
-Changeling.
“We shall not cease from exploration
And the end of all our exploring
Will be to arrive where we started
And know the place for the first time.”
- T.S. Eliot, Four Quartets
「人は冒険をやめてはならぬ
長い冒険の果てに
出発点へ辿り着くのだから
そして 初めて居場所を知るのだ」
ーT・S・エリオット『四つの四重奏』
“If they were complicated enough, both sides could sustain observers who would perceive time going in opposite directions. Any intelligent beings there would define their arrow of time as moving away from this central state. They would think we now live in their deepest past." -Julian Barbour
時間反転した宇宙において観測される物理事象に対称性があると仮定するなら、そこにいる観測者にとって定義された「時間の矢」が逆であっても、認識上では同じ方向に進んでいるはずである。したがって、彼らと私たちにとっては、「今この瞬間」が、お互いに最も遠い「過去」と「未来」となる。
言葉と感情は二分化出来ない。行為するという決定論的事象において感情を起因とするのは、発話という行為に認識を縛られているからに過ぎない。「動機」こそが後付けの概念なのだ。それでも影踏みを止めることはない。理由を探すということ自体、アトラクターに組み込まれている「理由」があるからだ。
そして私たちは、「今」を証明をするために、未来を生きる。


□ GARFIELD classifies disease-relevant genomic features through integration of functional annotations with association signals:
>> https://www.nature.com/articles/s41588-018-0322-6
GARFIELD is a novel approach that leverages genome-wide association studies’ findings with regulatory or functional annotations to classify features relevant to a phenotype of interest. they assess enrichment of genome-wide association studies for 19 traits within Encyclopedia of DNA Elements- and Roadmap-derived regulatory regions. GARFIELD uncovered statistically significant enrichments for the majority of traits being considered, and highlighted clear differences in enrichment patterns between traits.

□ Apollo: A Sequencing-Technology-Independent, Scalable, and Accurate Assembly Polishing Algorithm:
>> https://arxiv.org/pdf/1902.04341.pdf
Apollo, a universal assembly polishing algorithm that is scalable to polish an assembly of any size with reads from all sequencing technologies. Apollo models an assembly as a profile hidden Markov model (pHMM), uses read- to-assembly alignment to train the pHMM with the Forward-Backward algorithm, and decodes the trained model with the Viterbi algorithm to produce a polished assembly.

□ Skyhawk: An Artificial Neural Network-based discriminator for reviewing clinically significant genomic variants:
>> https://www.biorxiv.org/content/biorxiv/early/2019/01/28/311985.full.pdf
Skyhawk, an artificial neural network-based discriminator that mimics the process of expert review on clinically significant genomics variants. Among the false positive singletons identified by GATK HaplotypeCaller, UnifiedGenotyper and 16GT in the HG005 GIAB sample, 79.7% were rejected by Skyhawk.
Skyhawk mimics how a human visually identifies genomic features comprising a variant and decides whether the evidence supports or contradicts the sequencing read alignments. Skyhawk repurposed the network architecture they developed in a previous study named Clairvoyante.
□ SORA: Scalable Overlap-graph Reduction Algorithms for Genome Assembly using Apache Spark in the Cloud:
>> https://ieeexplore.ieee.org/abstract/document/8621546
SORA adapts string graph reduction algorithms for the genome assembly using a distributed computing platform. To efficiently compute coverage for enormous paths, useing Apache Spark which is a cluster-based engine designed on top of Hadoop to handle large datasets in the cloud. The results show that SORA can process a nearly one billion edge graph in a distributed cloud cluster as well as smaller graphs on a local cluster with a short turnaround time. Their algorithms scale almost linearly with increasing numbers of virtual instances in the cloud.
□ CONSENT: Scalable self-correction of long reads with multiple sequence alignment:
>> https://www.biorxiv.org/content/biorxiv/early/2019/02/11/546630.full.pdf
CONSENT (sCalable self-cOrrectioN of long reads with multiple SEquence alignmeNT) is a self-correction method for long reads. It works by computing overlaps b/n the long reads, in order to define an alignment pile (a set of overlapping reads used for correction) for each read. CONSENT compares well to the latest state-of-the-art self-correction methods, and even outperforms them on real Oxford Nanopore datasets. CONSENT is the only method able to scale to a human dataset containing Oxford Nanopore ultra-long reads, reaching lengths up to 340 kbp.
□ Fast and accurate long-read assembly with wtdbg2:
>> https://www.biorxiv.org/content/biorxiv/early/2019/01/26/530972.full.pdf
a novel long-read assembler wtdbg2 that, for human data, is tens of times faster than published tools while achieving comparable contiguity and accuracy. Wtdbg2 broadly follows the overlap-layout-consensus paradigm. It advances the existing assemblers with a fast all-vs-all read alignment implementation and a novel layout algorithm based on fuzzy-Bruijn graph (FBG).
Wtdbg2 bins read sequences to speed up the next step in alignment: dynamic programming (DP). With 256bp binning, the DP matrix is 65536 (=256 ́256) times smaller than a per-base DP matrix. For all human data, wtdbg2 finishes the assembly in a few days on a single computer. This performance broadly matches the throughput of a PromethION machine.

