□ Beyond low-Earth orbit: Characterizing the immune profile following simulated spaceflight conditions for deep space missions
>> https://www.cell.com/iscience/fulltext/S2589-0042(20)30944-5
Circulating immune biomarkers are defined by distinct deep space irradiation types coupled to simulated microgravity and could be targets for future space health initiatives.
Unique immune signatures and microRNA (miRNA) profiles would be produced by distinct experimental conditions of simulated GCR, SPE, and gamma irradiation, singly or in combination with HU.
Linear energy transfer (LET) is defined as the amount of energy that is deposited or transferred in a material from an ion. High-LET irradiation can cause more damaging ionizing tracks and pose a higher relative biological effectiveness (RBE) risk compared to low-LET irradiation.

□ Advancing the Integration of Biosciences Data Sharing to Further Enable Space Exploration
>> https://www.cell.com/cell-reports/fulltext/S2211-1247(20)31430-3
This open access science perspective invites investigators to participate in a transformative collaborative effort for interpreting spaceflight effects by integrating omics and physiological data to the systems level.
Integration of data from GeneLab and ALSDA will enable spaceflight health risk modeling. All data would then benefit from applied FAIR principles.

□ Super-robust data storage in DNA by de Bruijn graph-based decoding
>> https://www.biorxiv.org/content/10.1101/2020.12.20.423642v1.full.pdf
De Bruijn Graph-based Greedy Path Search (DBG-GPS) algorithm can efficient reconstruction of DNA strands from multiple error-rich sequences directly.
DBG-GPS is designed as inner decoding mechanism for correction of errors within DNA strands. And shows 50 times faster than the clustering and multiple alignment-based methods. The revealed linear decoding complexity makes DBG-GPS a suitable solution for large-scale data storage.

□ STARRPeaker: uniform processing and accurate identification of STARR-seq active regions
>> https://genomebiology.biomedcentral.com/articles/10.1186/s13059-020-02194-x
STARRPeaker, an algorithm optimized for processing and identifying functionally active enhancers from STARR-seq data. This approach statistically models the basal level of transcription, accounting for potential confounding factors, and accurately identifies reproducible enhancers.
To model the fragment coverage from STARR-seq using discrete probability distribution, assuming each genomic bin is independent, as specified in the Bernoulli trials. STARRPeaker calculates fragment coverage and the basal transcription rate using negative binomial regression.

□ RedOak: a reference-free and alignment-free structure for indexing a collection of similar genomes
>> https://www.biorxiv.org/content/10.1101/2020.12.19.423583v1.full.pdf
The parallelization of the data structure construction allows, through the use of networking resources, to efficiently index and query those genomes. RedOak is inspired by Bloom Filter Trie, using a probabilistic approach.
RedOak can also be applied to reads from unassembled genomes, and it provides a nucleotide sequence query function. This software is based on a k-mer approach and has been developed to be heavily parallelized and distributed on several nodes of a cluster.
□ TAPER: Pinpointing errors in multiple sequence alignments despite varying rates of evolution
>> https://www.biorxiv.org/content/10.1101/2020.11.30.405589v1.full.pdf
a strategy to combine several k values, each with a different p, q setting. And run the 2D outlier algorithm on multiple k values and report their union.
TAPER, Two-dimensional Algorithm for Pinpointing ERrors that takes a multiple sequence alignment as input and outputs outlier sequence positions. TAPER is able to pinpoint errors in multiple sequence alignments without removing large parts of the alignment.
□ WENGAN: Efficient hybrid de novo assembly of human genomes
>> https://www.nature.com/articles/s41587-020-00747-w
WENGAN, a hybrid genome assembler that, unlike most long-read assemblers, entirely avoids the all-versus-all read comparison, does not follow the OLC paradigm and integrates short reads in the early phases of the assembly process (short-read-first).
WENGAN starts by building short-read contigs using a de Bruijn graph assembler. Then, the pair-end reads are pseudo-aligned back to detect and error-correct chimeric contigs as well as to classify them as repeats or unique sequences.
Wengan builds a new sequence graph called the Synthetic Scaffolding Graph. The SSG is built from a spectrum of synthetic mate-pair libraries extracted from raw long-reads. Longer alignments are then built by peforming a transitive reduction of the edges.
□ Learning interpretable latent autoencoder representations with annotations of feature sets
>> https://www.biorxiv.org/content/10.1101/2020.12.02.401182v1.full.pdf
In f-scLVM, deterministic approximate Bayesian inference based on variational methods is used to approximate the posterior over all random variables of the model.
a scalable alternative to f-scLVM to learn latent representations of single-cell RNA-seq data that exploit prior knowledge such as Gene Ontology, resulting in interpretable factors.
□ FastK: A K-mer counter for HQ assembly data sets
>> https://github.com/thegenemyers/FASTK
FastK is a k-mer counter that is optimized for processing high quality DNA assembly data sets such as those produced with an Illumina instrument or a PacBio run in HiFi mode.
FastK is about 2 times faster than KMC3 when counting 40-mers in a 50X HiFi data set. Its relative speedup decreases with increasing error rate or increasing values of k, but regardless is a general program that works for any DNA sequence data set and choice of k.

