
□ Nexus: Pan-genome de Bruijn graph using the bidirectional FM-index
>> https://bmcbioinformatics.biomedcentral.com/articles/10.1186/s12859-023-05531-6
Nexus, a memory-efficient representation of the colored compacted de Bruijn graph enabling subgraph visualization and lossless approximate pattern matching of reads to the graph, developed to store pan-genomes.
Nexus provides other functionalities (such as visualization) next to read alignment. In contrast to a k-mer hash table, both the A4 algorithm by Beller and Ohlebusch and Nexus are based on a full-text index of the concatenation of all input genomes.


□ VRP Assembler: haplotype-resolved de novo assembly of diploid and polyploid genomes using quantum computing
>> https://www.biorxiv.org/content/10.1101/2023.10.19.563028v1
VRP assembler, a haplotype assembly method to combines both phasing and assembly process into a single optimization model. It enables the optimization procedure to be solved on quantum annealers as well as gate-based quantum computers to harness potential quantum acceleration.
The core system in quantum annealing for VRP is a time-dependent Hamiltonian of transverse-field Ising model. The reconstructed sequences exactly match the original sequences with zero hamming distance in all runs.
The VRP assembler has demonstrated its potential and feasibility through a proof of concept on short synthetic diploid and triploid genomes using a D-Wave quantum annealer.

□ Rosace: a robust deep mutational scanning analysis framework employing position and mean-variance shrinkage
>> https://www.biorxiv.org/content/10.1101/2023.10.24.562292v1
Rosace, the first growth-based Deep Mutational Scanning method that incorporates local positional information. Rosace attempts to simulate several properties of DMS such as bimodality, similarities in behavior across similar substitutions, and the overdispersion of counts.
Rosace uses Rosette to simulate several screening modalities. Rosace implements a hierarchical model that parameterizes each variant's effect as a function of the positional effect, providing a way to incorporate both position-specific information and shrinkage into the model.

□ AAMB: Adversarial and variational autoencoders improve metagenomic binning
>> https://www.nature.com/articles/s42003-023-05452-3
AAMB (Adversarial Autoencoders for Metagenomic Binning), an extension of the VAMB program. AAMB leverages AAEs to yield more accurate bins than VAMB’s VAE-based approach.
AAMB consists of: Tetra Nucleotide Frequencies (TNF) and per sample co-abundances are extracted from the contigs and BAM files of reads mapped to contigs, and input to the AAMB model as a concatenated vector. AAMB uses both a continuous and a categorical latent space.

□ A Safety Framework for Flow Decomposition Problems via Integer Linear Programming
>> https://academic.oup.com/bioinformatics/advance-article/doi/10.1093/bioinformatics/btad640/7325350
mfd-safety is a tool reporting maximal safe paths for minimum flow decompositions (mfd) using Integer Linear Programming (ILP) calls, and implementing several optimization to reduce the number of ILP calls or their size (number of variables/constrains).
Computing the weighted precision of a graph as the average weighted precision over all reported paths in the graph, and the maximum coverage of a graph as the average maximum coverage over all ground truth paths in the graph.
The two algorithms for finding all maximal safe paths. Both algorithms use a similar approach, however the first uses a top-down approach starting from the original full solution paths and reports all safe paths, and then trims all the unsafe paths to find new maximal safe paths.

□ aMeta: an accurate and memory-efficient ancient metagenomic profiling workflow
>> https://genomebiology.biomedcentral.com/articles/10.1186/s13059-023-03083-9
aMeta, an accurate metagenomic profiling workflow for ancient DNA designed to minimize the amount of false discoveries and computer memory requirements. aMeta consumed nearly half as much computer memory as Heuristic Operations for Pathogen Screening.
Meta represents a combination of taxonomic classification steps with KrakenUniq. aMeta performs alignments with the MALT aligner. The main advantage of MALT and motivation for us to use it in aMeta was that MALT is a metagenomic-specific aligner which applies the LCA algorithm.

