God made everything out of nothing. But the nothingness shows through.
─── Paul Valéry( 1871–1945)
□ STARS AS SIGNALS / “We Are Stars”

□ HyperGen: Compact and Efficient Genome Sketching using Hyperdimensional Vectors
>> https://academic.oup.com/bioinformatics/advance-article/doi/10.1093/bioinformatics/btae452/7714688
HyperGen is a Rust library used to sketch genomic files and boost genomic Average Nucleotide Identity (ANI) calculation. HyperGen combines FracMinHash and hyperdimensional computing (HDC) to encode genomes into quasi-orthogonal vectors (Hypervector) in high-dimensional space.
HyperGen adds a key step - Hyperdimensional Encoding for k-mer Hash. This step essentially converts the discrete and numerical hashes in the k-mer hash set to a D-dimensional and nonbinary vector, called sketch hypervector. HyperGen relied on recursive random bit generation.

□ ENGRAM: Symbolic recording of signalling and cis-regulatory element activity to DNA
>> https://www.nature.com/articles/s41586-024-07706-4
ENGRAM, a multiplex strategy for biologically conditional genomic recording in which signal-specific CREs drive the insertion of signal-specific barcodes to a common DNA Tape.
ENGRAM is a recorder assay in which measurements are written to DNA, and an MPRA is a reporter assay in which measurements are made from RNA.
All components would be genomically encoded by a recorder locus within the millions to billions of cells of a model organism, capturing biology as it unfolds over time, and collectively read out at a single endpoint.

□ scGFT: single-cell RNA-seq data augmentation using generative Fourier transformer
>> https://www.biorxiv.org/content/10.1101/2024.07.09.602768v1
scGFT (single-cell Generative Fourier Transformer), a cell-centric generative model built upon the principles of the Fourier Transform. It employs a one-shot transformation paradigm to synthesize GE profiles that reflect the natural biological variability in authentic datasets.
scGFT eschews the reliance on identifying low-dimensional data manifolds, focusing instead on capturing the intricacies of cell expression profiles into a complex space via the Discrete Fourier Transform and reconstruction of synthetic profiles via the Inverse Fourier Transform.

□ scKEPLM: Knowledge enhanced large-scale pre-trained language model for single-cell transcriptomics
>> https://biorxiv.org/cgi/content/short/2024.07.09.602633v1
scKEPLM is the first single-cell foundation model. scKEPLM covers over 41 million single-cell RNA sequences and 8.9 million gene relations. scKEPLM is based on a Masked Language Model (MLM) architecture. It leverages MLMs to predict missing or masked elements in the sequences.
sKEPLM consists of two parallel encoders. scKEPLM employs a Gaussian attention mechanism within the transformer architecture to model the complex high-dimensional interaction. scKEPLM precisely aligns cell semantics with genetic information.

□ HERMES: Holographic Equivariant neuRal network model for Mutational Effect and Stability prediction
>> https://www.biorxiv.org/content/10.1101/2024.07.09.602403v1
HERMES, a 3D rotation equivariant neural network with a more efficient architecture than Holographic Convolutional Neural Network (HCNN), pre-trained on amino-acid propensity, and computationally-derived mutational effects using their open-source code.
HERMES uses a the resulting Fourier encoding of the data an holographic encoding, as it presents a superposition of 3D spherical holograms. Then, the resulting holograms are fed to a stack of SO(3)-Equivariant layers, which convert the holograms to an SO(3)-equivariant embedding.

□ FoldToken3: Fold Structures Worth 256 Words or Less
>> https://www.biorxiv.org/content/10.1101/2024.07.08.602548v1
FoldToken3 re-designs the vector quantization module. FoldToken3 uses a 'partial gradient' trick to allow the encoder and quantifier receive stable gradient no matter how the temperature is small.
Compared to ESM3, whose encoder and decoder have 30.1M and 618.6M parameters with 4096 code space, FoldToken3 has 4.31M and 4.92M parameters with 256 code space.
FoldToken uses only 256 code vectors. FoldToken3 replaces the 'argmax' operation as sampling from a categorical distribution, making the code selection process to be stochastic.

