

□ Terminus: a new data-driven approach for grouping together transcripts in an experiment based on their inferential uncertainty
>> https://www.biorxiv.org/content/10.1101/2020.04.07.029967v1.full.pdf
Terminus writes the new expression estimates and posterior samples with the group information in the same exact format as that of Salmon, enabling any downstream pipeline that accepts a similar format to directly run on terminus output.
Terminus prunes the possible space of pairwise collapses by examining the structure induced by the range-factorized equivalence classes, and uses a iterative greedy technique that locally maximize the objective being optimized i.e. the reduction in inferential relative variance.
□ Skygrid: Hamiltonian Monte Carlo sampling to estimate past population dynamics using the skygrid coalescent model in a Bayesian phylogenetics framework
>> https://wellcomeopenresearch.org/articles/5-53
Hamiltonian Monte Carlo proceeds by introducing fictitious auxiliary “momentum” variables and reduces simulating from the posterior distribution to a matter of tracing Hamiltonian dynamics.
an HMC transition kernel for the nonparametric skygrid coalescent model and compare its performance to the Block-updating Markov chain Monte Carlo sampling (BUMCMC) sampler.
a Hamiltonian Monte Carlo gradient-based sampler to infer the parameters of the skygrid model. it outperforms the BUMCMC transition kernel that was specifically designed for GMRF models and relied on and exploited many aspects of the skygrid structure.

□ SCHNEL: Scalable clustering of high dimensional single-cell data
>> https://www.biorxiv.org/content/10.1101/2020.03.30.015925v1.full.pdf
SCHNEL (Scalable Clustering of Hierarchical Stochastic Neighbour Embedding hierarchies using Louvain community detection) transforms large high-dimensional data to a hierarchy of datasets containing subsets of data points following the original data manifold.
SCHNEL combines the hierarchical representation of the data with graph clustering, making graph clustering scalable to millions of cells. SCHNEL was able to produce meaningful clustering results for datasets of 3.5 and 17.2 million cells within workable timeframes.
□ SSRE: Cell Type Detection Based on Sparse Subspace Representation and Similarity Enhancement
>> https://www.biorxiv.org/content/10.1101/2020.04.08.028779v1.full.pdf
SSRE computes the sparse representation similarity of cells based on the subspace theory, and designed a gene selection process and an enhancement strategy based on the characteristics of different similarities to learn more reliable similarities.
SSRE performs eigengap on the learned similarity matrix to estimate the number of clusters. Eigengap is a typical cluster number estimation method, and it determines the number of clusters by calculating max gap between eigenvalues of a Laplacian matrix.

□ HELLO: A hybrid variant calling approach
>> https://www.biorxiv.org/content/10.1101/2020.03.23.004473v1.full.pdf
HELLO (Hybrid Evaluation of smaLL genOmic variants) is a caller, based on the Mixture-of-Experts paradigm which allows multiple experts to make independent predictions, and a switch, or meta-expert to choose one of the experts’ predictions.
The meta-expert is a DNN that learns what types of genomic regions are suited for each expert. In addition, each expert and meta-expert uses specialized architectures tailored for genome sequencing data, which allows us to reduce the computational complexity of the analysis.
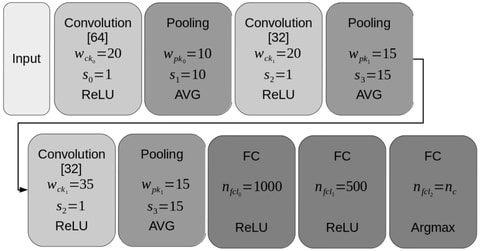
□ TERL: Classification of Transposable Elements by Convolutional Neural Networks
>> https://www.biorxiv.org/content/10.1101/2020.03.25.000935v1.full.pdf
TERL, a transposable elements representation learner, that use four preprocessing steps, a transformation of one-dimensional nucleic acid sequences into two-dimensional space data and apply it to deep convolutional neural networks.
TERL can learn how to predict any hierarchical level of the TEs classification system, is on average 162 times and four orders of magnitude faster than TEclass and PASTEC.

□ Enumerated Radix Trees (ERT): Accelerating Maximal-Exact-Match Seeding
>> https://www.biorxiv.org/content/10.1101/2020.03.23.003897v1.full.pdf
a memory bandwidth-aware data structure for maximal-exact-match seeding called Enumerated Radix Tree (ERT). ERT supports multi-character lookup using a multi-level index table and radix tree.
Maximum read length is used as a parameter to decide the maximum depth of the radix trees in ERT. ERT improves read alignment throughput of BWA-MEM2 by 1.28×, ERT exposes significant acceleration potential by designing an FPGA prototype.

