
□ PseudoGA: cell pseudotime reconstruction based on genetic algorithm
>> https://academic.oup.com/nar/advance-article/doi/10.1093/nar/gkab457/6318502
PseudoGA uses genetic algorithm to come up with a best possible trajectory of cells that explains expression patterns for individual genes. Another advantage of this method is that it can identify any lineage structure or branching while constructing pseudotime trajectory.
PseudoGA can capture expression that (i) increases or decreases (ii) increases - decreases (iii) increases - decreases - increases. assuming that ranks of gene expression values along pseudotime trajectory, can be either linear, quadratic or cubic function of the pseudo-time.
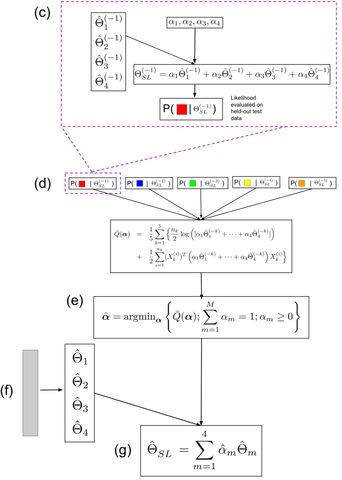
□ SpiderLearner: An ensemble approach to Gaussian graphical model estimation
>> https://www.biorxiv.org/content/10.1101/2021.07.13.452248v1.full.pdf
The Spider-Learner considers a library of candidate Gaussian graphical model (GGM) estimation methods and constructs the optimal convex combination of their results, eliminating the need for the researcher to make arbitrary decisions in the estimation process.
Under mild conditions on the loss function and the set of candidate learners, the expected difference between the risk of the Super Learner ensemble model and the risk of the oracle model converges to zero as the sample size goes to infinity.
□ Infinite re-reading of single proteins at single-amino-acid resolution using nanopore sequencing
>> https://www.biorxiv.org/content/10.1101/2021.07.13.452225v1
a system in which a DNA-peptide conjugate is pulled through a biological nanopore by a helicase that is walking on the DNA section.
This approach increases identification fidelity dramatically to 100% by obtaining indefinitely many independent re-readings of the same individual molecule with a succession of controlling helicases, eliminating the random errors that lead to inaccuracies in nanopore sequencing.
□ SiGraC: Node Similarity Based Graph Convolution for Link Prediction in Biological Networks
>> https://academic.oup.com/bioinformatics/advance-article-abstract/doi/10.1093/bioinformatics/btab464/6307262
Laplacian-based convolution is not well suited to single layered GCNs, as it limits the propagation of information to immediate neighbors of a node.
Coupling of Deep Graph Infomax (DGI’s) neural network architecture and loss function with convolution matrices that are based on node similarities can deliver superior link prediction performance as compared to convolution matrices that directly incorporate the adjacency matrix.
□ FlowGrid enables fast clustering of very large single-cell RNA-seq data
>> https://academic.oup.com/bioinformatics/advance-article/doi/10.1093/bioinformatics/btab521/6325016
a new automated parameter tuning procedure, FlowGrid can achieve comparable clustering accuracy as state-of-the-art clustering algorithms but at a substantially reduced run time. FlowGrid can complete a 1-hour clustering task for one million cells in about 5 minutes.
FlowGrid combines the benefit of DBSCAN and a grid-based approach to achieve scalability. The key idea of FlowGrid algorithm is to replace the calculation of density from individual points to discrete bins as defined by a uniform grid.
□ SDImpute: A statistical block imputation method based on cell-level and gene-level information for dropouts in single-cell RNA-seq data
>> https://journals.plos.org/ploscompbiol/article?id=10.1371/journal.pcbi.1009118
SDImpute automatically identifies the dropout events based on the gene expression levels and the variations of gene expression across similar cells and similar genes, and it implements block imputation for dropouts by utilizing gene expression unaffected by dropouts from similar cells.
SDImpute combines gene expression levels and the variations of gene expression across similar cells and similar genes to construct a dropout index matrix to identify dropout events and true zeros. It can be considered the expression of single cells in a one-dimensional manifold.