□ RNAFlow: RNA Structure & Sequence Design via Inverse Folding-Based Flow Matching
>> https://arxiv.org/pdf/2405.18768
RNAFlow, a flow matching model for RNA sequence-structure design. In each iteration, RNAFlow first generates a RNA sequence given a noisy protein-RNA complex and then uses RF2NA to fold into a denoised RNA structure.
RNAFlow generates an RNA sequence and its structure simultaneously. Second, it is much easier to train because they do not fine-tune a large structure prediction network. Third, enables us to model the dynamic nature of RNA structures for inverse folding.

□ Mettannotator: a comprehensive and scalable Nextflow annotation pipeline for prokaryotic assemblies
>> https://www.biorxiv.org/content/10.1101/2024.07.11.603040v1
Mettannotator - a comprehensive Nextflow pipeline for prokaryotic genome
annotation that identifies coding and non-coding regions, predicts protein functions, including antimicrobial resistance, and delineates gene clusters.
The Mettannotator pipeline parses the results of each step and consolidates them into a final valid GFF file per genome. The ninth column of the file contains carefully chosen key-value pairs to report the salient conclusions from each tool.

□ Enhancing SNV identification in whole-genome sequencing data through the incorporation of known genetic variants into the minimap2 index
>> https://bmcbioinformatics.biomedcentral.com/articles/10.1186/s12859-024-05862-y
A linear reference sequence index that takes into account known genetic variants using the features of the internal representation of the reference sequence index of the minimap2 tool.
The possibility of modifying the minimap2 tool index is provided by the fact that the hash table does not impose any restrictions on the number of minimizers at a given position of the linear reference sequence.
Adding information about genetic variants does not affect the subsequent alignment algorithm. The linear reference sequence index allows the addition of branches induced by the addition of genetic variants, similar to a genomic graph.

□ GeneBayes: Bayesian estimation of gene constraint from an evolutionary model with gene features
>> https://www.nature.com/articles/s41588-024-01820-9
GeneBayes is an Empirical Bayes framework that can be used to improve estimation of any gene property that one can relate to available data through a likelihood function.
GeneBayes trains a gradient-boosted trees to predict the parameters of the prior distribution by maximizing the likelihood. GeneBayes computes a per-gene posterior distribution for the gene property of interest, returning a posterior mean and 95% credible interval for each gene.

□ METASEED: a novel approach to full-length 16S rRNA gene reconstruction from short read data
>> https://bmcbioinformatics.biomedcentral.com/articles/10.1186/s12859-024-05837-z
METASEED, an alternative where they use amplicon 16S rRNA data and shotgun sequencing data from the same samples, helping the pipeline to determine how the original 16S region would look.
METASEED eliminates undesirable noises and produce high quality, reasonable length 16S sequences. The method is designed to broaden the repertoire of sequences in 16S rRNA reference databases by reconstructing novel near full length sequences.
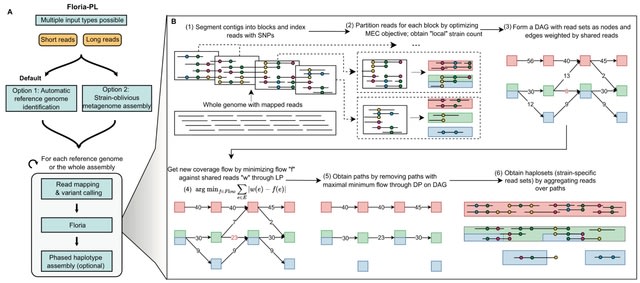
□ Floria: fast and accurate strain haplotyping in metagenomes
>> https://academic.oup.com/bioinformatics/article/40/Supplement_1/i30/7700908
Floria, a novel method designed for rapid and accurate recovery of strain haplotypes from short and long-read metagenome sequencing data, based on minimum error correction (MEC) read clustering and a strain-preserving network flow model.
Floria can function as a standalone haplotyping method, outputting alleles and reads that co-occur on the same strain, as well as an end-to-end read-to-assembly pipeline (Floria-PL) for strain-level assembly.

