
(The Genocide Memorial in Yerevan, Armenia, by architects Artur Tarkhanyan and Sashur Kalashyan.)


□ Dynamo: Mapping Vector Field of Single Cells
>> https://dynamo-release.readthedocs.io/en/latest/Differential_geometry.html
Dynamo goes beyond discrete RNA velocity vectors to continous RNA vector field functions. With differential geometry analysis of the continous vector field fuctions, Dynamo calculates the RNA Jacobian, which is a cell by gene by gene tensor, encoding the gene regulatory network.
Dynamo builds a cell-wise transition matrix by translating the velocity vector direction and the spatial relationship of each cell to transition probabilities. Dynamo uses a few different kernels to build a transition matrix which can then be used to run Markov chain simulations.
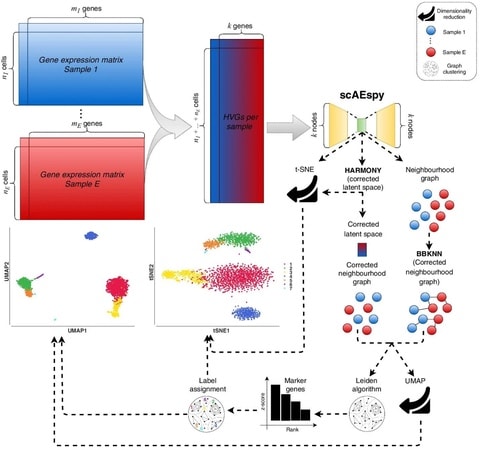
□ scAEspy: Analysis of single-cell RNA sequencing data based on autoencoders
>> https://bmcbioinformatics.biomedcentral.com/articles/10.1186/s12859-021-04150-3
scAEspy can be used to deal with the existing batch-effects among samples. Indeed, the application of batch-effect removal tools into the latent space allowed us to outperform state-of-the-art methods as well as the same batch-effect removal tools applied on the PCA space.
GMMMD and GMMMDVAE, two novel Gaussian-mixture AEs that combine MMDAE and MMDVAE with GMVAE to exploit more than one Gaussian distribution.
scAEspy is used to reduce the HVG space (k dimensions), and the obtained latent space can be used to calculate a t-SNE space. The corrected latent space by Harmony is then used to build a neighbourhood graph, which is clustered by using the Leiden algorithm.

□ Model guided trait-specific co-expression network estimation as a new perspective for identifying molecular interactions and pathways
>> https://journals.plos.org/ploscompbiol/article?id=10.1371/journal.pcbi.1008960
a mathematically justified bridge between parametric approaches & co-expression networks in light of identifying molecular interactions underlying complex traits. a methodological fusion to cross-exploit all scheme-specific strengths via a built-in information-sharing mechanism.
A novel dependency metric is provided to account for certain collinearities in data that are considered problematic w/ the parametric methods. The underlying parametric model is used again to provide a parametric interpretation for the estimated co-expression network elements.

□ Recovering Spatially-Varying Cell-Specific Gene Co-expression Networks for Single-Cell Spatial Expression Data
>> https://www.frontiersin.org/articles/10.3389/fgene.2021.656637/full
a simple and computationally efficient two-step algorithm to recover spatially-varying cell-specific gene co-expression networks for single-cell spatial expression data.
The algorithm first estimates the gene expression covariance matrix for each cell type and then leverages the spatial locations of cells to construct cell-specific networks.
The second step uses expression covariance matrices estimated in step one and label information from neighboring cells as an empirical prior to obtain thresholded Bayesian posterior estimates.

□ scSNV: accurate dscRNA-seq SNV co-expression analysis using duplicate tag collapsing
>> https://genomebiology.biomedcentral.com/articles/10.1186/s13059-021-02364-5
Identifying single nucleotide variants has become common practice for dscRNA-seq; however, a pipeline does not exist to maximize variant calling accuracy. Molecular duplicates generated in these experiments have not been utilized to optimally detect variant co-expression.
scSNV is designed from the ground up to “collapse” molecular duplicates and accurately identify variants and their co-expression. scSNV has fewer false-positive SNV calls than Cell Ranger and STARsolo when using pseudo-bulk samples.

□ Capturing dynamic relevance in Boolean networks using graph theoretical measures
>> https://academic.oup.com/bioinformatics/advance-article/doi/10.1093/bioinformatics/btab277/6275260
the selection captures two types of compounds based on static properties. First, the detectable highly connected dynamic influencing drivers. Second, a new set of dynamic drivers, which called gatekeepers - nodes with high dynamic relevance but no high connectivity.
The existence of paths from gatekeeper nodes to hubs having a higher maximal mutual information than other classes further demonstrates that this principle extends to longer paths, that is there exist channels of information flow which are more stable carriers of signals.