□ RUV-z: A causal inference framework for estimating genetic variance and pleiotropy from GWAS summary data:
>> https://www.biorxiv.org/content/biorxiv/early/2019/01/28/531673.full.pdf
RUV-z (Removing Unwanted Variation in GWAS z-score matrix), with which we characterize undesired sources of information lurking in summary statistics, and selectively remove them to improve accuracy and statistical power of local variance/covariance calculation. zQTL (z-score based quantitative trait locus analysis), a suite of machine learning methods for summary-based regression and matrix factorization, then demonstrate how they can successively apply the factorization and regression steps to design a new confounder-correction method.
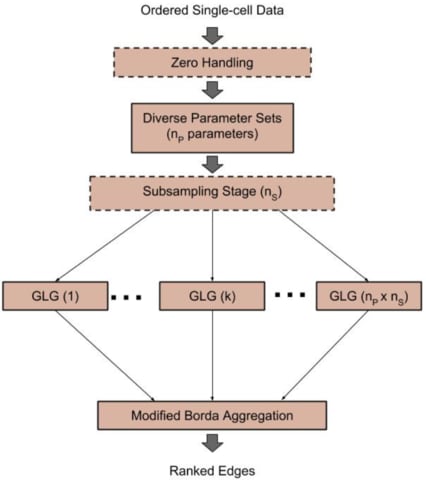
□ SCINGE: Network Inference with Granger Causality Ensembles on Single-Cell Transcriptomic Data:
>> https://www.biorxiv.org/content/biorxiv/early/2019/01/30/534834.full.pdf
Single-Cell Inference of Networks using Granger Ensembles (SCINGE) algorithm, an ensemble-based GRN reconstruction tech- nique that uses modified Granger Causality on single-cell data annotated with pseudotimes. Within SCINGE, GLG uses a kernel function to smooth the past expression values of candidate regulators, mitigating the irregularly-spaced pseudotimes and zero values that are prevalent in single- cell expression data.
SCINGE compares favorably with existing GRN inference methods designed for temporal or pseudotemporal GE data. it reveals important caveats about GRN evaluation and the value of pseudotime for GRN inference that are broadly applicable for pseudotime-based GRN reconstruction.
□ Causal network reconstruction from time series: From theoretical assumptions to practical estimation:
>> https://aip.scitation.org/doi/full/10.1063/1.5025050
The goal of causal network reconstruction or causal discovery is to distinguish direct from indirect dependencies and common drivers among multiple time series. A variety of different assumptions have been shown to be sufficient to estimate the true causal graph. focussing on three main assumptions under which the time series graph represents causal relations: Causal Sufficiency, the Causal Markov Condition, and Faithfulness.

