

□ scLTNN: Identify the origin and end cells and infer the trajectory of cellular fate automatically
>> https://www.biorxiv.org/content/10.1101/2022.09.28.510020v1
scLTNN (single cell latent time neuron network) identifies origin and end cell states from scRNA-seq data by combining a priori latent time predictions using scVelo, and genes whose expression patterns correlate with gene counts.
scLTNN uses the raw matrix to calculate the origin and end cells by ANN-time prediction and automatically selects the origin cells as the root of the PAGA graph. The scLTNN then constructed a RANN regression model to predict the intermediate moments using the LSI vectors.

□ Minigraph-Cactus: Pangenome Graph Construction from Genome Alignment
>> https://www.biorxiv.org/content/10.1101/2022.10.06.511217v1
Minigraph-Cactus combines Minigraph’s fast assembly-to-graph mapping with Cactus’s base aligner in order to produce base-level pangenome graphs at the scale of hundreds of vertebrate haplotypes.
Minigraph-Cactus combines the chromosome level results. Nodes are replaced with their reverse complement to ensure that reference paths only ever visit them. The original SV graph remains at this stage, with each minigraph node being represented by a separate embedded path.
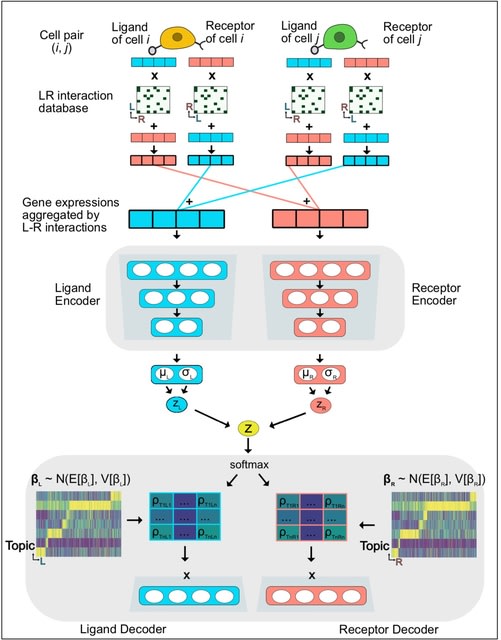
□ SPRUCE: Single-cell Pairwise Relationships Untangled by Composite Embedding model
>> https://www.biorxiv.org/content/10.1101/2022.09.16.508327v1
SPRUCE, Single-cell Pairwise Relationship Untangled by Composite Embedding, to analyze tens of millions of cell pairs in a scalable way. Adopting known ligand and receptor protein-protein interactions.
SPRUCE is based on an Embedded Topic Model, and represents single-cell vector data in low-dimension topic space with an interpretable topic-specific GE dictionary matrix. The SPRUCE model considers cell-cell interaction patterns as a stream of edges, or a giant incidence matrix.

□ scSemiGAN: a single-cell semi-supervised annotation and dimensionality reduction framework based on generative adversarial network
>> https://academic.oup.com/bioinformatics/advance-article-abstract/doi/10.1093/bioinformatics/btac652/6747954
scSemiGAN, a semi-supervised cell-type annotation and dimensionality reduction framework based on generative adversarial network, modeling scRNA-seq data from the aspect of data generation.
scSemiGAN is capable of performing deep latent representation learning and cell-type label prediction simultaneously. Guided by a few known cell-type labels, dimensionality reduction and cell-type annotation are jointly optimized.

□ xAI: Obtaining genetics insights from deep learning via explainable artificial intelligence
>> https://www.nature.com/articles/s41576-022-00532-2
The model parameters are sensitive to random selection of training examples and the initialization parameters. Model-based interpretations are most sensitive to this un-identifiability issue; however, This phenomenon affects all interpretation techniques to varying degrees.
xAI algorithms can examine the inner workings of black box such as DNNs to reveal the basis on which predictions are made. A transparent neural network model is one in which the hidden nodes are constructed to physically correspond to biological units at a level of granularity.

□ Deciphering multi-way interactions in the human genome
>> https://www.nature.com/articles/s41467-022-32980-z
Using incidence matrix-based representation and analysis of multi-way chromatin structure directly captured by Pore-C data (Algorithm 1), which is mathematically simple and computationally efficient, and yet can provide insights into genome architecture.
In this hypergraph framework, nodes are genomic loci and hyperedges are multi-way contacts among loci. Rows are genomic loci and columns are individual hyperedges. This representation enabled quantitative measurements of chromatin architecture through hypergraph entropy.

