
(Created with Midjourney v5.2)

□ spVIPES: Integrative learning of disentangled representations from single-cell RNA-sequencing datasets
>> https://www.biorxiv.org/content/10.1101/2023.11.07.565957v1
spVIPES (shared-private Variational Inference via Product of Experts with Supervision) is a deep probabilistic framework to encode grouped single-cell RNA-seq data into shared and private factors of variation.
spVIPES accurately disentangles distinct sources of variation into private and shared representations. spVIPES leverages VAEs and PoE to model groups of cells into a common explainable latent space and their respective private latent spaces.
spVIPES takes an additional categorical vector representing batches or other covariates of interest that could drive technical differences. spVIPES outputs: the joint latent representation, each group's private representation, and the weights from each group's decoder network.

□ scTensor detects many-to-many cell–cell interactions from single cell RNA-sequencing data
>> https://bmcbioinformatics.biomedcentral.com/articles/10.1186/s12859-023-05490-y
scTensor is a novel method for predicting cell-cell interactions (CCIs) that utilizes a tensor decomposition algorithm to extract representative triadic relationships, or hypergraphs, which encompass ligand expression, receptor expression, and associated ligand-receptor (L-R) pairs.
scTensor does not perform the label permutation. It simply utilizes the factor matrices after the decomposition of the CCI-tensor. The order of computational complexity is reduced to O(N^2L(R1 + R2)); R1 & R2 are the number of columns or "rank" parameters for the factor matrices.

□ DeepGSEA: Explainable Deep Gene Set Enrichment Analysis for Single-cell Transcriptomic Data
>> https://www.biorxiv.org/content/10.1101/2023.11.03.565235v1
DeepGSEA, a DL-enhanced GSE analysis framework that predicts the phenotype while summarizing and enabling visualization of complex gene expression distributions of a gene set by utilizing intrinsically explainable prototype-based DNNs to provide an in-depth analysis og GSE.
DeepGSEA is able to learn the common encoding knowledge shared across gene sets, which is shown to improve the model's ability to mine phenotype knowledge from each gene set.
DeepGSEA is interpretable, as one can always explain how a gene set is enriched by visualizing the latent distributions and gene set projected expression profiles of cells around the learned prototypes.
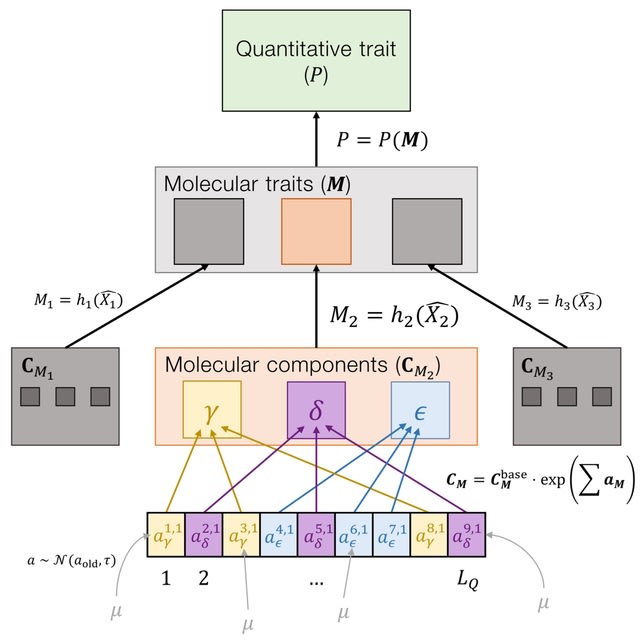
□ The distribution of fitness effects during adaptive walks using a simple genetic network
>> https://www.biorxiv.org/content/10.1101/2023.10.26.564303v2
Modeling quantitative traits as products of genetic networks via systems of ordinary differential equations. This allows us to mechanistically explore the effects of network structures on adaptation. By studying a simple gene regulatory network, the negative autoregulation motif.
Using forward-time genetic simulations, they measure adaptive walks towards a phenotypic optimum in both additive and network models. A key expectation from adaptive walk theory is that the distribution of fitness effects of new beneficial mutations is exponential.