□ USDL: A Unified Approach for Sparse Dynamical System Inference from Temporal Measurements:
>> https://academic.oup.com/bioinformatics/advance-article/doi/10.1093/bioinformatics/btz065/5305020
Unified Sparse Dynamics Learning (USDL), constitutes of two steps. First, an atemporal system of equations is derived through the application of the weak formulation. Then, assuming a sparse representation for the dynamical system, the inference problem can be expressed as a sparse signal recovery problem, allowing the application of an extensive body of algorithms and theoretical results.
Results on simulated data demonstrate the efficacy and superiority of the USDL algorithm under multiple interventions and/or stochasticity. Additionally, USDL’s accuracy significantly correlates with theoretical metrics such as the exact recovery coefficient. On real single-cell data, the proposed approach is able to induce high-confidence subgraphs of the signaling pathway.

□ LuxUS: Detecting differential DNA methylation using generalized linear mixed model with spatial correlation structure:
>> https://www.biorxiv.org/content/biorxiv/early/2019/02/01/536722.full.pdf
LuxGLM Using Spatial correlation (LuxUS) is a tool for differential methylation analysis. The tool is based on generalized linear mixed model with spatial correlation structure. The model parameters are fitted using probabilistic programming language Stan. Savage-Dickey Bayes factor estimates are used for statistical testing of a covariate of interest. LuxUS supports both continuous and binary variables. The model takes into account the experimental parameters, such as bisulfite conversion efficiency.

□ catch22: CAnonical Time-series CHaracteristics:
>> https://www.biorxiv.org/content/biorxiv/early/2019/01/28/532259.full.pdf
catch22 captures a diverse and interpretable signature of time series in terms of their properties, including linear and non-linear autocorrelation, successive differences, value distributions and outliers, and fluctuation scaling properties. For 85 of the 93 datasets, unbalanced classification accuracies were provided for different shape-based classifiers such as dynamic time warping (DTW) nearest neighbor, as well as for hybrid approaches such as COTE.
□ Bayesian Multiple Emitter Fitting using Reversible Jump Markov Chain Monte Carlo:
>> https://www.biorxiv.org/content/biorxiv/early/2019/01/26/530261.full.pdf
a Bayesian inference approach to multiple- emitter fitting that uses Reversible Jump Markov Chain Monte Carlo to identify and localize the emitters in dense regions of data. The output is both a posterior probability distribution of emitter locations that includes uncertainty in the number of emitters and the background structure, and a set of coordinates and uncertainties from the most probable model.

