

□ DEWÄKSS: Optimal tuning of weighted kNN- and diffusion-based methods for denoising single cell genomics data
>> https://www.biorxiv.org/content/10.1101/2020.02.28.970202v1.full.pdf
DEWÄKSS (Denoising Expression data with a Weighted Affinity Kernel and Self-Supervision) uses a self-supervised technique to tune its parameters.
DEWÄKSS performs at par with or better than other state-of-the-art methods, while providing a self-supervised and hence easily searchable hyper-parameter space, greatly simplifying the application of optimal denoising.
DEWÄKSS expression matrix will have decreased stochastic sampling noise; expression values, incl. zeros that are likely the result of undersampling, will be weighted according to the context. DEWÄKSS can accept any graph derived with any distance metric to create the kNN matrix.

□ MultiChain: Storing and analyzing a genome on a blockchain
>> https://www.biorxiv.org/content/10.1101/2020.03.03.975334v1.full.pdf
Data including but not limited to electronic health records, vcf files from multiple or single individuals, and somatic mutation datasets from cancer patients can be stored in blockchain using our indexing schemes, allowing for rapid and partial retrieval of the data.
MultiChain is a platform specifically designed for building and deploying private blockchain applications. it has a data stream feature, which allows users to create multiple key-value. A data stream in MultiChain can span multiple blocks based on the time of the transaction.
□ β-VAE: Disentangling latent representations of single cell RNA-seq experiments
>> https://www.biorxiv.org/content/10.1101/2020.03.04.972166v1.full.pdf
Variational autoencoders (VAEs) have emerged as a tool for scRNA-seq denoising and data harmonization, but the correspondence between latent dimensions in these models and generative factors remains unexplored.
VAE latent dimensions correspond more directly to data generative factors when using these modified objective functions. β-VAE encourages disentanglement in VAE latent spaces. these methods improve the correspondence between dimensions of the latent space and generative factors.
□ BATI: Efficient and Flexible Integration of Variant Characteristics in Rare Variant Association Studies Using Integrated Nested Laplace Approximation
>> https://www.biorxiv.org/content/10.1101/2020.03.12.988584v1.full.pdf
Integrated Nested Laplace Approximation (INLA)is a recent approach to implement Bayesian inference on latent Gaussian models, which are a versatile and flexible class of models ranging from generalized linear mixed models (GLMMs) to spatial and spatio-temporal models.
Unlike existing RVAS tests BATI - (a Bayesian rare variant Association Test using Integrated Nested Laplace Approximation) allows integration of individual or variant-specific features as covariates, while efficiently performing inference based on full model estimation.

□ Bayesian model selection reveals biological origins of zero inflation in single-cell transcriptomics
>> https://www.biorxiv.org/content/10.1101/2020.03.03.974808v1.full.pdf
a Bayesian model selection approach to unambiguously demonstrate zero inflation in multiple biologically realistic scRNA-Seq datasets.
the primary causes of zero inflation are not technical but rather biological in nature. The parameter estimates from the zero-inflated negative binomial distribution are an unreliable indicator of zero inflation.
Persistence of zero inflation or high levels of over dispersion after accounting for cell type are indicators of unknown sources of biological variation that may prove to be useful in refining cell type hierarchies or positioning cells along the trajectories of a continuum.
□ scPCA: Exploring High-Dimensional Biological Data with Sparse Contrastive Principal Component Analysis
>> https://academic.oup.com/bioinformatics/advance-article-abstract/doi/10.1093/bioinformatics/btaa176/5807607
Several classes of procedures, among them classical dimensionality reduction techniques have provided effective advances; however, no procedure currently satisfies the dual objectives of recovering stable and relevant features simultaneously.
scPCA, a variant of principal component analysis, sparse contrastive principal component analysis, that extracts sparse, stable, interpretable, and relevant biological signal.