□ RegDiffusion: From Noise to Knowledge: Probabilistic Diffusion-Based Neural Inference of Gene Regulatory Networks
>> https://www.biorxiv.org/content/10.1101/2023.11.05.565675v1
RegDiffusion, a novel neural network structure inspired by Denoising Diffusion Probabilistic Models but focusing on the regulatory effects among feature variables.
RegDiffusion introduces Gaussian noise to the input data following a diffusion schedule. It is subsequently trained to predict the added noise using a neural network with a parameterized adjacency matrix.
RegDiffusion only models the reverse (de-noising) process. Therefore, it avoids the costly adjacency matrix inversion step used by DAZZLE and DeepSEM. RegDiffusion enforces a trajectory to normality by its diffusion process, which helps stabilize the learning process.

□ Movi: a fast and cache-efficient full-text pangenome index
>> https://www.biorxiv.org/content/10.1101/2023.11.04.565615v1
Movi, a pangenome full-text index based on the move structure. Movi is much faster than alternative pangenome indexes like the r-index. They measure Movi's cache characteristics and show that, as hypothesized, queries achieve a small (nearly minimal) number of cache misses.
Movi can implement the same algorithms as alternative pangenome tools. Despite having a larger size compared to other pangenome indexes, Movi grows more slowly than other pangenome indexes as genomes are added.
Movi is the fastest available tool for full-text pangenome indexing and querying, and their open source implementation enables its application in various classification and alignment scenarios, including in speed-critical scenarios like adaptive sampling for nanopore sequencing.

□ TrimNN: Exploring building blocks of cell organization by estimating network motifs using graph isomorphism network
>> https://www.biorxiv.org/content/10.1101/2023.11.04.565623v1
TrimNN (Triangulation Network Motif Neural Network), neural network-based approach designed to estimate the prevalence of network motifs of any size in a triangulated cell graph.
TrimNN simplifies the intricate task of occurrence regression by decomposing it into binary present/absent predictions on small graphs. TrimNN is trained using representative pairs of predefined subgraphs and triangulated cell graphs to estimate overrepresented network motifs.
TrimNN robustly infers the presence of a large-size network motif in seconds. TrimNN only models the specific triangulated graphs after Delaunay triangulation on spatial omics data, where the spatial space is filled with only triangles.

□ MiRGraph: A transformer-based feature learning approach to identify miRNA-target interactions
>> https://www.biorxiv.org/content/10.1101/2023.11.04.565620v1
MiRGraph is a transformer-based, multi-view feature learning method capable of modeling both heterogeneous network and sequence features. TransCNN is a transformer-based CNN module that is designed for miRNAs and genes respectively to extract their personalized sequence features.
Then a heterogeneous graph transformer (HGT) module is adopted to learn the network features through extracting the relational and structural information in a heterogeneous graph consisting of miRNA-miRNA, gene-gene and miRNA-target interactions.
MiRGraph utilizes a multilayer perceptron (MLP) to map the learned features of miRNAs and genes into a same space, and a bilinear function to calculate the prediction scores of MTIs.

□ Algebraic Dynamical Systems in Machine Learning: An algebraic analogue of dynamical systems, based on term rewriting
>> https://arxiv.org/abs/2311.03118
A recursive function applied to the output of an iterated rewriting system defines a formal class of models into which all the main architectures for dynamic machine learning models (incl. recurrent neural networks, graph neural networks, and diffusion models) can be embedded.
In category theory, Algebraic models are a natural language for describing the compositionality of dynamic models. These models provide a template for the generalisation of the dynamic models to learning problems on structured or non-numerical - ‘Hybrid Symbolic-Numeric’ models.

□ SpatialAnno: Probabilistic cell/domain-type assignment of spatial transcriptomics data
>> https://academic.oup.com/nar/advance-article/doi/10.1093/nar/gkad1023/7370069
SpatialAnno, an efficient and accurate annotation method for spatial transcriptomics datasets, with the capability to effectively leverage a large number of non-marker genes as well as ‘qualitative’ information about mark er genes without using a reference dataset.
Uniquely, SpatialAnno estimates low-dimensional embeddings for a large number of non-marker genes via a factor model while promoting spatial smoothness among neighboring spots via a Potts model.

□ CINEMA-OT: Causal identification of single-cell experimental perturbation effects
>> https://www.nature.com/articles/s41592-023-02040-5
CINEMA-OT (causal independent effect module attribution + optimal transport) applies independent component analysis (ICA) and filtering on the basis of a functional dependence statistic to identify and separate confounding factors and treatment-associated factors.
CINEMA-OT then applies weighted optimal transport, a natural and mathematically rigorous framework that seeks the minimum-cost distributional matching, to achieve causal matching of individual cell pairs.
In CINEMA-OT, a Chatterjee’s coefficient-based distribution-free test is used to quantify whether each component correlates with the treatment event. Cells are matched across treatment conditions by entropy-regularized optimal transport in the confounder space to generate a causal matching plan.

