
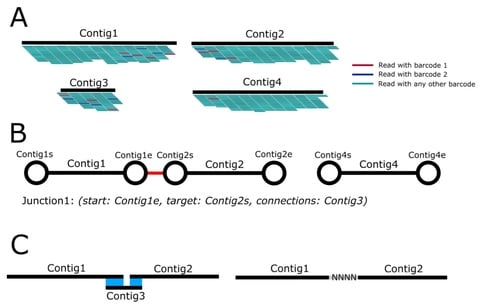
□ AnVIL: An overlap-aware genome assembly scaffolder for linked reads
>> https://www.biorxiv.org/content/10.1101/2020.04.29.065847v1.full.pdf
While ARCS/ARKS are able to estimate a gap distance between putative linked contigs, LINKS does not resolve cases where there is an estimated negative distance, i.e. an overlap between contigs, and simply merges them with a single lower-case “n” in between.
AnVIL (Assembly Validation and Improvement using Linked reads) generates scaffolds from genome drafts using 10X Chromium data, with a focus on minimizing the number of gaps in resulting scaffolds by incorporating an OLC step to resolve junctions between linked contigs.
AnVIL was developed for application on genomes that have been assembled using long-read sequencing and an OLC method. Ideally, the genome size is over 1Gb and the number of input contigs is over 10000. it can additionally scaffold Supernova assemblies of pure 10X Chromium data.
□ LVREML: Restricted maximum-likelihood method for learning latent variance components in gene expression data with known and unknown confounders
>> https://www.biorxiv.org/content/10.1101/2020.05.06.080648v1.full.pdf
LVREML, a restricted maximum-likelihood method which estimates the latent variables by maximizing the likelihood on the restricted subspace orthogonal to the known confounding factors, this reduces to probabilistic PCA on that subspace.
While the LVREML solution is not guaranteed to be the absolute maximizer of the total likelihood function, it is guaranteed analytically that for any given number p of latent variables, the LVREML solution attains minimal unexplained variance among all possible choices of p latent variables.
□ grünifai: Interactive multi-parameter optimization of molecules in a continuous vector space
>> https://academic.oup.com/bioinformatics/advance-article-abstract/doi/10.1093/bioinformatics/btaa271/5830268
grünifai, an interactive in-silico compound optimization platform to support the ideation of the next generation of compounds under the constraints of a multi-parameter objective.
grünifai integrates adjustable in-silico models, a continuous vector space, a scalable particle swarm optimization algorithm and the possibility to actively steer the compound optimization through providing feedback on generated intermediate structures.
□ DeepArk: modeling cis-regulatory codes of model species with deep learning
>> https://www.biorxiv.org/content/10.1101/2020.04.23.058040v1.full.pdf
DeepArk accurately predicts the presence of thousands of different context-specific regulatory features, including chromatin states, histone marks, and transcription factors.
DeepArk can predict the regulatory impact of any genomic variant (including rare or not previously observed), and enables the regulatory annotation of understudied model species.
□ ARCS: Optimizing Regularized Cholesky Score for Order-Based Learning of Bayesian Networks
>> https://ieeexplore.ieee.org/document/9079582
Combined, the two approaches allow us to quickly and effectively search over the space of DAGs without the need to verify the acyclicity constraint or to enumerate possible parent sets given a candidate topological sort.
Annealing Regularized Cholesky Score (ARCS) algorithm to search over topological sorts for a high-scoring Bayesian network. ARCS combines global simulated annealing over permutations with a fast proximal gradient algorithm, operating on triangular matrices of edge coefficients.

□ AD-AE: Adversarial Deconfounding Autoencoder for Learning Robust Gene Expression Embeddings
>> https://www.biorxiv.org/content/10.1101/2020.04.28.065052v1.full.pdf
AD-AE (Adversarial Deconfounding AutoEncoder) can learn embeddings generalizable to different domains, and deconfounds gene expression latent spaces.
The AD-AE model consists of two neural networks: (i) an autoencoder to generate an embedding that can reconstruct original measurements, and (ii) an adversary trained to predict the confounder from that embedding.