□ Scribe: Inferring Causal Gene Regulatory Networks from Coupled Single-Cell Expression Dynamics Using Scribe
>> https://www.cell.com/cell-systems/fulltext/S2405-4712(20)30036-3
Scribe employs Restricted Directed Information to determine causality by estimating the strength of information transferred from a potential regulator to its downstream target by taking advantage of time-delays.
applying Scribe and other leading approaches for causal network reconstruction to several types of single-cell measurements, there is a dramatic drop in performance for “pseudotime”-ordered single-cell data compared with true time-series data.
□ Ultraplexing: increasing the efficiency of long-read sequencing for hybrid assembly with k-mer-based multiplexing
>> https://genomebiology.biomedcentral.com/articles/10.1186/s13059-020-01974-9
Ultraplexing, a new method that allows the pooling of multiple samples in long-read sequencing without relying on molecular barcodes.
To distinguish between Ultraplexing-mediated effects and intrinsic assembly complexity for the selected isolates, the reported assembly accuracy for random (in all experiments) and perfect (in simulations) assignment of long reads.

□ Correlating predicted epigenetic marks with expression data to find interactions between SNPs and genes
>> https://www.biorxiv.org/content/10.1101/2020.02.29.970962v1.full.pdf
a method to make these eQTLs more robust. Instead of correlating the gene expression with the SNP value like in eQTLs, and correlate it with epigenomic data.
predict the epigenomic data from the DNA sequence using the deep learning framework DeepSEA. And calculate all the correlations and focus the interest only on those who have a high difference in DeepSEA.
□ ConsHMM Atlas: conservation state annotations for major genomes and human genetic variation
>> https://www.biorxiv.org/content/10.1101/2020.03.01.955443v1.full.pdf
ConsHMM is a method recently introduced to annotate genomes into conservation states, which are defined based on the combinatorial and spatial patterns of which species align to and match a reference genome in a multi-species DNA sequence alignment.
The ConsHMM Atlas annotates reference genomes at single nucleotide resolution into different conservation states based on the combinatorial and spatial patterns within a multiple species alignment inferred using a multivariate Hidden Markov Model.

□ scRMD: Imputation for single cell RNA-seq data via robust matrix decomposition
>> https://academic.oup.com/bioinformatics/advance-article/doi/10.1093/bioinformatics/btaa139/5771334
a single cell RNA-seq imputation method scRMD based on the robust matrix decomposition. An efficient alternating direction method of multiplier (ADMM) is developed to minimize the objective function.
scRMD assumes the the underlying expression profile of genes is low rank and the dropout events are rare compared with true zero expression.
□ V-SVA: an R Shiny application for detecting and annotating hidden sources of variation in single cell RNA-seq data
>> https://academic.oup.com/bioinformatics/advance-article/doi/10.1093/bioinformatics/btaa128/5771333
Visual Surrogate Variable Analysis (V-SVA) that provides a web-browser interface for the identification and annotation of hidden sources of variation in scRNA-seq data.
V-SVA requires a two-dimensional matrix containing feature counts and sample identifiers. using V-SVA with the IA-SVA algorithm to infer the SV associated with the IFN-β response and genes associated with it.

□ Supervised-learning is an accurate method for network-based gene classification
>> https://academic.oup.com/bioinformatics/advance-article/doi/10.1093/bioinformatics/btaa150/5780279
a comprehensive benchmarking of supervised-learning for network-based gene classification, evaluating this approach and a classic label-propagation technique on hundreds of diverse prediction tasks and multiple networks using stringent evaluation schemes.
supervised-learning on a gene’s full network connectivity outperforms label-propagation and achieves high prediction accuracy by efficiently capturing local network properties, rivaling label-propagation’s appeal for naturally using network topology.

□ reference flow: Reducing reference bias using multiple population reference genomes
>> https://www.biorxiv.org/content/10.1101/2020.03.03.975219v1.full.pdf
the “reference flow” alignment method that uses information from multiple population reference genomes to improve alignment accuracy and reduce reference bias.
Reference flow’s use of pairwise alignments also helps to solve an “N+1” problem; adding one additional reference to the second pass requires only that we index the new genome and obtain an additional whole-genome alignment.
the RandFlow and RandFlow-LD methods that align to “random individuals” from each super population. Reference flow consistently performs worst on the AFR super population, and could imagine building a deeper “tree” of AFR-covering references.

□ graphsim: An R package for simulating gene expression data from graph structures of biological pathways
>> https://www.biorxiv.org/content/10.1101/2020.03.02.972471v1.full.pdf
graphism, a versatile statistical framework to simulate correlated gene expression data from biological pathways, by sampling from a multivariate normal distribution derived from a graph structure.
Computing the nearest positive definite matrix is necessary to ensure that the variance-covariance matrix could be inverted when used as a parameter in multivariate normal simulations, particularly when negative correlations are included for inhibitions.