□ DSBS: A new approach to decode DNA methylome and genomic variants simultaneously from double strand bisulfite sequencing
>> https://academic.oup.com/bib/advance-article/doi/10.1093/bib/bbab201/6289882
DSBS analyzer is a pipeline to analyzing Double Strand Bisulfite Sequencing data, which could simultaneously identify SNVs and evaluate DNA methylation levels in a single base resolution.
In DSBS, bisulfite-converted Watson strand and reverse complement of bisulfite-converted Crick strand derived from the same double-strand DNA fragment were sequenced in read 1 and read 2, and aligned to the same position on reference genome.
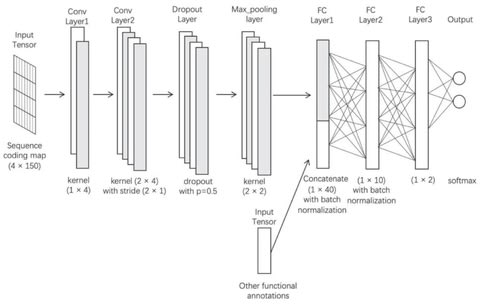
□ A semi-supervised deep learning approach for predicting the functional effects of genomic non-coding variations
>> https://bmcbioinformatics.biomedcentral.com/articles/10.1186/s12859-021-03999-8
The semi-supervised deep learning model coupled with pseudo labeling has advantages in studying with limited datasets, which is not unusual in biology. This study provides an effective approach in finding non-coding mutations potentially associated with various biological phenomena.
This model included three fully-connected (FC) layers, which are also known as dense layers. The input to the first FC layer is generated by concatenating the output of the max pooling function with the additional feature map of the epigenetic and nucleotide composition features.

□ Deciphering biological evolution exploiting the topology of Protein Locality Graph
>> https://www.biorxiv.org/content/10.1101/2021.06.03.446976v1.full.pdf
The lossless graph compression from PLG to a power graph called Protein Cluster Interaction Network (PCIN) results in a 90% size reduction and aids in improving computational time.
the topology of PCIN and capability of deriving the correct species tree by focusing on the cross-talk between the protein modules. Traces of evolution are not only present at the level of the PPI, but are also very much present at the level of the inter-module interactions.
□ SSG-LUGIA: Single Sequence based Genome Level Unsupervised Genomic Island Prediction Algorithm
>> https://academic.oup.com/bib/advance-article-abstract/doi/10.1093/bib/bbab116/6290171
SSG-LUGIA, a completely automated and unsupervised approach for identifying GIs and horizontally transferred genes.
SSG-LUGIA leverages the atypical compositional biases of the alien genes to localize GIs in prokaryotic genomes. The anomalous segments thus identified are further refined following a post-processing step, and finally, the proximal segments are merged to produce the list of GIs.

□ TreeVAE: Reconstructing unobserved cellular states from paired single-cell lineage tracing and transcriptomics data
>> https://www.biorxiv.org/content/10.1101/2021.05.28.446021v1.full.pdf
TreeVAE uses a variational autoencoder (VAE) to model the observed transcriptomic data while accounting for the phylogenetic relationships between cells.
TreeVAE couples a complex non-linear observation model with a more simple correlation model in latent space (any marginal distribution for the GRW is tractable). TreeVAE could be improved by exploiting just-in-time compilation(e.g. JAX), to speed-up the message passing algorithm.
□ FDDH: Fast Discriminative Discrete Hashing for Large-Scale Cross-Modal Retrieval
>> https://ieeexplore.ieee.org/document/9429177/
Formulating the learning of similarity-preserving hash codes in terms of orthogonally rotating the semantic data, so as to minimize the quantization loss of mapping data to hamming space and propose a fast discriminative discrete hashing for large-scale cross-modal retrieval.
FDDH introduces an orthogonal basis to regress the targeted hash codes of training examples to their corresponding semantic labels and utilizes the ϵ-dragging technique to provide provable large semantic margins.
FDDH theoretically approximates the bi-Lipschitz continuity. An orthogonal transformation scheme is further proposed to map the nonlinear embedding data into the semantic subspace. The discriminative power of semantic information can be explicitly captured and maximized.

