

□ Adjoining colimits
>> https://arxiv.org/abs/2111.12117v1
a theory of colimit sketches ‘with constructions’ in higher category theory, formalising the input to the ubiquitous procedure of adjoining specified ‘constructible’ colimits to a category such that specified ‘relation’ colimits are enforced.
Morel-Voevodsky’s category of motivic spaces, resp. Robalo’s category of non-commutative motives are universal among categories under Sch, resp. ncSch, admitting all colimits such that Nisnevich descent is preserved and A1-localisation is enforced.
This language makes explicit the rôle colimit diagrams play as presentations of objects of ∞-categories, expressing how they are put together from objects of a dense subcategory. It may be useful to theory builders embarking on a construction of their own ‘designer’ ∞-category.

□ SAT: Efficient iterative Hi-C scaffolder based on N-best neighbors
>> https://bmcbioinformatics.biomedcentral.com/articles/10.1186/s12859-021-04453-5
Hi-C based scaffolding tool, pin_hic, which takes advantage of contact information from Hi-C reads to construct a scaffolding graph iteratively based on N-best neighbors of contigs. It identifies potential misjoins and breaks them to keep the scaffolding accuracy.
SAT, a new format which is inspired by the GFA and extended to keep scaffolding information. In each iteration, if the SAT file is used as an input, the paths will be construct first and each original contig in the draft assembly will keep a record of its corresponding scaffold.

□ EnGRaiN: A Supervised Ensemble Learning Method for Recovery of Large-scale Gene Regulatory Networks
>> https://academic.oup.com/bioinformatics/advance-article-abstract/doi/10.1093/bioinformatics/btab829/6458321
EnGRaiN , the first supervised ensemble learning method to construct gene networks. The supervision for training is provided by small training datasets of true edge connections (positives) and edges known to be absent (negatives) among gene pairs.
EnGRaiN integrates interaction/co-expression predictions from multiple gene network inference methods to generate a comprehensive ensemble network of gene interactions. EnGRaiN leverages the ground truth to learn optimal distribution over its various features.

□ SCRIP: an accurate simulator for single-cell RNA sequencing data
>> https://academic.oup.com/bioinformatics/advance-article-abstract/doi/10.1093/bioinformatics/btab824/6454945
SCRIP provides a flexible Gamma-Poisson mixture and a Beta-Gamma-Poisson mixture framework to simulate scRNA-seq data. SCRIP package was built based on the framework of splatter. Both Gamma-Poisson and Beta-Poisson distribution model the over dispersion of scRNA-seq data.
Specifically, Beta-Poisson model was used to model bursting effect. The dispersion was accurately simulated by fitting the mean-BCV dependency using Generalized Additive Model.
SCRIP modeles other key characteristics of scRNA-seq data incl. library size, zero inflation and outliers. SCIRP enables various application for different experimental designs and goals including DE analysis, clustering analysis, trajectory-based analysis and bursting analysis.

□ schist: Nested Stochastic Block Models applied to the analysis of single cell data
>> https://bmcbioinformatics.biomedcentral.com/articles/10.1186/s12859-021-04489-7
schist is a convenient wrapper to the graph-tool python library, designed to be used with scanpy. The most prominent function is schist.inference.nested_model() which takes a AnnData object as input and fits a nested Stochastic Block Model on the kNN graph built with scanpy.
The Bayesian formulation of Stochastic Block Models provides the possibility to perform inference on a graph for any partition configuration, thus allowing reliable model selection using an interpretable measure, entropy.

□ scShaper: an ensemble method for fast and accurate linear trajectory inference from single-cell RNA-seq data
>> https://academic.oup.com/bioinformatics/advance-article/doi/10.1093/bioinformatics/btab831/6458323
scShaper, a new trajectory inference method that enables accurate linear trajectory inference. The ensemble approach of scShaper generates a continuous smooth pseudotime based on a set of discrete pseudotimes.
scShaper is a fast method with few hyperparameters, making it a promising alternative to the principal curves method for linear pseudotemporal ordering.
scShaper is based on graph theory and solves the shortest Hamiltonian path of a clustering, utilizing a greedy algorithm to permute clusterings computed using the k-means method to obtain a set of discrete pseudotimes.