□ Probabilistic model based on circular statistics for quantifying coverage depth dynamics originating from DNA replication
>> https://peerj.com/articles/8722/
a method of modeling coverage depth dynamics using probabilistic statistics. combining multinomial and directional distributions to mimic the read sampling process and bias of the DNA quantity.
this method enables detailed measurement of DNA quantity changes by using circular distributions in the model. Moreover, by combining multiple distributions, it became possible to estimate the growth of organisms with multiple replication origins.

□ Deep learning at base-resolution reveals motif syntax of the cis-regulatory code
>> https://www.biorxiv.org/content/10.1101/737981v1.full.pdf
BPNet, a novel convolutional neural networks (CNNs) and model interpretation techniques to decipher the cis-regulatory code of in vivo transcription factor binding from ChIP- nexus data.
a deep learning model that uses DNA sequence to predict base-resolution binding profiles of four pluripotency transcription factors Oct4, Sox2, Nanog, and Klf4.
Interpreting deep learning models applied to high-resolution binding data is a powerful and versatile approach to uncover the motifs and syntax of cis-regulatory sequences.
□ Hellinger distance-based stable sparse feature selection for high-dimensional class-imbalanced data
>> https://bmcbioinformatics.biomedcentral.com/articles/10.1186/s12859-020-3411-3
sssHD is based on the Hellinger distance (HD) coupled with sparse regularization techniques. Hellinger distance is not only class-insensitive but also translation-invariant.
Hellinger distance-based selection algorithm is effective in recognizing key features and control false discoveries for class-imbalance learning.

□ HARVESTMAN: A framework for hierarchical feature learning and selection from whole genome sequencing data
>> https://www.biorxiv.org/content/10.1101/2020.03.24.005603v1.full.pdf
HARVESTMAN selects a rich combination of representations that are adapted to the learning task, and performs better than a binary representation of SNPs alone. HARVESTMAN automatically learns the best feature encoding while performing feature selection.
HARVESTMAN employs supervised hierarchical feature selection under a wrapper- based regime, as it solves an optimization problem over the knowledge graph designed to select a small and non-redundant subset of maximally informative features.
□ Wavelet Screening: a novel approach to analysing GWAS data
>> https://www.biorxiv.org/content/10.1101/2020.03.24.006163v1.full.pdf
Wavelet Screening provides a substantial gain in power compared to both the traditional GWAS modelling as well as another popular regional-based association test called ‘SNP-set (Sequence) Kernel Association Test’ (SKAT).
The null and alternative hypotheses are modelled using the posterior distribution of the wavelet coefficients. And enhancig the decision procedure by using additional information from the regression coefficients and by taking advantage of the pyramidal structure of wavelets.

□ SCYN: Single cell CNV profiling method using dynamic programming
>> https://www.biorxiv.org/content/10.1101/2020.03.27.011353v1.full.pdf
SCYN integrates SCOPE, which partitions chromosomes into consecutive bins and computes the cell- by-bin read depth matrix, to process the input BAM files and get the raw and normalized read depth matrices.
The segmentation detection algorithm is then performed on the raw and normalized read depth matrices using our dynamic programming to identify the optimal segmentation along each chromosome.

□ REINDEER: efficient indexing of k-mer presence and abundance in sequencing datasets
>> https://www.biorxiv.org/content/10.1101/2020.03.29.014159v1.full.pdf
REINDEER (REad Index for abuNDancE quERy) constructs the compacted de Bruijn graph (DBG) of each dataset, then conceptually merges those DBGs into a single global one.
Then, REINDEER constructs and indexes monotigs, which in a nutshell are groups of k-mers of similar abundances, discretization and compression of counts, on-disk row de-duplication algorithm of the count matrix.

□ parEBEN: A Parallelized Strategy for Epistasis Analysis Based on Empirical Bayesian Elastic Net Models
>> https://academic.oup.com/bioinformatics/advance-article-abstract/doi/10.1093/bioinformatics/btaa216/5813727
By parallelizing the iterations of the cross-validation over multiple CPU cores or multiple machines of a computing clusters, a drastic time reduction can seen with no negative effect on the resulting EBEN models.
parEBEN is a matrix manipulation strategy for pre-computing the correlation matrix and pre-filter to narrow down the search space for epistasis analysis.