□ Capricorn: Enhancing Hi-C contact matrices for loop detection with Capricorn, a multi-view diffusion model
>> https://www.biorxiv.org/content/10.1101/2023.10.25.564065v1
They hypothesize that resolution enhancement can produce contact matrices that can better capture these higher-order chromatin structures if we design a loss function that explicitly models structures like loops and TADs during resolution enhancement.
Capricorn incorporates additional biological views of the contact matrix to emphasize important chromatin interactions and leverages powerful computer vision diffusion models for the model backbone.
Capricorn learns a diffusion model that enhances a five-channel image, containing both the primary Hi-C matrix as well as representations of TADs, loops, and distance-normalized counts computed from the original low-resolution matrix.

□ dsRID: in silico identification of dsRNA regions using long-read RNA-seq data
>> https://academic.oup.com/bioinformatics/advance-article/doi/10.1093/bioinformatics/btad649/7328386
dsRID detects dRNA regions in an editing-agnostic manner. dsRID is built upon a previous observation and others that dRNA structures may induce region-skipping in RNA-seq reads, an artifact likely reflecting intra-molecular template switching in reverse transcription.
dsRNAs are potent triggers of innate immune responses upon recognition by cytosolic dsRNA sensor proteins. Identification of endogenous dsRNAs is critical to better understand the dsRNAome and its relevance to innate immunity related to human diseases.

□ TBLMM: Bayesian linear mixed model with multiple random effects for prediction analysis on high-dimensional multi-omics data
>> https://academic.oup.com/bioinformatics/advance-article/doi/10.1093/bioinformatics/btad647/7330404
TBLMM (a two-step Bayesian Linear mixed model for predictive modeling of multi-omics data) uses BLMM-based integrative framework to fuse multiple designated kernel functions, which can account for heterogeneous effects and interactions, into one kernel for each genomic region.
TBLMM uses random effect terms to capture both within omics interactions, where the variance-covariance is modeled using three non-linear kernels, including polynomial kernel with 2 degrees of freedom, the neural network kernel, and the Hadamard product between linear kernels.

□ Equivariant flow matching
>> https://arxiv.org/abs/2306.15030
A novel flow matching objective designed for invariant densities, yielding optimal integration paths. Additionally, they introduce A new invariant dataset of alanine dipeptide and a large Lennard-Jones cluster.
The Boltzmann Generator capable of producing samples from the equilibrium Boltzmann distribution of a molecule in Cartesian coordinates. This method exploits the physical symmetries of the target energy simulation-free training of equivariant continuous normalizing flows.

□ Flow-Lenia: Towards open-ended evolution in cellular automata through mass conservation and parameter localization
>> https://arxiv.org/abs/2212.07906
Some spatially localized patterns (SLPs) resemble life-like artificial creatures and display complex behaviors. However, those creatures are found in only a small subspace of the Lenia parameter space and are not trivial to discover, necessitating advanced search algorithms.
Flow Lenia can integrate the parameters of the Cellular Automata update rules within the CA dynamics, allowing for multi-species simulations, w/ locally coherent update rules that define properties of the emerging creatures, and that can be mixed with neighbouring rules.

□ CONE: COntext-specific Network Embedding via Contextualized Graph Attention
>> https://www.biorxiv.org/content/10.1101/2023.10.21.563390v1
The core component of CONE consists of a graph attention network with contextual conditioning, and it is trained in a noise contrastive fashion using contextualized interactome random walks localized around contextual genes.
CONE contains two main components, including a GN decoder and an MLP context encoder. The GNN decoder converts the raw, learnable, node embeddings into the final embeddings.
On the other hand, the MLP context encoder projects the context-specific similarity profile that describes the relationships among different contexts into a condition embedding.
When added with the raw embeddings, the condition embedding serves as a high-level contextual semantics, similar to the widely-used positional encodings in Transformer models.

□ GNorm2: an improved gene name recognition and normalization system
>> https://academic.oup.com/bioinformatics/advance-article/doi/10.1093/bioinformatics/btad599/7329714
GNorm2 integrates a range of advanced deep learning-based methods, resulting in the highest levels of accuracy and efficiency for gene recognition and normalization to date.
GNorm2 utilizes the Transformer-based infrastructure to recognize gene names mentioned in free text instead of Conditional Random Fields.
Bioformer is a language model based on the BERT architecture that is tailored for biomedical text mining. It employs a specialized vocabulary and reduces the model size by 60% compared to the original BERT, making it much more computationally efficient.