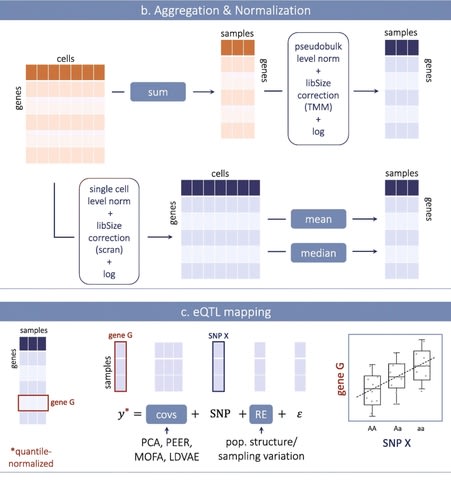
□ Optimizing expression quantitative trait locus mapping workflows for single-cell studies
>> https://genomebiology.biomedcentral.com/articles/10.1186/s13059-021-02407-x
Following methodological choices are currently optimal: scran normalization; mean aggregation of expression across cells from one donor (and sequencing run/batch if relevant);
including principal components as covariates in the Linear mixed models (LMM); including a random effect capturing sampling variation in the LMM; and accounting for multiple testing by using the conditional false discovery rate.
□ Accelerated regression-based summary statistics for discrete stochastic systems via approximate simulators
>> https://bmcbioinformatics.biomedcentral.com/articles/10.1186/s12859-021-04255-9
an approximate ratio estimator to inform when the approximation is significantly different and thus when need to simulate using the stochastic simulation algorithm (SSA) to prevent bias.
For the approximate simulators, ODE trajectories were generated using the adaptive LSODA integrator and τ-Leaping trajectories were generated using the adaptive τ-Leaping algorithm.
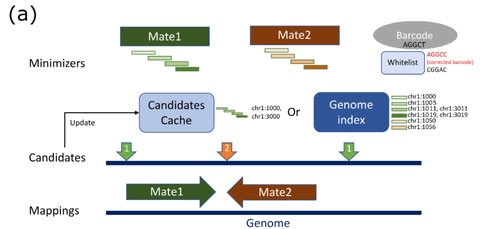
□ Chromap: Fast alignment and preprocessing of chromatin profiles
>> https://www.biorxiv.org/content/10.1101/2021.06.18.448995v1.full.pdf
Chromap, an ultrafast method for aligning chromatin profiles. Chromap is comparable to BWA-MEM and Bowtie2 in alignment accuracy and is over 10 times faster than other workflows on bulk ChIP-seq / Hi-C profiles and than 10x Genomics’ CellRanger v2.0.0 on scATAC-seq profiles.
Chromap considers every minimizer hit and uses the mate-pair information to rescue remaining missing alignments. Chromap caches the candidate read alignment locations in those regions to accelerate alignment of future reads containing the same minimizers.
□ Ultraplex: A rapid, flexible, all-in-one fastq demultiplexer
>> https://wellcomeopenresearch.org/articles/6-141
Ultraplex, a fast and uniquely flexible demultiplexer which splits a raw FASTQ file containing barcodes either at a single end or at both 5’ and 3’ ends of reads, trims the sequencing adaptors and low-quality bases, and moves UMIs into the read header.
Ultraplex is able to perform such single or combinatorial demultiplexing on both single- and paired-end sequencing data, and can process an entire Illumina HiSeq lane, consisting of nearly 500 million reads, in less than 20 minutes.
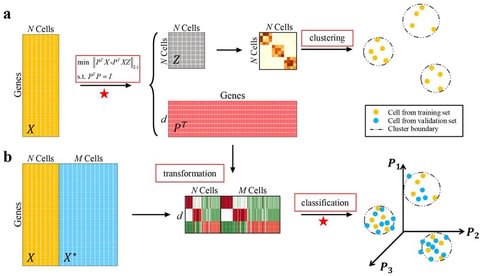
□ scDA: Single cell discriminant analysis for single-cell RNA sequencing data
>> https://www.sciencedirect.com/science/article/pii/S2001037021002270
Single cell discriminant analysis (scDA) simultaneously identifies cell groups and discriminant metagenes based on the construction of cell-by-cell representation graph, and then using them to annotate unlabeled cells in data.
With the optimal representation matrix, scDA is capable to estimate the involved cell types through a graph-based clustering method, e.g., spectral clustering; and classify the unlabeled cells to the acquired assignments based on discriminant vectors.
