
□ DRAGON: Determining Regulatory Associations using Graphical models on multi-Omic Networks
>> https://academic.oup.com/nar/advance-article/doi/10.1093/nar/gkac1157/6931867
DRAGON calibrates its parameters to achieve an optimal trade-off between the network’s complexity and estimation accuracy, while explicitly accounting for the characteristics of each of the assessed omics ‘layers.’
DRAGON is a partial correlation framework. Extending DRAGON to Mixed Graphical Models, which incorporate both continuous and discrete variables. DRAGON adapts to edge density and feature size differences between omics layers, improving model inference and edge recovery.

□ Sparse RNNs can support high-capacity classification
>> https://journals.plos.org/ploscompbiol/article?id=10.1371/journal.pcbi.1010759
A sparsely connected recurrent neural network (RNN) can perform classification in a distributed manner without ever bringing all of the relevant information to a single convergence site.
To investigate capacity and accuracy, networks were trained by back-propagation through time (BPTT). Hebbian-based sparse RNN readout accumulates evidence while the stimulus is on and amplifies the response when a +1-labeled input is shown.

□ Detecting bifurcations in dynamical systems with CROCKER plots
>> https://aip.scitation.org/doi/abs/10.1063/5.0102421
A CROCKER plot, was developed in the context of dynamic metric spaces. The additional restrictions means that the time-varying point clouds under study have labels on vertices from one parameter value to the next, allowing for more available theoretical results on continuity.
The CROCKER plot can be used for understanding bifurcations in dynamical systems. This construction is closely related to the 1-Wasserstein distance used for persistence diagrams and make connections b/n this and the maximum Lyapunov exponent, a commonly used measure for chaos.

□ novoRNABreak: local assembly for novel splice junction and fusion transcript detection from RNA-seq data
>> https://www.biorxiv.org/content/10.1101/2022.12.16.520791v1
novoRNABreak is based on a local assembly model, which offers a tradeoff between the alignment-based and de novo whole transcriptome assembly (WTA) approaches, namely, being more sensitive in assembling novel junctions that cannot be directly aligned.
novoRNABreak modifies the well-attested genomic structural variation breakpoint assembly novoBreak, assembles novel junctions. The assembled contigs are considerably longer than raw reads, are aligned against the Human genomic reference from Ensembl using Burrows-Wheeler Aligner.

□ Syntenet: an R/Bioconductor package for the inference and analysis of synteny networks
>> https://academic.oup.com/bioinformatics/advance-article/doi/10.1093/bioinformatics/btac806/6947985
syntenet infers synteny networks from whole-genome protein sequence data. syntenet offers a simple and complete framework, incl. data preprocessing, synteny detection and network inference, network clustering and phylogenomic profiling, and microsynteny-based phylogeny inference.
Network clustering is performed with the Infomap algorithm by default, which has been demonstrated as the best clustering for synteny networks, but users can also specify other algorithms implemented in the igraph, such as Leiden, label propagation, Louvain, and edge betweenness.

□ HAPNEST: efficient, large-scale generation and evaluation of synthetic datasets for genotypes and phenotypes
>> https://www.biorxiv.org/content/10.1101/2022.12.22.521552v1
HAPNEST simulates genotypes by resampling a set of existing reference genomes, according to a stochastic model that approximates the underlying processes of coalescent, recombination and mutation.
HAPNEST enables simulation of diverse biobank-scale datasets, as well as simultaneously generating multiple genetically correlated traits w/ population specific effects under different pleiotropy models. HAPNEST uses a model inspired by the sequential Markovian coalescent model.

□ SnapFISH: a computational pipeline to identify chromatin loops from multiplexed DNA FISH data
>> https://www.biorxiv.org/content/10.1101/2022.12.16.520793v1
SnapFISH collects the 3D localization coordinates of each genomic segment targeted by FISH and computes the pairwise Euclidean distances b/n all imaged targeted loci. SnapFISH compares the pairwise Euclidean distances b/n the pair of interest and its local neighborhood region.
SnapFISH converts the resulting P-values into FDRs, and defines a pair of targeted segments. Lastly, SnapFISH groups nearby loop candidates into clusters, identifies the pair with the lowest FDR within each cluster, and uses these summits as the final list of chromatin loops.