□ Dune: Improving replicability in single-cell RNA-Seq cell type discovery
>> https://www.biorxiv.org/content/10.1101/2020.03.03.974220v1.full.pdf
Dune optimizes the trade-off between the resolution of the clusters. It takes as input a set of clustering results on a single dataset, derived from any set of clustering algorithms and iteratively merges clusters in order to maximize their concordance between partitions.
Dune automatically stops at a meaningful resolution level, where all clustering algorithms are in agreement, while the other methods either keep merging until all clusters are merged into one or require user supervision.
□ MESA: automated assessment of synthetic DNA fragments and simulation of DNA synthesis, storage, sequencing, and PCR errors
>> https://academic.oup.com/bioinformatics/advance-article/doi/10.1093/bioinformatics/btaa140/5780281
MESA (Mosla Error Simulator), for the assessment of DNA fragments based on limitations of DNA synthesis, amplification, cloning, sequencing methods, and biological restrictions of host organisms.
MESA contains a mutation simulator, using either the error probabilities of the assessment calculation, literature-based or user-defined error rates and error spectra.
□ CENA: Inferring cellular heterogeneity of associations from single cell genomics
>> https://academic.oup.com/bioinformatics/advance-article-abstract/doi/10.1093/bioinformatics/btaa151/5780282
CENA is a method for a joint identification of pairwise association together with the particular subset of cells in which the association is detected. CENA is limited to associations between the genes' expression levels and an additional cellular meta-data of choice.
CENA may reveal dynamic modulation of dependencies along cellular trajectories of temporally evolving states.
□ EPIC: A Tool to Estimate the Proportions of Different Cell Types from Bulk Gene Expression Data
>> https://link.springer.com/protocol/10.1007/978-1-0716-0327-7_17
EPIC includes RNA-seq-based gene expression reference profiles from immune cells and other nonmalignant cell types found in tumors.
EPIC includes the ability to account for an uncharacterized cell type, the introduction of a renormalization step to account for different mRNA content in each cell type, and the use of single-cell RNA-seq data to derive biologically relevant reference gene expression profiles.
□ Incorporating Prior Knowledge into Regularized Regression
>> https://www.biorxiv.org/content/10.1101/2020.03.04.971408v1.full.pdf
the proposed regression with individualized penalties can outperform the standard LASSO in terms of both parameters estimation and prediction performance when the external data is informative.
Optimization of the marginal likelihood on which the empirical Bayes estimation is based is performed using a fast and stable majorization-minimization. The informativeness of the external metadata, which is controlled by the number of non-zero hyperparameters α.

□ Coral accurately bridges paired-end RNA-seq reads alignment
>> https://www.biorxiv.org/content/10.1101/2020.03.03.975821v1.full.pdf
The core of Coral is a novel optimization formulation that can capture the most reliable bridging path while also filter out false paths.
An efficient dynamic programming algorithm is designed to calculate the top N optimum. Coral implements a consensus approach to select the best solution among the N candidates by taking into account the distribution of fragment length.

□ MultiBaC: A strategy to remove batch effects between different omic data types
>> https://journals.sagepub.com/doi/10.1177/0962280220907365
MultiBaC (multiomic Multiomics Batch-effect Correction correction), a strategy to correct batch effects from multiomic datasets distributed across different labs or data acquisition events.
MultiBac strategy is based on the existence of at least one shared data type which allows data prediction across omics. batch effect correction within the same omic modality using traditional methods can be compared with the MultiBaC correction across data types.