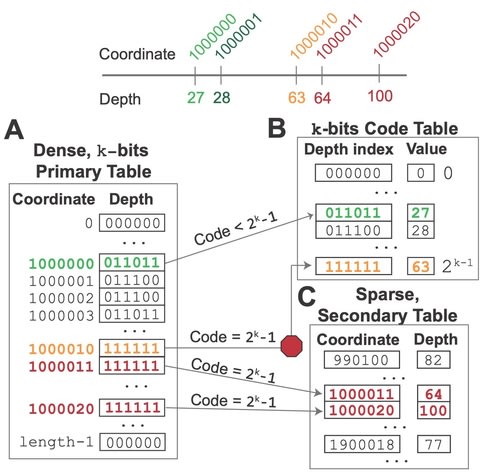
□ D4 - Dense Depth Data Dump: Balancing efficient analysis and storage of quantitative genomics data with the D4 format and d4tools
>> https://www.nature.com/articles/s43588-021-00085-0
The D4 format is adaptive in that it profiles a random sample of aligned sequence depth from the input sequence file to determine an optimal encoding that enables fast data access.
D4 algorithm uses a binary heap that fills with incoming alignments as it reports depth. Using this low entropy to efficiently encode quantitative genomics data in the D4 format. The average time complexity of this algorithm is linear with respect to the number of alignments.

□ SLOW5: a new file format enables massive acceleration of nanopore sequencing data analysis
>> https://www.biorxiv.org/content/10.1101/2021.06.29.450255v1.full.pdf
SLOW5 is a simple tab-separated values (TSV) file encoding metadata and time-series signal data for one nanopore read per line, with global metadata stored in a file header.
SLOW5 can be encoded in binary format (BLOW5) - this is analogous to the seminal SAM/BAM format for storing DNA sequence alignments. BLOW5 can be compressed using standard zlib, thereby minimising the data storage footprint while still permitting efficient parallel access.
Using a GPU- accelerated version of Nanopolish (described elsewhere21), with compressed-BLOW5 input data, we were able to complete whole-genome methylation profiling on a single 30X human dataset in just 10.5 hours with 48 threads.
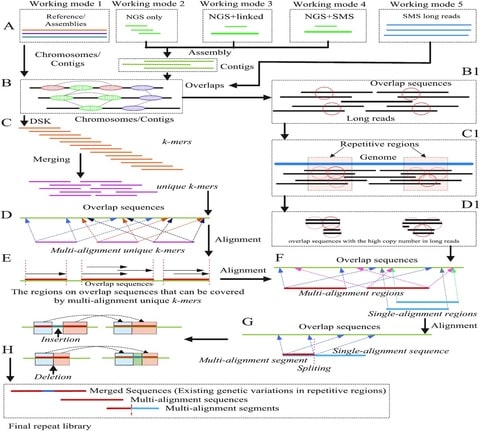
□ LongRepMarker: A sensitive repeat identification framework based on short and long reads
>> https://academic.oup.com/nar/advance-article/doi/10.1093/nar/gkab563/6313241
LongRepMarker uses the multiple sequence alignment to find the unique k-mers which can be aligned to different locations on overlap sequences and the regions on overlap sequences that can be covered by these multi-alignment unique k-mers.
The parallel alignment model based on the multi-alignment unique k-mers can greatly optimize the efficiency of data processing in LongRepMarker. By taking the corresponding identification strategies, structural variations that occur between repeats can be identified.
□ Dynamic Bayesian Network Learning to Infer Sparse Models from Time Series Gene Expression Data
>> https://ieeexplore.ieee.org/document/9466470/
Two new BN scoring functions, which are extensions to the Bayesian Information Criterion (BIC) score, with additional penalty terms and use them in conjunction with DBN structure search methods to find a graph structure that maximises the proposed scores.
GRNs are typically sparse but traditional approaches of BN structure learning to elucidate GRNs produce many spurious edges. This BN scoring offer better solutions with fewer spurious edges. These algorithms are able to learn sparse graphs from high-dimensional time series data.