□ totalVI: Joint probabilistic modeling of paired transcriptome and proteome measurements in single cells
>> https://www.biorxiv.org/content/10.1101/2020.05.08.083337v1.full.pdf
totalVI; Total Variational Inference, provides a cohesive solution for common analysis tasks like the integration of datasets with matched or unmatched protein panels, dimensionality reduction, clustering, evaluation of correlations between molecules, and differential expression.
totalVI is a deep generative model for relate the coordinates of its latent dimensions. totalVI learns a joint probabilistic representation of RNA and protein measurements that aims to account for the distinct noise and technical biases of each modality as well as batch effects.

□ PheLEx: Identifying novel associations in GWAS by hierarchical Bayesian latent variable detection of differentially misclassified phenotypes
>> https://bmcbioinformatics.biomedcentral.com/articles/10.1186/s12859-020-3387-z
a novel strategy for applying the PheLEx framework to explore new loci within a GWAS dataset by making use of misclassification probabilities for phenotype and strategic filtering of SNPs to improve accuracy and avoid model overfitting.
PheLEx consists of a hierarchical Bayesian latent variable model, and agnostic to the cause of misclassification and rather assumes that the underlying genetics can be leveraged to provide an accurate assessment of misclassification, regardless of cause.

□ Needlestack: an ultra-sensitive variant caller for multi-sample next generation sequencing data
>> https://academic.oup.com/nargab/article/2/2/lqaa021/5822688
Needlestack is based on the idea that the sequencing error rate can be dynamically estimated from analysing multiple samples together and is particularly appropriate to call variants that are rare in the sequenced material.
Needlestack provides a multi-sample VCF containing all candidate variants that obtain a QVAL higher than the input threshold in at least one sample, general information about the variant in the INFO field (maximum observed QVAL) and individual information in the GENOTYPE field.
□ CLUSBIC: A Model Selection Approach to Hierarchical Shape Clustering with an Application to Cell Shapes
>> https://www.biorxiv.org/content/10.1101/2020.04.29.067892v1.full.pdf
The existing hierarchical shape clustering methods are distance based. Such methods often lack a proper statistical foundation to allow for making inference on important parameters such as the number of clusters, often of prime interest to practitioners.
CLUSBIC takes a model selection perspective to clustering and propose a shape clustering method through linear models defined on Spherical Harmonics expansions of shapes.
□ SAGACITE: Riemannian geometry and statistical modeling correct for batch effects and control false discoveries in single-cell surface protein count data from CITE-seq
>> https://www.biorxiv.org/content/10.1101/2020.04.28.067306v1.full.pdf
Main computational challenges lie in computing the COM of a point cloud on the hypersphere and “parallel transporting” the point cloud along a specific path connecting the old and new COM, according to some notion of ge- ometry defined on the manifold.
SAGACITE (Statistical and Geometric Analysis of CITE-seq), the notion of high- dimensional Riemannian manifold endowed with the Fisher-Rao metric, and apply the idea to map the immunophenotype profiles of single cells to a hypersphere.
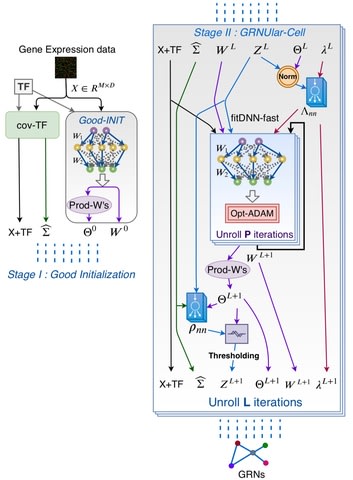
□ GRNUlar: Gene Regulatory Network reconstruction using Unrolled algorithm from Single Cell RNA-Sequencing data
>> https://www.biorxiv.org/content/10.1101/2020.04.23.058149v1.full.pdf
GRNUlar incorporates TF information using a sparse multi-task deep learning architecture.
GRNUlar, Unrolled model for recovering directed GRNs applies the Alternative Minimization (AM) algorithm and unroll it for certain number of iterations.
GRNUlar replaces the hyperparameters with problem dependent neural networks and treat all the unrolled iterations together as a deep model. And learn this unrolled architecture under supervision by defining a direct optimization objective.