□ CLADES: Unveiling Clonal Cell Fate and Differentiation Dynamics: A Hybrid NeuralODE-Gillespie Approach
>> https://www.biorxiv.org/content/10.1101/2024.07.08.602444v1
CLADES (Clonal Lineage Analysis with Differential Equations and Stochastic Simulations), a model estimator, namely a NeuralODE based framework, to delineate meta-clone specific trajectories and state-dependent transition rates.
CLADES is a data generator via the Gillespie algorithm, that allows a cell, for a randomly extracted time interval, to choose either a proliferation, differentiation, or apoptosis process in a stochastic manner.
CLADES can estimate the summary of the divisions between progenitors and progeny, and showed that the fate bias between all progenitor-fate pairs can be inferred probabilistically.

□ scRL: Reinforcement learning guides single-cell sequencing in decoding lineage and cell fate decisions https://www.biorxiv.org/content/10.1101/2024.07.04.602019v1
scRL utilizes a grid world created from a UMAP two-dimensional embedding of high-dimensional data, followed by an actor-critic architecture to optimize differentiation strategies and assess fate decision strengths.
The effectiveness of scRL is demonstrated through its ability to closely align pseudotime with distance trends in the two-dimensional manifold and to correlate lineage potential with pseudotime trends.

□ scMaSigPro: Differential Expression Analysis along Single-Cell Trajectories
>>
scMaSigPro, a method initially developed for serial analysis of transcriptomics data, to the analysis of scRNA-seq trajectories. scMaSigPro detects genes that change their expression in Pseudotime and b/n branching paths.
scMaSigPro establishes the polynomial model by assigning dummy variables to each branch, following the approach of the original maSigPro method for the Generalized Linear Model. scMaSigPro is therefore suited for diverse topologies and cell state compositions.

□ spASE: Detection of allele-specific expression in spatial transcriptomics
>> https://genomebiology.biomedcentral.com/articles/10.1186/s13059-024-03317-4
spASE detects ASE in spatial transcriptomics while accounting for cell type mixtures. spACE can estimate the contribution from each cell type to maternal and paternal allele counts at each spot, calculated based on cell type proportions and differential expression.
spASE enables modeling of the maternal allele probability spatial function both across and within cell types. spASE generates high resolution spatial maps of X-chromosome ASE and identify a set of genes escaping XCI.

□ Tuning Ultrasensitivity in Genetic Logic Gates using Antisense RNA Feedback
>> https://www.biorxiv.org/content/10.1101/2024.07.03.601968v1
The antisense RNAs (asRNAs) are expressed with the existing messenger RNA (mRNA) of a logic gate in a single transcript and target mRNAs of adjacent gates, creating a feedback of the protein-mediated repression that implements the core function of the logic gates.
A gate with multiple inputs logically consistent with the single-transcript RNA feedback connection must implement a generalized inverter structure on the molecular level.

□ GS-LVMOGP: Scalable Multi-Output Gaussian Processes with Stochastic Variational Inference
>> https://arxiv.org/abs/2407.02476
The Latent Variable MOGP (LV-MOGP) models the covariance between outputs using a kernel applied to latent variables, one per output, leading to a flexible MOGP model that allows efficient generalization to new outputs with few data points.
GS-LVMOGP, a generalized latent variable multi-output Gaussian process model w/in a stochastic variational inference. By conducting variational inference for latent variables and inducing values, GS-LVMOGP manages large-scale datasets with Gaussian/non-Gaussian likelihoods.

□ scTail: precise polyadenylation site detection and its alternative usage analysis from reads 1 preserved 3' scRNA-seq data
>> https://www.biorxiv.org/content/10.1101/2024.07.05.602174v1
scTail, an all-in-one stepwise computational method. scTail takes an aligned bam file from STARsolo (with higher tolerance of low-quality mapping) as input and returns the detected PASs and a PAS-by-cell expression matrix.
scTail embedded a pre-trained sequence model to remove the false positive clusters, which enabled us to further evaluate the reliability of the detection by examining the supervised performance metrics and learned sequence motifs.

