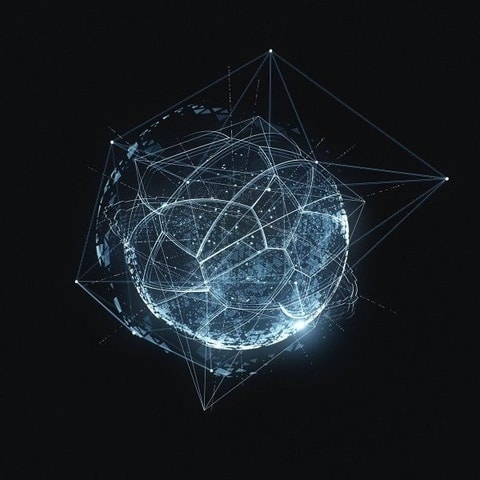
地球は丸く、時間は丸い。
- [x] 『議員』を無作為選挙にして、『国策』の最適性を解析-投票するシステムが実現できると仮定すれば、多くの問題は解決する。


□ Evolutionary implementation of Bayesian computations
>> https://www.biorxiv.org/content/biorxiv/early/2019/06/28/685842.full.pdf
many fundamental Darwinian phenomena can now be translated to the language of Bayesian computations, including selection, mutation and multilevel evolutionary processes.
a coherent mathematical discussion of these observations in terms of Bayesian graphical models and a step-by-step introduction to their evolutionary interpretation.
a deeper algorithmic analogy between evolutionary dynamics and statistical learning, pointing towards a unified computational understanding of mechanisms Nature invented to adapt to high-dimensional and uncertain environments.

□ Dimensionality reduction by UMAP to visualize physical and genetic interactions
>> https://www.biorxiv.org/content/biorxiv/early/2019/06/25/681726.full.pdf
Proximity in low-dimensional UMAP space identifies clusters of genes that correspond to protein complexes and pathways, and finds novel protein interactions even within well-characterized complexes.
Performing clustering in UMAP space ought to produce clusters containing more true interactions than distance in other spaces.

□ RADICL-seq identifies general and cell type-specific principles of genome-wide RNA-chromatin interactions
>> https://www.biorxiv.org/content/biorxiv/early/2019/06/27/681924.full.pdf
RNA And DNA Interacting Complexes Ligated and sequenced (RADICL-seq), a technology that maps genome-wide RNA-chromatin interactions in intact nuclei.
RADICL-seq is a proximity ligation-based methodology that reduces the bias for nascent transcription, while increasing genomic coverage and unique mapping rate efficiency compared to existing methods.
RADICL-seq identifies distinct patterns of genome occupancy for different classes of transcripts as well as cell type-specific RNA-chromatin interactions, and emphasizes the role of transcription in the establishment of chromatin structure.
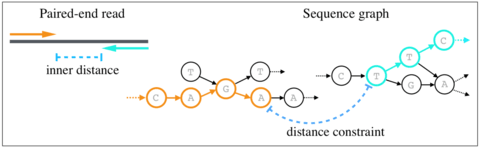
□ Validating paired-end read alignments in sequence graphs
>> https://www.biorxiv.org/content/biorxiv/early/2019/06/26/682799.full.pdf
the first mathematical formulation of the problem of validating paired-end distance constraints in sequence graphs, and propose an exact algorithm to solve it that is also practical.
The proposed algorithm exploits sparsity in sequence graphs to build an index, which can be queried quickly using a simple lookup during the read mapping process.
a trivial pseudo-polynomial time algorithm to solve the paired-end distance validation problem. The problem of validating distance constraints between two vertices can be solved using dynamic programming.

□ Odyssey: a semi-automated pipeline for phasing, imputation, and analysis of genome-wide genetic data
>> https://bmcbioinformatics.biomedcentral.com/articles/10.1186/s12859-019-2964-5
Odyssey is a pipeline that integrates programs such as PLINK, SHAPEIT, Eagle, IMPUTE, Minimac, and several R packages to create a seamless, which are handled automatically via the Singularity container solution.
Outliers that fall outside of the X-dimensional centroid are determined based on a specified standard deviation or inter quartile range cutoff. the exclusion method performed by Odyssey only occurs once as opposed to Eigensoft’s iterative exclusion method.

