
We do not know about death, because we never know about life.
機能としてのOrganum(器官)は、Organism(全体構造)を網羅する動的反応経路の局在性を表象する概念に過ぎない。失われた反応経路のエンタングルメントの複雑性は保存され、深い洞察を依代として違う道が見出される。
□ PathRacer: racing profile HMM paths on assembly graph:
>> https://www.biorxiv.org/content/biorxiv/early/2019/02/27/562579.full.pdf
PathRacer is a novel standalone tool that aligns profile HMM directly to the assembly graph (performing the codon translation on fly for amino acid pHMMs) by modern assemblers in standard Graphical Fragment Assembly (GFA) format. PathRacer provides the set of most probable paths traversed by a HMM through the whole assembly graph, regardless whether the sequence of interested is encoded on the single contig or scattered across the set of edges, therefore significantly improving the recovery of sequences of interest even from fragmented metagenome assemblies.
□ The complexity dividend: when sophisticated inference matters:
>> https://www.biorxiv.org/content/biorxiv/early/2019/02/28/563346.full.pdf
constructing a hierarchy of strategies that vary in complexity between these limits and find a power law of diminishing returns: increasing complexity gives progressively smaller gains in accuracy. Moreover, the rate at which the gain decrements depends systematically on the statistical uncertainty in the world, such that complex strategies do not provide substantial benefits over simple ones when uncertainty is too high or too low. In between, when the world is neither too predictable nor too unpredictable, there is a complexity dividend.


□ Accurate inference of tree topologies from multiple sequence alignments using deep learning:
>> https://www.biorxiv.org/content/biorxiv/early/2019/02/24/559054.full.pdf
a deep convolutional neural network (CNN) to infer quartet topologies from multiple sequence alignments. This CNN can readily be trained to make inferences using both gapped and ungapped data. The confidence scores produced by this CNN can more accurately assess support for the chosen topology than bootstrap and posterior probability scores. This approach is highly accurate and is remarkably robust to bias-inducing regions of parameter space such as the Felsenstein zone and the Farris zone.
□ Omnipresent Maxwell's demons orchestrate information management in living cells:
>> https://onlinelibrary.wiley.com/doi/full/10.1111/1751-7915.13378
A core gene set encoding these functions belongs to the minimal genome required to allow the construction of an autonomous cell. These Maxwell's demon (MxD) allow the cell to perform computations in an energy‐efficient way that is vastly better than our contemporary computers.
□ synder: inferring genomic orthologs from synteny maps:
>> https://www.biorxiv.org/content/biorxiv/early/2019/02/19/554501.full.pdf
Ortholog inference is a key step in understanding the evolution and function of a gene or other genomic feature. Yet often no similar sequence can be identified, or the true ortholog is hidden among false positives. A solution is to consider the sequence’s genomic context. synder is the generic program for tracing features of interest between genomes based on a synteny map.

□ SViCT: Structural variation and fusion detection using targeted sequencing data from circulating cell free DNA:
>> https://academic.oup.com/nar/advance-article/doi/10.1093/nar/gkz067/5316733
SViCT can detect breakpoints and sequences of various structural variations including deletions, insertions, inversions, duplications and translocations. SViCT extracts discordant read pairs, one-end anchors and soft-clipped/split reads, assembles them into contigs, and re-maps contig intervals to a reference genome using an efficient k-mer indexing approach. The intervals are then joined using a combination of graph and greedy algorithms to identify specific structural variant signatures.

□ An MCMC-based method for Bayesian inference of natural selection from time series DNA data across linked loci:
>> https://www.biorxiv.org/content/biorxiv/early/2019/02/27/562967.full.pdf
This approach relies on a hidden Markov model incorporating the two-locus Wright-Fisher diffusion with selection, which enables us to explicitly model genetic recombination. The posterior probability distribution for selection coefficients is obtained by using the particle marginal Metropolis-Hastings algorithm, which also allows for co-estimation of the population haplotype frequency trajectories.
□ SCANVIS: a tool for SCoring, ANnotating and VISualing splice junctions:
>> https://www.biorxiv.org/content/biorxiv/early/2019/02/14/549261.full.pdf
SCANVIS generates reasonable PSI scores by demonstrating that tissue/cancer types in GTEX and TCGA are well separated and easily predicted from a few thousand SJs. SCANVIS scores and annotates SJs with attention to many details that can assist in the inference of any downstream consequences. It runs quite efficiently.