□ On the robustness of graph-based clustering to random network alterations
>> https://www.biorxiv.org/content/10.1101/2020.04.24.059758v1.full.pdf
the robustness of a range of graph-based clustering algorithms in the presence of network-level noise, including algorithms common across domains. the results of all clustering algorithms measured were profoundly sensitive to injected network noise.
Using simulated noise to predict the effects of future network alterations relies on noise being representative of those real-world alterations. the running time scales linearly w/ N, clust.perturb will be time-intensive if the original clustering algorithm is time-intensive.
□ High dimensional model representation of log-likelihood ratio: binary classification with expression data
>> https://bmcbioinformatics.biomedcentral.com/articles/10.1186/s12859-020-3486-x
The proposed HDMR-based approach appears to produce a reliable classifier that additionally allows one to describe how individual genes or gene-gene interactions affect classification decisions.
HDMR expansion optimally decomposes a high dimensional non-linear system into a hierarchy of lower dimensional non-linear systems. a regression based approach to circumvent solving complex integral equations.

□ Succinct dynamic variation graphs
>> https://www.biorxiv.org/content/10.1101/2020.04.23.056317v1.full.pdf
libbdsg and libhandlegraph, which use a simple, field-proven interface, designed to expose elementary features of these graphs while preventing common graph manipulation mistakes.
The efficiency of these methods and their encapsulation within a coherent programming interface will support their reuse within a diverse set of application domains. Variation graphs have deep similarity with graphs used in assembly.

□ Self-normalizing learning on biomedical ontologies using a deep Siamese neural network
>> https://www.biorxiv.org/content/10.1101/2020.04.23.057117v1.full.pdf
a novel method that applies named entity recognition and normalization methods on texts to connect the structured information in biomedical ontologies with the information contained in natural language.
The normalized ontologies and text are then used to generate embeddings, and relations between entities are predicted using a deep Siamese neural network model that takes these embeddings as input.
□ Detecting rare copy number variants (CNVs) from Illumina genotyping arrays with the CamCNV pipeline: segmentation of z-scores improves detection and reliability.
>> https://www.biorxiv.org/content/10.1101/2020.04.23.057158v1.full.pdf
CamCNV uses the information from all samples to convert intensities to z-scores, thus adjusting for variance between probes.
CamCNV calculates the mean and standard deviation of the LRR for each probe across all samples and convert the LRR into z-scores.

□ SingleCellNet: A Computational Tool to Classify Single Cell RNA-Seq Data Across Platforms and Across Species
>> https://www.cell.com/cell-systems/fulltext/S2405-4712(19)30199-1
SCN-TP is resilient to regressing on stage of cell cycle except when only the query data were adjusted, whereas classifiers trained directly on expression levels are prone to performance degradation.
Another output of SCN is the attribution plot, in which SCN assigns a single identity to each cell based on the category with the maximum classification score.
□ BioGraph: Leveraging a WGS compression and indexing format with dynamic graph references to call structural variants
>> https://www.biorxiv.org/content/10.1101/2020.04.24.060202v1.full.pdf
BioGraph, a novel structural variant calling pipeline that leverages a read compression and indexing format to discover alleles, assess their supporting coverage, and assign useful quality scores.
BioGraph calls were sensitive to a greater number of SV calls given the same false discovery rate compared to the other pipelines. After merging discovered calls and running BioGraph Coverage to create a squared-off project-level VCF.
BioGraph QUALclassifier uses coverage signatures to assign quality scores to discovered alleles to increase specificity and assist prioritization of variants.