□ Andrew Carroll
>> https://github.com/google/deepvariant/releases/tag/v1.1.0
Release of DeepVariant v1.1: Introducing DeepTrio, with greater accuracy for trio or duos. Pre-trained models for Illumina WGS, WES, and PacBio HiFi. Also in DV1.1 (non-trio_, better speed for long reads. 21% reduction in PacBio Indel Errors.
□ Coupled co-clustering-based unsupervised transfer learning for the integrative analysis of single-cell genomic data
>> https://academic.oup.com/bib/advance-article-abstract/doi/10.1093/bib/bbaa347/6024740
Clustering similar genomic features reduces the noise in single-cell data and facilitates transfer of knowledge across single-cell datasets.
coupleCoC builds upon the information theoretic co-clustering framework. In co-clustering, both the cells and the genomic features are simultaneously clustered.
□ GeneTerpret: a customizable multilayer approach to genomic variant prioritization and interpretation
>> https://www.biorxiv.org/content/10.1101/2020.12.04.408336v1.full.pdf
GeneTerpret platform collates data from current interpretation tools and databases, and applies a phenotype-driven query to categorize the variants identified in a given genome.
GeneTerpret improves the GVI process. GeneTerpret is encouragingly accurate when compared with expert-curated datasets in such well- established public records of clinically relevant variants as DECIPHER and ClinGen.
□ Selective Inference for Hierarchical Clustering
>> https://arxiv.org/pdf/2012.02936.pdf
a selective inference framework to test for a difference in means after any type of clustering. This framework exploits ideas from the recent literature on selective inference for regression and changepoint detection.
This framework avoids the need for bootstrap resampling and provides exact finite-sample inference for the difference in means between a single pair of estimated clusters.

□ multiGSEA: a GSEA-based pathway enrichment analysis for multi-omics data
>> https://bmcbioinformatics.biomedcentral.com/articles/10.1186/s12859-020-03910-x
multiGSEA, a highly versatile tool for multi-omics pathway integration that minimizes previous restrictions in terms of omics layer selection and the mapping of feature IDs. Pathway definitions can be downloaded from up to 8 different pathway databases by means of the graphite.
multiGSEA utilizes three different p value combination methods. By default, combinePvalues() will apply the Z-method or Stouffer’s method which has no bias towards small or large p values.

□ Giraffe: Genotyping common, large structural variations in 5,202 genomes using pangenomes, the Giraffe mapper, and the vg toolkit
>> https://www.biorxiv.org/content/10.1101/2020.12.04.412486v1.full.pdf
Giraffe, a new pangenome mapper that focuses on mapping to collections of aligned haplotypes. Giraffe is a short read to graph mapper designed to map to haplotypes, producing alignments embedded within a sequence graph.
The Giraffe algorithm can only find a correct mapping if the read contains instances of minimizers that exactly match minimizers in the true placement in the graph, which then form a cluster, which is then extended to produce an alignment.
□ FEATS: feature selection-based clustering of single-cell RNA-seq data
>> https://pubmed.ncbi.nlm.nih.gov/33285568/
FEATS, a univariate feature selection-based approach for clustering, which involves the selection of top informative features to improve clustering performance.
FEATS gives superior performance compared with the current tools, in terms of adjusted Rand index and estimating the number of clusters.