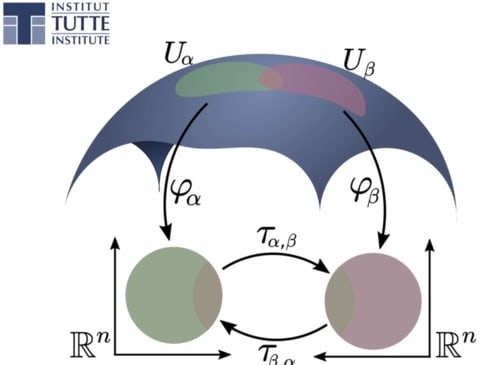
□ Learning Topology: topological methods for unsupervised learning:
>> https://speakerdeck.com/lmcinnes/learning-topology-topological-methods-for-unsupervised-learning
"Topology gives a different way on classical problems. Dimensional Reduction, Clustering, Anomaly Detection, everything is in one pocket."
Data is uniformly distributed on the manifold. Define a Riemannian metric on the manifold to make this assumption true. Topology and category theory provide a different language to frame problems.
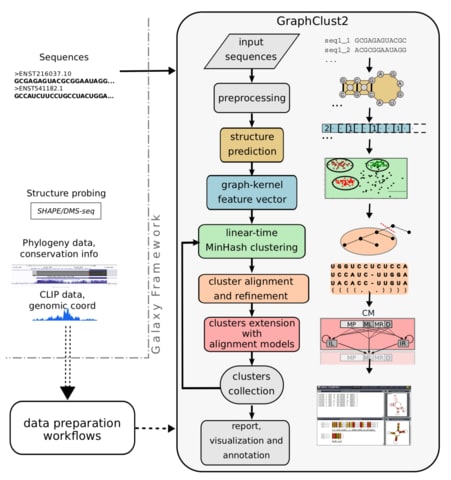
□ GraphClust2: Empowering the annotation and discovery of structured RNAs with scalable and accessible integrative clustering:
>> https://www.biorxiv.org/content/biorxiv/early/2019/02/20/550335.full.pdf
The GraphClust methodology uses a graph kernel approach to integrate both sequence and structure information into high-dimensional sparse feature vectors. hese vectors are then rapidly clustered, with a linear-time complexity over the number of sequences, using a locality sensitive hashing technique.
The linear-time alignment-free methodology of GraphClust2 , accompanied by cluster refinement and extension using RNA comparative methods and structure probing data.
□ BRIDGE: Biomolecular Reaction & Interaction Dynamics Global Environment:
>> https://academic.oup.com/bioinformatics/advance-article-abstract/doi/10.1093/bioinformatics/btz107/5317160
the foundations of BRIDGE developed on the Galaxy platform that makes possible fundamental molecular dynamics of proteins through workflows and pipelines via commonly used packages such as NAMD, GROMACS and CHARMM. BRIDGE can be used to set up and simulate biological macromolecules, perform conformational analysis from trajectory data and conduct data analytics of large scale protein motions using statistical rigor.

□ KrakenLinked: Novel Algorithms for the Taxonomic Classification of Metagenomic Linked Reads:
>> https://www.biorxiv.org/content/biorxiv/early/2019/02/14/549667.full.pdf
If reads from the same long fragment of DNA are identified via assembly (e.g. Athena) or by barcode deconvolution (e.g. Minerva) the markers from both reads could be used together for taxonomic classification. Read-clouds provide useful additional taxonomic information even without clearly identifying which reads originated from the same fragment. A purpose built Linked-Read taxonomic classifier could search for a parsimonious set of taxonomic assignments for each read cloud.