□ MaxComp: Prediction of single-cell chromatin compartments from single-cell chromosome structures
>> https://www.biorxiv.org/content/10.1101/2024.07.02.600897v1
MaxComp, an unsupervised method to predict single-cell compartments using graph-based programming. MaxComp determines single-cell A/B compartments from geometric considerations in 3D chromosome structures.
Segregation of chromosomal regions into two compartments can then be modeled as the Max-cut problem, a semidefinite graph programming method, which optimizes a cut through a set of edges such that the total weights of the cut edges will be maximized.

□ REGLE: Unsupervised representation learning on high-dimensional clinical data improves genomic discovery and prediction
>> https://www.nature.com/articles/s41588-024-01831-6 https://www.nature.com/articles/s41588-024-01831-6
REGLE (Representation Learning for Genetic Discovery on Low-Dimensional Embeddings) is based on the variational autoencoder (VAE) model. REGEL learns a nonlinear, low-dimensional, disentangled representation.
REGLE performs GWAS on all learned coordinates. Finally, It trains a small linear model to learn weights for each latent coordinate polygenic risk scores to obtain the final disease-specific polygenic risk scores.

□ GALEON: A Comprehensive Bioinformatic Tool to Analyse and Visualise Gene Clusters in Complete Genomes
>> https://academic.oup.com/bioinformatics/advance-article/doi/10.1093/bioinformatics/btae439/7709405
GALEON identifies gene clusters by studying the spatial distribution of pairwise physical distances among gene family members along with the genome-wide gene density.
GALEON can also be used to analyse the relationship between physical and evolutionary distances. It allows the simultaneous study of two gene families at once to explore putative co-evolution.
GALEON implements the Cst statistic, which measures the proportion of the genetic distance attributable to unclustered genes. Cst values are estimated separately for each chromosome (or scaffold), as well as for the whole genome data.

□ DNA walk of specific fused oncogenes exhibit distinct fractal geometric characteristics in nucleotide patterns
>> https://www.biorxiv.org/content/10.1101/2024.07.05.602166v1
Fractal geometry and DNA walk representation were employed to investigate the geometric features i.e., self-similarity and heterogeneity in DNA nucleotide coding sequences of wild-type and mutated oncogenes, tumour-suppressor, and other unclassified genes.
The mutation-facilitated self-similar and heterogenous features were quantified by the fractal dimension and lacunarity coefficient measures. The geometrical orderedness and disorderedness in the analyzed sequences were interpreted from the combination of the fractal measures.

□ Mutational Constraint Analysis Workflow for Overlapping Short Open Reading Frames and Genomic Neighbours
>> https://www.biorxiv.org/content/10.1101/2024.07.07.602395v1
sORFs show a similar mutational background to canonical genes, yet they can contain a higher number of high impact variants.
This can have multiple explanations. It might be that these regions are not intolerant against loss-of-function variants or that these non-constrained sORFs do not encode functional microproteins.
This similarity in distribution does not provide sufficient evidence for a potential coding effect in sORFs, as it may be fully explainable probabilistically, given that synonymous and protein truncating variants have fewer opportunities to occur compared to missense variants.
sORFs are mostly embedded into a moderately constraint genomic context, but within the gencode dataset they identified a subset of highly constrained sORFs comparable to highly constrained canonical genes.

□ SimSpliceEvol2: alternative splicing-aware simulation of biological sequence evolution and transcript phylogenies
>> https://bmcbioinformatics.biomedcentral.com/articles/10.1186/s12859-024-05853-z
SimSpliceEvol2 generates an output that comprises the gene sequences located at the leaves of the guide gene tree. The output also includes the transcript sequences associated with each gene at each node of the guide gene tree, by providing details about their exon content.
SimSpliceEvol2 also outputs all groups of orthologous transcripts. Moreover, SimSpliceEvol2 outputs the phylogeny for all the transcripts at the leaves of the guide tree. This phylogeny consists of a forest of transcript trees, describing the evolutionary history of transcripts.