□ EagleImp: Fast and Accurate Genome-wide Phasing and Imputation in a Single Tool
>> https://academic.oup.com/bioinformatics/advance-article/doi/10.1093/bioinformatics/btac637/6706779
EagleImp combines the core methods from Eagle2 and PBWT, since both tools are used by the established SIS web service and both use the same-named Position- based Burrows-Wheeler Transform (PBWT) data structure.
Its main advantages are the compact representation of binary data and the ability to quickly look up any binary sequence at any position in the data.
To create a PBWT, the algorithm determines permutations of the input sequences for each genomic site such that the subsequences ending at that site are sorted when read backwards.
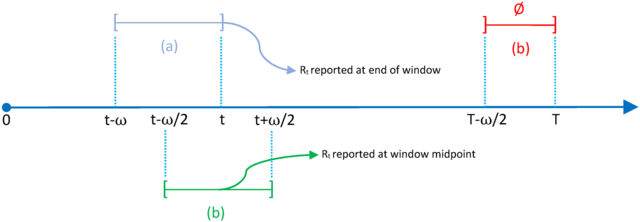
□ EpiLPS: A fast and flexible Bayesian tool for estimation of the time-varying reproduction number
>> https://journals.plos.org/ploscompbiol/article?id=10.1371/journal.pcbi.1010618
The proposed Bayesian methodology is based on a latent Gaussian model for the B-spline amplitudes and opens up two possible paths for inference. LPSMAP, a fully sampling-free approach based on Laplace approximations to the conditional posterior of B-spline coefficients.
The Laplacian-P-splines with a Metropolis-adjusted Langevin algorithm uses Langevin dynamics for efficient sampling of the target posterior distribution and is a MCMC approach based on the Langevin diffusion for exploration of the posterior distribution of latent variables.
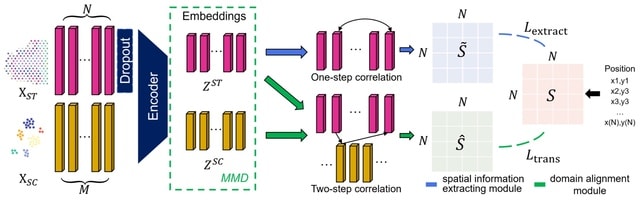
□ STEM: Learning Spatially-Aware Representations of Transcriptomic Data via Transfer Learning
>> https://www.biorxiv.org/content/10.1101/2022.09.23.509186v1
The STEM encoder represents SC and ST gene expression vectors as embeddings in a unified latent space. The embeddings are simultaneously optimized by two modules of predictor: the spatial information extracting module and the domain alignment module.
STEM identifies spatially dominant genes (SDGs) that highly dominate the inferred spatial location of a cell, which could benefit the understanding of underlying mechanisms related to cellular spatial organization or communication.
The domain alignment module uses SC and ST embeddings and eliminates the SC-ST domain gap by first minimizing the Maximum Mean Discrepancy (MMD) of SC and ST embeddings and then constructing ST-SC-ST spatial associations as ST adjacency to find the optimal mapping matrix.
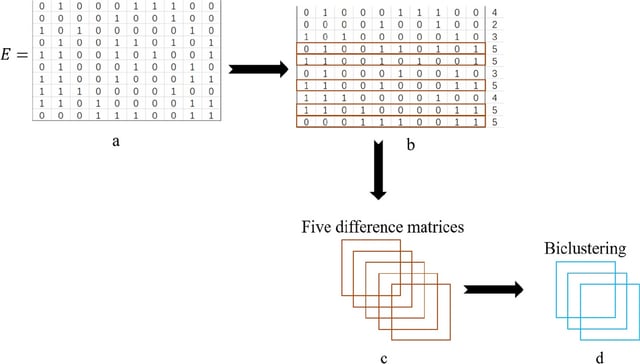
□ AMBB: A binary biclustering algorithm based on the adjacency difference matrix for gene expression data analysis
>> https://bmcbioinformatics.biomedcentral.com/articles/10.1186/s12859-022-04842-4
AMBB, the Adjacency Difference Matrix Binary Biclustering algorithm constructs the adjacency matrix based on the adjacency difference values, and the submatrix obtained by continuously updating the adjacency difference matrix is called a bicluster.
The adjacency matrix allows for clustering of gene that undergo similar reactions under different conditions into clusters, which is important for subsequent genes analysis. The AMBB algorithm outperforms BiBit, QUBIC and Bimax algorithms in the synthetic dataset.
The AMBB algorithm uses the row with the highest number of 1’s in the binary matrix as the seed, and iterates the row and column elements continuously. The AMBB algorithm does not require to encode and traverse all rows for continuous seed acquisition.