□ constclust: Consistent Clusters for scRNA-seq
>> https://www.biorxiv.org/content/10.1101/2020.12.08.417105v1.full.pdf
constclust is a novel meta-clustering method based on the idea that if the data contains distinct populations which a clustering method can identify, meaningful clusters should be robust to small changes in the parameters used to derive them.
constclust finds labels which match ground truth, so does running the underlying clustering method with default parameters. constclust formalizes the operations by automatically detecting the clusters which are consistently found within contiguous regions of parameter space.
□ Prioritizing genes for systematic variant effect mapping
>> https://academic.oup.com/bioinformatics/advance-article/doi/10.1093/bioinformatics/btaa1008/6029515
Missense VUS (variant of uncertain significance) collected through clinical testing were extracted from the ClinVar and Invitae databases. The first strategy ranked genes based on their unique VUS count.
The second strategy ranked genes based on their movability- and reappearance-weighted impact score(s) (MARWIS) to give extra weight to reappearing, movable VUS.
The third strategy ranked the genes by their difficulty-adjusted impact score(s) (DAIS), calculated to account for the costs associated with studying longer genes.

□ TrancriptomeReconstructoR: data-driven annotation of complex transcriptomes
>> https://www.biorxiv.org/content/10.1101/2020.12.10.418897v1.full.pdf
ONT Direct RNA-seq has four key limitations. First, up to 30-40% of bases can be called with errors. To tolerate the sequencing errors, the dedicated aligners allow for more mismatches and thus inevitably sacrifice the accuracy of alignments.
TranscriptomeReconstructoR takes three datasets as input: i) full-length RNA-seq (e.g. ONT Direct RNA-seq) to resolve splicing patterns; ii) 5' tag sequencing (e.g. CAGE-seq) to detect TSS; iii) 3' tag sequencing (e.g. PAT-seq) to detect polyadenylation sites (PAS).

□ HiddenVis: a Hidden State Visualization Toolkit to Visualize and Interpret Deep Learning Models for Time Series Data
>> https://www.biorxiv.org/content/10.1101/2020.12.11.422030v1.full.pdf
Hidden State Visualization Toolkit (HiddenVis) visualizes and facilitate the interpretations of sequential models for accelerometer data. HiddenVis can visualize the hidden states, match input samples with similar patterns and explore the potential relation among covariates.
The HiddenViz model is suitable for a wide range of Deep Learning based accelerometer data analyses. It can be easily extended to the visualization and analysis of other temporal data.

□ Unbiased integration of single cell multi-omics data
>> https://www.biorxiv.org/content/10.1101/2020.12.11.422014v1.full.pdf
bindSC, a single-cell data integration tool that realizes simultaneous alignment of the rows and the columns between data matrices without making approximations.
The alignment matrix derived from bi-CCA (bi-order canonical correlation analysis) can be utilized to derive in silico multiomics profiles from aligned cells. Bi-CCA outputs canonical correlation vectors (CCVs), which project cells from two datasets onto a shared latent space.
□ FFD: Fast Feature Detector
>> https://ieeexplore.ieee.org/document/9292438
The robust and accurate keypoints exist in the specific scale-space domain. And formulating the superimposition problem into a mathematical model and then derive a closed-form solution for multiscale analysis.
The model is formulated via difference-of-Gaussian (DoG) kernels in the continuous scale-space domain, and it is proved that setting the scale-space pyramid’s blurring ratio and smoothness to 2 and 0.627, respectively, facilitates the detection of reliable keypoints.
□ Cytosplore-Transcriptomics: a scalable inter-active framework for single-cell RNA sequenc-ing data analysis
>> https://www.biorxiv.org/content/10.1101/2020.12.11.421883v1.full.pdf
The two-dimensional embeddings of the HSNE hierarchy can be used to cluster and define cell populations at different levels of the hierarchy, or to visualize the expression of selected genes and metadata across cells.
Cytosplore-Transcriptomics, a framework to analyze scRNA-seq data. At its core, it uses a hierarchical, manifold preserving representation of the data that allows the inspection and annotation of scRNA-seq data at different levels of detail.
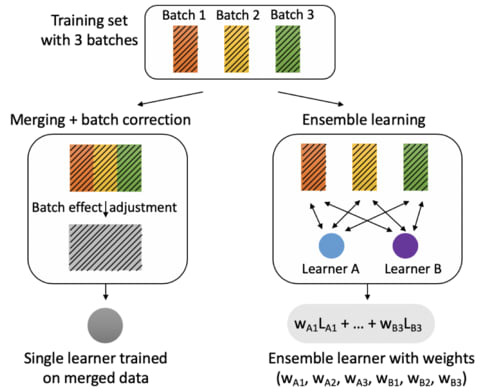
□ Robustifying genomic classifiers to batch effects via ensemble learning
>> https://academic.oup.com/bioinformatics/advance-article-abstract/doi/10.1093/bioinformatics/btaa986/6007261