□ BioMANIA: Simplifying bioinformatics data analysis through conversation
>> https://www.biorxiv.org/content/10.1101/2023.10.29.564479v1
BioMANIA employs an Abstract Syntax Tree (AST) parser to extract API attributes, incl. function description, input parameters, and return values. BioMANIA learns from tutorials, identifies the interplay between API usage, and aggregates APIs into meaningful functional ensembles.
BioMANIA prompts LLMs to comprehend the API and generates synthetic instructions corresponding to API calls. BioMANIA provides a diagnosis report with documentation improvement suggestions and an evaluation report concerning the quantitative performance of each step.

□ PS: Decoding Heterogenous Single-cell Perturbation Responses
>> https://www.biorxiv.org/content/10.1101/2023.10.30.564796v1
PS (Perturbation Score), a computational framework to detect heterogenous perturbation outcomes in single-cell transcriptomics. The PS score, estimated from constrained quadratic optimization, quantitatively measures the strength of perturbation outcome at a single cell level.
PS presents two major conceptual advances in analyzing single-cell perturbation data: the dosage analysis of perturbation, and the identification of novel biological determinants that govern the heterogeneity of perturbation responses.

□ ntsm: an alignment-free, ultra low coverage, sequencing technology agnostic, intraspecies sample comparison tool for sample swap detection
>> https://www.biorxiv.org/content/10.1101/2023.11.01.565041v1
ntsm minimizes upstream processing as much as possible. It starts by counting the relevant variant k-mers from a sample only keeping information needed to perform the downstream analysis. The counting can be set to terminate early if sufficient read coverage is obtained.
Once generated the counts can be compared in a pairwise manner using a likelihood-ratio based test. During this, sequence error rate is also estimated using the counts.
The number of tests can be reduced by specifying an optional PCA rotation matrix and normalization matrix adding a prefiltering step on high quality samples. Finally, matching sample pairs are outputted in a tsv file.

□ DeepSipred: A deep-learning-based approach on siRNA inhibition prediction
>> https://www.biorxiv.org/content/10.1101/2023.11.02.565277v1
DeepSipred enriches the characteristics of sequence context via one-hot encoding and pretrained RNA foundation model (RNA-FM). Features also consist of thermodynamic proper-ties, the secondary structure, the nucleotide composition, and other expert knowledge.
DeeoSipred utilizes different kernels to detect potential motifs in sequence embedding, followed by a pooling operation. DeepSipred concatenates the output of pooling and all other features together. It is fed into a deep and wide network with a sigmoid activation function.

□ GIN-TONIC: Non-hierarchical full-text indexing for graph-genomes
>> https://www.biorxiv.org/content/10.1101/2023.11.01.565214v1
GIN-TONIC (Graph INdexing Through Optimal Near Interval Compaction). It is designed to handle string-labelled directed graphs of arbitrary topology by indexing all possible string walks without explicitly storing them.
GIN-TONIC allows for efficient exact lookups of substring queries of unrestricted length in polynomial time and space; it does not require the construction of multiple indices or explicit enumeration of walks, and it easily scales up to human (pan)genomes and transcriptomes.

□ A Generalized Supervised Contrastive Learning Framework for Integrative Multi-omics Prediction Models
>> https://www.biorxiv.org/content/10.1101/2023.11.01.565241v1
MB-SupCon-cont, a generalized contrastive learning framework for both categorical and continuous covariates on multi-omics data. It generalizes the concept of "similar data pairs" based on the distance of responses b/n two data points and use it in a generalized contrastive loss.
The generalized contrastive loss should be employed in this context to accommodate various types of covariate data. Prediction heads (classifiers/regressors) are utilized on the embeddings. A unique trend related to the covariates can be visualized in the lower-dimensional space.

□ GPSite: Genome-scale annotation of protein binding sites via language model and geometric deep learning
>> https://www.biorxiv.org/content/10.1101/2023.11.02.565344v1
GPSite (Geometry-aware Protein binding Site predictor), a fast, accurate and versatile network for concurrently predicting binding residues of ten types of biologically relevant molecules including DNA, RNA, peptide, protein, ATP, HEM, and metal ions in a multi-task framework.
GPSite was trained on informative sequence embeddings and predicted structures generated by protein language models. A comprehensive geometric featurizer along with an edge-enhanced graph neural network is designed to extract the residual and relational geometric contexts.