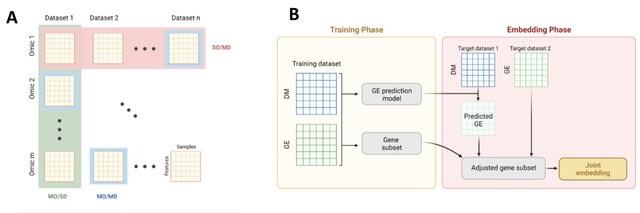
□ INTEND: Integration of Gene Expression and DNA Methylation Data Across Different Experiments
>> https://www.biorxiv.org/content/10.1101/2022.09.21.508920v1
INTEND (IntegratioN of Transcriptomic and EpigeNomic Data) learns a function that predicts its expression based on the methylation levels in sites located proximal to it. INTEND first predicts for each methylation profile its expression profile.
INTEND identifies a set of genes that will be used for the joint embedding of the expression and predicted expression datasets. At this stage, both datasets share the same feature space. INTEND then employs canonical-correlation analysis (CCA) to jointly reduce their dimension.
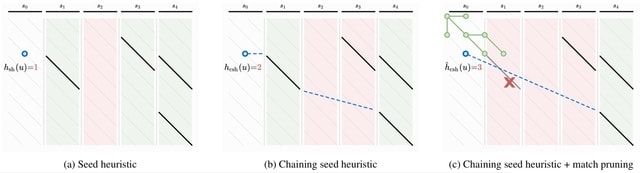
□ Astar Pairwise Aligner: Exact global alignment using A* with seed heuristic and match pruning
>> https://www.biorxiv.org/content/10.1101/2022.09.19.508631v1
Solving exact global pairwise alignment with respect to edit distance by using the A⋆ shortest path algorithm on the edit graph. And extending the seed heuristic for A⋆ with match chaining, inexact matches, and the novel match pruning optimization.
For random sequences with up to 15% uniform errors, the runtime of A*PA scales near-linearly to very long sequences (107 bp) and outperforms other exact aligners.
Since it is unlikely that edit distance in general can be solved in strongly subquadratic time, it is inevitable that there are inputs for which the algorithm requires quadratic time. Regions with high error rate, long indels, and too many matches trigger quadratic exploration.
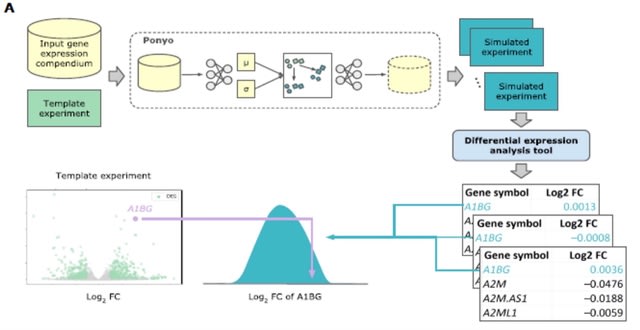
□ SOPHIE: Generative Neural Networks Separate Common and Specific Transcriptional Responses
>> https://www.sciencedirect.com/science/article/pii/S1672022922001279
Specific cOntext Pattern Highlighting In Expression data (SOPHIE), for distinguishing common / specific transcriptional patterns using a generative neural network to create a background set of experiments from which a null distribution of gene / pathway changes can be generated.
SOPHIE returned consistent genes and pathways, by percentile. SOPHIE’s specificity score can be a complementary indicator of activity compared to the traditional log fold change measure and can help drive future analyses.
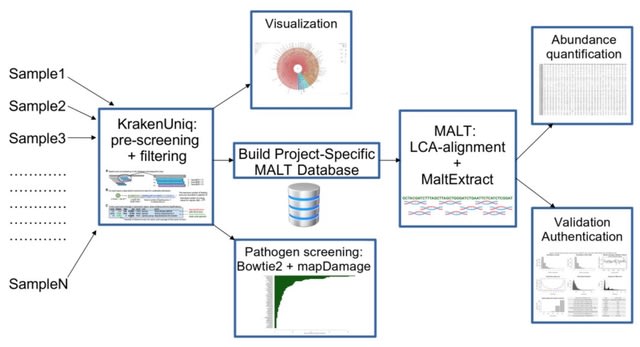
□ aMeta: an accurate and memory-efficient ancient Metagenomic profiling workflow
>> https://www.biorxiv.org/content/10.1101/2022.10.03.510579v1
aMeta combines the strengths of both classification- and alignment-based approaches with low detection and authentication errors. aMeta uses KrakenUniq for initial taxonomic profiling of metagenomic samples and informing MALT reference database construction.
aMeta performs an alignment with the Lowest Common Ancestor (LCA) algorithm implemented in MALT. aMeta minimizes potential conflicts between classification (KrakenUniq) and alignment (MALT) approaches by ensuring consistent use of the reference database.