□ SURGE: Uncovering context-specific genetic-regulation of gene expression from single-cell RNA-sequencing using latent-factor models
>> https://www.biorxiv.org/content/10.1101/2022.12.22.521678v1
SURGE (Single-cell Unsupervised Regulation of Gene Expression), a novel probabilistic model that uses matrix factorization to learn a continuous representation of the cellular contexts that modulate genetic effects.
SURGE achieves this goal by leveraging information across genome-wide variant-gene pairs to jointly learn both a continuous representation of the latent cellular contexts defining each measurement and the interaction eQTL effect sizes corresponding to each SURGE latent context.
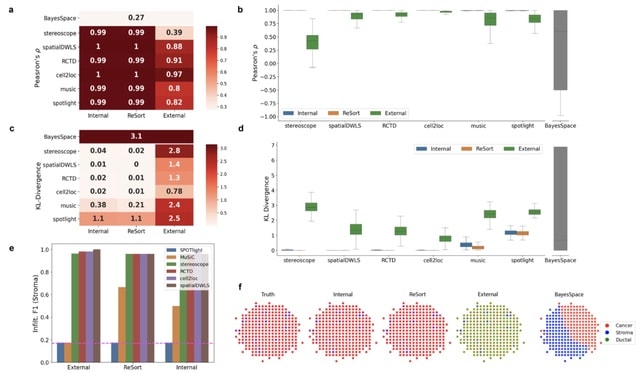
□ ReSort: Accurate cell type deconvolution in spatial transcriptomics using a batch effect-free strategy
>> https://www.biorxiv.org/content/10.1101/2022.12.15.520612v1
A Region-based cell type Sorting strategy (ReSort) that creates a pseudo-internal reference by extracting primary molecular regions from the ST data and leaves out spots that are likely to be mixtures.
By detecting these regions with diverse molecular profiles, ReSort can approximate the pseudo-internal reference to accurately estimate the composition at each spot, bypassing an external reference that could introduce technical noise.

□ Fast two-stage phasing of large-scale sequence data
>> https://www.cell.com/ajhg/fulltext/S0002-9297(21)00304-9
The method uses marker windowing and composite reference haplotypes. It incorporates a progressive phasing algorithm that identifies confidently phased heterozygotes in each iteration and fixes the phase of these heterozygotes in subsequent iterations.
The Method employs HMM w/ a parsimonious state space of composite reference haplotype. It uses a two-stage phasing algorithm that phases high-frequency markers via progressive phasing in the first stage and phases low-frequency markers via genotype imputation in the second stage.

□ Mabs, a suite of tools for gene-informed genome assembly
>> https://www.biorxiv.org/content/10.1101/2022.12.19.521016v1
Mabs tries to find values of parameters of a genome assembler that maximize the number of accurately assembled BUSCO genes. BUSCO is a program that is supplied with a number of taxon-specific datasets that contain orthogroups whose genes are present and single-copy.
Mabs-hifiasm is intended for assembly using PacBio HiFi reads, while Mabs-flye is intended for assembly using reads of more error-prone technologies, namely Oxford Nanopore Technologies and PacBio CLR. Mabs reduces the number of haplotypic duplications.

□ BioNumPy: Fast and easy analysis of biological data with Python
>> https://www.biorxiv.org/content/10.1101/2022.12.21.521373v1
BioNumPy is able to efficiently load biological datasets (e.g. FASTQ-files, BED-files and BAM-files) into NumPy-like data structures, so that NumPy operations like indexing, vectorized functions and reductions can be applied to the data.
A RaggedArray is similar to a NumPy array/matrix but can represent a matrix consisting of rows with varying lengths. An EncodedRaggedArray supports storing and operating on non-numeric data (e.g. DNA-sequences) by encoding the data and keeping track of the encoding.

□ BUSZ: Compressed BUS files
>> https://www.biorxiv.org/content/10.1101/2022.12.19.521034v1
BUSZ is a binary file consisting of a header, followed by zero / more compressed blocks of BUS records, ending with an empty block. The BUSZ header incl. all information from the BUS header, along w/ compression parameters. BUSZ files have a different magic number than BUS files.
The algorithm assumes a sorted input. The input is sorted lexicographically by barcodes first, then by UMIs, and finally by the equivalence classes. Within each block, the columns are compressed independently, each with a customized compression-decompression codec.