□ scVI/scANVI: Harmonization and Annotation of Single-cell Transcriptomics data with Deep Generative Models:
>> https://www.biorxiv.org/content/biorxiv/early/2019/01/29/532895.full.pdf
scANVI, a semi-supervised variant of scVI designed to leverage any available cell state annotations — for instance when only one data set in a cohort is annotated, or when only a few cells in a single data set can be labeled using marker genes. scVI and scANVI methods provide a complete probabilistic representation of the data, which non-linearly controls not only for sample-to-sample bias but also for other technical factors of variation such as over-dispersion, library size discrepancies and zero-inflation.
□ Re-curation and Rational Enrichment of Knowledge Graphs in Biological Expression Language:
>> https://www.biorxiv.org/content/biorxiv/early/2019/01/31/536409.full.pdf
A workflow for re-curating and rationally enriching knowledge graphs encoded in Biological Expression Language using pre-extracted content from INDRA. Furthermore, INDRA is flexible enough to generate curation sheets for curators familiar with formats other than BEL, such as BioPAX or SBML.
□ Computational analysis of molecular networks using spectral graph theory, complexity measures and information theory:
>> https://www.biorxiv.org/content/biorxiv/early/2019/02/02/536318.full.pdf
Spectral graph theory, reciprocal link and complexity measures were utilized to quantify network motifs. It was found that graph energy, reciprocal link and cyclomatic complexity can optimally specify network motifs with some degree of degeneracy. Biological networks are built up from a finite number of motif patterns; hence, a graph energy cutoff exists and the Shannon entropy of the motif frequency distribution is not maximal. Network similarity was quantified by gauging their motif frequency distribution functions using Jensen-Shannon entropy. This method allows us to determine the distance between two networks regardless of their node identities and network sizes.
□ SuperCRUNCH: A toolkit for creating and manipulating supermatrices and other large phylogenetic datasets:
>> https://www.biorxiv.org/content/biorxiv/early/2019/02/02/538728.full.pdf
SuperCRUNCH can be used to generate interspecific supermatrix datasets (one sequence per taxon per locus) or population-level datasets (multiple sequences per taxon per locus). It can also be used to assemble phylogenomic datasets with thousands of loci.
□ Simulating the DNA String Graph in Succinct Space:
>> https://arxiv.org/pdf/1901.10453.pdf
rBOSS is a de Bruijn graph in practice, but it simulates any length up to k and can compute overlaps of size at least m between the labels of the nodes, with k and m being parameters. As most BWT-based structures, rBOSS is unidirectional, but it exploits the property of the DNA reverse complements to simulate bi-directionality with some time-space trade-offs.
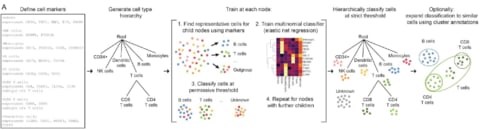
□ Garnett: Supervised classification enables rapid annotation of cell atlases
>> https://www.biorxiv.org/content/biorxiv/early/2019/02/04/538652.full.pdf
Garnett, an algorithm and accompanying software for rapidly annotating cell types in scRNA-seq and scATAC-seq datasets, based on an interpretable, hierarchical markup language of cell type-specific genes. Garnett will expand classifications to similar cells to generate a separate set of cluster-extended type assignments. Garnett successfully classifies cell types in tissue and whole organism datasets, as well as across species.
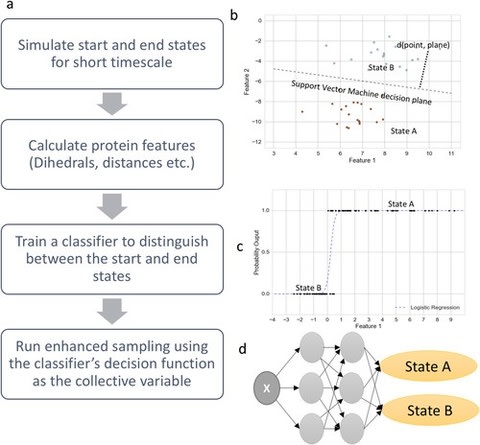
□ Automated design of collective variables using supervised machine learning:
>> https://aip.scitation.org/doi/full/10.1063/1.5029972
SMLCV shows how the distance to the support vector machines’ decision hyperplane, the output probability estimates from logistic regression, the outputs from deep neural network classifiers, and other classifiers may be used to reversibly sample slow structural transitions.
□ Attractor reconstruction by machine learning:
>> https://aip.scitation.org/doi/full/10.1063/1.5039508
a theoretical framework that describes conditions under which reservoir computing can create an empirical model capable of skillful short-term forecasts and accurate long-term ergodic behavior. a theory of how prediction with discrete-time reservoir computing or related machine-learning methods can “learn” a chaotic dynamical system well enough to reconstruct the long-term dynamics of its attractor.
□ Isospectral deformations, the spectrum of Jacobi matrices, infinite continued fraction and difference operators. Application to dynamics on infinite dimensional systems:
>> https://arxiv.org/pdf/1902.00225.pdf
The use of tau functions related to infinite dimensional Grassmannians, Fay identities, vertex operators and the Hirota’s bilinear formalism led to obtaining important results concerning these algebras of infinite order differential operators. In addition many problems related to algebraic geometry, combinatorics, probabilities and quantum gauge theory,..., have been solved explicitly by methods inspired by techniques from the study of dynamical integrable systems.
□ COMPLETENESS OF INFINITARY HETEROGENEOUS LOGIC:
>> https://arxiv.org/pdf/1902.00064.pdf
Heterogeneous quantifiers (infinite alternations of universal and existential quantification) present a new kind of quantification in infinitary logic related to game semantics. a proof system for classical infinitary logic that includes heterogeneous quantification (i.e., infinite alternate sequences of quantifiers) within the language Lκ+,κ. In κ-Grothendieck toposes in particular, and, when κ<κ = κ, also in Kripke models.