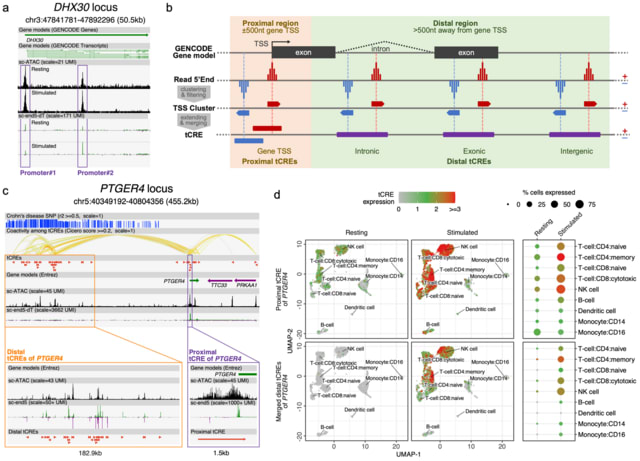
□ SCAFE: a software suite for analysis of transcribed cis-regulatory elements in single cells
>> https://academic.oup.com/bioinformatics/advance-article/doi/10.1093/bioinformatics/btac644/6730725
SCAFE (Single Cell Analysis of Five-prime Ends), a software suite that processes sc- end5-seq data to de novo identify TSS clusters based on multiple logistic regression. It annotates tCREs based on the identified TSS clusters and generates a tCRE-by-cell count matrix.
SCAFE defines tCREs by merging closely located TSS clusters and annotates these tCREs as proximal or distal based on their distance. It defines hyperactive distal loci by stitching closely located distal tCREs with disproportionately high activities, analogous to super-enhancers.
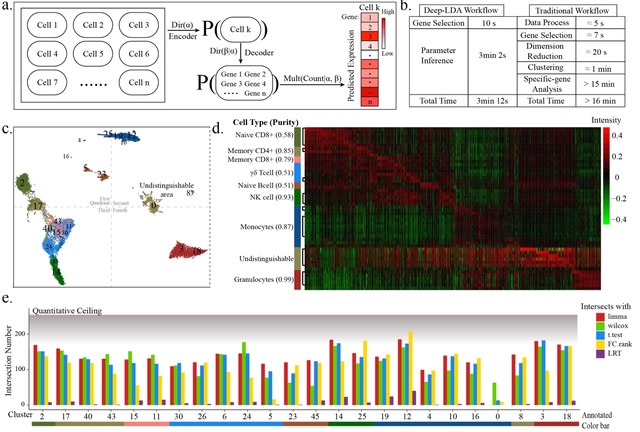
□ Optimization and redevelopment of single-cell data analysis workflow based on deep generative models
>> https://www.biorxiv.org/content/10.1101/2022.09.12.507562v1
Deep-LDA (a latent Dirichlet allocation-based deep generative model) model was applied on the 3-phase data, whose clustering results had a high consistency with the real distribution at all phases.
The distribution shape drawn from this model was more similar with the real distribution shape, and did not form a blocky distribution like other clustering procedures, which suggested Deep-LDA has a higher nonlinear fitting ability.
The outcome of the model was not optimized according to the uniform dimensionality reduction space which was the space for internal clustering metrics calculation, but was optimized according to the inferred feature space of different classes.
The generative architecture of Deep-LDA in this project was the classical LDA architecture of topic modeling and was not re-designed according to the characteristic of scRNA-seq data, such as incorporating the parameter for controlling the 0-inflation ratio.

□ Dictys: dynamic gene regulatory network dissects developmental continuum with single-cell multi-omics
>> https://www.biorxiv.org/content/10.1101/2022.09.14.508036v1
Dictys model single-cell transcriptional kinetics to allow for feedback loops, using the Ornstein-Uhlenbeck (OU) process with empirical contributions from basal transcription, direct GRN by TF binding, and stochasticity.
Dictys steady-state distribution then characterizes the biological variations in single-cell expression. Conversely, single-cell technical variation/noise is modeled with sparse binomial sampling. Dictys includes a suite of functions to understand and compare context specific networks.