□ Tensor decomposition-Based Unsupervised Feature Extraction Applied to Single-Cell Gene Expression Analysis https://www.biorxiv.org/content/biorxiv/early/2019/06/27/684225.full.pdf
Because of the insufficient information available, unsupervised clustering, e.g., tSNE and UMAP, is usually employed to obtain low dimensional embedding that can help to understand cell-cell relationship.
Since PCA based unsupervised FE outperformed other three popular unsupervised gene selection methods, highly variable genes, bimodal genes and dpFeature, tensor decomposition based unsupervised FE can do so as well.
□ Dynamic genetic regulation of gene expression during cellular differentiation
>> https://www.dropbox.com/s/v60k5qjb0tm9lh2/1287.full.pdf
nonlinear dynamic eQTLs, which affect only intermediate stages of differentiation and cannot be found by using data from mature tissues.
characterized global patterns of GE across time by applying split-GPM, an unsupervised probabilistic model that infers time-course trajectories of gene expression using Gaussian processes, while simultaneously performing clustering of genes and cell lines.
Using this approach, identified two clusters of cell lines that displayed broad differences in the expression patterns of multiple clusters of genes; in each gene cluster, genes exhibit shared expression changes over time.
□ SORA: Scalable Overlap-graph Reduction Algorithms for Genome Assembly using Apache Spark in the Cloud
>> https://ieeexplore.ieee.org/abstract/document/8621546
SORA adapts string graph reduction algorithms for the genome assembly using a distributed computing platform.
SORA uses Apache Spark which is a cluster-based engine designed on top of Hadoop to handle very large datasets in the cloud.
SORA can process a nearly one billion edge graph in a distributed cloud cluster as well as smaller graphs on a local cluster with a short turnaround time.
□ Control of Intracellular Molecular Networks Using Algebraic Methods
>> https://www.biorxiv.org/content/biorxiv/early/2019/06/27/682989.full.pdf
As this method uses polynomial algebra over a finite field, all network nodes need to take values in a common finite field, in particular, all nodes need to have the same number of possible values.
a method to convert models with a general number of mixed discrete states into a model that satisfies the computational algebra requirements, without changing the model’s steady states, and which is not equivalent to the well-known reduction to a Boolean network that adds new nodes to the network, as done in.
□ ABMDA: Adaptive boosting-based computational model for predicting potential miRNA-disease associations
>> https://academic.oup.com/bioinformatics/advance-article-abstract/doi/10.1093/bioinformatics/btz297/5481952
balanced the positive and negative samples by performing random sampling based on k-means clustering on negative samples, whose process was quick and easy, and ABMDA had higher efficiency and scalability for large datasets than previous methods.
As a boosting technology, ABMDA was able to improve the accuracy of given learning algorithm by integrating weak classifiers that could score samples to form a strong classifier based on corresponding weights.
□ BIOINFORMATICS IN THE ERA OF GENOMICS IN AFRICA
>> http://ngbioinformaticsconference.com
The Nigerian Bioinformatics and Genomics Network (NBGN) is pleased to organise the First Nigerian Bioinformatics Conference (FNBC) with the theme "Bioinformatics in the Era of Genomics in Africa" in Lagos, Nigeria June 25 -26, 2019.

□ genesorteR: Feature Ranking in Clustered Single Cell Data
>> https://www.biorxiv.org/content/biorxiv/early/2019/06/25/676379.full.pdf
genesorteR calculates a specificity score to rank all genes in each cell cluster. It can then use this ranking to find sets of marker genes or to find highly variable genes.
genesorteR is orders of magnitude faster than current implementations of differential expression analysis methods and can operate on data containing millions of cells.

□ Read correction for non-uniform coverages
>> https://www.biorxiv.org/content/biorxiv/early/2019/06/25/673624.full.pdf
BCT, being scalable to large metagenomic datasets as well as correcting shallow single cell RNA-seq data, can be a general corrector for non-uniform data.
the graph cleaning strategy combined with the mapping strategy leads to save more rare k-mers, resulting in a more conservative correction than previous methods.
BCT is also capable to better take advantage of the signal of high depth datasets.