□ Grid-LMM: Fast and flexible linear mixed models for genome-wide genetics:
>> https://journals.plos.org/plosgenetics/article?id=10.1371/journal.pgen.1007978
Grid-LMM, an extendable algorithm for repeatedly fitting complex linear models that account for multiple sources of heterogeneity, such as additive and non-additive genetic variance, spatial heterogeneity, and genotype-environment interactions. Grid-LMM includes functions for both frequentist and Bayesian GWAS, (Restricted) Maximum Likelihood evaluation, Bayesian Posterior inference of variance components, and Lasso/Elastic Net fitting of high-dimensional models with random effects.
Supposedly “uniformative” versions of both the inverse-Gamma and half-Cauchy-type priors are actually highly informative for variance component proportions. A uniform prior over the grid was assumed, and the intercept was assigned a Gaussian prior with infinite variance.

□ hilldiv: an R package for the integral analysis of diversity based on Hill numbers:
>> https://www.biorxiv.org/content/biorxiv/early/2019/02/10/545665.full.pdf
Hill numbers provide a powerful framework for measuring, estimating, comparing and partitioning the diversity of biological systems as characterised using high throughput DNA sequencing approaches. The statistical framework developed around Hill numbers encompasses many of the most broadly employed diversity (e.g. richness, Shannon index, Simpson index), phylogenetic diversity (quadratic entropy) and dissimilarity (e.g. Sørensen index, Unifrac distances) metrics.

□ cSG-MCMC: Cyclical Stochastic Gradient MCMC for Bayesian Deep Learning:
>> https://arxiv.org/pdf/1902.03932.pdf
Several attempts have been made to improve the sampling efficiency of SG-MCMC. Stochastic Gradient Hamiltonian Monte Carlo (SGHMC) introduces the momentum variable to the Langevin dynamics.
the posteriors over neural network weights are high dimensional and multimodal. Each mode typically characterizes a meaningfully different representation of the data. Cyclical Stochastic Gradient MCMC (SG-MCMC) automatically explore such distributions. cyclical SG-MCMC methods provide more accurate uncertainty estimation, by capturing more diversity in the hypothesis space corresponding to settings of model parameters.
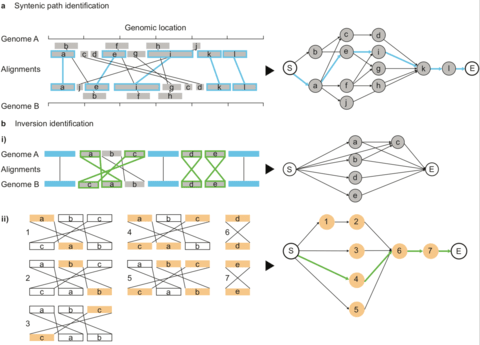
□ SyRI: identification of syntenic and rearranged regions from whole-genome assemblies:
>> https://www.biorxiv.org/content/biorxiv/early/2019/02/11/546622.full.pdf
Any pair of nodes is then connected by an edge if the two underlying alignments are colinear. Alignments are defined as colinear if the underlying regions are non-rearranged to each other and if no other co-linear alignment is between them. SyRI identifies the maximal syntenic path (i.e. the optimal set of non-conflicting, co-linear regions) by selecting the highest scoring path between node S (Start) and E (End) using dynamic programming.