□ CETYGO: Uncertainty quantification of reference-based cellular deconvolution algorithms
>> https://www.tandfonline.com/doi/full/10.1080/15592294.2022.2137659
An accuracy metric that quantifies the CEll TYpe deconvolution GOodness (CETYGO) score of a set of cellular heterogeneity variables derived from a genome-wide DNAm profile for an individual sample.
CETYGO, as the root mean square error (RMSE) between the observed bulk DNAm profile and the expected profile across the M cell type specific DNAm sites used to perform the deconvolution, calculated from the estimated proportions for the N cell types.

□ CONGA: Copy number variation genotyping in ancient genomes and low-coverage sequencing data
>> https://journals.plos.org/ploscompbiol/article?id=10.1371/journal.pcbi.1010788
CONGA (Copy Number Variation Genotyping in Ancient Genomes and Low-coverage Sequencing Data), a CNV genotyping algorithm tailored for ancient and other low coverage genomes, which estimates copy number beyond presence/absence of events.
CONGA first calculates the number of reads mapped to each given interval in the reference genome, which we call “observed read-depth”. It then calculates the “expected diploid read-depth”, i.e., the GC-content normalized read-depth given the genome average.
CONGA calculates the likelihood for each genotype by modeling the read-depth distribution as Poisson. CONGA uses a split-read step in order to utilize paired-end information. It splits reads and remaps the split within the genome, treating the two segments as paired-end reads.

□ motifNet: Functional motif interactions discovered in mRNA sequences with implicit neural representation learning
>> https://www.biorxiv.org/content/10.1101/2022.12.20.521305v1
Many existing neural network models for mRNA event prediction only take the sequence as input, and do not consider the positional information of the sequence
motifNet is a lightweight neural network that uses both the sequence and its positional information as input. This allows for the implicit neural representation of the various motif interaction patterns in human mRNA sequences.

□ SCIBER: a simple method for removing batch effects from single-cell RNA-sequencing data
>> https://academic.oup.com/bioinformatics/advance-article/doi/10.1093/bioinformatics/btac819/6957084
SCIBER (Single-Cell Integrator and Batch Effect Remover) matches cell clusters across batches according to the overlap of their differentially expressed genes. SCIBER is a simple method that outputs the batch- effect corrected expression data in the original space/dimension.
SCIBER is computationally more efficient than Harmony, LIGER, and Seurat, and it scales to datasets with a large number of cells. SCIBER can be further accelerated by replacing K-means with a more efficient clustering algorithm or using a more efficient implementation of K-means.

□ CODA: a combo-Seq data analysis workflow
>> https://academic.oup.com/bib/advance-article/doi/10.1093/bib/bbac582/6955042
CODA (Combo-seq Data Analysis), a a custom-tailored workf low for the processing of Combo-Seq data which uses existing tools com- monly used in RNA-Seq data analysis and compared it to exceRpt.
Because of the chosen trimmer, the maximum read length of trimmed reads when using CODA is higher than the one with exceRpt, and it results in more reads successfully passing. This is more dramatic the shorter the sequenced reads are.
This tends to affect gene-mapping reads, rather than miRNA mapping ones: The absolute number of reads mapping to genes increases, especially for shorter sequencing reads, where the proportion of reads with an incomplete/missing adapter increases.
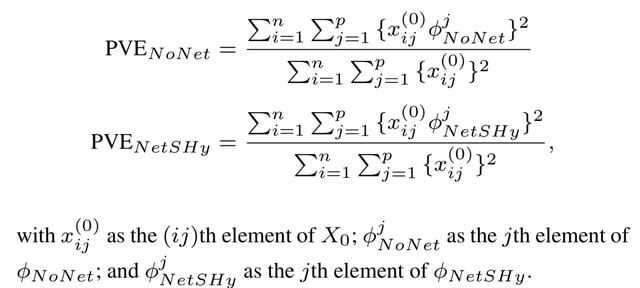
□ NetSHy: Network Summarization via a Hybrid Approach Leveraging Topological Properties
>> https://academic.oup.com/bioinformatics/advance-article/doi/10.1093/bioinformatics/btac818/6957083
NetSHy applies principal component analysis (PCA) on a combination of the node profiles and the well-known Laplacian matrix derived directly from the network similarity matrix to extract a summarization at a subject level.