□ PROPERTIES OF A MULTIDIMENSIONAL LANDSCAPE MODEL FOR DETERMINING CELLULAR NETWORK THERMODYNAMICS https://www.biorxiv.org/content/biorxiv/early/2019/06/26/682690.full.pdf
A network can be characterized by a multidimensional potential landscape and a diffusion matrix of the dynamic fluctuations between N-number of intracellular network variables.
These steady state and dynamic features contribute to the heat associated with maintaining a nonequilibrium steady state. The Boltzmann H-function defines the rate of free energy dissipation of a system and provides a framework for determining the heat associated with the nonequilibrium steady state.
the measurable covariances in an NxN diffusion matrix, which contribute to the thermodynamics of the network together with the gradients of a landscape which are derived from the multi-dimensional steady state probability density. The nonequilibrium steady state in this open thermodynamic system is supported by an influx of free energy from outside the system, which is dissipated as heat.
□ diBELLA: Distributed Long Read to Long Read Alignment
>> https://people.eecs.berkeley.edu/~aydin/diBELLA_ICPP19.pdf
diBELLA, is the first distributed memory overlapper and aligner specifically designed for long reads and parallel scalability.
Alignment is a key step in long read assembly and other analysis problems, and often the dominant computation. diBELLA avoids the expensive all-to-all alignment by looking for short, error-free seeds (k-mers) and using those to identify potentially overlapping reads.

□ Graph analytics for phenome-genome associations inference
>> https://www.biorxiv.org/content/biorxiv/early/2019/06/26/682229.full.pdf
a statistical framework based on graph theory to infer direct associations between HPO and GO terms that do not share co-annotated genes.
The method enables to map genotypic features to phenotypic features thus providing a valid tool for bridging functional and pathological annotations.


□ Enabling Semantic Queries Across Federated Bioinformatics Databases
>> https://www.biorxiv.org/content/biorxiv/early/2019/06/28/686600.full.pdf
an ontology-driven approach to bioinformatic resource inte- gration. This approach enables complex federated queries across multiple domains of biological knowledge, such as gene expression and orthology, without requiring data duplication.
The integration of the three sources promises to open the path for novel comparative studies across species, for example through the analysis of orthologs (OMA) of human disease-causing genes (UniProt) and their expression patterns in model organisms (Bgee).
a federated SPARQL query endpoint along with an RDF store that exclusively contains metadata about the virtual links, and the SPARQL endpoints of the Uniprot, OMA and Bgee data stores.
These metadata based on the VoIDext schema precisely define and document how the distributed datasets can be interlinked.
□ Hierarchical domain model explains multifractal scaling of chromosome contact maps
>> https://www.biorxiv.org/content/biorxiv/early/2019/06/28/686279.full.pdf
a simple analytical model that describes the structure of chromosomes as a hierarchical set of domains nested in each other and solve it exactly.
The predicted multifractal spectrum is characterized by a phase transition between two phases with different fractal dimension, in excellent agreement with experimental data.

□ High-throughput identification of human SNPs affecting regulatory element activity
>> https://www.nature.com/articles/s41588-019-0455-2
leveraging the throughput and resolution of the survey of regulatory elements (SuRE) reporter technology to survey the effect of 5.9 million SNPs, including 57% of the known common SNPs, on enhancer and promoter activity.
And identified more than 30,000 SNPs that alter the activity of putative regulatory elements, partially in a cell-type-specific manner.

□ CAUSE: Mendelian randomization accounting for horizontal and correlated pleiotropic effects using genome-wide summary statistics
>> https://www.biorxiv.org/content/biorxiv/early/2019/06/26/682237.full.pdf
a new method (Causal Analysis Using Summary Effect Estimates; CAUSE) that uses genome-wide summary statistics to identify patterns that are consistent with causal effects, while accounting for pleiotropic effects, including correlated pleiotropy.
CAUSE identifies a smaller number of trait pairs as consistent with causal effects than methods that do not account for correlated pleiotropy. Many of the pairs that CAUSE does detect have a plausible causal connection.
□ D-Genies: dot plot large genomes in an interactive, efficient and simple way
>> https://peerj.com/articles/4958/
D-GENIES is a standalone and web application performing large genome alignments using minimap2 software package and generating interactive dot plots.
To limit minimap2 time and memory consumption, D-GENIES implements a chunking strategy. Large sequences are split in ten mega-base chunks which are aligned individually.
□ Pygenprop: a Python library for programmatic exploration and comparison of organism Genome Properties
>> https://academic.oup.com/bioinformatics/advance-article-abstract/doi/10.1093/bioinformatics/btz522/5522910
Pygenprop assigns YES, NO, or PARTIAL support for each property based on InterProScan annotations of open reading frames from an organism’s genome.
The library contains classes for representing the Genome Properties database as a whole and methods for detecting differences in property assignments between organisms.