□ GNNImpute: An efficient scRNA-seq dropout imputation method using graph attention network
>> https://bmcbioinformatics.biomedcentral.com/articles/10.1186/s12859-021-04493-x
GNNImpute, an autoencoder structure network that uses graph attention convolution to aggregate multi-level similar cell information and implements convolution operations on non-Euclidean space.
GNNImpute compensates for the lack of low expression intensity of some genes by aggregating the features information of similar cells. It can recover the dropout events in the scRNA-seq data and remain the specificity between cells to avoid excessive smoothing of expression.
GNNImpute can accurately and effectively impute the dropout and reduce dropout noise. GNNImpute enables the expression of the cells in the same tissue area to be embedded in low-dimensional vectors.

□ scBERT: a Large-scale Pretrained Deep Langurage Model for Cell Type Annotation of Single-cell RNA-seq Data
>> https://www.biorxiv.org/content/10.1101/2021.12.05.471261v1.full.pdf
scBERT (single-cell Bidirectional Encoder Representations from Transformers) follows the state-of-the-art paradigm of pre-train and fine-tune in the deep learning field.
scBERT formulates the expression profile of each single cell into embeddings for genes. scBERT computes the probability for the provided cell to be any cell type labelled in the reference dataset.
scBERT keeps the full gene-level interpretation, abandons the use of HVGs and dimensionality reduction, and lets discriminative genes and useful interaction come to the surface by themselves.
scBERT allows for the discovery of gene expression patterns that account for cell type annotation in an unbiased data-driven manner. scBERT pioneered the application of Transformer architectures in scRNA-seq data analysis with innovatively designed embeddings for genes.

□ GINCCo: Unsupervised construction of computational graphs for gene expression data with explicit structural inductive biases
>> https://academic.oup.com/bioinformatics/advance-article-abstract/doi/10.1093/bioinformatics/btab830/6458322
GINCCo (Gene Interaction Network Constrained Construction), an unsupervised method for automated construction of computational graph models for gene expression data that are structurally constrained by prior knowledge of gene interaction networks.
Each of the entities in the GINCCo computational graph represent biological entities such as genes, candidate protein complexes and phenotypes instead of arbitrary hidden nodes of a neural network.
GINCCo performs the model construction in a completely automated and deterministic; this can be seen as a preprocessing step allowing GINCCo to scale immensely and study factor graphs without the influence of task specific optimization dictating the shape of the models.

□ sciCAN: Single-cell chromatin accessibility and gene expression data integration via Cycle-consistent Adversarial Network
>> https://www.biorxiv.org/content/10.1101/2021.11.30.470677v1.full.pdf
sciCAN removes modality differences while keeping true biological variation. the model architecture of sciCAN, which contains two major components, representation learning and modality alignment.
sciCAN doesn’t require cell anchors and thus, it can be applied to most non-joint profiled single-cell data. sciCAN enabled us to co-embed and co- cluster RNA-seq and ATAC-seq data. sciCAN reduces each dataset into 128-dimension spaces.