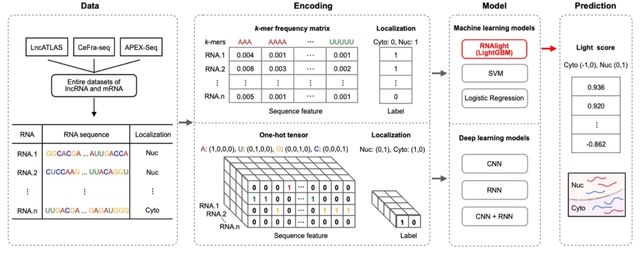
□ RNAlight: a machine learning model to identify nucleotide features determining RNA subcellular localization
>> https://www.biorxiv.org/content/10.1101/2022.09.16.508211v1
RNAlight identifies nucleotide k-mers contributing to the subcellular localizations of mRNAs and lncRNAs. With embedded Tree SHAP algorithm, RNAlight further reveals distinct key sequence features and their associated RBPs for subcellular localizations.
By assembling k-mers to sequence features and subsequently mapping to known RBP-associated motifs, different types of sequence features and their associated RBPs were additionally uncovered for lncRNAs and mRNAs with distinct subcellular localizations.

□ TandemAligner: a new parameter-free framework for fast sequence alignment
>> https://www.biorxiv.org/content/10.1101/2022.09.15.507041v1
Counterintuitively, the classical alignment approaches, such as the Smith-Waterman algorithm, that work well for most sequences, fail to construct biologically adequate alignments of the extra-long tandem repeats (ETRs).
TandemAligner — the parameter-free sequence alignment algorithm that introduces a sequence-dependent alignment scoring that automatically changes for any pair of compared sequences. TandemAligner illustrates its performance using human centromeres and primate immunoglobulin loci.

□ FrameRate: learning the coding potential of unassembled metagenomic reads
>> https://www.biorxiv.org/content/10.1101/2022.09.16.508314v1
The FrameRate model can predict the coding frame(s) from unassembled DNA sequencing reads directly, thus greatly reducing the computational resources required for genome assembly and similarity-based inference to pre-computed databases.
FrameRate captured equivalent functional profiles from the coding frames while reducing the required storage and time resources significantly. FrameRate was also able to annotate reads that were not represented in the assembly, capturing this ’missing’ information.
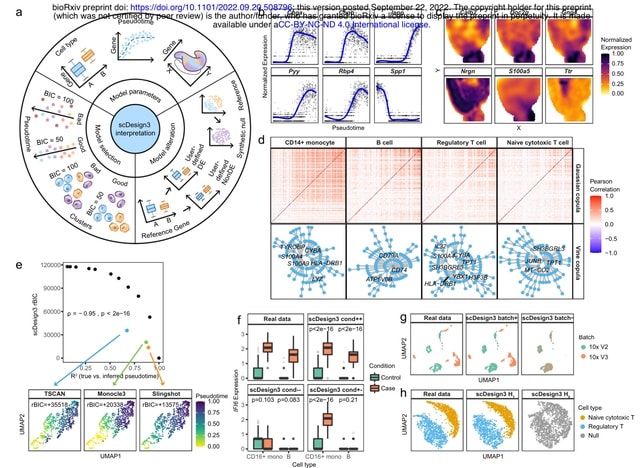
□ scDesgin3: A unified framework of realistic in silico data generation and statistical model inference for single-cell and spatial omics
>> https://www.biorxiv.org/content/10.1101/2022.09.20.508796v1
scDesign3 is beyond a versatile simulator and has unique advantages for generating customized in silico data, which can serve as negative and positive controls for computational analysis, and for assessing the quality of cell clusters and trajectories with statistical rigor.
scDesign3 resembles two single-cell chro- matin accessibility datasets profiled by the sci-ATAC-seq and 10x scATAC-seq protocols. scDesign3 mimics a CITE-seq dataset and simulates a multi-omics dataset from separately measured RNA expression and DNA methylation modalities.

□ Totem: a user-friendly tool for clustering-based inference of tree-shaped trajectories from single-cell data
>> https://www.biorxiv.org/content/10.1101/2022.09.19.508535v1
Totem generates a large number of clustering results, estimates their topologies as minimum spanning trees (MST), and uses them to measure the connectivity of the cells.
Totem uses a k-medoids algorithm. Totem is built upon the Slingshot method, which uses a clustering to construct an MST and the simultaneous principal curves algorithm to obtain a directed trajectory along w/ pseudotime that quantifies cell differentiation at the sc-level.

□ cell2sentence: Representing cells as sentences enables natural-language processing for single-cell transcriptomics
>> https://www.biorxiv.org/content/10.1101/2022.09.18.508438v1
cell2sentence, a novel method for the transformation of expression matrices to abundance-ordered lists, where genes are analogous to words, and cells are analogous to sentences. It can be directly rendered as space-delimited text, in a manner similar to natural language.
This adapted approach incorporates prior knowledge of gene homologs by using fused Gromov-Wasserstein optimal transport, which smoothly interpolates between pure Wasserstein / pure Gromov optimal transport, with cost weighting subject to a hyperparameter.