□ Linear functional organization of the omic embedding space
>> https://academic.oup.com/bioinformatics/advance-article/doi/10.1093/bioinformatics/btab487/6313162
the Graphlet Degree Vector Positive Pointwise Mutual Information (PPMI) matrix of the PPI network to capture different topological (structural) similarities of the nodes in the molecular network.
the embeddings obtained by the Non-Negative Matrix Tri-Factorization-based decompositions of the PPMI matrix, as well as of the GDV PPMI matrix, compared to the SVD-based decompositions, uncover more enriched clusters and more enriched genes in the obtained clusters.
□ S4PRED: Increasing the Accuracy of Single Sequence Prediction Methods Using a Deep Semi-Supervised Learning Framework
>> https://academic.oup.com/bioinformatics/advance-article/doi/10.1093/bioinformatics/btab491/6313164
PASS - Profile Augmentation of Single Sequences, a general framework for mapping multiple sequence information to cases where rapid and accurate predictions are required for orphan sequences.
S4PRED uses a variant of the powerful AWD-LSTM. S4PRED uses the PASS framework to develop a pseudo-labelling approach that is used to generate a large set of single sequences with highly accurate artificial labels.
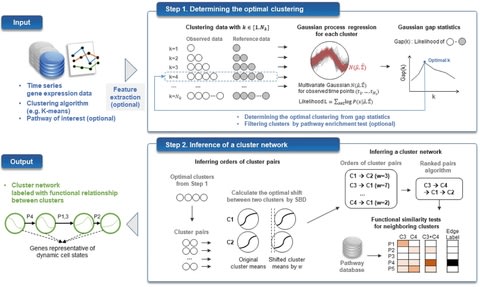
□ TRACS: Inferring transcriptomic cell states and transitions only from time series transcriptome data
>> https://www.nature.com/articles/s41598-021-91752-9
TRACS, a novel time series clustering framework to infer TRAnscriptomic Cellular States only from time series transcriptome data by integrating Gaussian process regression, shape-based distance, and ranked pairs algorithm in a single computational framework.
TRACS determines patterns that correspond to hidden cellular states by clustering gene expression data. The final output of TRACS is a cluster network describing dynamic cell states and transitions by ordered clusters, where cluster genes imply representative genes of each cell state.
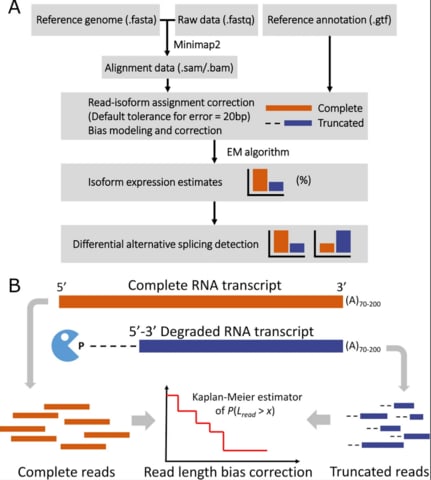
□ LIQA: long-read isoform quantification and analysis
>> https://genomebiology.biomedcentral.com/articles/10.1186/s13059-021-02399-8
LIQA is the first long-read transcriptomic tool that takes these limitations of long-read RNA-seq data into account. LIQA models observed splicing information, high error rate of data, and read length bias.
LIQA is computationally intensive because the approximation of nonparametric Kaplan-Meier estimator of function f(Lr) relies on empirical read length distribution and the parameters are estimated using EM algorithm.
□ libOmexMeta: Enabling semantic annotation of models to support FAIR principles
>> https://academic.oup.com/bioinformatics/advance-article-abstract/doi/10.1093/bioinformatics/btab445/6300512
The goal of semantic annotations are to make explicit the biology that underlies the semantics of biosimulation models. LibOmexMeta is a library aimed at providing developer-level support for reading, writing, editing and managing semantic annotations for biosimulation models.

□ GPcounts: Non-parametric modelling of temporal and spatial counts data from RNA-seq experiments
>> https://academic.oup.com/bioinformatics/advance-article/doi/10.1093/bioinformatics/btab486/6313161
GPcounts is Gaussian process regression package for counts data with negative binomial and zero-inflated negative binomial likelihoods. GPcounts can be used to model temporal and spatial counts data in cases where simpler Gaussian and Poisson likelihoods are unrealistic.
GPcounts uses a Gaussian process with a logarithmic link function to model variation in the mean of the counts data distribution across time or space.
