coordinates and imaging information for cell segmentation, and is recursively learned by alternating between the optimization of network parameters and estimation of training labels for noise-tolerant training.
GeneSegNet exploits both imaging information and spatial locations of RNA reads for cell segmentation, based on a general U-Net architecture. U-Net downsamples convolutional features several times and then reversely upsamples them in a mirror-symmetric manner.

□ scHiCDiff: Detecting Differential Chromatin Interactions in Single-cell Hi-C Data
>> https://academic.oup.com/bioinformatics/advance-article/doi/10.1093/bioinformatics/btad625/7320006
scHiCDiff, a novel statistical software tool, which applied two non-parametric tests (KS and CVM) and two parametric models (NB and ZINB) to distinguish the bin pairs showing significant changes in contact frequencies between two groups of scHi-C data.
scHiCDiff detects DCIs. Each scHi-C data is imputed by a Gaussian convolution filter to tackle the sparsity issue, then processed by scHiNorm w/ the Negative Binomial Hurdle option to remove systematic biases, and finally normalized for the cell-specific genomic distance effect.

□ iLSGRN: Inference of large-Scale Gene Regulatory Networks based on multi-model fusion
>> https://academic.oup.com/bioinformatics/advance-article/doi/10.1093/bioinformatics/btad619/7321113
iLSGRN reconstructs large-scale GRNs from steady-state and time-series GE data based on nonlinear ODEs. The regulatory gene recognition algorithm calculates the Maximal Information Coefficient and excludes redundant regulatory relationships to achieve dimensionality reduction.
The feature fusion algorithm constructs a model leveraging the feature importance derived from XGBoost and Random Forest models, which can effectively train the nonlinear ODEs model of GRNs and improve the accuracy and stability of the inference algorithm.

□ scLinaX: Quantification of the escape from X chromosome inactivation with the million cell-scale human single-cell omics datasets reveals heterogeneity of escape across cell types and tissues
>> https://www.biorxiv.org/content/10.1101/2023.10.14.561800v1
scLinaX directly quantifies relative gene expression from the inactivated X chromosome with droplet-based scRNA-seq data. scLinaX-multi, an extension for the multiome (RNA + ATAC) dataset to evaluate the escape at the chromatin accessibility level.
First, pseudobulk allele-specific expression profiles are generated for cells expressing each candidate reference SNP. Then, alleles of the reference SNPs on the same X chromosome are listed by correlation analysis of the pseudobulk ASE profiles.
scLinaX assigns which X chromosome is inactivated to each cell based on the allelic expression of the reference SNPs and generates a nearly complete XCI skewed condition in silico and the estimates for the ratio of the expression from Xi.

□ Asterics: a simple tool for the ExploRation and Integration of omiCS data
>> https://bmcbioinformatics.biomedcentral.com/articles/10.1186/s12859-023-05504-9
ASTERICS is designed to make both standard and complex exploratory and integration analysis workflows easily available to biologists and to provide high quality interactive plots.
ASTERICS allows the integration of multiple omics, i.e., it includes exploratory analysis able to explain the typology of individuals described by omics and/or characters simultaneously obtained at different levels of the living organisms.

□ AIWrap: Artificial Intelligence based wrapper for high dimensional feature selection
>> https://bmcbioinformatics.biomedcentral.com/articles/10.1186/s12859-023-05502-x
AIWrap, a novel Artificial Intelligence based Wrapper algorithm. The algorithm predicts the performance of unknown feature subset using an AI model referred here as Performance Prediction Model (PPM).
The performance of AIWrap is evaluated and compared with standard algorithms like LASSO, Adaptive LASSO (ALASSO), Group LASSO (GLASSO), Elastic net (Enet), Adaptive Elastic net (AEnet) and Sparse Partial Least Squares (SPLS) for both the simulated datasets and real data studies.

□ GENEPT: A SIMPLE BUT HARD-TO-BEAT FOUNDATION MODEL FOR GENES AND CELLS BUILT FROM CHATGPT
>> https://www.biorxiv.org/content/10.1101/2023.10.16.562533v1
GenePT demonstrates that LLM embedding of literature is a simple and effective path for biological foundation models. GenePT achieves comparable, and often better, performance than Geneformer and other methods.
GenePT generates single-cell embeddings in two ways: (i) by averaging the gene embeddings, weighted by each gene’s expression level; or (ii) by creating a sentence embedding for each cell, using gene names ordered by the expression level.

□ TDS: Privacy-Preserving Federated Genome-wide Association Studies via Dynamic Sampling
>> https://academic.oup.com/bioinformatics/advance-article/doi/10.1093/bioinformatics/btad639/7323577
TDS (Two-Step Dynamic Sampling), a new efficient, privacy-preserving federated GWAS framework. In the first phase, local parties collaboratively identify loci in their local data that are not significantly associated.
This phase substantially curbs computation and communication costs by removing a large number of non-significant loci from subsequent analysis.
In the second phase, all the local parties iteratively share portions of their private datasets with the server. The server performs GWAS on the pooled data and returns the results to the local parties.