□ GRAIGH: Gene Regulation accessibility integrating GeneHancer database
>> https://www.biorxiv.org/content/10.1101/2023.10.24.563720v1
GRAIGH, a novel computational approach to interpret scATAC-seq features and understand the information they provide. GRAIGH aims to integrate scATAC-seq datasets with the GenHancer database, which describes genome-wide enhancer-to-gene and promoter-to-gene associations.
These associations have unique identifiers which have the potential to overcome one of the limitations of the scATAC-seq data, thus enabling interoperability of datasets obtained from different experiments.
GRAIGH is validated by comparing the results obtained from the GH matrix data with the original scATAC-seq data, showing the integration does not introduce any significant biases.

□ CoreDetector: A flexible and efficient program for core-genome alignment of evolutionary diverse genomes
>> https://academic.oup.com/bioinformatics/advance-article/doi/10.1093/bioinformatics/btad628/7329718
CoreDetector generates a multiple core-genome alignment for closely and more distantly related genomes. A single longest genome with the least number of ambiguous bases (non ATGC) is initially selected as the query from the pool of genomes for pairwise alignment using Minimap2.
CoreDetector computationally scaled from the diploid smaller fungal pathogen to larger rodent and hexaploid plant genomes without the need for high-performance computing (HPC) resources, and in the case of the larger and more diverse rodent dataset.

□ Pumping the brakes on RNA velocity by understanding and interpreting RNA velocity estimates
>> https://genomebiology.biomedcentral.com/articles/10.1186/s13059-023-03065-x
Deconstructing the underlying workflow by separating the (gene-level) velocity estimation from the vector field visualization. Their findings reveal a significant dependence of the RNA velocity workflow on smoothing via the k-nearest-neighbors (k-NN) graph of the observed data.
They analyzed how the methods for mapping and visualizing the vector field impact the interpretation of RNA velocity and discover the central role played by the k-NN graph in both velocity estimation and vector field visualization.

□ The Quartet Data Portal: integration of community-wide resources for multiomics quality control
>> https://genomebiology.biomedcentral.com/articles/10.1186/s13059-023-03091-9
The Quartet Data Portal facilitates community access to well-characterized reference materials / datasets, and related resources. Users can request DNA, RNA, protein, and reference materials, as well as datasets generated across omics, platforms, labs, protocols, and batches.
The Quartet Data Portal uses a “distribution-collection-evaluation-integration” closed-loop workflow. Continuous requests for reference materials by the community will generate large amounts of data from the Quartet reference samples under different platforms and labs.

□ AMAS: An Automated Model Annotation System for SBML Models
>> https://academic.oup.com/bioinformatics/advance-article/doi/10.1093/bioinformatics/btad658/7330406
AMAS may produce an empty prediction set. This occurs if the query element is rejected by the Element Filter. It also occurs if the largest match score for the query element is smaller than the match score cutoff.
AMAS calculates the similarity between two species based on the similarity of strings associated with the two species. For the query species, the preferred strings is the SBML display name if it exists.

□ deltaXpress (ΔXpress): a tool for mapping differentially correlated genes using single-cell qPCR data
>> https://bmcbioinformatics.biomedcentral.com/articles/10.1186/s12859-023-05541-4
ΔXpress uses cycle threshold (Ct) values and categorical information for each sample. ΔXpress emulates a bulk analysis by observing differentially expressed genes. It allows the discovery of pairwise genes differentially correlated when comparing two experimental conditions.
ΔXpress uses the NormFinder algorithm. The NormFinder algorithm will show two gene lists (single and paired) with their respective stability values. ΔXpress use the best pair of genes to calculate the mean value per sample and normalize all genes using the Livak method.