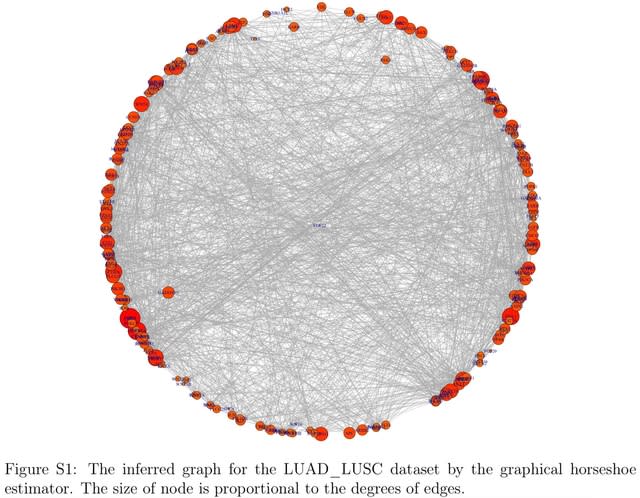
□ The GR2D2 estimator for the precision matrices
>> https://academic.oup.com/bib/advance-article-abstract/doi/10.1093/bib/bbac426/6731716
GR2D2 (Graphical R^2-induced Dirichlet Decomposition), a new Gaussian Graphical Model based on the R2D2 priors for linear models. Posterior samples under the GR2D2 hierarchical model are drawn by an augmented block Gibbs sampler algorithm.
The GR2D2 model puts R2D2 priors on the off-diagonal elements of the precision matrix. When the true precision matrix is sparse and of high dimension, the GR2D2 provides the estimates with smallest information divergence from the underlying truth.
In high-dimensional precision matrix estimation, the global shrinkage parameter adapts to the sparsity of the entire matrix and shrinks the estimates of the off-diagonal elements toward zero. The local shrinkage parameters preserve the magnitude of nonzero off-diagonal elements.
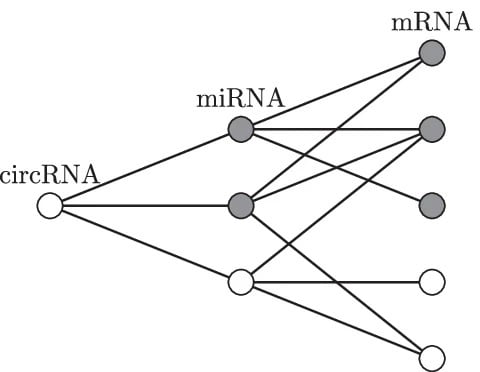
□ circGPA: circRNA functional annotation based on probability-generating functions
>> https://bmcbioinformatics.biomedcentral.com/articles/10.1186/s12859-022-04957-8
circGPA (circRNA generating-polynomial annotator), an efficient and exact procedure that is based on the principle of probability-generating functions. circGPA calculates all the p-values exactly.
A statistic that quantifies the size of the neighborhood of the circRNA that is annotated with a term of certain cardinality is introduced. The probability mass function of the statistic, which is a discrete random variable, is represented as a power series.
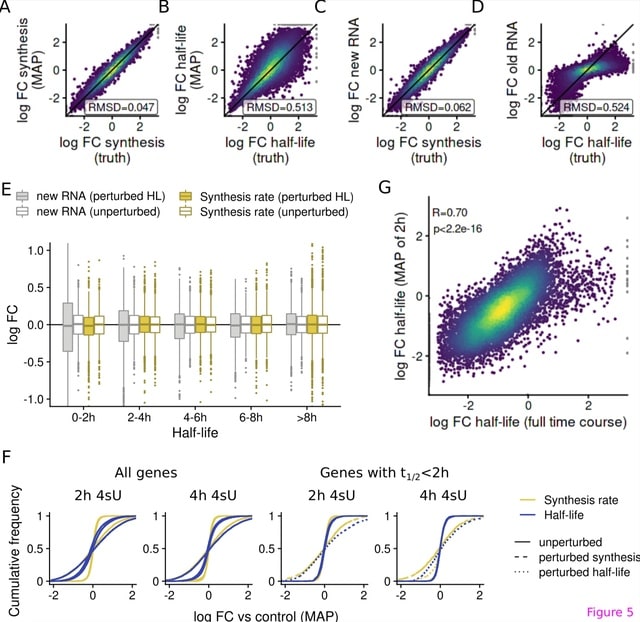
□ grandR: a comprehensive package for nucleotide conversion sequencing data analysis
>> https://www.biorxiv.org/content/10.1101/2022.09.12.507665v1
grandR facilitates analyses of nucleotide conversion sequencing experiments. It includes new methods for quality control and recalibrating labeling times.
grandR is designed as a comprehensive and easy-to-use toolkit for all types of nucleotide conversion sequencing data such as SLAM-seq, Timelapse-seq or TUC-seq.