□ propeller: testing for differences in cell type proportions in single cell data
>> https://www.biorxiv.org/content/10.1101/2021.11.28.470236v1.full.pdf
propeller, a robust and flexible method that leverages biological replication to find statistically significant differences in cell type proportions between groups.
Propeller leverages biological replication to estimate the high sample- to-sample variability in cell type counts often observed in real single cell data.
The minimal annotation information that propeller requires for each cell is cluster/cell type, sample and group/condition, which can be automatically extracted from Seurat and SingleCellExperiment class objects.
The propeller function calculates cell type proportions for each biological replicate, performs a variance stabilising transformation on the matrix of proportions and fits a linear model for each cell type or cluster using the limma framework.
□ AlphaFill: enriching the AlphaFold models with ligands and co-factors
>> https://www.biorxiv.org/content/10.1101/2021.11.26.470110v1.full.pdf
AlphaFill, an algorithm based on sequence and structure similarity, to “transplant” such “missing” small molecules and ions from experimentally determined structures. AlphaFill should be complemented by structure-based transfer algorithms.
The sequence of the AlphaFold model is BLASTed8 against the sequence file of the LAHMA webserver9 which contains all sequences present in the PDB-REDO databank. The hits are sorted by E-value and a maximum of 250 hits, as is the default for BLAST, is returned.
The selection of hits is then structurally aligned, based on the Cα-atoms of the residues matched in the BLAST8 alignment. The root-mean-square deviation (RMSD) of this global alignment is stored in the AlphaFill metadata.
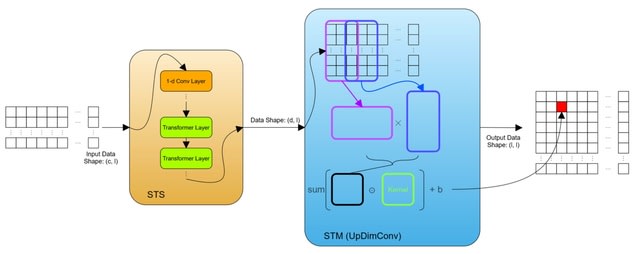
□ HiCArch: A Deep Learning-based Hi-C Data Predictor
>> https://www.biorxiv.org/content/10.1101/2021.11.26.470146v1.full.pdf
HiCArch, a transformer-based model architecture for Hi-C contact matrices prediction based on the 11 types of K562 epigenomic features, consisting of chromatin binding factors and histone modifications.
HiCArch processes the sequential input and generates the 2D Hi-C matrix via two main modules: sequence-to-sequence (seqToSeq, or STS) module, sequence-to-matrix (seqToMat, or STM) module.
□ propeller: testing for differences in cell type proportions in single cell data
>> https://www.biorxiv.org/content/10.1101/2021.11.28.470236v1.full.pdf
propeller, a robust and flexible method that leverages biological replication to find statistically significant differences in cell type proportions between groups.
Propeller leverages biological replication to estimate the high sample- to-sample variability in cell type counts often observed in real single cell data. The minimal annotation information that propeller requires for each cell is cluster/cell type, sample and group/condition, which can be automatically extracted from Seurat and SingleCellExperiment class objects.
The propeller function calculates cell type proportions for each biological replicate, performs a variance stabilising transformation on the matrix of proportions and fits a linear model for each cell type or cluster using the limma framework.

□ Predicting environmentally responsive transgenerational differential DNA methylated regions (epimutations) in the genome using a hybrid deep-machine learning approach
>> https://bmcbioinformatics.biomedcentral.com/articles/10.1186/s12859-021-04491-z
a hybrid DL-ML approach that uses a deep neural network for extracting molecular features and a non-DL classifier to predict environmentally responsive transgenerational differential DNA methylated regions (DMRs), termed epimutations, based on the extracted DL-based features.
The process of generating features is supervised. A 1000 bp input DNA sequence is one-hot encoded using a 5 × 1000 binary matrix. After each convolutional layer is a batch-normalization layer following by a ReLU transformer layer.

□ Navigating the pitfalls of applying machine learning in genomics
>> https://www.nature.com/articles/s41576-021-00434-9
Jacob Schreiber:
Although this high-level explanation covers our main point, we describe five specific (related) pitfalls that one can encounter in this space through the lens of train/test/prediction sets to drive home how common it is to make a mistake in an evaluation setting.
Importantly: CROSS-FOLD VALIDATION IS NOT THE SOLUTION. In fact, blindly applying cross-fold validation to biological data without thinking about your anticipated use case (the prediction set) can give you a false sense of security in the face of complexity.
□ Codex DNA increases productivity & efficiency of mRNA synthesis, launching BioXP kits with CleanCap Reagent AG
Automated platform accelerates development of mRNA-based #vaccines & therapies
>> https://codexdna.com/products/bioxp-kits/mrna-synthesis/
□ KaKs_Calculator 3.0: calculating selective pressure on coding and non-coding sequences
>> https://www.biorxiv.org/content/10.1101/2021.11.25.469998v1.full.pdf
Similar to the nonsynonymous/synonymous substitution rate ratio for coding sequences, selection on non-coding sequences can be quantified as non-coding nucleotide substitution rate normalized by synonymous substitution rate of adjacent coding sequences.
KaKs_Calculator detects the mode of selection operated on molecular sequences, accordingly demonstrating its great potential to achieve genome-wide scan of natural selection on diverse sequences and identification of potentially functional elements at whole genome scale.

□ Systematic evaluation of cell-type deconvolution pipelines for sequencing-based bulk DNA methylomes
>> https://www.biorxiv.org/content/10.1101/2021.11.29.470374v1.full.pdf
All compared sequencing-based methods consist of two common steps, informative region selection and cell-type composition estimation.
In the informative region selection step, the sequencing-based cell-type deconvolution methods filter out CpGs where the methylation patterns do not clearly demonstrate cell-type heterogeneity.
Whereas selecting similar genomic regions to DMRs generally contributed to increasing the performance in bi-component mixtures, the uniformity of cell-type distribution showed a high correlation with the performance in five cell-type bulk analyses.