□ Highly Efficient Hypothesis Testing Methods for Regression-type Tests with Correlated Observations and Heterogeneous Variance Structure:
>> https://www.biorxiv.org/content/biorxiv/early/2019/02/15/552331.full.pdf
As fast and numerically stable replacements for the weighted LMER test, the PB-transformed tests are especially suitable for “messy” high-throughput data that include both independent and matched/repeated samples. By using this method, the practitioners no longer have to choose between using partial data or ignoring the correlation in the data.

□ IRIS-EDA: An integrated RNA-Seq interpretation system for gene expression data analysis:
>> https://journals.plos.org/ploscompbiol/article?id=10.1371/journal.pcbi.1006792
IRIS-EDA, which is a Shiny web server for expression data analysis. Seven discovery-driven methods (correlation analysis, heatmap, clustering, biclustering, Principal Component Analysis, Multidimensional Scaling, and t-SNE are provided for gene expression exploration. IRIS-EDA provides a framework to expedite submission of data and results to NCBI’s Gene Expression Omnibus following the FAIR (Findable, Accessible, Interoperable and Reusable) Data Principles.

□ EpiAlignment: alignment with both DNA sequence and epigenomic data:
>> https://www.biorxiv.org/content/biorxiv/early/2019/02/27/562199.full.pdf
EpiAlignment takes DNA sequence and epigenomic profiles derived by ChIP- seq, DNase-seq, or ATAC-seq from two species as input data, and outputs the best semi-global alignments. These alignments are based on EpiAlignment scores, computed by a dynamic programming algorithm that accounts for both sequence alignment and epigenome similarity.
□ Novel-X: Detection and assembly of novel sequence insertions using Linked-Read technology:
>> https://www.biorxiv.org/content/biorxiv/early/2019/02/15/551028.full.pdf
Novel-X detects and genotypes novel sequence insertions in 10X sequencing dataset using non-trivial read alignment signatures and barocde information. In the case of SuperNova assembler, user has no control for any assembler parameters & default parameters are maximally tuned for whole human genome diploid assembly. A future direction would be to develop a specific local assembler designed for SV detection in Linked-Read data.
□ ReorientExpress: Transcriptome long-read orientation with Deep Learning:
>> https://github.com/comprna/reorientexpress
ReorientExpress is a tool to predict the orientation of cDNA reads from error-prone long-read sequencing technologies. It was developed with the aim to orientate nanopore long-reads from unstranded cDNA libraries without the need of a genome or transcriptome reference, but it is applicable to any set of long-reads. It builds kmer-based models using Deep Neural Networks using as training input a transcriptome annotation or any other fasta/fasq file for which the sequences orientation is known.
□ High-Fidelity Nanopore Sequencing of Ultra-Short DNA Sequences:
>> https://www.biorxiv.org/content/biorxiv/early/2019/02/16/552224.full.pdf
a nanopore-based sequencing strategy in which short target sequences are first circularized and then amplified via rolling-circle amplification to produce long stretches of concatemeric repeats. These can be sequenced on the Oxford Nanopore Technology’s (ONT) MinION platform, and the resulting repeat sequences aligned to produce a highly-accurate consensus that reduces the high error-rate present in the individual repeats.
□ Fastq-pair: efficient synchronization of paired-end fastq files:
>> https://www.biorxiv.org/content/biorxiv/early/2019/02/19/552885.full.pdf
The algorithm creates a hash of objects that contain the sequence identifier and the position in the file for all the identifiers in the first file. This significantly reduces the memory requirements for fastq-pair compared to trivial solutions that store sequence identifier. fastq-pair provides a rapid and memory efficient solution, written in C for portability, that synchronizes paired-end fastq files for subsequent analysis and places unmatched reads into singleton files.
□ Xeno Nucleic Acids (XNAs) Revisted:
>> http://zon.trilinkbiotech.com/2019/02/19/xeno-nucleic-acids-xnas-revisted/
X (‘Xeno’) in XNAs Are Structurally Strange Congeners of DNA or RNA
Xenobiology Is an Emerging and Intriguing New Frontier
□ Hachimoji DNA and RNA: A genetic system with eight building blocks:
>> http://science.sciencemag.org/content/363/6429/884
DNA- and RNA-like systems built from eight nucleotide “letters” (hence the name “hachimoji”) that form four orthogonal pairs. Has normal A, T, G, and C, plus new bases of B, Z, P, and S. 8-letter DNA can also be transcribed using an engineered T7 RNA polymerase.
These synthetic systems meet the structural requirements needed to support Darwinian evolution, including a polyelectrolyte backbone, predictable thermodynamic stability, and stereoregular building blocks that fit a Schrödinger aperiodic crystal.
□ A FUBINI RULE FOR ∞-COENDS:
>> https://arxiv.org/pdf/1902.06086.pdf
a Fubini rule for ∞-co/ends of ∞-functors F : Co × C → D. This allows to lay down “integration rules”, similar to those in classical co/end calculus, also in the setting of ∞-categories. the definition of twisted arrow ∞-category of an ∞-category; in this paves the way to the definition of co/end for a ∞-functor F : Co × C → D. in the ∞-category of spaces, the object (C,C′)/Σ exhibits the same universal property of MapC(C,C′).