□ A quantitative framework for evaluating single-cell data structure preservation by dimensionality reduction techniques
>> https://www.biorxiv.org/content/biorxiv/early/2019/06/27/684340.full.pdf
cursory exploration of the perplexity parameter in t-SNE and UMAP reveals a range of optimal values that yield favorable structure preservation metrics, endorsing the need for parameter optimization for dimensionality reduction of scRNA-seq datasets.
This isn an unbiased, quantitative framework for evaluation of data structure preservation by dimensionality reduction transformations.

□ Invariants of Frameshifted Variants
>> https://www.biorxiv.org/content/biorxiv/early/2019/06/27/684076.full.pdf
By analyzing complete proteomes from all three domains of life, several key physicochemical properties of protein sequences exhibit significant robustness against +1 and -1 frameshifts in their mRNA coding sequences.
frameshift invariance is directly embedded in the structure of the universal genetic code and may have contributed to shaping it.

□ Block Forests: random forests for blocks of clinical and omics covariate data
>> https://bmcbioinformatics.biomedcentral.com/articles/10.1186/s12859-019-2942-y
block forest outperformed all other approaches in the comparison study. In particular, it performed significantly better than standard random survival forest.
Block forest is particularly effective for the special case of using clinical covariates in combination with measurements of a single omics data type.
□ HiChIP-Peaks: A HiChIP peak calling algorithm
>> https://www.biorxiv.org/content/biorxiv/early/2019/06/27/682781.full.pdf
A new tool based on a representation of HiChIP data centred on the re-ligation sites to identify peaks from HiChIP datasets, which can subsequently be used in other tools for loop discovery. This increases the reliability of these tools and improves recall rate as sequencing depth is reduced.
□ JUCHMME: A Java Utility for Class Hidden Markov Models and Extensions for biological sequence analysis
>> https://academic.oup.com/bioinformatics/advance-article-abstract/doi/10.1093/bioinformatics/btz533/5524600
JUCHMME is an open-source software package designed to fit arbitrary custom Hidden Markov Models (HMMs) with a discrete alphabet of symbols.
JUCHMME integrates a wide range of decoding algorithms such as Viterbi, N–Best, posterior–Viterbi and Optimal Accuracy Posterior Decoder. Moreover, decoding of partially labeled data is offered with all algorithms in order to allow incorporation of experimental information.
To overcome HMM limitations, a number of extensions have been developed or developed such as segmental k–means both for Maximum Likelihood and for Conditional Maximum Likelihood, Hidden Neural Networks,
models that condition on previous observations and a method for semi-supervised learning of HMMs that can incorporate labeled, unlabeled and partially-labeled data (semi–supervised learning).

□ Priority index for human genetics and drug discovery
>> https://www.nature.com/articles/s41588-019-0460-5
a framework to prioritize potential targets by integrating genome-wide association data with genomic features, disease ontologies and network connectivity.
□ Isoform function prediction based on bi-random walks on a heterogeneous network
>> https://academic.oup.com/bioinformatics/advance-article-abstract/doi/10.1093/bioinformatics/btz535/5524603
IsoFun uses the available Gene Ontology annotations of genes, gene-gene interactions, and the relations between genes and isoforms to construct a heterogeneous network.
IsoFun performs a tailored bi-random walk on the heterogeneous network to predict the association between Gene Ontology terms and isoforms, thus accomplishing the prediction of GO annotations of isoforms.
□ Should we zero-inflate scVI?
>> https://yoseflab.github.io/2019/06/25/ZeroInflation/
a purely computational, data-driven approach to investigate whether scRNA-seq data is zero inflated.
rely on Bayesian model selection rules to determine for a given list of scRNA-seq datasets whether a zero-inflated model can fit the data significantly better.