□ IterCluster: a barcode clustering algorithm for long fragment read analysis
>> https://peerj.com/articles/8431/
IterCluster had a higher precision and recall rate on BGI stLFR data compared to 10X Genomics Chromium read data.
IterCluster improves the de novo assembly results when using a divide-and-conquer strategy on a human genome data set (scaffold/contig N50 = 13.2 kbp/7.1 kbp vs. 17.1 kbp/11.9 kbp before and after IterCluster, respectively).
IterCluster uses a k-mer frequency diversity select model to control the false positives generated during barcode clustering, and have used a Markov clustering (MCL) model to ensure that the clustering results only contain barcodes from one fragment area.
□ G2S3: a gene graph-based imputation method for single-cell RNA sequencing data
>> https://www.biorxiv.org/content/10.1101/2020.04.01.020586v1.full.pdf
G2S3 imputes dropouts by borrowing information from adjacent genes in a sparse gene graph learned from gene expression profiles across cells.
G2S3 learns a sparse graph representation of gene-gene relationships from scRNA-seq data, in which each node represents a gene and is associated with a vector of expression levels in all cells that can be viewed as a signal on the graph.

□ Succinct Dynamic de Bruijn Graphs
>> https://www.biorxiv.org/content/10.1101/2020.04.01.018481v1.full.pdf
DynamicBOSS, a succinct representation of the de Bruijn graph that allows for an unlimited number of additions and deletions of nodes and edges.
DynamicBOSS is the only method that supports both addition and deletion and is applicable to very large samples (greater than 15 billion k-mers). DynamicBOSS is the only full- dynamic, space-efficient de Bruijn method that can be constructed on large samples.

□ SimiC: A Single Cell Gene Regulatory Network Inference method with Similarity Constraints
>> https://www.biorxiv.org/content/10.1101/2020.04.03.023002v1.full.pdf
SimiC, that jointly infers the GRNs corresponding to each state. SimiC models the GRN inference problem as a LASSO optimization problem with an added similarity constraint, on the GRNs associated to contiguous cell states.
SimiC is able to model the transition regulatory dynamics via the inferred GRNs. SimiC generates multiple networks at the same time, providing a framework to further evaluate the network dynamics.
□ GenMap: Ultra-fast Computation of Genome Mappability
>> https://academic.oup.com/bioinformatics/advance-article/doi/10.1093/bioinformatics/btaa222/5815974
GenMap computes the (k, e)-mappability. extending the mappability algorithm, such that it can also be computed across multiple genomes where a k-mer occurrence is only counted once per genome.
GenMap still outperforms Umap as GenMap needs less than one hour without parallelization to compute the (k, 0)-mappability (see table 1), while Umap needs about 200 hours.
□ Joint learning dimension reduction and clustering of single-cell RNA-sequencing data
>> https://academic.oup.com/bioinformatics/advance-article-abstract/doi/10.1093/bioinformatics/btaa231/5815975
a flexible and accurate algorithm for scRNA-Seq data by jointly learning dimension reduction and cell clustering (aka DRjCC), where dimension reduction is performed by projected matrix decomposition and cell type clustering by nonnegative matrix factorization.
the overall complexity of DRjCC model is O(tnk1m + k1m^2 + tm^3 + tk1k2m). Since the number of dimension reduction is usually much lower than that of original size, i.e. k1, k2 ≪ n, then the complexity is basically O(tm^3).
□ Prioritizing genetic variants in GWAS with lasso using permutation-assisted tuning
>> https://academic.oup.com/bioinformatics/advance-article-abstract/doi/10.1093/bioinformatics/btaa229/5815976
a new permutation-assisted tuning procedure in lasso (plasso) to identify phenotype-associated SNPs in a joint multiple-SNP regression model in GWAS.
plasso first generates permutations as pseudo-SNPs that are not associated with the phenotype. Then, the lasso tuning parameter is delicately chosen to separate true signal SNPs and noninformative pseudo-SNPs.