□ Unbiased estimation of linkage disequilibrium from unphased data:
>> https://www.biorxiv.org/content/biorxiv/early/2019/02/22/557488.1.full.pdf
an approach to compute unbiased estimators for a broad set of two-locus statistics, for either phased or unphased data. This includes commonly used statistics, such as D and D2, the additional statistics in the Hill-Robertson system (D(1 − 2p)(1 − 2q) and p(1 − p)q(1 − q)), and, in general, any statistic that can be expressed as a polynomial in haplotype frequencies (f’s) or in terms of p, q, and D.
□ Latent Space Phenotyping: Automatic Image-Based Phenotyping for Treatment Studies:
>> https://www.biorxiv.org/content/biorxiv/early/2019/02/22/557678.full.pdf
Beyond computational requirements, another limitation of the method is a substantial difference in interpretability compared to GWAS using standard image-based phenotyping techniques. the measured phenotype can be directly interpreted as the biomass of the sample and QTL can be found which correlate with the effect of the treatment on biomass. candidate loci obtained through Latent Space Phenotyping must be interpreted differently.

□ Cooler: scalable storage for Hi-C data and other genomically-labeled arrays:
>> https://www.biorxiv.org/content/biorxiv/early/2019/02/22/557660.full.pdf
Cooler is a sparse data model and file format for genomically-labelled arrays with minimal redundancy but enough flexibility to support a wide range of data types, data sizes and future metadata requirements. A sparse representation in particular is crucial for developing robust tools and algorithms for use on increasingly high-resolution multi-dimensional genomic data sets that need to operate on subsets of data at a time.
□ A semi-parametric Bayesian approach, iSBA, for differential expression analysis of RNA-seq data:
>> https://www.biorxiv.org/content/biorxiv/early/2019/02/22/558270.full.pdf
modeling the RNA-seq count data with a Poisson-Gamma mixture model, and propose a Bayesian mixture modeling procedure with a Dirichlet process as the prior model for the distribution of fold changes between the two treatment means. Markov chain Monte Carlo (MCMC) posterior simulation using Metropolis Hastings algorithm to generate posterior samples for differential expression analysis while controlling false discovery rate.
□ CHETAH: a selective, hierarchical cell type identification method for single-cell RNA sequencing:
>> https://www.biorxiv.org/content/biorxiv/early/2019/02/22/558908.full.pdf
CHETAH (CHaracterization of cEll Types Aided by Hierarchical clustering) is an accurate cell type identification algorithm that is rapid and selective, including the possibility of intermediate or unassigned categories. For cells of an unknown type, CHETAH is more selective, yielding a classification that is as fine-grained as is justified by the available data.