□ scHiCExplorer: Approximate k-nearest neighbors graph for single-cell Hi-C dimensional reduction with MinHash
>> https://www.biorxiv.org/content/10.1101/2020.03.05.978569v1.full.pdf
The presented method is able to pro- cess a 10kb single-cell Hi-C data set with 2500 cells and needs 53 GB of memory while the exact k-nearest neighbors approach is not computable with 1 TB of memory.
The fast mode of MinHash by only using the number of collisions as an approximation of the Jaccard similarity offers an additional k-nearest neighbors graph. an implementation of an approximate nearest neighbors method based on local sensitive hashing running in O(n).
□ Algorithm for theoretical mapping of bio-strings for co-expression: bridging genotype to phenotype
>> https://www.biorxiv.org/content/10.1101/2020.03.05.979781v1.full.pdf
Time is the scaling factor for co-expression of two objects; therefore system objects will be known to be co-expressed if they are present at same instance of time.
the theoretical seed base has been presented for bridging between biostring-pairs and their possible co-expression. The algorithm presented a generalized base for observation of bio-string pairs in reference of their possible co-expression.
□ Optimised use of Oxford Nanopore Flowcells for Hybrid Assemblies
>> https://www.biorxiv.org/content/10.1101/2020.03.05.979278v1.full.pdf
a simple washing step allows several libraries to be run on the same flowcell, facilitating the ability to take advantage of shorter running times.
a rapid and simple workflow which potentially reduces the consumables cost of ONT sequencing by at least 20% with no apparent impact on assembly accuracy.
□ Loop detection using Hi-C data with HiCExplorer
>> https://www.biorxiv.org/content/10.1101/2020.03.05.979096v1.full.pdf
It is optimized for a high parallelization by providing the option to assign one thread per chromosome and multiple threads within a chromosome.
The sparser a Hi-C interaction matrix is, the more likely it is that possible valid regions detected by the continuous negative binomial distribution filtering are rejected by Wilcoxon rank-sum test.
□ SkSES: Sketching algorithms for genomic data analysis and querying in a secure enclave
>> https://www.nature.com/articles/s41592-020-0761-8
The SkSES approach is based on trusted execution environments (TEEs) offered by current-generation microprocessors—in particular, Intel’s SGX.
SkSES is a hardware–software hybrid approach for privacy-preserving collaborative GWAS, which improves the running time of the most advanced cryptographic protocols by two orders of magnitude.
□ InvBFM: finding genomic inversions from high-throughput sequence data based on feature mining
>> https://bmcgenomics.biomedcentral.com/articles/10.1186/s12864-020-6585-1
InvBFM uses multiple relevant sequence properties. Pindel only uses split-mapped reads, and both Delly and Lumpy use ISPE of paired-end reads and split-mapped reads.
InvBFM first gathers the results of existing inversion detection tools as candidates for inversions. It then extracts features from the inversions. Finally, it calls the true inversions by a trained support vector machine (SVM) classifier.
□ GRGMF: A Graph Regularized Generalized Matrix Factorization Model for Predicting Links in Biomedical Bipartite Networks
>> https://academic.oup.com/bioinformatics/advance-article-abstract/doi/10.1093/bioinformatics/btaa157/5799077
GRGMF formulates a generalized matrix factorization model to exploit the latent patterns behind observed links.
it can take into account the neighborhood information of each node when learning the latent representation for each node, and the neighborhood information of each node can be learned adaptively.
GRGMF can achieve competitive performance on all these datasets, which demonstrate the effectiveness of GRGMF in prediction potential links in biomedical bipartite networks.
□ PyBSASeq: a simple and effective algorithm for bulked segregant analysis with whole-genome sequencing data
>> https://bmcbioinformatics.biomedcentral.com/articles/10.1186/s12859-020-3435-8
PyBSASeq, a novel, simple, and effective algorithm for analysis of the BSA-Seq data via quantifying the enrichment of likely trait-associated SNPs in a chromosomal interval.
Using PyBSASeq, the significant SNPs (sSNPs), SNPs likely associated with the trait, were identified via Fisher’s exact test, and then the ratio of the sSNPs to total SNPs in a chromosomal interval was used to detect the genomic regions that condition the trait of interest.
□ SECNVs: A Simulator of Copy Number Variants and Whole-Exome Sequences From Reference Genomes
>> https://www.frontiersin.org/articles/10.3389/fgene.2020.00082/full
Variants generated by SECNVs are detected with high sensitivity and precision by tools commonly used to detect copy number variants. Custom codes and algorithms were used to simulate rearranged genomes.
SECNVs finds gaps in the reference genome and fills them with random nucleotides. Because there is no limitation in the number of input chromosome/scaffolds/contigs, SECNVs can be applied to highly fragmented assemblies of nonmodel organisms.
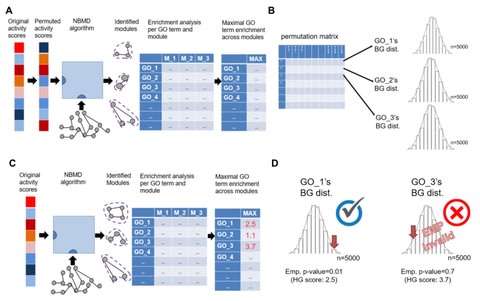
□ DOMINO: a novel network-based module detection algorithm with reduced rate of false calls
>> https://www.biorxiv.org/content/10.1101/2020.03.10.984963v1.full.pdf
DOMINO (Discovery of Modules In Networks using Omics) – a novel NBMD method, and demonstrated that its solutions outperform extant methods in terms of the novel metrics and are typically characterized by a high rate of validated GO terms.
DOMINO receives as input a set of genes flagged as the active genes in a dataset (e.g., the set of genes that passed a differential expression test) and a network of gene interactions, aiming to find disjoint connected subnetworks in which the active genes are enriched.