□ Integrating single-cell RNA-seq datasets with substantial batch effects
>> https://www.biorxiv.org/content/10.1101/2023.11.03.565463v1
Given that many widely adopted and scalable methods are based on conditional variational autoencoders (cVAE), they hypothesize that machine learning interventions to standard cVAEs improves batch effect removal while potentially preserving biological variation more effectively.
Cycle-consistency and VampPrior improved batch correction while retaining high biological preservation, with their combination further increasing performance.
While adversarial learning led to the strongest batch correction, its preservation of within-cell type variation did not match that of VampPrior or cycle-consistency models, and it was also prone to mixing unrelated cell types with different proportions across batches.
KL regularization strength tuning had the least favorable performance, as it jointly removed biological and batch variation by reducing the number of effectively used embedding dimensions.

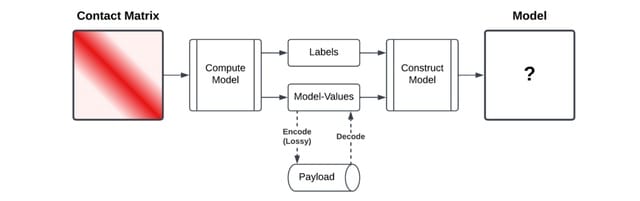
□ HiCMC: High-Efficiency Contact Matrix Compressor
>> https://www.biorxiv.org/content/10.1101/2023.11.03.565487v1
The key idea of CMC is to sort the matrix values such that in each row of a contact matrix, the number of bits required for each value, i.e., the magnitude of the values, is similar. The probability of contact can be viewed as a function of distance for contacts within a chromosome.
HiCMC(High-Efficiency Contact Matrix Compressor), an approach for the matrix compression. It comprises splitting the genome-wide contact matrix into intra/inter-chromosomal sub-contact matrices, row/column masking, model-based transformation, row binarization, and entropy coding.

□ SuPreMo: a computational tool for streamlining in silico perturbation using sequence-based predictive models
>> https://www.biorxiv.org/content/10.1101/2023.11.03.565556v1
SuPreMo (Sequence Mutator for Predictive Models) generates reference and perturbed sequences for input into predictive models. SuPreMo-Akita applies the tool to an existing sequence-to-profile model, Akita, and generates scores that measure disruption to genome folding.
SuPreMo incorporates variants one at a time into the reference genome and generates reference and alternate sequences for each perturbation under each provided augmentation parameter. The sequences are accompanied by the relative position of the perturbation for each sequence.

□ reconcILS: A gene tree-species tree reconciliation algorithm that allows for incomplete lineage sorting
>> https://www.biorxiv.org/content/10.1101/2023.11.03.565544v1
reconcILS, a new algorithm for carrying out reconciliation that accurately accounts for incomplete lineage sorting by treating ILS as a series of nearest neighbor interchange (NNI) events.
For discordant branches of the gene tree identified by last common ancestor (LCA) mapping, our algorithm recursively chooses the optimal history by comparing the cost of duplication and loss to the cost of NNI and loss.
reconcILS uses a new simulation engine (dupcoal) that can accurately generate gene trees produced by the interaction of duplication, ILS, and loss. reconcILS outputs the minimum number of duplications/losses/NNIs. Inferred events are all also assigned to nodes in the gene tree.

□ SPAN: Hidden Markov random field models for cell-type assignment of spatially resolved transcriptomics
>> https://academic.oup.com/bioinformatics/advance-article/doi/10.1093/bioinformatics/btad641/7379666
SPAN (a statistical spatial transcriptomics cell assignment framework) assigns cells or spots into known types in the SRI data with prior knowledge of predefined marker genes and spatial information.
The SPAN model combines a mixture model with an HMRF to model spatial dependency b/n neighboring spots and annotates cells or spots from SRT data using predefined overexpressed marker genes. The discrete counts of SRT data are characterized by the negative binomial distribution.
The framework of SPAN consists of two modules: a mixture negative binomial distribution module and an Hidden Markov Random Field module. The mixture module takes the gene expression matrix and the marker gene indicator matrix as input to determine region assignments.

□ PhylteR: efficient identification of outlier sequences in phylogenomic datasets
>> https://academic.oup.com/mbe/advance-article/doi/10.1093/molbev/msad234/7330000
PhylteR, a method that allows a rapid and accurate detection of outlier sequences in phylogenomic datasets, i.e. species from individual gene trees that do not follow the general trend.
PhylteR relies on DISTATIS, an extension of multidimensional scaling to 3 dimensions to compare multiple distance matrices at once. These distance matrices extracted from individual gene phylogenies represent evolutionary distances between species according to each gene.