□ SCIRA: Leveraging high-powered RNA-Seq datasets to improve inference of regulatory activity in single-cell RNA-Seq data:
>> https://www.biorxiv.org/content/biorxiv/early/2019/02/22/553040.full.pdf
SCIRA (Single Cell Inference of Regulatory Activity), which leverages the power of large-scale bulk RNA-Seq datasets to infer high-quality tissue-specific regulatory networks, from which regulatory activity estimates in single cells can be subsequently obtained.
Power-calculation for SCIRA/SEPIRA: the strategy to estimate the sensitivity of SEPIRA/SCIRA to detect highly expressed cell-type specific TFs in a given tissue, as a function of the corresponding cell-type proportion in the tissue.

□ AKRON: Approximate kernel reconstruction for time-varying networks:
>> https://biodatamining.biomedcentral.com/track/pdf/10.1186/s13040-019-0192-1
AKRON supersedes the Lasso regularization by starting from the Lasso-Kalman inferred network and judiciously searching the space for a sparser solution that is more representative of the ground truth. The results demonstrate that the proposed approach is better at recovering sparse time-varying networks than l1-KF. Not only was the reconstruction error of the proposed approach lower than l1-KF, but it was also better at detecting whether an edge exists in a network.

□ ACValidator: a novel assembly-based approach for in silico validation of circular RNAs:
>> https://www.biorxiv.org/content/biorxiv/early/2019/02/21/556597.full.pdf
ACValidator is a bioinformatics approach to perform in silico validation of selected circular RNA junction(s). ACValidator operates in three phases: extraction of reads from SAM file, generation of a “pseudo-reference” file, and assembly and alignment of extracted reads. ACValidator extracts reads from a user-defined window on either side of the circRNA junction and assembles them to generate contigs. These contigs are aligned against the circRNA sequence to find contigs spanning the back-spliced junction.
□ CausalTAB: the PSI-MITAB 2.8 updated format for signalling data representation and dissemination:
>> https://academic.oup.com/bioinformatics/advance-article-abstract/doi/10.1093/bioinformatics/btz132/5355053
a common standard for the representation and dissemination of signalling information: the PSI Causal Interaction tabular format (CausalTAB) which is an extension of the existing PSI-MI tab-delimited format, now designated PSI-MITAB 2.8. As the causal regulatory mechanism is taken from the PSI-MI ontology, the database name is 'psi-mi'. When the ‘Interaction Types’ column is equal to the psi-mi term: psi-mi:"MI:2286", then the causal regulatory mechanism must be absolutely filled - otherwise it can be empty.