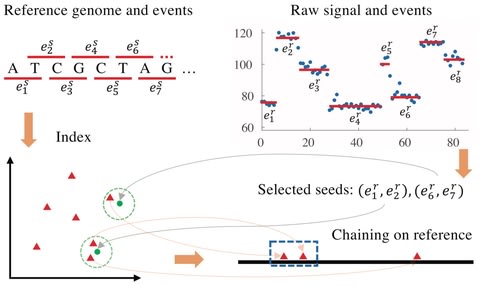
□ Sigmap: Real-time mapping of nanopore raw signals
>> https://academic.oup.com/bioinformatics/article/37/Supplement_1/i477/6319675
Sigmap is a streaming method for mapping raw nanopore signal to reference genomes. The method features a new way to index reference genomes using k-d trees, a novel seed selection strategy and a seed chaining algorithm tailored toward the current signal characteristics.
The method avoids any conversion of signals to sequences and fully works in signal space, which holds promise for completely base-calling-free nanopore sequencing data analysis.

□ CVAE–NCEM: Learning cell communication from spatial graphs of cells
>> https://www.biorxiv.org/content/10.1101/2021.07.11.451750v1.full.pdf
Node-centric expression modeling (NCEM), a computational method based on graph neural networks which reconciles variance attribution and communication modeling in a single model of tissue niches.
NCEMs can be extended to mixed models of explicit cell communication events and latent intrinsic sources of variation in conditional variational autoencoders to yield holistic models of cellular variation in spatial molecular profiling data.
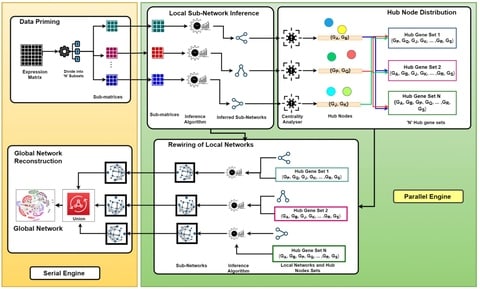
□ Parallel Framework for Inferring Genome-Scale Gene Regulatory Networks
>> https://www.biorxiv.org/content/10.1101/2021.07.11.451988v1.full.pdf
a generic parallel inference framework using which any original inference algorithm without any alterations, can parallelly run on humongous datasets in the multiple cores of the CPU to provide efficacious inferred networks.
a strict use of the data about the application executions within the formula for Amdahl's Law gives a much more pessimistic estimate than the scaled speedup formula.
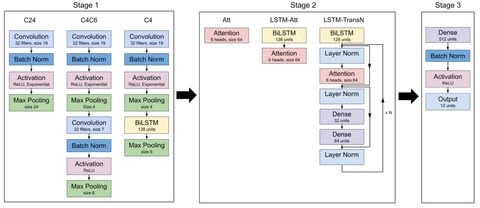
□ Designing Interpretable Convolution-Based Hybrid Networks for Genomics
>> https://www.biorxiv.org/content/10.1101/2021.07.13.452181v1.full.pdf
Systematically investigate the extent that architectural choices in convolution-based hybrid networks influence learned motif representations in first layer filters, as well as the reliability of their attribution maps generated by saliency analysis.
As attention-based models are gaining interest in regulatory genomics, hybrid networks would benefit from incorporating these design principles to bolster their intrinsic interpretability.
□ HieRFIT: A hierarchical cell type classification tool for projections from complex single-cell atlas datasets
>> https://academic.oup.com/bioinformatics/advance-article-abstract/doi/10.1093/bioinformatics/btab499/6320801
HieRFIT (Hierarchical Random Forest for Information Transfer) uses a priori information about cell type relationships to improve classification accuracy, taking as input a hierarchical tree structure representing the class relationships, along with the reference data.
HieRFIT uses an ensemble approach combining multiple random forest models, organized in a hierarchical decision tree structure. HieRFIT improves accuracy and reduces incorrect predictions especially for inter-dataset tasks which reflect real life applications.