□ sciCSR infers B cell state transition and predicts class-switch recombination dynamics using single-cell transcriptomic data
>> https://www.nature.com/articles/s41592-023-02060-1
sciCSR, a Markov state model is built to infer the dynamics and direction of CSR. sciCSR utilizes data from an earlier time point in the collected time-course to predict the isotype distribution of B cell receptor repertoires at subsequent time points with high accuracy.
sciCSR identifies isotype signatures using NMF to both productive and sterile transcripts of all isotypes, and uaing these signatures to score the CSR status. sciCSR characterizes the expression levels of all IgH productive and sterile transcripts in naive/memory B cell states.
sciCSR imports functionality implemented in CellRank to fit Markov models, and allows user to use either CSR or SHM as input for estimating the transition matrix; these can be compared against CellRank models fitted using RNA velocity.

□ FracMinHash: Fast, lightweight, and accurate metagenomic functional profiling using FracMinHash sketches
>> https://www.biorxiv.org/content/10.1101/2023.11.06.565843v1
FracMinHash, a k-mer-sketching algorithm to obtain functional profiles of metagenome samples. Their pipeline can take FracMinHash sketches of a given metagenome and the KOs, and progressively discovers what KOs are present in the metagenome using the algorithm sourmash gather.
The pipeline can also annotate the relative abundances of the KOs. It is fast and lightweight because of using FracMinHash sketches, and is accurate when the sequencing depth is moderately high.

□ GERONIMO: A tool for systematic retrieval of structural RNAs in a broad evolutionary context
>> https://academic.oup.com/gigascience/article/doi/10.1093/gigascience/giad080/7319579
GERONIMO (GEnomic RNA hOmology aNd evolutIonary MOdeling), a bioinformatics pipeline that uses the Snakemake framework to conduct high-throughput homology searches of ncRNA genes using covariance models on any evolutionary scale.
GERONIMO offers a covariance model or multiple alignments in Stockholm format, allowing users to search by defining a target database. These databases can be easily configured at NCBI’s database service and can range in scale from order to family, clade, phylum, or kingdom.
GERONIMO generates accessible tables that present all essential information regarding the query and target sequence similarity levels. These tables are enriched with a broad taxonomy context, which enables effective data filtering and minimizes false-positive results.

□ biomapp::chip: Large-Scale Motif Analysis
>> https://www.biorxiv.org/content/10.1101/2023.11.06.565033v1
Biomapp::chip is a computational tool designed for the efficient discovery of biological motifs, specifically optimized for ChIP-seq data. Utilizing advanced k-mer counting algorithms and data structures, it offers a streamlined, accurate, and fast approach to motif discovery.
The Biomapp::chip algorithm adopts a two-step approach for motif discovery: counting and optimization. The sMT (Sparse Motif Tree) is employed for efficient kmer counting, enabling rapid and precise analysis. BIOMAPP::CHIP employs an enhanced version of the EM algorithm.

□ Hybrid deep learning approach to improve classification of low-volume high-dimensional data
>> https://bmcbioinformatics.biomedcentral.com/articles/10.1186/s12859-023-05557-w
The method proceeds by training a supervised DNN for feature extraction for the targeted classification task and using the extracted feature representation from the DNN for training a traditional ML classifier.
This approach takes advantage of learning a data representation from raw data using DL methods. This is based in part on the increased interpretability of the classifications made by decision-tree-based classifiers, like XGBoost.

□ FitMultiCell: Simulating and parameterizing computational models of multi-scale and multi-cellular processes
>> https://academic.oup.com/bioinformatics/advance-article/doi/10.1093/bioinformatics/btad674/7382208
FitMultiCell, a scalable platform that integrates modeling, simulation, and parameter estimation, to simplify the analysis of multi-scale and multi-cellular systems. FitMultiCell integrates Morpheus for model building and simulation, and pyABC for parameter estimation.
In summary, their evaluation confirmed an overall good scaling of the FitMultiCell pipeline, yielding a wall-time reduction of several ten-fold compared to a single-node execution and several hundred-fold compared to single-core execution.
□ bioRxiv has launched a pilot to provide AI-generated summaries for all preprints thanks to @Science_Cast. We hope this will increase a preprint’s reach.
>> https://biorxiv.org/about-biorxiv
※コメント投稿者のブログIDはブログ作成者のみに通知されます