□ The Linked Selection Signature of Rapid Adaptation in Temporal Genomic Data:
>> https://www.biorxiv.org/content/biorxiv/early/2019/02/24/559419.full.pdf
develop theory that predicts the magnitude of these temporal autocovariances, showing that it is determined by the level of additive genetic variation, recombination, and linkage disequilibria in a region. Furthermore, by using analytic expressions for the temporal variances and autocovariances in allele frequency, demonstrate one can estimate the additive genetic variation for fitness and the drift-effective population size from temporal genomic data.
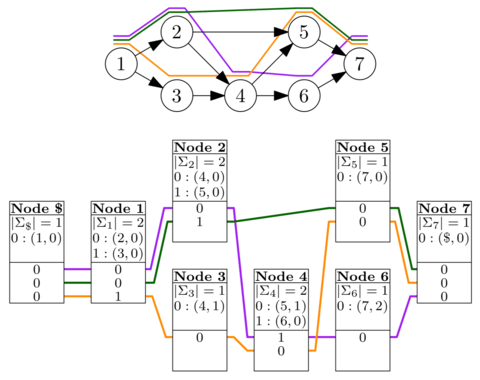
□ Haplotype-aware graph indexes:
>> https://www.biorxiv.org/content/biorxiv/early/2019/02/24/559583.full.pdf
GBWT, a scalable implementation of the graph extension of the positional Burrows–Wheeler transform, to store the haplotypes as paths in the graph. Haplotypes are essentially sequences of nodes in the variation graph, and GBWT is best seen as the multi-string BWT of the node sequences. VG graphs are connected, we can use the long-range information in the GBWT for mapping long reads and paired-end reads.
□ FQSqueezer: k-mer-based compression of sequencing data:
>> https://www.biorxiv.org/content/biorxiv/early/2019/02/24/559807.full.pdf
QSqueezer, a novel compression algorithm for sequencing data able to process single- and paired-end reads of variable lengths. It is based on the ideas from the famous prediction by partial matching and dynamic Markov coder algorithms.
□ Massive computational acceleration by using neural networks to emulate mechanism-based biological models:
>> https://www.biorxiv.org/content/biorxiv/early/2019/02/24/559559.full.pdf
With this framework, we can predict not only the 1-D distribution in space (partial differential equation models) and probability density function (stochastic differential equation models) of variables of interest with high accuracy, but also novel system dynamics not present. the NN is more likely to fail when the system output is highly sensitive to local parameter variations, i.e., where the output landscape is rugged in the parametric space.
□ SCINA: Semi-Supervised Analysis of Single Cells in silico:
>> https://www.biorxiv.org/content/biorxiv/early/2019/02/25/559872.full.pdf
SCINA, a semi-supervised model, for analyses of scRNA-Seq and flow cytometry/CyTOF data, and other data of similar format, by automatically exploiting previously established gene signatures using an expectation–maximization (EM) algorithm. The average correct assignment probability of the total of 2000 cells was 98.44% for SCINA, 52.50% for KC, 52.72% for SINCERA and 18.46% for PhenoGraph, which indicated that SCINA achieved a much more stable performance than unsupervised clustering.

□ A component overlapping attribute clustering (COAC) algorithm for single-cell RNA sequencing data analysis and potential pathobiological implications:
>> https://journals.plos.org/ploscompbiol/article?id=10.1371/journal.pcbi.1006772
a network-based approach, termed Component Overlapping Attribute Clustering (COAC), to infer novel gene-gene subnetworks in individual components (subsets of whole components) representing multiple cell types and phases of scRNA-seq data. COAC can reduce batch effects and identify specific cell types in two large-scale human scRNA-seq datasets.

□ Graph Peak Caller: Calling ChIP-seq peaks on graph-based reference genomes:
>> https://journals.plos.org/ploscompbiol/article?id=10.1371/journal.pcbi.1006731
Graph Peak Caller is based on the same principles used by MACS2, and is able to call peaks with or without a set of control alignments. For the case of a graph that merely reflects a linear reference genome, our peak-caller produces the same results as MACS2.
□ R-DECO: An open-source Matlab based graphical user interface for the detection and correction of R-peaks:
>> https://www.biorxiv.org/content/biorxiv/early/2019/02/25/560706.full.pdf
Within R-DECO, the R-peaks are detected by an adaptation of the Pan-Tompkins algorithm. Instead of using all the pre-processing steps of the latter algorithm, the proposed algorithm uses only an envelope-based procedure to flatten the ECG and enhance the QRS-complexes.
□ DeepSV: Accurate calling of genomic deletions from high throughput sequencing data using deep convolutional neural network:
>> https://www.biorxiv.org/content/biorxiv/early/2019/02/26/561357.full.pdf
DeepSV is based on a novel method of visualizing sequence reads. The visualization is designed to capture multiple sources of information in the sequence data that are relevant to long deletions.
□ ANEVA-DOT prioritized a gene in a patient that led to a new diagnosis. A pseudoexon insertion caused by an intronic variant that resulted in NMD. continue incorporating it into the Mendelian RNA-seq workflow.
ANEVA estimates of regulatory variation correlate with other estimates of constraint (GTEx v7 data, 10k RNA-seq samples spanning 48 tissues and 620 individuals, total 14k genes used, mostly protein-coding genes) #grd19
※コメント投稿者のブログIDはブログ作成者のみに通知されます