□ CFSP: a collaborative frequent sequence pattern discovery algorithm for nucleic acid sequence classification
>> https://peerj.com/articles/8965/
a Teiresias-like feature extraction algorithm to discover frequent sub-sequences (CFSP) can find frequent sequence pairs with a larger gap.
The combinations of frequent sub-sequences in given protracted sequences capture the long-distance correlation, which implies a specific molecular biological property.

□ Boundary-Forest Clustering: Large-Scale Consensus Clustering of Biological Sequences
>> https://www.biorxiv.org/content/10.1101/2020.04.28.065870v1.full.pdf
Boundary-Forest Clustering (BFClust) can generate cluster confidence scores, as well as allow cluster augmentation.
Since each of the trees generated in BFClust has a small depth, the number of comparisons one needs to make for a new sequence set is relatively small (tree depth × 10 trees).

□ Scedar: A scalable Python package for single-cell RNA-seq exploratory data analysis
>> https://journals.plos.org/ploscompbiol/article?id=10.1371/journal.pcbi.1007794
Scedar provides analytical routines for visualization, gene dropout imputation, rare transcriptomic profile detection, clustering, and identification of cluster separating genes.
a novel cell clustering algorithm: Minimum Description Length (MDL) Iteratively Regularized Agglomerative Clustering (MIRAC) extends hierarchical agglomerative clustering (HAC) in a divide and conquer manner for scRNA-seq data.
MIRAC starts with one round of bottom-up HAC to build a tree with optimal linear leaf ordering, and the tree is then divided into small sub-clusters, which are further merged iteratively into clusters.
The asymptotic time complexity of the MIRAC algorithm is O(n4+mn2) where n is the number of samples, and m is the number of features. The space complexity is O(n2+mn).
□ Gromov-Wasserstein optimal transport to align single-cell multi-omics data
>> https://www.biorxiv.org/content/10.1101/2020.04.28.066787v1.full.pdf
SCOT calculates a probabilistic coupling matrix that matches cells across two datasets. The optimization uses k-nearest neighbor graphs, thus preserving the local geometry of the data.
SCOT uses Gromov Wasserstein-based optimal transport to perform unsupervised integration of single-cell multi-omics data, performs well when compared to two state-of-the-art methods but in less time and with fewer hyperparameters.
SCOT computes the shortest path distance on the graph between each pair of nodes. And set the distance of any unconnected nodes to be the maximum (finite) distance in the graph and rescale the resulting distance matrix by dividing by the maximum distance for numerical stability.
□ ArchR: An integrative and scalable software package for single-cell chromatin accessibility analysis
>> https://www.biorxiv.org/content/10.1101/2020.04.28.066498v1.full.pdf
ArchR provides an intuitive interface for complex single-cell analyses incl. single-cell clustering, robust peak set generation, cellular trajectory identification, DNA element to gene linkage, expression level prediction from chromatin accessibility, and multi-omic integration.
ArchR provides a facile platform to interrogate scATAC-seq data from multiple scATAC-seq implementations, including the 10x Genomics Chromium system , the Bio-Rad droplet scATAC-seq system, single-cell combinatorial indexing , and the Fluidigm C1 system.
ArchR takes as input aligned BAM or fragment files, which are first parsed in small chunks per chromosome, read in parallel to conserve memory, then efficiently stored on disk using the compressed random-access hierarchical data format version 5 (HDF5) file format.