□ BIDARA: Bio-Inspired Design and Research Assistant (NASA)
>> https://www1.grc.nasa.gov/research-and-engineering/vine/petal/
BIDARA can guide users through the Biomimicry Institute’s Design Process, a step-by-step method to propose biomimetic solutions using Generative AI. This process includes defining the problem, biologizing the challenge, discovering natural models, and emulating the strategies.
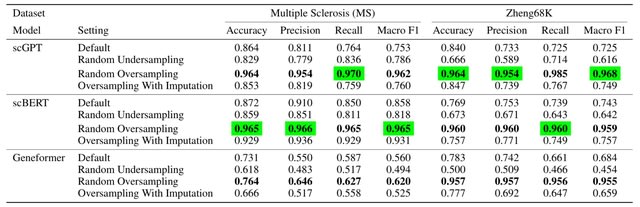
□ Foundation Models Meet Imbalanced Single-Cell Data When Learning Cell Type Annotations
>> https://www.biorxiv.org/content/10.1101/2023.10.24.563625v1
Benchmarking foundation models, scGPT, scBERT, and Geneformer, for cell-type annotation. scGPT, using FlashAttention, has the fastest computational speed, whereas scBERT is much more memory-efficient.
Notably, in contrast to scGPT and scBERT, Geneformer uses ordinal positions of the tokenized genes rather than actual raw gene expression values. Random oversampling, but not random undersampling, improved the performance for all three foundation models.

□ JIVE: Joint and Individual Variation Explained: Batch-effect correction in single-cell RNA sequencing data
>> https://www.biorxiv.org/content/10.1101/2023.10.25.563973v1
JIVE, a multi-source dimension reduction method that decomposes two or more biological datasets into three low-rank approximation components: a joint structure among the datasets, individual structures unique to each distinct dataset, and residual noise.
The JIVE decomposition estimates the joint and individual structures by minimizing the sum of squared error of the residual matrix.
Given an initial estimate for the joint structure, it finds the individual structures to minimize the sum of squared error. Then, given the new individual structures, it finds a new estimate for the joint structure which minimizes the sum of squared error.
The original R. JIVE code utilizes singular value decompositions (SVD) in many different areas, however JIVE uses a partial SVD function which returns the largest singular values/vectors of a given matrix.

□ Flash entropy search to query all mass spectral libraries in real time
>> https://www.nature.com/articles/s41592-023-02012-9
Public repositories of metabolomics mass spectra encompass more than 1 billion entries. With open search, dot product or entropy similarity, comparisons of a single tandem mass spectrometry spectrum take more than 8 h.
Flash entropy search speeds up calculations more than 10,000 times to query 1 billion spectra in less than 2 s, without loss in accuracy. It benefits from using multiple threads and GPU calculations.


□ Linked-Pair Long-Read Sequencing Strategy for Targeted Resequencing and Enrichment
>> https://www.biorxiv.org/content/10.1101/2023.10.26.564243v1
A linked-pair sequencing strategy. This approach relies on generating library-sized DNA fragments from long DNA molecules such that the 300-1000 bp at the ends of the adjacent DNA fragments are duplicated.
A long contiguous DNA molecule was non-randomly fragmented into many smaller fragments in such a way that the ends of the fragments shared the specific identical sequences up to 1000 bp, called linkers or linker sequences.
The sequencing library constructed using these fragments maintains the contiguity of reads through the tandem duplicated sequences at fragment ends and improves the sequencing efficiency of targeted regions.

□ Rank and Select on Degenerate Strings
>>
Recently, Alanko et al. generalized the rank-select problem to degenerate strings, where given a character c and position i the goal is to find either the ith set containing c or the number of occurrences of c in the first i sets.
The problem has applications to pangenomics; in another work by Alanko et al. they use it as the basis for a compact representation of de Bruijn Graphs that supports fast membership queries.
They revisit the rank-select problem on degenerate strings, providing reductions to rank-select on regular strings. Plugging in standard data structures, they improve the time bounds for queries exponentially while essentially matching, or improving, the space bounds.