□ GraphPrompt: Biomedical Entity Normalization Using Graph-based Prompt Templates
>> https://www.biorxiv.org/content/10.1101/2021.11.29.470486v1.full.pdf
OBO-syn encompasses 70 biomedical entity types and 2 million entity- synonym pairs. OBO-syn has demonstrated small overlaps with existing datasets and more challenging entity-synonym predictions.
GraphPrompt, a prompt-based learning method for entity normalization with the consideration of graph structures. GraphPrompt solves a masked-language model task. GraphPrompt has obtained superior performance to the other approaches on both few-shot and zero-shot settings.
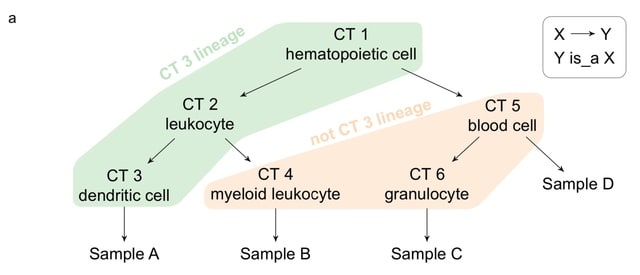
□ CLA: Automated identification of cell-type–specific genes and alternative promoters
>> https://www.biorxiv.org/content/10.1101/2021.12.01.470587v1.full.pdf
Cell Lineage Analysis (CLA), a computational method which identifies transcriptional features with expression patterns that discriminate cell types, incorporating Cell Ontology knowledge on the relationship between different cell types.
CLA uses random forest classification with a stratified bootstrap to increase the accuracy of binary classifiers when each cell type have a different number of samples.
CLA runs multiple instances of regularized random forest and reports the transcriptional features consistently selected. CLA not only discriminates individual cell types but can also discriminate lineages of cell types related in the developmental hierarchy.

□ CSmiR: Exploring cell-specific miRNA regulation with single-cell miRNA-mRNA co-sequencing data
>> https://bmcbioinformatics.biomedcentral.com/articles/10.1186/s12859-021-04498-6
CSmiR (Cell-Specific miRNA regulation) to combine single-cell miRNA-mRNA co-sequencing data and putative miRNA-mRNA binding information to identify miRNA regulatory networks at the resolution of individual cells.
CSmiR is effective in predicting cell-specific miRNA targets. Finally, through exploring cell–cell similarity matrix characterized by cell-specific miRNA regulation, CSmiR provides a novel strategy for clustering single-cells and helps to understand cell–cell crosstalk.

□ CombSAFE: Identification, semantic annotation and comparison of combinations of functional elements in multiple biological conditions
>> https://academic.oup.com/bioinformatics/advance-article/doi/10.1093/bioinformatics/btab815/6448225
CombSAFE allows analyzing the whole genome, by clustering patterns of regions with similar functional elements and through enrichment analyses to discover ontological terms significantly associated with them.
CombSAFE allows comparing functional states of a specific genomic region to analyze their different behavior throughout the various semantic annotations.

□ KAGE: Fast alignment-free graph-based genotyping of SNPs and short indels
>> https://www.biorxiv.org/content/10.1101/2021.12.03.471074v1.full.pdf
Since traditional reference genomes do not include genetic variation, traditional genotypers suffer from reference bias and poor accuracy in variation-rich regions where reads cannot accurately be mapped.
These methods work by representing genetic variants by their surrounding kmers (sequences with length k covering each variant) and looking for support for these kmers in the sequenced reads.
KAGE, a genotyper for SNPs and short indels that is inspired by recent developments within graph-based genome representations and alignment-free genotyping.

□ FastMLST: A Multi-core Tool for Multilocus Sequence Typing of Draft Genome Assemblies
>> https://journals.sagepub.com/doi/10.1177/11779322211059238
FastMLST, a tool that is designed to perform PubMLST searches using BLASTn and a divide-and-conquer approach that processes each genome assembly in parallel.
The output offered by FastMLST includes a table with the ST, allelic profile, and clonal complex or clade (when available), detected for a query, as well as a multi-FASTA file or a series of FASTA files with the concatenated or single allele sequences detected.
FastMLST assigns STs to thousands of genomes in minutes with 100% concordance in genomes without suspected contamination in a wide variety of species with different genome lengths, %GC, and assembly fragmentation levels.