□ FunSet: an open-source software and web server for performing and displaying Gene Ontology enrichment analysis
>> https://bmcbioinformatics.biomedcentral.com/articles/10.1186/s12859-019-2960-9
The enriched terms are displayed in a 2D plot that captures the semantic similarity between terms, with the option to cluster terms via spectral clustering and identify a representative term for each cluster.
while FunSet can determine an optimal number of clusters with the eigengap procedure, users still have the option (and are encouraged) to explore with different number of clusters, to identify groups of terms that match their biological intuition at the desired granularity level.
□ Evaluation of deep-learning-based lncRNA identification tools
>> https://www.biorxiv.org/content/biorxiv/early/2019/06/28/683425.full.pdf
Being aware of the difficulty of assembling full- length transcripts from RNA-seq dataset, LncADeep’s default model is for transcripts including partial-length.
LncADeep actually performs quite well for lncRNA identification, while Amin et al. used a non-default setting (i.e., model for full-length transcripts) of LncADeep to identify lncRNAs from transcripts including partial-length and much underestimated LncADeep.
□ Improving ATAC-seq Data Analysis with AIAP, a Quality Control and Integrative Analysis Package
>> https://www.biorxiv.org/content/biorxiv/early/2019/06/28/686808.full.pdf
optimized the analysis strategy for ATAC-seq and defined a series of QC metrics, including reads under peak ratio (RUPr), background (BG), promoter enrichment (ProEn), subsampling enrichment (SubEn), and other measurements.
incorporated these QC tests into our recently developed ATAC-seq Integrative Analysis Package (AIAP) to provide a complete ATAC-seq analysis system, including quality assurance, improved peak calling, and downstream differential analysis.
a significant improvement of sensitivity (20%~60%) in both peak calling and differential analysis by processing paired-end ATAC-seq datasets using AIAP. AIAP is compiled into Docker/Singularity, and with one command line execution, it generates a comprehensive QC report.
□ FastqCleaner: an interactive Bioconductor application for quality-control, filtering and trimming of FASTQ files
>> https://bmcbioinformatics.biomedcentral.com/articles/10.1186/s12859-019-2961-8
The interface shows diagnostic information for the input and output data and allows to select a series of filtering and trimming operations in an interactive framework.
It accepts files with qualities in both Phred+ 33 and Phred+ 64 encoding, detecting Sanger, Solexa and Illumina 1.3+, 1.5+, and > 1.8+ formats.
□ Principal Component Analysis for Multivariate Extremes
>> https://arxiv.org/pdf/1906.11043v1.pdf
Within the statistical learning framework of empirical risk minimization, the main focus is to analyze the squared reconstruction error for the exceedances over large radial thresholds. the empirical risk converges to the true risk, uniformly over all projection subspaces.
the best projection subspace is shown to converge in probability to the optimal one, in terms of the Hausdorff distance between their intersections with the unit sphere.

□ Deep Learning-Based Decoding of Constrained Sequence Codes
>> https://arxiv.org/pdf/1906.06172v1.pdf
using deep learning approaches to decode fixed-length and variable-length Constrained sequence (CS) codes.
the implementation of FL capacity- achieving CS codes with long codewords, which has been considered impractical, becomes practical with deep learning-based CS decoding.
fixed-length constrained sequence decoding based on multiple layer perception (MLP) networks and convolutional neural networks, to achieve low bit error rates that are close to maximum a posteriori probability (MAP) decoding as well as improve the system throughput.

□ PLIER: Pathway-level information extractor for gene expression data
>> https://www.nature.com/articles/s41592-019-0456-1
PLIER is a broadly applicable solution for the problem that outperforms available cell proportion inference algorithms and can automatically identify specific pathways that regulate gene expression.
PLIER improves interstudy replicability and reveals biological insights when applied to trans-eQTL (expression quantitative trait loci) identification.
□ Power series method for solving TASEP-based models of mRNA translation
>> https://www.biorxiv.org/content/biorxiv/early/2019/06/30/687335.full.pdf
the TASEP with codon-dependent elongation rates, premature termination due to ribosome drop-off and translation reinitiation due to circularisation of the mRNA.
a versatile method for studying TASEP-based models that account for several mechanistic details of the translation process: codon- dependent elongation, premature termination and mRNA circularisation.

□ Sourmash: Large-scale sequence comparisons:
>> https://www.biorxiv.org/content/biorxiv/early/2019/06/30/687285.full.pdf
version 2.0 of sourmash, a Python library for building and utilizing MinHash sketches of DNA, RNA, and protein data.
Sourmash is accomplished with two modifications: building sketches via a modulo approach, and implementing a modified Sequence Bloom Tree to enable both similarity and containment searches.

□ RNA proximity sequencing reveals the spatial organization of the transcriptome in the nucleus
>> https://www.nature.com/articles/s41587-019-0166-3
The simultaneous detection of multiple RNAs in proximity to each other distinguishes RNA-dense from sparse compartments.
Application of Proximity RNA-seq will facilitate study of the spatial organization of transcripts in the nucleus, including non-coding RNAs, and its functional relevance.
※コメント投稿者のブログIDはブログ作成者のみに通知されます