□ Redeconve: Spatial transcriptomics deconvolution at single-cell resolution
>> https://www.biorxiv.org/content/10.1101/2022.12.22.521551v1
Redeconve, a new algorithm to estimate the cellular composition of ST spots. Redeconve introduces a regularizing term to solve the collinearity problem of high-resolution deconvolution, with the assumption that similar single cells have similar abundance in ST spots.
Redeconve is a quadratic programming model for single-cell deconvolution. A regularization term in the deconvolution model os based on non-negative least regression. Redeconve further improves the accuracy of estimated cell abundance based on a ground truth by nucleus counting.

□ CRAM compression: practical across-technologies considerations for large-scale sequencing projects
>> https://www.biorxiv.org/content/10.1101/2022.12.21.521516v1
Using CRAM for the Emirati Genome Program, which aims to sequence the genomes of ~1 million nationals in the United Arab Emirates using short- and long-read sequencing technologies (Illumina, MGI and Oxford Nanopore Sequencing).

□ SIMBSIG: Similarity search and clustering for biobank-scale data
>> https://academic.oup.com/bioinformatics/advance-article/doi/10.1093/bioinformatics/btac829/6958553
“SIMBSIG = SIMmilarity Batched Search Integrated GPU”, which can efficiently perform nearest neighbour searches, principal component analysis (PCA), and K-Means clustering on central processing units (CPUs) and GPUs, both in-core and out-of-core.

□ Igv.js: an embeddable JavaScript implementation of the Integrative Genomics Viewer (IGV)
>> https://academic.oup.com/bioinformatics/advance-article/doi/10.1093/bioinformatics/btac830/6958554
igv.js is an embeddable JavaScript implementation of the Integrative Genomics Viewer (IGV). It can be easily dropped into any web page with a single line of code and has no external dependencies.
igv.js supports a wide range of genomic track types and file formats, including aligned reads, variants, coverage, signal peaks, annotations, eQTLs, GWAS, and copy number variation. A particular strength of IGV is manual review of genome variants, both single-nucleotide and structural variants.

□ A Pairwise Strategy for Imputing Predictive Features When Combining Multiple Datasets
>> https://academic.oup.com/bioinformatics/advance-article/doi/10.1093/bioinformatics/btac839/6964381
This method maximizes common genes for imputation based on the intersection between two studies at a time. This method has significantly better performance than the omitting and merged methods in terms of the Root Mean Square Error of prediction on an external validation set.

□ Sc2Mol: A Scaffold-based Two-step Molecule Generator with Variational Autoencoder and Transformer
>> https://academic.oup.com/bioinformatics/advance-article/doi/10.1093/bioinformatics/btac814/6964383
Sc2Mol, a generative model-based molecule generator without any prior scaffold patterns. Sc2Mol uses SMILES strings for molecules. It consists of two steps: scaffold generation and scaffold decoration, which are carried out by a variational autoencoder and a transformer.

□ scAVENGERS: a genotype-based deconvolution of individuals in multiplexed single-cell ATAC-seq data without reference genotypes
>> https://academic.oup.com/nargab/article/4/4/lqac095/6965979
scAVENGERS (scATAC-seq Variant-based EstimatioN for GEnotype ReSolving) introduces an appropriate read alignment tool, variant caller, and mixture model to appropriately process the demultiplexing of scATAC-seq data.
scAVENGERS uses Scipy's sparse matrix structure to enable large data processing. scAVENGERS conveys the process of selecting alternative allele counts to maximize the expected value of total log-likelihood, a probability value of zero inevitably appears during the calculation.

□ gget: Efficient querying of genomic reference databases
>> https://academic.oup.com/bioinformatics/advance-article/doi/10.1093/bioinformatics/btac836/6971843
gget, a free and open-source software package that queries information stored in several large, public databases directly from a command line or Python environment.
gget consists of a collection of separate but interoperable modules, each designed to facilitate one type of database querying required for genomic data analysis in a single line of code.

□ Metadata retrieval from sequence databases with ffq
>> https://academic.oup.com/bioinformatics/advance-article-abstract/doi/10.1093/bioinformatics/btac667/6971839
ffq efficiently fetches metadata and links to raw data in JSON format. ffq’s modularity and simplicity makes it extensible to any genomic database exposing its data for programmatic access.