□ Macarons: Uncovering complementary sets of variants for the prediction of quantitative phenotypes
>> https://www.biorxiv.org/content/10.1101/2020.12.11.419952v1.full.pdf
Macarons takes into account the correlations between SNPs to avoid the selection of redundant pairs of SNPs in linkage disequilibrium.
Macarons features two simple, interpretable parameters to control the time/performance trade-off: The number of SNPs to be selected (k), and maximum intra-chromosomal distance (D, in base pairs) to reduce the search space for redundant SNPs.

□ TraNCE: Scalable Analysis of Multi-Modal Biomedical Data
>> https://www.biorxiv.org/content/10.1101/2020.12.14.422781v1.full.pdf
TraNCE, a framework that automates the difficulties of designing distributed analyses with complex biomedical data types. TraNCE is capable of outperforming the common alternative, based on “flattening” complex data structures.
TraNCE is a compilation framework that transforms declarative programs over nested collections into distributed execution plans.
□ Hapo-G, Haplotype-Aware Polishing Of Genome Assemblies
>> https://www.biorxiv.org/content/10.1101/2020.12.14.422624v1.full.pdf
Hapo-G maintains two stacks of alignments, the first (all-ali) contains all the alignments that overlap the currently inspected base, and the second (hap-ali) contains only the read alignments that agree with the last selected haplotype.
Hapo-G selects a reference alignment and tries to use it as long as possible to polish the region where it aligns, which will minimize mixing between haplotypes.
□ AdRoit: an accurate and robust method to infer complex transcriptome composition
>> https://www.biorxiv.org/content/10.1101/2020.12.14.422697v1.full.pdf
AdRoit, an accurate and robust method to infer transcriptome composition. The method estimates the proportions of each cell type in the compound RNA-seq data using known single cell data of relevant cell types.
AdRoit uniquely uses an adaptive learning approach to correct the bias gene-wise. due to the difference in sequencing techniques. AdRoit also utilizes cell type specific genes while control their cross-sample variability.
□ DeMaSk: a deep mutational scanning substitution matrix and its use for variant impact prediction
>> https://academic.oup.com/bioinformatics/advance-article/doi/10.1093/bioinformatics/btaa1030/6039113
DeMaSk, an intuitive and interpretable method based only upon DMS datasets and sequence homologs that predicts the impact of missense mutations within any protein.
DeMaSk first infers a directional amino acid substitution matrix from DMS datasets and then fits a linear model that combines these substitution scores with measures of per-position evolutionary conservation and variant frequency.
□ HTSlib - C library for reading/writing high-throughput sequencing data
>> https://www.biorxiv.org/content/10.1101/2020.12.16.423064v1.full.pdf
The HTSlib library is structured as follows: the media access layer is a collection of low-level system and library (libcurl, knet) functions, which facilitate access to files on different storage environments and over multiple protocols to various online storage providers.
Over the lifetime of HTSlib the cost of sequencing has decreased by approximately 100-fold with a corresponding increase in data volume.
□ TIPS: Trajectory Inference of Pathway Significance through Pseudotime Comparison for Functional Assessment of single-cell RNAseq Data
>> https://www.biorxiv.org/content/10.1101/2020.12.17.423360v1.full.pdf
TIPS leverages the common trajectory mapping principle of pseudotime assignment to build pathway-specific trajectories from a pool of single cells.
The pseudotime values for each cell along these pathway-specific trajectories are compared to identify the processes with highest similarity to an overall trajectory. This latter source of variation may have significant ramifications on the accuracy of pseudotime alignment.
□ Minimally-overlapping words for sequence similarity search
>> https://academic.oup.com/bioinformatics/advance-article/doi/10.1093/bioinformatics/btaa1054/6042707
a simple sparse-seeding method: using seeds at positions of certain “words” (e.g. ac, at, gc, or gt). Sensitivity is maximized by using words with minimal overlaps. in a random sequence, minimally-overlapping words are anti-clumped.
using increasingly long minimum-variance words, with fixed sparsity n, the sensitivity might approach that of every-nth seeding. The seed count of every-nth seeding has zero variance.
□ VCFShark: how to squeeze a VCF file
>> https://www.biorxiv.org/content/10.1101/2020.12.18.423437v1.full.pdf
VCFShark, a dedicated fully-fledged com- pressor of VCF files. It significantly outperforms the universal tools in terms of compression ratio; sometimes its advantage is severalfold.
VCFShark dominates over BCF, pigz, and 7z by a large margin, achieving 3- to 32-fold better compression. It is mainly a result of an algorithm for compression of genotypes. The advantage over genozip, which uses similar compression for genotypes, up to 5.5-fold for HRC.
□ A monotonicity-based gene clustering algorithm for enhancing clarity in single-cell RNA sequencing data
>> https://www.biorxiv.org/content/10.1101/2020.12.20.423308v1.full.pdf
When clustering genes based on a monotonicity-based metric, it is important to note that uniformly expressed genes (with either very scarce dropout values or very abundant dropout values) are dangerous because they are likely to have high monotonicity values with many genes, even when a meaningful relationship may not exist.
Due to the high dimensionality of scRNA-seq data, genes with high variances, which will tend to serve as the cluster “centroids”, will tend to be well-separated.
□ scTypeR: Framework to accurately classify cell types in single-cell RNA-sequencing data
>> https://www.biorxiv.org/content/10.1101/2020.12.22.424025v1.full.pdf
The advantage of scTypeR and other related tools is that the cell type’s properties are learned from a reference dataset, but the reference dataset is no longer necessary to apply the model.
scTypeR uses SVM learning models organised in a tree-like structure to improve the classification of closely related cell types. scTypeR reports classification probabilities for every cell type and reports ambiguous classification results.