□ BAVARIA: Simultaneous dimensionality reduction and integration for single-cell ATAC-seq data using deep learning
>> https://www.biorxiv.org/content/10.1101/2021.05.11.443540v1.full.pdf
Several methods have been introduced for dimensionality reduction using scATAC- seq data, including latent Dirichlet allocation (cisTopic), latent Semantic indexing (LSI), SnapATAC and SCALE.
BAVARIA, a batch-adversarial variational auto- encoder (VAE) that facilitates dimensionality reduction and integration for scATAC-seq data, which facilitates simultaneous dimensionality reduction and batch correction via an adversarial learning strategy.

□ ontoFAST: An R package for interactive and semi-automatic annotation of characters with biological ontologies
>> https://www.biorxiv.org/content/10.1101/2021.05.11.443562v1.full.pdf
The commonly used Entity-Quality (EQ) syntax provides rich semantics and high granularity for annotating phenotypes and characters using ontologies. However, EQ syntax might be time inefficient if this granularity is unnecessary for downstream analysis.
ontoFAST that aids production of fast annotations of characters and character matrices with biological ontologies. OntoFAST enhances data interoperability between various applications and support further integration of ontological and phylogenetic methods.
□ Unsupervised weights selection for optimal transport based dataset integration
>> https://www.biorxiv.org/content/10.1101/2021.05.12.443561v1.full.pdf
Horizontal integration describes the problem of merging two or more datasets expressed in a common feature space, each of those containing samples gathered across distinct sources or experiments.
Vertical dataset integration re- duces to horizontal dataset integration in this latent space. The extra layer of difficulty in this approach comes from con- structing a relevant latent space via mappings that preserve enough information.
a variant of the optimal transport (OT)- and Gromov-Wasserstein (GW)- based dataset integration algorithm introduced in SCOT.
Formulating a constrained quadratic program to adjust sample weights before OT or GW so that weighted point density is close to be uniform over the point cloud, for a given kernel.

□ Novel feature selection via kernel tensor decomposition for improved multi-omics data analysis
>> https://www.biorxiv.org/content/10.1101/2021.05.21.445049v1.full.pdf
Kernel tensor decomposition (KTD)-based unsupervised feature extraction (FE) was extended to integrate multi-omics datasets measured over common samples in a weight-free manner.
□ A graphical, interactive and GPU-enabled workflow to process long-read sequencing data
>> https://www.biorxiv.org/content/10.1101/2021.05.11.443665v1.full.pdf
An Extended Biodepot-workflow-builder (Bwb) to provide a modular and easy-to-use graphical interface that allows users to create, customize, execute, and monitor bioinformatics workflows.
And observed a 34x speedup and a 109x reduction in costs for the rate-limiting basecalling step in the cell line data. The graphical interface and greatly simplified deployment facilitate the adoption of GPUs for rapid, cost-effective analysis of long-read sequencing.
□ bathometer: lightning fast depth-of-reads query
>> https://academic.oup.com/bioinformatics/advance-article/doi/10.1093/bioinformatics/btab372/6275265
Bathometer aims for an index that is compact and can be used without having to be read into memory completely. An index stores for each strand of each reference sequence the list of starting positions and the list of end positions of all reads.