□ d-Fulgor: Where the patterns are: repetition-aware compression for colored de Bruijn graphs
>> https://www.biorxiv.org/content/10.1101/2024.07.09.602727v1
The algorithms factorize the color sets into patterns that repeat across the entire collection and represent these patterns once, instead of redundantly replicating their representation as would happen if the sets were encoded as atomic lists of integers.
d-Fulgor, is a "horizontal" compression method which performs a representative/differential encoding of the color sets. The other scheme, m-Fulgor, is a "vertical" compression method which instead decomposes the color sets into meta and partial color sets.

□ MAGA: a contig assembler with correctness guarantee
>> https://www.biorxiv.org/content/10.1101/2024.07.10.602853v1
MAGA (Misassembly Avoidance Guaranteed Assembler), a model for structural correctness in de Bruijn graph based assembly. MAGA estimates the probability of misassembly for each edge in the de Bruijn graph.
when k-mer coverage is high enough for computing accurate estimates, MAGA produces as contiguous assemblies as a state-of-the-art assembler based on heuristic correction of the de Bruin graph such as tip and bulge removal.

□ SDAN: Supervised Deep Learning with Gene Annotation for Cell Classification
>> https://www.biorxiv.org/content/10.1101/2024.07.15.603527v1
SDAN encodes gene annotations using a gene-gene interaction graph and incorporates gene expression as node attributes. It then learns gene sets such that the genes in a set share similar expression and are located close to each other in the graph.
SDAN combines gene expression data and gene annotations (gene-gene interaction graph) to learn a gene assignment matrix, which specifies the weights of each gene for all latent components.
SDAN uses the gene assignment matrix to reduce the gene expression data of each cell to a low-dimensional space and then makes predictions in the low-dimensional space using a feed-forward neural network.

□ Orthanq: transparent and uncertainty-aware haplotype quantification with application in HLA-typing
>> https://bmcbioinformatics.biomedcentral.com/articles/10.1186/s12859-024-05832-4
Orthanq relies on the statistically accurate determination of posterior variant allele frequency (VAF) distributions of the known genomic variation each haplotype (HLA allele) is made of, while still enabling to use local phasing information.
Orthanq can directly utilize existing pangenome alignments and type all HLA loci. By combining the posterior VAF distributions in a Bayesian latent variable model, Orthanq can calculate the posterior probability of each possible combination of haplotypes.

□ R2Dtool: Integration and visualization of isoform-resolved RNA features
>> https://www.biorxiv.org/content/10.1101/2022.09.23.509222v3
R2Dtool exploits the isoform- resolved mapping of RNA features, such as those obtained from long-read sequencing, to enable simple, reproducible, and lossless integration, annotation, and visualization of isoform-specific RNA features.
R2Dtool's core function liftover transposes the transcript-centric coordinates of the isoform-mapped sites to genome-centric coordinates.
R2Dtool introduces isoform-aware metatranscript plots and metajunction plots to study the positonal distribution of RNA features around annotated RNA landmarks.

□ Composite Hedges Nanopores: A High INDEL-Correcting Codec System for Rapid and Portable DNA Data Readout
>> https://www.biorxiv.org/content/10.1101/2024.07.12.603190v1
The Composite Hedges Nanopores (CHN) coding algorithm tailored for rapid readout of digital information storage in DNA. The Composite Hedges Nanopores could independently accelerate the readout of stored DNA data with less physical redundancy.
The core of CHN's encoding process features constructing DNA sequences that are synthesis-friendly and highly resistant to indel errors, launching a different hash function to generate discrete values about the encoding message bits, previous bits, and index bits.

□ Genome-wide analysis and visualization of copy number with CNVpytor in igv.js
>> https://academic.oup.com/bioinformatics/advance-article/doi/10.1093/bioinformatics/btae453/7715874
The CNVpytor track in igv.js provides enhanced functionality for the analysis and inspection of copy number variations across the genome.
CNVpytor and its corresponding track in igv.js provide a certain degree of standardization for inspecting raw data. In the future, developing a standard format for inspecting raw signals and converting outputs from various callers into such a format would be ideal.