□ MinNet: Single-cell multi-omics integration for unpaired data by a siamese network with graph-based contrastive loss
>> https://bmcbioinformatics.biomedcentral.com/articles/10.1186/s12859-022-05126-7
MinNet is a novel Siamese neural network design for single-cell multi-omics sequencing data integration. It ranked top among other methods in benchmarking and is especially suitable for integrating datasets with batch and biological variances.
MinNet reduces the distance b/n similar cells and separate different cells in the n-dimensional space. The distances b/n corresponding cells get smaller while the distances between negative pairs get larger. In this way, main biological variance is kept in the co-embedding space.

□ NetAct: a computational platform to construct core transcription factor regulatory networks using gene activity
>> https://genomebiology.biomedcentral.com/articles/10.1186/s13059-022-02835-3
NetAct infers regulators’ activity using target expression, constructs networks based on transcriptional activity, and integrates mathematical modeling for validation. NetAct infers TF activity for an individual sample directly from the expression of genes targeted by the TF.
NetAct calculates its activity using the mRNA expression of the direct targets of the TF. NetAct is robust against some inaccuracy in the TF-target database and noises in GE data, because of its capability of filtering out irrelevant targets as well as remaining key targets.

□ RabbitVar: ultra-fast and accurate somatic small-variant calling on multi-core architectures
>> https://www.biorxiv.org/content/10.1101/2023.01.06.522980v1
RabbitVar features a heuristic-based calling method and a subsequent machine-learning-based filtering strategy. RabbitVar has also been highly optimized by featuring multi-threading, a high-performance memory allocator, vectorization, and efficient data structures.

□ The probability of edge existence due to node degree: a baseline for network-based predictions
>> https://www.biorxiv.org/content/10.1101/2023.01.05.522939v1
The framework decomposes performance into the proportions attributable to degree. The edge prior can be estimated using the fraction of permuted networks in which a given edge exists—the maximum likelihood estimate for the binomial distribution success probability.
The modified XSwap algorithm by adding two parameters, allow_loops, and allow_antiparallel that allow a greater variety of network types to be permuted. The edge swap mechanism uses a bitset to avoid producing edges which violate the conditions for a valid swap.

□ HiDDEN: A machine learning label refinement method for detection of disease-relevant populations in case-control single-cell transcriptomics
>> https://www.biorxiv.org/content/10.1101/2023.01.06.523013v1
HiDDEN refines the casecontrol labels to accurately reflect the perturbation status of each cell. HiDDEN’s superior ability to recover biological signals missed by the standard analysis workflow in simulated ground truth datasets of cell type mixtures.

□ Hetnet connectivity search provides rapid insights into how two biomedical entities are related
>> https://www.biorxiv.org/content/10.1101/2023.01.05.522941v1
Transforming the DWPC across all source-target node pairs for a metapath to yield a distribution that is more compact and amenable to modeling. And calculate a path score heuristic, which can be used to compare the importance of paths between metapaths.

□ scEMAIL: Universal and Source-free Annotation Method for scRNA-seq Data with Novel Cell-type Perception
>> https://www.sciencedirect.com/science/article/pii/S1672022922001747
scEMAIL, a universal transfer learning-based annotation framework for scRNA-seq data, which incorporates expert ensemble novel cell-type perception and local affinity constraints of multi-order, with no need for source data.
scEMAIL can deal with atlas-level datasets with mixed batches. scEMAIL achieved intra-cluster compactness and inter-cluster separation, which indicated that the affinity constraints guide the network to learn the correct intercellular relationships.

□ RCL: Unsupervised Contrastive Peak Caller for ATAC-seq
>> https://www.biorxiv.org/content/10.1101/2023.01.07.523108v1
RCL uses ResNET as the backbone module with only five layers, making the network architecture shallow but efficient. RCL showed no problems with class imbalance, probably because the region selection step effectively discards nonpeak regions and balances the data.
RCL could be extended to take coverage vectors for multiple fragment lengths, the fragments themselves, or even annotation information, as used by the supervised method CNN-Peaks.
※コメント投稿者のブログIDはブログ作成者のみに通知されます