□ DeepMNE-CNN: Integrating multi-network topology for gene function prediction using deep neural networks
>> https://academic.oup.com/bib/advance-article/doi/10.1093/bib/bbaa036/5816013
DeepMNE-CNN, utilizes a convolutional neural network based on the integrated feature embedding to annotate unlabeled gene functions.
DeepMNE is an iteratively stacked model, which includes one AE layer and four semi-autoencoder layers.
□ GenoPheno: cataloging large-scale phenotypic and next-generation sequencing data within human datasets
>> https://academic.oup.com/bib/advance-article/doi/10.1093/bib/bbaa033/5816026
The GenoPheno-CatalogShiny is a dynamic catalog that puts together a descriptive and informative summary of datasets with genomic and phenotypic data, to help investigators to identify resources for their research.
This catalog only includes those data repositories that comply with over 500 human subjects, Contain both genotype and phenotype data for the same subjects, Include WGS and/or WES data, Include at least 100 recorded phenotypic variables per subject.
□ ShinyLearner: A containerized benchmarking tool for machine-learning classification of tabular data
>> https://academic.oup.com/gigascience/article/9/4/giaa026/5813098
ShinyLearner provides a uniform interface for performing classification, irrespective of the library that implements each algorithm, thus facilitating benchmark comparisons
ShinyLearner enables researchers to optimize hyperparameters and select features via nested cross-validation; it tracks all nested operations and generates output files that make these steps transparent.
ShinyLearner gains deeper insight into decisions made during nested cross-validation, andseeks to evaluate the tradeoff between predictive accuracy and time of execution.

□ LIGER: Jointly Defining Cell Types from Multiple Single-Cell Datasets
>> https://www.biorxiv.org/content/10.1101/2020.04.07.029546v1.full.pdf
Linked Inference of Genomic Experimental Relationships (LIGER) that uses integrative nonnegative matrix factorization to address this challenge.
a step-by-step protocol for using LIGER to jointly define cell types from multiple single-cell datasets. The main steps of the protocol include data preprocessing and normalization, joint factorization, quantile normalization and joint clustering, and visualization.
□ MasterOfPores: A Workflow for the Analysis of Oxford Nanopore Direct RNA Sequencing Datasets
>> https://www.frontiersin.org/articles/10.3389/fgene.2020.00211/full
MasterOfPores, a scalable and parallelizable workflow for the analysis of direct RNA sequencing, which uses as input raw direct RNA sequencing FAST5 reads format, which includes current intensity values, metadata of the sequencing run and base-called fasta sequences.
MasterOfPores starts with a pre-processing module, which converts raw current intensities into multiple types of processed data including FASTQ and BAM, providing metrics of the quality of the run, quality-filtering, demultiplexing, base-calling and mapping.

□ Multiview learning for understanding functional multiomics
>> https://journals.plos.org/ploscompbiol/article?id=10.1371/journal.pcbi.1007677
formulating multiview learning in a unified mathematical framework called multiview empirical risk minimization (MV-ERM), an extension of empirical risk minimization (ERM).
a method for simultaneous clustering of multiview cancer data using a multiview spectral clustering. In this gradient descent method, the gradient calculated from a Euclidean space in each iteration is projected onto an embedded matrix manifold.
□ HAPPI GWAS: Holistic Analysis with Pre and Post Integration GWAS
>> https://www.biorxiv.org/content/10.1101/2020.04.07.998690v1.full.pdf
Many GWAS tools lack a comprehensive pipeline that includes both pre-GWAS analysis such as outlier removal, data transformation, and calculation of Best Linear Unbiased Pre- dictions (BLUPs) or Best Linear Unbiased Estimates (BLUEs).
HAPPI GWAS eliminates the need for multiple tools by providing a comprehensive GWAS pipeline for all phases of a GWAS analysis.
HAPPI GWAS compiles GWAS results creating a combined GWAS re- sults summary that includes significant SNP IDs, gene names, gene de- scriptions, and haploblock information.