□ Crinet: A computational tool to infer genome-wide competing endogenous RNA (ceRNA) interactions
>> https://journals.plos.org/plosone/article?id=10.1371/journal.pone.0251399
Crinet (CeRna Interaction NETwork) considers all mRNAs, lncRNAs, and pseudogenes as potential ceRNAs and incorporates a network deconvolution method to exclude the spurious ceRNA pairs.
Crinet incorporates miRNA-target interactions with binding scores, gene-centric copy number aberration (CNA), and expression datasets. If binding scores are not available, the same score for all interactions could be used.
□ FAME: A framework for prospective, adaptive meta-analysis (FAME) of aggregate data from randomised trials
>> https://journals.plos.org/plosmedicine/article?id=10.1371/journal.pmed.1003629
FAME can reduce the potential for bias, and produce more timely, thorough and reliable systematic reviews of aggregate data.
The FAME estimates of absolute information size and power, and the associated decision on meta-analysis timing should be included. FAME is suited to situations where quick and robust answers are needed, but prospective IPD meta-analysis would be too protracted.
□ POEMColoc: Estimating colocalization probability from limited summary statistics
>> https://bmcbioinformatics.biomedcentral.com/articles/10.1186/s12859-021-04170-z
POEMColoc (POint EstiMation of Colocalization) imputes missing summary statistics for one or both traits using LD structure in a reference panel, and performs colocalization using the imputed summary statistics.
POEMColoc does not discard information when full summary statistics are available for one but not both of the traits and does not assume that both traits have a causal variant in the region.
□ Swarm: A federated cloud framework for large-scale variant analysis
>> https://journals.plos.org/ploscompbiol/article?id=10.1371/journal.pcbi.1008977
With Swarm, large genomic datasets hosted on different cloud platforms or on-premise systems can be jointly analyzed with reduced data motion. Swarm can in principle facilitate federated learning by transferring models across clouds.
Swarm can help transfer intermediate results of the machine learning models across the cloud, so that the model can continue to learn and improve using the new data in the second cloud. For instance, gradients of deep learning models can be transferred by Swarm.
□ Adversarial generation of gene expression data
>> https://academic.oup.com/bioinformatics/advance-article-abstract/doi/10.1093/bioinformatics/btab282/6278292
This model preserves several gene expression properties significantly better than widely used simulators such as SynTReN or GeneNetWeaver.
it exhibits real gene clusters and ontologies both at local and global scales, suggesting that the model learns to approximate the gene expression manifold in a biologically meaningful way.
□ XGraphBoost: Extracting Graph Neural Network-Based Features for a Better Prediction of Molecular Properties
>> https://pubs.acs.org/doi/10.1021/acs.jcim.0c01489
XGBOOST is an algorithm combining GNN and XGBOOST, which can introduce the machine learning algorithm XGBOOST under the existing GNN network architecture to improve the algorithm capability.The GNN used in this paper includes DMPNN, GGNN and GCN.
the integrated framework XGraphBoost extracts the features using a GNN and build an accurate prediction model of molecular properties using the classifier XGBoost. The XGraphBoost framework fully inherits the merits of the GNN-based automatic molecular feature extraction.
□ Caution against examining the role of reverse causality in Mendelian Randomization
>> https://onlinelibrary.wiley.com/doi/10.1002/gepi.22385
the MR Steiger approach may fail to correctly identify the direction of causality. This is true, especially in the presence of pleiotropy.
reverseDirection which runs simulations for user-specified scenarios to examine when the MR Steiger approach can correctly determine the causal direction between two phenotypes in any user specified scenario.
□ Robust Inference for Mediated Effects in Partially Linear Models
>> https://link.springer.com/article/10.1007/s11336-021-09768-z
G-estimators for the direct and indirect effects and demonstrate consistent asymptotic normality for indirect effects when models for the conditional means of M or X/Y are correctly specified, and for direct effects, when models for the conditional means of Y, or X/M are correct.
the GMM-based tests perform better in terms of power and small sample performance compared with traditional tests in the partially linear setting, with drastic improvement under model misspecification.
□ ARAMIS: From systematic errors of NGS long reads to accurate assemblies
>> https://academic.oup.com/bib/advance-article/doi/10.1093/bib/bbab170/6278148
Within the hybrid methodologies, there are two main approaches: alignment of short reads to long reads using a variety of aligners to achieve maximum accuracy (e.g., HECIL); or to perform firstly an assembly with short reads and then to align against it the long reads to correct them (e.g., HALC).
Accurate long-Reads Assembly correction Method for Indel errorS (ARAMIS), the first NGS long-reads indels correction pipeline that combines several correction software in just one step using accurate short reads.
□ Superscan: Supervised Single-Cell Annotation
>> https://www.biorxiv.org/content/10.1101/2021.05.20.445014v1.full.pdf
Superscan (Supervised Single-Cell Annotation): a supervised classification approach built around a simple XGBoost model trained on manually labelled data.
Superscan aims to reach high overall performance across a range of datasets by including a large collection of training data. This is in contrast to a method like CaSTLE, which also employs an XGBoost model but requires specification of a sufficiently similar pre-labeled dataset.

□ Kmerator Suite: design of specific k-mer signatures and automatic metadata discovery in large RNA-Seq datasets.
>> https://www.biorxiv.org/content/10.1101/2021.05.20.444982v1.full.pdf
The core tool, Kmerator, produces specific k-mers for 97% of human genes, enabling the measure of gene expression with high accuracy in simulated datasets.
KmerExploR, a direct application of Kmerator, uses a set of predictor genes specific k-mers to infer metadata including library protocol, sample features or contaminations from RNA-seq datasets.
□ ccdf: Distribution-free complex hypothesis testing for single-cell RNA-seq differential expression analysis
>> https://www.biorxiv.org/content/10.1101/2021.05.21.445165v1.full.pdf
ccdf tests the association of each gene expression with one or many variables of interest (that can be either continuous or discrete), while potentially adjusting for additional covariates.
To test such complex hypotheses, ccdf uses a conditional independence test relying on the conditional cumulative distribution function, estimated through multiple regressions.