□ VarSAn: Associating pathways with a set of genomic variants using network analysis
>> https://www.biorxiv.org/content/10.1101/2020.12.22.424077v1.full.pdf
VarSAn analyzes a configurable network whose nodes represent variants, genes and pathways, using a Random Walk with Restarts algorithm to rank pathways for relevance to the given variants, and reports p-values for pathway relevance.
VarSAn ranks pathways first by their empirical p-values, which represent their connectivity to the query set, and then (to break ties) by their equilibrium probabilities, which are determined by both the connectivity and the network topology.

□ KATK: fast genotyping of rare variants directly from unmapped sequencing reads
>> https://www.biorxiv.org/content/10.1101/2020.12.23.424124v1.full.pdf
KATK is a fast and accurate software tool for calling variants directly from raw NGS reads. It uses predefined k-mers to retrieve only the reads of interest from the FASTQ file and calls genotypes by aligning retrieved reads locally.
KATK identifies unreliable variant calls and clearly distinguishes them in the output. KATK does not use data about known polymorphisms and has NC (No Call) as default genotype.
□ ARPIR: automatic RNA-Seq pipelines with interactive report
>> https://bmcbioinformatics.biomedcentral.com/articles/10.1186/s12859-020-03846-2
ARPIR allows the analysis of RNA-Seq data from groups undergoing different treatment allowing multiple comparisons in a single launch and can be used either for paired-end or single-end analysis.
Automatic RNA-Seq Pipelines with Interactive Report (ARPIR) makes a final tertiary-analysis that includes a Gene Ontology and Pathway analysis.
□ glmGamPoi: Fitting Gamma-Poisson Generalized Linear Models on Single Cell Count Data
>> https://doi.org/10.1093/bioinformatics/btaa1009
glmGamPoi provides inference of Gamma-Poisson generalized linear models with the following improvements over edgeR / DESeq2. glmGamPoi is more than 5 times faster than edgeR and more than 18 times faster than DESeq2.
glmGamPoi provides a quasi-likelihood ratio test with empirical Bayesian shrinkage to identify differentially expressed genes. glmGamPoi scales sub-linearly with the number of cells, which explains the observed performance benefit.
※コメント投稿者のブログIDはブログ作成者のみに通知されます