□ GOMCL: a toolkit to cluster, evaluate, and extract non-redundant associations of Gene Ontology-based functions
>> https://bmcbioinformatics.biomedcentral.com/articles/10.1186/s12859-020-3447-4
GOMCL facilitates biological interpretation of a large number of GO terms by condensing them into GO clusters representing non-overlapping functional themes.
GOMCL efficiently identifies clusters within a list of GO terms using the Markov Clustering (MCL) algorithm, based on the overlap of gene members between GO terms.
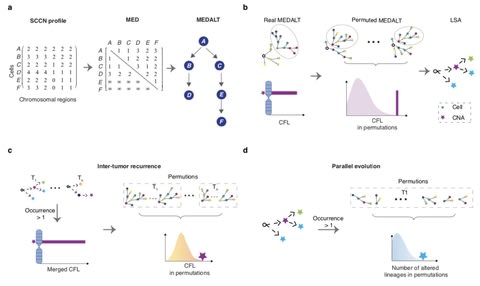
□ MEDALT: Single-cell copy number lineage tracing enabling gene discovery https://www.biorxiv.org/content/10.1101/2020.04.12.038281v1.full.pdf
a Minimal Event Distance Aneuploidy Lineage Tree (MEDALT) algorithm that infers the evolution history of a cell population based on single-cell copy number (SCCN) profiles.
MEDALT allows a genomic region to be repetitively altered by multiple single-copy gains or losses. It provides a parsimonious interpretation, the minimal number of single-copy gains or losses that may have led to the evolution of the entire cell population.
□ Pooled variable scaling for cluster analysis
>> https://academic.oup.com/bioinformatics/article-abstract/doi/10.1093/bioinformatics/btaa243/5819546
Many clustering methods are not scale invariant because they are based on Euclidean distances. Even methods using scale invariant distances such as the Mahalanobis distance lose their scale invariance when combined with regularization and/or variable selection.
Unlike available scaling procedures such as the standard deviation and the range, this proposed scale avoids dampening the beneficial effect of informative clustering variables.
□ MAGIC: A tool for predicting transcription factors and cofactors driving gene sets using ENCODE data
>> https://journals.plos.org/ploscompbiol/article?id=10.1371/journal.pcbi.1007800
Mining Algorithm for GenetIc Controllers (MAGIC) uses data derived from ChIPseq tracks archived at ENCODE to decipher which Factors are most likely to preferentially bind lists of genes that are altered from one biological state to another.
MAGIC circumvents the principal confounds of current methods to identify Factors, the use of TF motif searches, inability to identify cofactors due the absence of any binding site motifs, and assignment of Factors to genes based on hard cutoffs of ChIP-seq signals.
□ Temporal ordering of omics and multiomic events inferred from time series data
>> https://www.biorxiv.org/content/10.1101/2020.04.14.040527v1.full.pdf
Minardo-Model provides a novel method for analysing and visualizing time series data sets from omics and multiomics experiments.
Minardo-Model helps interpret the resulting clusters by automatically inferring an event ordering (often, at better temporal resolution than in the original experiment), and by providing concise, integrated visual summaries.
□ A faster implementation of association mapping from k-mers
>> https://www.biorxiv.org/content/10.1101/2020.04.14.040675v1.full.pdf
In the Hawk pipeline, population structure was estimated using Eigenstrat and subsequently p-values were adjusted for population structure using the glm function (for fitting logistic regression models) and ANOVA function.
The re-implemented Hawk with the goal to reduce its execution time, and also extends support for Jellyfish 2 and implemented Benjamini–Hochberg procedure, which can be used to correct for multiple tests when the study is underpowered for Bonferroni correction.

□ Bio-Node – Bioinformatics in the Cloud
>> https://www.biorxiv.org/content/10.1101/2020.04.15.043596v1.full.pdf
Bio-Node, a universal biological computing workflow platform. Bio-Node enables building complex workflows using a sophisticated web interface.
Bio-Node uses "Auto-Clustering", a workflow that automati- cally extracts the most suited clustering parameters for specific data types and subsequently enables to optimally segregate unknown samples of the same type.
□ Overlap Detection on Long, Error-Prone Sequencing Reads via Smooth q-Gram
>> https://academic.oup.com/bioinformatics/article-abstract/doi/10.1093/bioinformatics/btaa252/5822881
Studying the overlap detection problem for error-prone reads, which is the first and most critical step in the de novo fragment assembly.
smooth q-gram, a variant of q-gram that captures q-gram pairs within small edit distances and design a novel algorithm for detecting overlapping reads using smooth q-gram-based seeds.
□ ntJoin: Fast and lightweight assembly-guided scaffolding using minimizer graphs
>> https://academic.oup.com/bioinformatics/article/doi/10.1093/bioinformatics/btaa253/5822878
ntJoin, an assembly-guided scaffolder, which uses a lightweight, alignment-free mapping strategy in lieu of alignments to quickly contiguate a target assembly using one or more references.
When scaffolding a human short read assembly using the reference human genome or a long read assembly, ntJoin improves the NGA50 length 23- and 13-fold, respectively, in under 13 m, using less than 11 GB of RAM.
ntJoin performs minimizer graph-based scaffolding quickly and with a small memory footprint, while still producing chromosome-level contiguity.
□ Short paired-end reads trump long single-end reads for expression analysis
>> https://bmcbioinformatics.biomedcentral.com/articles/10.1186/s12859-020-3484-z
the extra information provided by reads pairs during the mapping or pseudoalignment phase outweighs any potential penalty of short reads.
This approach to evaluating the performance of short paired-end reads vs. longer single-end ones is to compare expression estimates and differential expression results derived from these strategies to a truth set.
※コメント投稿者のブログIDはブログ作成者のみに通知されます