The most accurate results are obtained by directly utilizing the posteriors from GRAND-SLAM to estimate the kinetic model. A Bayesian hierarchical model dissects the mode of gene regulation from snapshot experiments.

□ ortho_seqs: A Python tool for sequence analysis and higher order sequence-phenotype mapping
>> https://www.biorxiv.org/content/10.1101/2022.09.14.506443v1
ortho_seqs quantifies higher order sequence-phenotype interactions based on our previously published method of applying multivariate tensor-based orthogonal polynomials to biological sequences.
Using ortho_seqs, nucleotide or amino acid sequence information is converted to a 4-dimensional vector, which are then used to build and compute the first- and higher order tensor-based orthogonal polynomials.
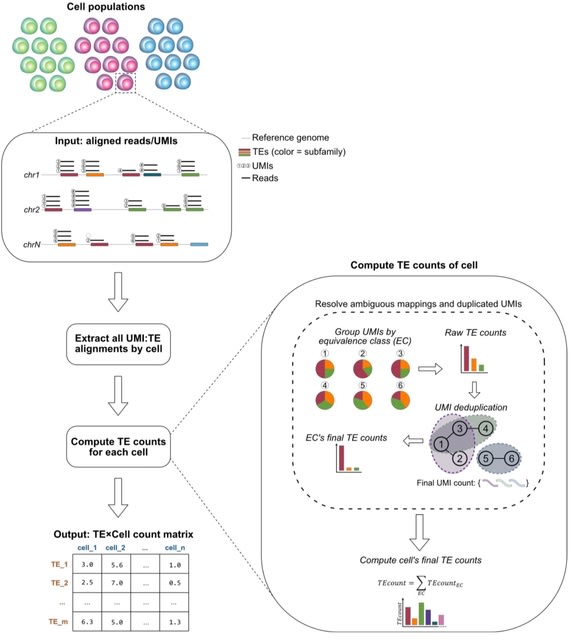
□ IRescue: single cell uncertainty-aware quantification of transposable elements expression
>> https://www.biorxiv.org/content/10.1101/2022.09.16.508229v1
IRescue (Interspersed Repeats single-cell quantifier), a software to quantify TE expression in scRNA-seq using a UMI-TE equivalence class-based algorithm to solve the allocation of reads ambiguously mapped on interspersed TEs.
IRescue is currently the only software that, in case of UMIs mapping multiple times on different TE subfamilies, takes into account all mapped features to estimate the correct one, rather than excluding multi-mapping UMIs or picking one randomly.
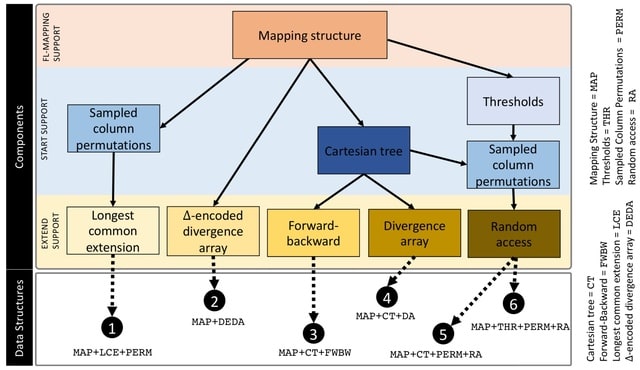
□ Compressed Data Structures for Population-Scale Positional Burrows–Wheeler Transforms
>> https://www.biorxiv.org/content/10.1101/2022.09.16.508250v1
The time complexity of finding maximal haplotype matches using the PBWT is a significant improvement over the naïve pattern-matching algorithm that requires O(h2w)-time.
A comprehensive study of the memory footprint of data structures supporting maximal haplotype matching in conjunction with the PBWT. The study contributes formal definition of finding set-maximal exact match (SMEMs) in the PBWT, and the queries needed to support finding SMEMs.
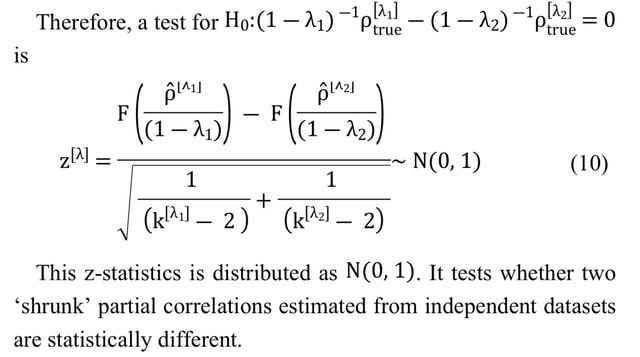
□ GeneNetTools: Tests for Gaussian graphical models with shrinkage
>> https://academic.oup.com/bioinformatics/advance-article/doi/10.1093/bioinformatics/btac657/6731926
While the covariance matrix can always be estimated from data, in this case the estimated matrix must be invertible and well-conditioned. This requirement ensures that the inverse of the covariance matrix exists and that its computation is stable.