□ BLight: Indexing De Bruijn graphs with minimizers:
>> https://www.biorxiv.org/content/biorxiv/early/2019/02/11/546309.full.pdf
BLight is a scalable and exact index structure able to associate unique identifiers to indexed k-mers and to reject alien k-mers. The proposed structure combines an extremely compact representation along with a high throughput. BLight, an ubiquitous, efficient and exact associative structure for indexing k-mers, relying on De Bruijn graphs. Based on efficient hashing techniques and light memory structure.
□ FLAM-seq: Full-length mRNA sequencing reveals principles of poly(A) tail length control:
>> https://www.biorxiv.org/content/biorxiv/early/2019/02/11/547034.full.pdf
a new method for high-throughput sequencing of polyadenylated RNAs in their entirety, including the transcription start site, the splicing pattern, the 3’ end and the poly(A) tail for each sequenced molecule. By providing full-length mRNA sequence including the poly(A) tail, FLAM-seq allows to reconstruct dependencies between different levels of gene regulation - in particular promoter choice, alternative splicing, 3’ UTR choice, and polyA tail length.
□ MIA: Andrew Blumberg, Using random matrix theory to model single-cell RNA; topological data analysis
a method for low-rank approximation of a data matrix arising from single-cell RNA sequencing data. The basic observation is that such data is consistent with a sparse version of the "spike model" studied in random matrix theory.

□ Network inference performance complexity: a consequence of topological, experimental, and algorithmic determinants:
>> https://academic.oup.com/bioinformatics/advance-article/doi/10.1093/bioinformatics/btz105/5319942
conducted a multifactorial analysis that demonstrates how stimulus target, regulatory kinetics, induction and resolution dynamics, and noise differentially impact widely used algorithms in significant and previously unrecognized ways. The results show how even if high-quality data are paired with high-performing algorithms, inferred models are sometimes susceptible to giving misleading conclusions.
□ Scalable multiple whole-genome alignment and locally collinear block construction with SibeliaZ:
>> https://www.biorxiv.org/content/biorxiv/early/2019/02/13/548123.full.pdf
SibeliaZ-LCB for identifying collinear blocks in closely related genomes based on the analysis of the de Bruijn graph. SibeliaZ shows drastic run-time improvements over other methods on both simulated and real data, with only a limited decrease in accuracy. SibeliaZ works by first constructing the compacted de Bruijn graph using our previoulsy published TwoPaCo tool, then finding locally collinear blocks using using SibeliaZ-LCB, and finally, running a multiple-sequence aligner on each of the found blocks.
□ SCENT: Estimating Differentiation Potency of Single Cells Using Single-Cell Entropy:
>> https://link.springer.com/protocol/10.1007%2F978-1-4939-9057-3_9
The estimation of differentiation potency is based on an explicit biophysical model that integrates the RNA-Seq profile of a single cell with an interaction network to approximate potency as the entropy of a diffusion process on the network.

□ Kevlar: a mapping-free framework for accurate discovery of de novo variants:
>> https://www.biorxiv.org/content/biorxiv/early/2019/02/13/549154.full.pdf
Kevlar identifies high-abundance k-mers unique to the individual of interest and retrieves the reads containing these k-mers. These reads are easily partitioned into disjoint sets by shared k-mer content for subsequent locus-by-locus processing and variant calling. Kevlar employs a novel probabilistic model to score variant predictions and distinguish miscalled inherited variants and true de novo mutations.
kevla predicts de novo genetic variants without mapping reads to a reference genome. kevlar's k-mer abundance based method calls single nucleotide variants, multinucleotide variants, insertion/deletion variants, and structural variants simultaneously with a single simple model.

□ Compositional Data Network Analysis via Lasso Penalized D-Trace Loss:
>> https://academic.oup.com/bioinformatics/advance-article-abstract/doi/10.1093/bioinformatics/btz098/5319971
A sparse matrix estimator for the direct interaction network is defined as the minimizer of lasso penalized CD-trace loss under positive-definite constraint. Simulation results show that CD-trace compares favorably to gCoda and that it is better than sparse inverse covariance estimation for ecological association inference (SPIEC-EASI) (hereinafter S-E) in network recovery with compositional data.

□ Virtual ChIP-seq: Predicting transcription factor binding by learning from the transcriptome:
>> https://www.biorxiv.org/content/biorxiv/early/2019/02/13/168419.full.pdf
Virtual ChIP-seq, which predicts binding of individual transcription factors in new cell types using an artificial neural network that integrates ChIP-seq results from other cell types and chromatin accessibility data in the new cell type. Virtual ChIP-seq also uses learned associations between gene expression and transcription factor binding at specific genomic regions.
※コメント投稿者のブログIDはブログ作成者のみに通知されます