□ forgeNet: a graph deep neural network model using tree-based ensemble classifiers for feature graph construction
>> https://academic.oup.com/bioinformatics/advance-article-abstract/doi/10.1093/bioinformatics/btaa164/5803642
Sparse learning by incorporating known functional relations between the biological units, such as the graph-embedded deep feedforward network (GEDFN) model, has been a solution to np problem.
a forest graph-embedded deep feedforward network (forgeNet) model, to integrate the GEDFN architecture with a forest feature graph extractor, so that the feature graph can be learned in a supervised manner and specifically constructed for a given prediction task.
□ Kernel integration by Graphical LASSO:
>> https://www.biorxiv.org/content/10.1101/2020.03.11.986968v1.full.pdf
a method for data integration in the framework of an undirected graphical model, where the nodes represent individual data sources of varying nature in terms of complexity and underlying distribution, and where the edges represent the partial correlation between two blocks of data.
a modified GLASSO for estimation of the graph, with a combination of cross-validation and extended Bayes Information Criterion for sparsity tuning.
□ NormiRazor: Tool Applying GPU-accelerated Computing for Determination of Internal References in MicroRNA Transcription Studies
>> https://www.biorxiv.org/content/10.1101/2020.03.11.986901v1.full.pdf
NormiRazor - A high-speed parallel processing software platform for unbiased combinatorial reference gene selection for normalization of expression data.
Mathematical optimization consisted mainly in limiting operations repeated in every iteration to only one execution. Parallel implementation on CUDA-enabled GPU.

□ MetaRib: Reconstructing ribosomal genes from large scale total RNA meta-transcriptomic data
>> https://academic.oup.com/bioinformatics/advance-article/doi/10.1093/bioinformatics/btaa177/5804982
“Total RNA metatranscriptomics” enables us to investigate structural (rRNA) and functional (mRNA) information from samples simultaneously without any PCR or cloning step.
MetaRib performs similarly to EMIRGE in terms of recovering the underlying full-length true sequences, at the same time avoiding generating as many unreliable sequences (false positives) with a significant speedup.
□ GraphBin: Refined binning of metagenomic contigs using assembly graphs
>> https://academic.oup.com/bioinformatics/advance-article-abstract/doi/10.1093/bioinformatics/btaa180/5804980
GraphBin is a new binning method that makes use of the assembly graph and applies a label propagation algorithm to refine the binning result of existing tools.
GraphBin can make use of the assembly graphs constructed from both the de Bruijn graph and the overlap-layout-consensus approach.
□ FAME: Fast And Memory Efficient multiple sequences alignment tool through compatible chain of roots
>> https://academic.oup.com/bioinformatics/advance-article-abstract/doi/10.1093/bioinformatics/btaa175/5805384
The calculated computational complexity of methods supports the results in a way that combining FAME and the MSA tools leads to at least four times faster execution on the datasets.
FAME vertically divides sequences from the places that they have common areas; then they are arranged in consecutive order.
□ BC-t-SNE: Projected t-SNE for batch correction
>> https://academic.oup.com/bioinformatics/advance-article-abstract/doi/10.1093/bioinformatics/btaa189/5807609
Results on artificial single-cell transcription profiling data show that the proposed procedure successfully removes multiple batch effects from t-SNE embeddings, while retaining fundamental information on cell types.
The proposed methods are based on linear algebra and constrained optimization, leading to efficient algorithms and fast computation in many high-dimensional settings.
※コメント投稿者のブログIDはブログ作成者のみに通知されます