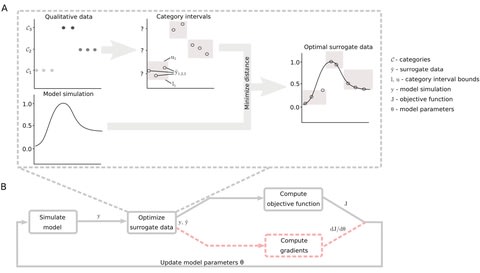
□ Efficient gradient-based parameter estimation for dynamic models using qualitative data
>> https://academic.oup.com/bioinformatics/advance-article/doi/10.1093/bioinformatics/btab512/6321450
a semi-analytical algorithm for gradient calculation of the optimal scaling method developed for qualitative data. This enables the use of efficient gradient-based optimization algorithms.
Validating the accuracy of the obtained gradients by comparing them to finite differences and assessed the advantage of using gradient information on five application examples by performing optimization with a gradient-free and a gradient-based algorithm.
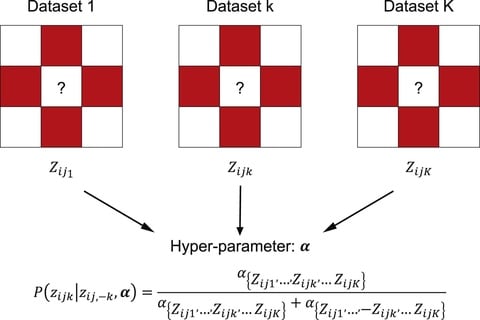
□ MUNIn: A statistical framework for identifying long-range chromatin interactions from multiple samples
>> https://www.cell.com/hgg-advances/fulltext/S2666-2477(21)00017-8
MUNIn (multiple-sample unifying long-range chromatin-interaction detector) MUNIn adopts a hierarchical hidden Markov random field (H-HMRF) model.
MUNIn jointly models multiple samples and explicitly accounts for the dependency across samples. It simultaneously accounts for both spatial dependency within each sample and dependency across samples.
□ xPore: Identification of differential RNA modifications from nanopore direct RNA sequencing
>> https://www.nature.com/articles/s41587-021-00949-w
RNA modifications can be identified from direct RNA-seq data with high accuracy, enabling analysis of differential modifications and expression from a single high-throughput experiment.
xPore identifies positions of m6A sites at single-base resolution, estimates the fraction of modified RNA species in the cell and quantifies the differential modification rate across conditions.

□ ELIMINATOR: Essentiality anaLysIs using MultIsystem Networks And inTeger prOgRamming
>> https://www.biorxiv.org/content/10.1101/2021.07.21.453265v1.full.pdf
ELIMINATOR, an in-silico method for the identification of patient-specific essential genes using constraint-based modelling (CBM). It expands the ideas behind traditional CBM to accommodate multisystem networks, that is a biological network that focuses on complex interactions.
ELIMINATOR calculates the minimum number of non-expressed genes required to be active by the cell to sustain life as defined by a set of requirements; and performs an exhaustive in-silico gene knockout to find those that lead to the need of activating extra non-expressed genes.
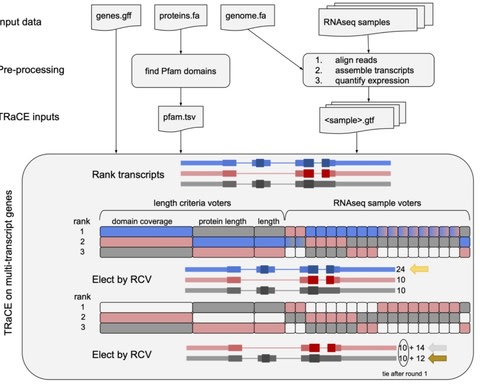
□ TRaCE: Ranked Choice Voting for Representative Transcripts
>> https://academic.oup.com/bioinformatics/advance-article/doi/10.1093/bioinformatics/btab542/6326792
TRaCE (Transcript Ranking and Canonical Election) holds an ‘election’ in which a set of RNA-seq samples rank transcripts by annotation edit distance.
TRaCE identies the most common isoforms from a broad expression atlas or prioritize alternative transcripts expressed in specific contexts. TRaCE tallies votes for top-ranked candidates; as there is a tie for first place, votes for the subsequent rankings are added to the tally.
□ NMFLRR: Clustering scRNA-seq data by integrating non-negative matrix factorization with low rank representation
>> https://ieeexplore.ieee.org/document/9495191/
NMFLRR, a new computational framework to identify cell types by integrating low-rank representation (LRR) and nonnegative matrix factorization (NMF).