□ GoM DE: interpreting structure in sequence count data with differential expression analysis allowing for grades of membership
>> https://genomebiology.biomedcentral.com/articles/10.1186/s13059-023-03067-9
The concept of “Grade of Membership Differential Expression” (GoM DE) builds upon existing methods to analyze differential expression. By extending these established techniques, we can explore a variety of cell features beyond just discrete cell populations.
Investigateing the question of how to interpret the individual dimensions of a parts-based representation learned by fitting a topic model (in the topic model, the dimensions are also called “topics”)
The GoM DE analysis yields much larger LFC estimates of the cell-type-specific genes. This is because the topic model isolates the biological processes related to cell type while removing background biological processes that do not relate to cell type.
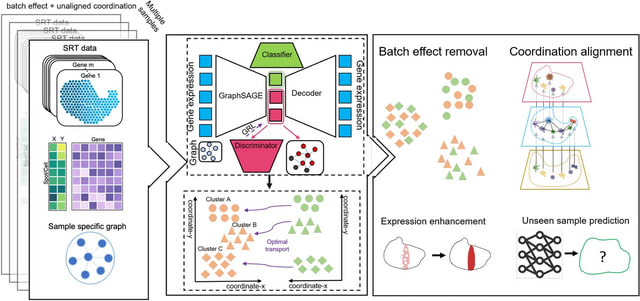
□ SPIRAL: integrating and aligning spatially resolved transcriptomics data across different experiments, conditions, and technologies
>> https://genomebiology.biomedcentral.com/articles/10.1186/s13059-023-03078-6
SPIRAL effectively integrates data in both feature space, including low-dimensional embeddings, high-dimensional gene expressions, and physical space.
SPIRAL combines gene expressions and spatial relationships in the consecutive processes of batch effect removal and coordinate alignment by employing graph-based domain adaption and cluster-aware Gromov-Wasserstein optimal transport.
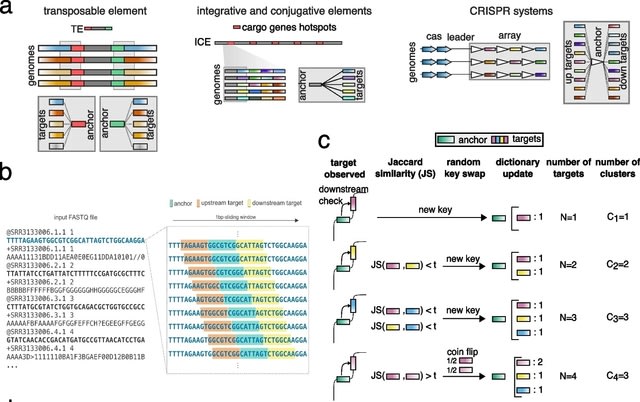
□ DIVE: a reference-free statistical approach to diversity-generating and mobile genetic element discovery
>> https://genomebiology.biomedcentral.com/articles/10.1186/s13059-023-03038-0
DIVE, a novel reference-free algorithm designed to identify sequences that cause genetic diversification such as transposable elements, within MGE variability hotspots, or CRISPR repeats. DIVE operates directly on sequencing reads and does not rely on a reference genome.
DIVE makes the preceding logic into a statistical algorithm. DIVE aims to find anchors with neighboring statistically highly diverse sequences. DIVE processes each read sequentially using a sliding window to construct target dictionaries for each anchor encountered in each read.

□ stVAE deconvolves cell-type composition in large-scale cellular resolution spatial transcriptomics
>> https://academic.oup.com/bioinformatics/advance-article/doi/10.1093/bioinformatics/btad642/7325351
stVAE employs a variational encoder-decoder framework to decompose cell-type mixtures for cellular resolution spatial transcriptomic data. stVAE is scalable to large-scale datasets and has less running time.
stVAE constructs a pseudo-spatial transcriptomic dataset to guide the training of stVAE on the small spatial transcriptomic dataset. stVAE could accurately capture the sparsity of cell-type composition in the spots of cellular resolution spatial transcriptomic data.

□ SEM: sized-based expectation maximization for characterizing nucleosome positions and subtypes
>> https://www.biorxiv.org/content/10.1101/2023.10.17.562727v1
SEM (the Size-based Expectation Maximization), a new nucleosome-calling package. SEM analyzes the overall fragment size distribution to determine which types of nucleosomes are detectable within a given MNase-seq dataset.
SEM employs a hierarchical Gaussian mixture model to accurately estimate the locations and occupancy properties of nucleosomes and to assign subtype identities to each detected nucleosome.

□ MOAL: Multi-Omic Analysis at Lab. A simplified methodology workflow to make reproducible omic bioanalysis.
>> https://www.biorxiv.org/content/10.1101/2023.10.17.562686v1
MOAL (Multi Omic Analysis at Lab), an R package including a omic() function that automates most classical tasks. MOAL automates the bioanalysis corresponding to biostatistics and functional integration procedures.
For annotation tasks, symbols are automatically re-annotated using synonym checking to avoid information loss. MOAL also integrates the NBCI orthologs gene database to open functional enrichment analysis for species that have identified ortholog genes in human.

□ OMICmAge: An integrative multi-omics approach to quantify biological age with electronic medical records
>> https://www.biorxiv.org/content/10.1101/2023.10.16.562114v1
A robust, predictive biological aging phenotype, EMRAge, that balances clinical biomarkers with overall mortality risk and can be broadly recapitulated across EMRs.
Subsequently, they applied elastic-net regression to model EMRAge with DNA-methylation (DNAm) and multiple omics, generating DNAmEMRAge and OMICmAge, respectively.

□ CRAQ: Identification of errors in draft genome assemblies at single-nucleotide resolution for quality assessment and improvement
>> https://www.nature.com/articles/s41467-023-42336-w
CRAQ (Clipping information for Revealing Assembly Quality), a reference-free tool which maps raw reads back to assembled sequences to identify regional and structural assembly errors based on effective clipped alignment information.
CRAQ can identify assembly errors at different scales and transform error counts into corresponding assembly quality indicators (AQIs) that reflect assembly quality at the regional and structural levels.
※コメント投稿者のブログIDはブログ作成者のみに通知されます