□ EM-MUL: An effective method to resolve ambiguous bisulfite-treated reads
>> https://bmcbioinformatics.biomedcentral.com/articles/10.1186/s12859-021-04204-6
EM-MUL not only rescues multireads overlapped with unique reads, but also uses the overall coverage and accurate base-level alignment to resolve multireads that cannot be handled by current methods.
The EM-MUL method can align partial BS-reads to the repeated regions, which is beneficial to the further analysis of the repeated regions.

□ Vulcan: Improved long-read mapping and structural variant calling via dual-mode alignment
>> https://www.biorxiv.org/content/10.1101/2021.05.29.446291v1.full.pdf
Vulcan leverages the computed normalized edit distance of the mapped reads via e.g. minimap2 to identify poorly aligned reads and realigns them using the more accurate yet computationally more expensive long read mapper.
Vulcan runs up to 4X faster than NGMLR alone and produces lower edit distance alignments than minimap2, on both simulated and real datasets. Vulcan could be used for any combination of long-read mappers that output the edit distance (NM tag) directly within sam/bam file output.
□ findere: fast and precise approximate membership query
>> https://www.biorxiv.org/content/10.1101/2021.05.31.446182v1.full.pdf
findere is a simple strategy for speeding up queries and for reducing false positive calls from any Approximate Membership Query data structure (AMQ). With no drawbacks, queries are two times faster with two orders of magnitudes less false positive calls.
The findere implementation proposed here uses a Bloom filter as AMQ. It proposes a way to index and query Kmers from biological sequences (fastq or fasta, gzipped or not, possibly considering only canonical Kmers) or from any textual data.
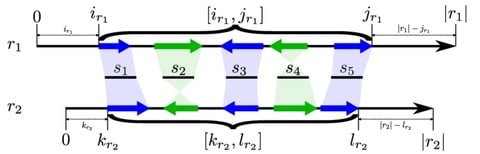
□ LazyB: fast and cheap genome assembly
>> https://almob.biomedcentral.com/articles/10.1186/s13015-021-00186-5
LazyB starts from a bipartite overlap graph between long reads and restrictively filtered short-read unitigs. This graph is translated into a long-read overlap graph G.
Instead of the more conventional approach of removing tips, bubbles, and other local features, LazyB stepwisely extracts subgraphs whose global properties approach a disjoint union of paths.

□ DIMA: Data-Driven Selection of an Imputation Algorithm
>> https://pubs.acs.org/doi/10.1021/acs.jproteome.1c00119
DIMA can take a numeric matrix or the file path to a MaxQuant ProteinGroups file as an input. The data is reduced to the columns which include pattern in their sample names.
DIMA reliably suggests a high-performing imputation algorithm, which is always among the three best algorithms and results in a root mean square error difference (ΔRMSE) ≤ 10% in 80% of the cases.

□ scRegulocity: Detection of local RNA velocity patterns in embeddings of single cell RNA-Seq data
>> https://www.biorxiv.org/content/10.1101/2021.06.01.446674v1.full.pdf
scRegulocity focuses on velocity switching patterns, local patterns where velocity of nearby cells change abruptly. These different transcriptional dynamics patterns can be indicative of transitioning cell states.
scRegulocity annotates these patterns with genes and enriched pathways and also analyzes and visualizes the velocity switching patterns at the regulatory network level. scRegulocity also combines velocity estimation, pattern detection and visualization steps.
□ Optimizing Network Propagation for Multi-Omics Data Integration
>> https://www.biorxiv.org/content/10.1101/2021.06.10.447856v1.full.pdf
Random Walk with Restart (RWR) and Heat Diffusion has revealed specific characteristics of the algorithms. Optimal parameters could also be obtained by either maximizing the agreement between different omics layers or by maximizing the consistency between biological replicates.
□ The reciprocal Bayesian LASSO
>> https://onlinelibrary.wiley.com/doi/10.1002/sim.9098
BayesRecipe includes a set of computationally efficient MCMC algorithms for solving the Bayesian reciprocal LASSO in linear models. It also includes a modified S5 algorithm to solve the reduced reciprocal LASSO problem in linear regression.
a fully Bayesian formulation of the rLASSO problem, which is based on the observation that the rLASSO estimate for linear regression parameters can be interpreted as a Bayesian posterior mode estimate when the regression parameters are assigned independent inverse Laplace priors.
※コメント投稿者のブログIDはブログ作成者のみに通知されます