□ CluStrat: Structure-informed clustering for population stratification in association studies
>> https://bmcbioinformatics.biomedcentral.com/articles/10.1186/s12859-023-05511-w
CluStrat, which corrects for complex arbitrarily structured populations while leveraging the linkage disequilibrium induced distances between genetic markers. It performs an agglomerative hierarchical clustering using the Mahalanobis distance covariance matrix of the markers.
The regularized Mahalanobis distance-based GRM used in CluStrat has a straightforward yet possibly not widely recognized connection with the leverage and cross-leverage scores, which becomes particularly interesting when applied to the genotype matrix.
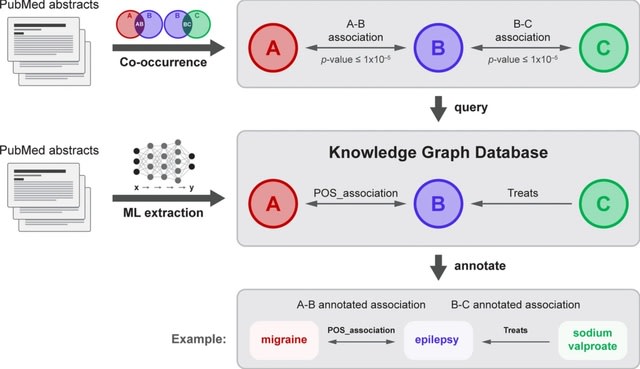
□ Serial KinderMiner (SKiM) discovers and annotates biomedical knowledge using co-occurrence and transformer models
>> https://bmcbioinformatics.biomedcentral.com/articles/10.1186/s12859-023-05539-y
SKiM performs LBD searches to discover relationships between arbitrary user-defined concepts. SKiM is generalized for any domain, can perform searches with many thousands of C term concepts, and moves beyond the simple identification of an existence of a relationship.
The knowledge graph, built by extracting biomedical entities and relationships from PubMed abstracts with ML, is queried for the A–B and B–C relationships. If these are found in the database, the relationships that SKiM found are annotated.

□ Matrix and analysis metadata standards (MAMS) to facilitate harmonization and reproducibility of single-cell data
>> https://www.biorxiv.org/content/10.1101/2023.03.06.531314v1
MAMS captures the relevant information about the data matrices. MAMS defines fields that describe what type of data is contained within a matrix, relationships between matrices, and provenance related to the algorithm that created the matrix.
Feature and observation matrices (FOMs) contain biological data at different stages of processing incl. reduced dimensional representations. Metadata fields for the other classes were defined in MAMS. Fields are incl. to denote if an ID is a compound ID separated by a delimiter.

□ MedCPT: Contrastive Pre-trained Transformers with Large-scale PubMed Search Logs for Zero-shot Biomedical Information Retrieval
>> https://academic.oup.com/bioinformatics/advance-article/doi/10.1093/bioinformatics/btad651/7335842
MedCPT, a first-of-its-kind Contrastively Pre-trained Transformer model for zero-shot semantic IR in biomedicine. MedCPT re-ranker is trained with the negative distribution sampled from the pre-trained MedCPT retriever.
MedCPT contains a query encoder (QEnc), a document encoder (DEnc), and a cross-encoder (CrossEnc). The query encoder and document encoder compose of the MedCPT retriever, which is contrastively trained by 255M query-article pairs and in-batch negatives from PubMed logs.

□ Next-generation phenotyping: Introducing phecodeX for enhanced discovery research in medical phenomics
>> https://academic.oup.com/bioinformatics/advance-article/doi/10.1093/bioinformatics/btad655/7335839
phecodeX, an expanded version of phecodes with a revised structure and 1,761 new codes. PhecodeX adds granularity to phenotypes in key disease domains that are underrepresented in the current phecode structure.
PhecodeX 1) aligns its structure with the ICD-10 coding system, 2) revises the phecode labeling system, 3) leverages multi-mapping of both ICD-9 and -10 codes, 4) removes exclude ranges used to define controls, and 5) reorganizes phecode categories.

□ Oxford Nanopore
>> http://nanoporetech.com/about-us/news/blog-oxford-nanopore-meets-apples-m3-silicon-chip-hailing-new-era-distributed-genome
Today @Apple highlighted how their M3 silicon chip provides powerful, accessible compute — citing the ability to run the complex analysis required for DNA/RNA #nanopore sequencing, by anyone, anywhere in the world.
□ Coste energético de la bioinformática
>> https://bioinfoperl.blogspot.com/2023/10/coste-energetico-de-la-bioinformatica.html
□ Veera Rejagopal
>> https://www.businesswire.com/news/home/2023
Something big happened a few days ago. Industry leaders in the genomics fields (Regeneron, AstraZeneca, Novo Nordisk, Roche) announced their collaboration with the US's largest black medical school, Meharry Medical College, Nashville, to establish what might become the UK Biobank of Africa--the largest genomics database of 500,000 volunteers from African ancestries.
※コメント投稿者のブログIDはブログ作成者のみに通知されます