□ scGAN: Deep feature extraction of single-cell transcriptomes by generative adversarial network
>> https://www.biorxiv.org/content/10.1101/2020.04.29.066464v1.full.pdf
Single-cell Generative Adversarial Network. The Encoder projects each single-cell GE profile onto a low dimensional embedding. The Decoder takes the embedding as input and predicts the sufficient statistics of the Negative Binomial data likelihood of the scRNA-seq counts.
The Discriminator, being trained adversarially alongside the Encoder network, predicts the batch effects using as input the Encoder's embedding. Encoder, Decoder and the Discriminator are all parametric neural networks with learnable parameters.
□ MR-GAN: Predicting sites of epitranscriptome modifications using unsupervised representation learning based on generative adversarial networks
>> https://www.biorxiv.org/content/10.1101/2020.04.28.067231v1.full.pdf
MR-GAN, a generative adversarial network (GAN) based model, which is trained in an unsupervised fashion on the entire pre-mRNA sequences to learn a low dimensional embedding of transcriptomic sequences.
Using MR-GAN, it also investigated the sequence motifs for each modification type and uncovered known motifs as well as new motifs not possible with sequences directly.
□ ALiBaSeq: New alignment-based sequence extraction software and its utility for deep level phylogenetics
>> https://www.biorxiv.org/content/10.1101/2020.04.27.064790v1.full.pdf
ALiBaSeq, a program using freely available similarity search tools to find homologs in assembled WGS data with unparalleled freedom to modify parameters.
ALiBaSeq is capable of retrieving orthologs from well-curated, low and high depth shotgun, and target capture assemblies as well or better than other software in finding the most genes with maximal coverage and has a low comparable rate of false positives throughout all datasets.
□ VCFdbR: A method for expressing biobank-scale Variant Call Format data in a SQLite database using R
>> https://www.biorxiv.org/content/10.1101/2020.04.28.066894v1.full.pdf
VCFdbR, a pipeline for converting VCFs to simple SQLite databases, which allow for rapid searching and filtering of genetic variants while minimizing memory overhead.
After database creation, VCFdbR creates a GenomicRanges representation of each variant. The GenomicRanges data structure is the bedrock of 94 many Bioconductor genomics packages.
□ Reference-based QUantification Of gene Dispensability (QUOD)
>> https://www.biorxiv.org/content/10.1101/2020.04.28.065714v1.full.pdf
QUOD calculates a reference-based gene dispensability score for each annotated gene based on a supplied mapping file (BAM) and annotation of the reference sequence (GFF).
Instead of classifying a gene as core or dispensable, QUOD assigns a dispensability score to each gene. Hence, QUOD facilitates the identification of candidate dispensable genes which often underlie lineage-specific adaptation to varying environmental conditions.

□ wg-blimp: an end-to-end analysis pipeline for whole genome bisulfite sequencing data
>> https://bmcbioinformatics.biomedcentral.com/articles/10.1186/s12859-020-3470-5
wg-blimp provides a comprehensive analysis pipeline for whole genome bisulfite sequencing data as well as a user interface for simplified result inspection.
wg-blimp integrates established algorithms for alignment, quality control, methylation calling, detection of differentially methylated regions, and methylome segmentation, requiring only a reference genome and raw sequencing data as input.
□ NBAMSeq: Negative binomial additive model for RNA-Seq data analysis
>> https://bmcbioinformatics.biomedcentral.com/articles/10.1186/s12859-020-3506-x
NBAMSeq, a flexible statistical model based on the generalized additive model and allows for information sharing across genes in variance estimation.
NBAMSeq models the logarithm of mean gene counts as sums of smooth functions with the smoothing parameters and coefficients estimated simultaneously within a nested iterative method.