The LRR captures the global properties of original data by using nuclear norms, and a locality constrained graph regularization term is introduced to characterize the data's local geometric information.
The similarity matrix and low-dimensional features of data can be simultaneously obtained by applying the ADMM algorithm to handle each variable alternatively in an iterative way. NMFLRR uses a spectral algorithm based on the optimized similarity matrix.
□ SDPR: A fast and robust Bayesian nonparametric method for prediction of complex traits using summary statistics
>> https://journals.plos.org/plosgenetics/article?id=10.1371/journal.pgen.1009697
SDPR connects the marginal coefficients in summary statistics with true effect sizes through Bayesian multiple Dirichlet process regression.
SDPR utilizes the concept of approximately independent LD blocks and overparametrization to develop a parallel and fast-mixing MCMC algorithm. SDPR can provide estimation of heritability, genetic architecture, and posterior inclusion probability.
□ Using topic modeling to detect cellular crosstalk in scRNA-seq
>> https://www.biorxiv.org/content/10.1101/2021.07.26.453767v1.full.pdf
a new method for detecting genes that change as a result of interaction based on Latent Dirichlet Allocation (LDA). This method does not require prior information in the form of clustering or generation of synthetic reference profiles.
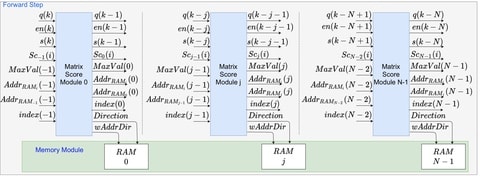
□ Parallel Implementation of Smith-Waterman Algorithm on FPGA
>> https://www.biorxiv.org/content/10.1101/2021.07.27.454006v1.full.pdf
The development of the algorithm was carried out using the development platform provided by the Field-Programmable Gate Array (FPGA) manufacturer, in this case, Xilinx.
From the strategy of storing alignment path distances and maximum score position during Forward Stage processing. It was possible to reduce the complexity of Backtracking Stage processing which allowed to follow the path directly.
This platform allows the user to develop circuits using the block diagram strategy instead of VHDL or Verilog. The architecture was deployed on the FPGA Virtex-6 XC6VLX240T.
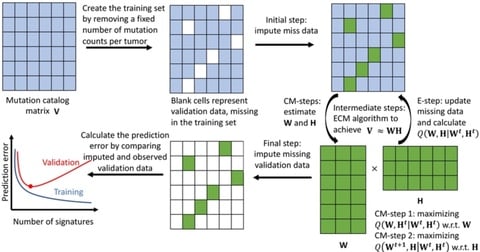
□ SUITOR: selecting the number of mutational signatures through cross-validation
>> https://www.biorxiv.org/content/10.1101/2021.07.28.454269v1.full.pdf
SUITOR (Selecting the nUmber of mutatIonal signaTures thrOugh cRoss-validation), an unsupervised cross-validation method that requires little assumptions and no numerical approximations to select the optimal number of signatures without overfitting the data.
SUITOR extends the probabilistic model to allow missing data in the training set, which makes cross-validation feasible. an expectation/conditional maximization algorithm to extract signature profiles, estimate mutation contributions and impute the missing data simultaneously.
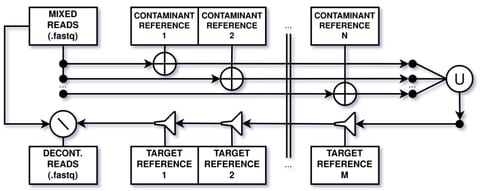
□ WGA-LP: a pipeline for Whole Genome Assembly of contaminated reads
>> https://www.biorxiv.org/content/10.1101/2021.07.31.454518v1.full.pdf
WGA-LP connects state-of-art programs and novel scripts to check and improve the quality of both samples and resulting assemblies. With its conservative decontamination approach, has shown to be capable of creating high quality assemblies even in the case of contaminated reads.
WGA-LP includes custom scripts to help in the visualization of node coverage by post processing the output of Samtools depth. For node reordering, WGA-LP uses the ContigOrderer option from Mauve aligner.
※コメント投稿者のブログIDはブログ作成者のみに通知されます