Deriving the statistical properties of the partial correlation obtained with the Ledoit-Wolf shrinkage. The result provides a toolbox for (differential) network analyses as i) confidence intervals, ii) a test for zero partial correlation (null-effects), and iii) a test to compare partial correlations.
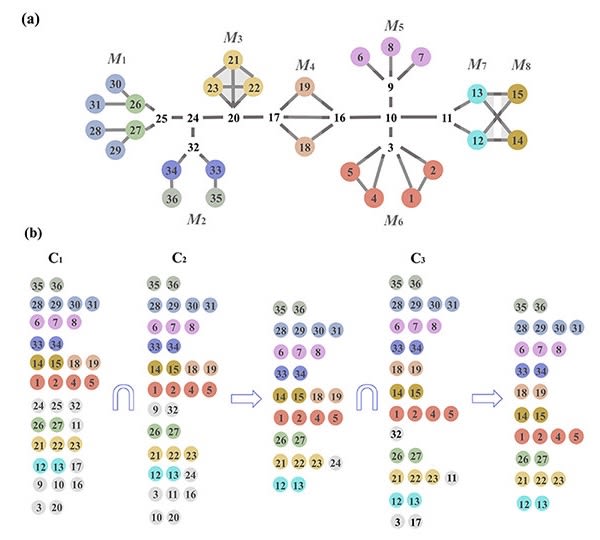
□ SPV: Structural position vectors and symmetries in complex networks
>> https://aip.scitation.org/doi/10.1063/5.0107583
Symmetric nodes can be used to develop coarse-grained simulations, identify the evolution law of the network, and determine the network’s synchronization dynamics.
SPV can identify symmetric nodes in linear time and dramatically speed up calculations. Nodes having equal SPV values is a strong necessary condition for them being symmetric to each other.
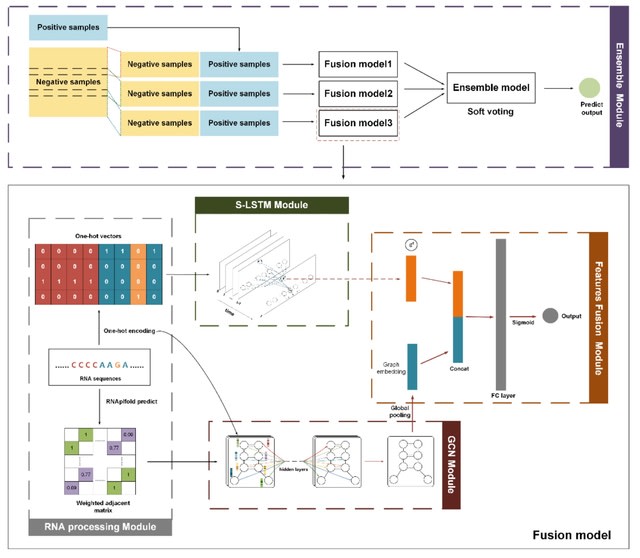
□ DeepCIP: a multimodal deep learning method for the prediction of internal ribosome entry sites of circRNAs
>> https://www.biorxiv.org/content/10.1101/2022.10.03.510726v1
DeepCIP is the first predictor for circRNA IRESs, which consists of an RNA processing module, an S-LSTM module, a GCN module, a feature fusion module, and an ensemble module. S-LSTM can represent circRNA IRES sequences more efficiently.
S-LSTM learns the representation of sequence by the Graph LSTM method. The performance of the sequence model is affected by many hyperparameters such as the number of sentence-level nodes, the window size, the time step, and the hidden layer size in the S-LSTM module.
□ GATK Dev Team
>> https://github.com/broadinstitute/gatk/releases/tag/4.3.0.0
GATK 4.3.0.0 adds stable support for the UltimaGenomics flow-based sequencing platform among other feature improvements.

□ Genetics of human telomere biology disorders
>> https://www.nature.com/articles/s41576-022-00527-z
#Review by Patrick Revy, Caroline Kannengiesser & @ABertuch
@Inserm @InstitutImagine @APHP @bcmhouston
※コメント投稿者のブログIDはブログ作成者のみに通知されます