□ PheKnowlator: A Framework for Automated Construction of Heterogeneous Large-Scale Biomedical Knowledge Graphs
>> https://www.biorxiv.org/content/10.1101/2020.04.30.071407v1.full.pdf
PheKnowlator, the first fully customizable KG construction framework enabling users to build complex KGs that are Semantic Web compliant and amenable to automatic OWL reasoning, conform to contemporary property graph standards.
PheKnowlator provides this functionality by offering multiple build types, can automatically include inverse edges, creates OWL-decoded KGs to support automated deductive reasoning, and outputs KGs in several formats e.g. triple edge lists, OWL API-formatted RDF/XML and graph-pickled MultiDiGraph.
By providing flexibility in the way relations are modeled and facilitates the creation of property graphs, PheKnowLator enables the use of cutting edge graph-based learning and sophisticated network inference algorithms.
□ Logicome Profiler: Exhaustive detection of statistically significant logic relationships from comparative omics data
>> https://www.ncbi.nlm.nih.gov/pubmed/32357172
Logicome Profiler adjusts a significance level by the Bonferroni or Benjamini-Yekutieli method for the multiple testing correction.
Logicome Profiler, which exhaustively detects statistically significant triplet logic relationships from a binary matrix dataset. Logicome means ome of logics.

□ Investigating the effect of dependence between conditions with Bayesian Linear Mixed Models for motif activity analysis
>> https://journals.plos.org/plosone/article?id=10.1371/journal.pone.0231824
the Bayesian Linear Mixed Model implementation outperforms Ridge Regression in a simulation scenario where the noise, which is the signal that can not be explained by TF motifs, is uncorrelated.
With these data there is no advantage to using the Bayesian Linear Mixed Model, due to the similarity of the covariance structure.

□ Geometric hashing: Global, Highly Specific and Fast Filtering of Alignment Seeds
>> https://www.biorxiv.org/content/10.1101/2020.05.01.072520v1.full.pdf
Geometric hashing, a new method for filtering alignment seeds achieves a high specificity by combining non-local information from different seeds using a simple hash function that only requires a constant and small amount of additional time per spaced seed.
□ DeepNano-blitz: A Fast Base Caller for MinION Nanopore Sequencers
>> https://academic.oup.com/bioinformatics/advance-article-abstract/doi/10.1093/bioinformatics/btaa297/5831289
DeepNano-blitz can analyze stream from up to two MinION runs in real time using a common laptop CPU (i7-7700HQ), with no GPU requirements.
The base caller settings allow trading accuracy for speed and the results can be used for real time run monitoring (sample composition, barcode balance, species identification) or pre-filtering of results for detailed analysis i.e. filtering out human DNA from pathogen runs.

□ DECoNT: Polishing Copy Number Variant Calls on Exome Sequencing Data via Deep Learning
>> https://www.biorxiv.org/content/10.1101/2020.05.09.086082v1.full.pdf
DECoNT (Deep Exome Copy Number Tuner) is a deep learning based software that corrects CNV predictions on exome sequencing data using read depth sequences.
DECoNT uses a single hidden layered Bi-LSTM architecture with 128 hidden neurons in each direction to process the read depth signal. DECoNT makes use of the calls made on the WGS data of the same sample as the ground truth for the learning procedure.
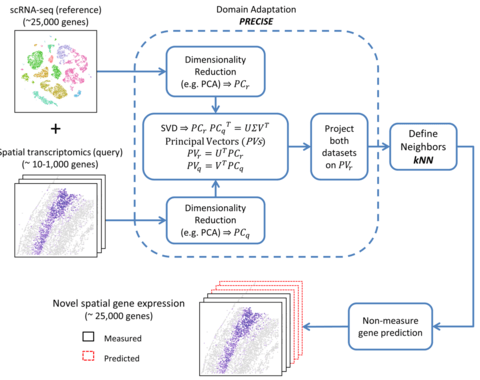
□ SpaGE: Spatial Gene Enhancement using scRNA-seq https://www.biorxiv.org/content/10.1101/2020.05.08.084392v1.full.pdf
SpaGE relies on domain adaptation using PRECISE to correct for differences in sensitivity of transcript detection between both single-cell technologies, followed by a k-nearest-neighbor (kNN) prediction of new spatial gene expression.
※コメント投稿者のブログIDはブログ作成者のみに通知されます