□ TRAWLING: a Transcriptome Reference Aware of spLIciNG events.
>> https://www.biorxiv.org/content/10.1101/2021.12.03.471115v1.full.pdf
TRAWLING simplifies the identification of splicing events from RNA-seq data in a simple and fast way, while leveraging the suite of tools developed for alignment-free methods. it allows the aggregation of read counts based on the donor and acceptor splice motifs.
TRAWLING using three different RNA sequencing datasets: whole transcriptome sequencing, single cell RNA sequencing and Digital RNA w/ pertUrbation of Genes. TRAWLING did not misalign or lose reads, it can be used by default w/o loss of generality for gene level quantification.

□ DARTS: an Algorithm for Domain-Associated RetroTransposon Search in Genome Assemblies
>> https://www.biorxiv.org/content/10.1101/2021.12.03.471067v1.full.pdf
DARTS has radically higher sensitivity of long terminal repeat retrotransposons (LTR-RTs) identification compared to a widely accepted LTRharvest tool.
DARTS returns a set of structurally annotated nucleotide and amino acid sequences which can be readily used in subsequent comparative and phylogenetic analyses.
□ pystablemotifs: Python library for attractor identification and control in Boolean networks
>> https://academic.oup.com/bioinformatics/advance-article/doi/10.1093/bioinformatics/btab825/6454946
pystablemotifs is a Python 3 library for analyzing Boolean networks. Its non-heuristic and exhaustive attractor identification algorithm was previously presented in (Rozum et al. 2021).
Illustrating its performance improvements over similar methods and discuss how it uses outputs of the attractor identification process to drive a system to one of its attractors from any initial state.

□ CMash: fast, multi-resolution estimation of k-mer-based Jaccard and containment indices
>> https://www.biorxiv.org/content/10.1101/2021.12.06.471436v1.full.pdf
Combining a modified MinHash technique (ArgMinHash) and a data structure called a k-mer ternary search tree (KTST), which allows Jaccard and containment indices to be computed at multiple k-mer sizes efficiently and simultaneously.
This truncation approach circumvents the reconstruction of new k-mer sets when changing k values, making analysis more time and space-efficient.
CMash estimate of the Jaccard and containment index does not deviate significantly from the ground truth, indicating that this approach can give fast and reliable results with minimal bias.

□ Genovo: A method to build extended sequence context models of point mutations and indels
>> https://www.biorxiv.org/content/10.1101/2021.12.06.471476v1.full.pdf
a new method that solves this problem by grouping similar k-mers using IUPAC patterns. It calculates a table with the number of times each possible k-mer is observed with the central base mutated and unmutated.
Genovo predicts the expected number of synonymous, missense, and other functional mutation types for each gene. the created mutation rate models increase the statistical power to detect genes containing disease-causing variants and to identify genes under strong constraint.

□ DALI (Diversity AnaLysis Interface): a novel tool for the integrated analysis of multimodal single cell RNAseq data and immune receptor profiling.
>> https://www.biorxiv.org/content/10.1101/2021.12.07.471549v1.full.pdf
Diversity AnaLysis Interface (DALI) interacts with the Seurat R package and is aimed to support the advanced bioinformatician with a set of novel methods and an easier integration of existing tools for BCR and TCR analysis in their single cell workflow.

□ LEXAS: a web application for life science experiment search and suggestion
>> https://www.biorxiv.org/content/10.1101/2021.12.05.471323v1.full.pdf
LEXAS (Life-science EXperiment seArch and Suggestion) curates the description of biomedical experiments and suggests the experiments on genes that could be performed next.
LEXAS allows users to choose between two machine learning models that are used for the suggestion. One is a “reliable” model that uses seven major biomedical databases such as the BioGRID and four knowledgebases such as the Gene Ontology.

□ MCKAT: a multi-dimensional copy number variant kernel association test
>> https://bmcbioinformatics.biomedcentral.com/articles/10.1186/s12859-021-04494-w
MCKAT utilizes both multi-dimensional features of the CNVs & their heterogeneity effect. The MCKAT is not only capable of indicating stronger evidence in detecting significant associations b/n CNVs & disease-related traits, but it is applicable to both rare & common CNV datasets.
※コメント投稿者のブログIDはブログ作成者のみに通知されます