

□ GCNCMI: A Graph Convolutional Neural Network Approach for Predicting circRNA-miRNA Interactions
>> https://www.frontiersin.org/articles/10.3389/fgene.2022.959701/full
GCNCMI predicts potential interactions between circRNAs and miRNAs. GCNCMI mines the latent interactions of adjacent nodes in a graph convolutional neural network, and then recursively propagates the interaction information on the graph convolutional layers.
GCNCMI propagates the information flow recursively over the graph structure and continuously aggregate the information of neighboring nodes to refine the embedding representation. GCNCMI concatenates the embeddings from different propagation layers and make the final prediction.

□ FFP: joint Fast Fourier transform and fractal dimension in amino acid property-aware phylogenetic analysis
>> https://bmcbioinformatics.biomedcentral.com/articles/10.1186/s12859-022-04889-3
Fractal dimension describes the complexity of geometric objects. Smits used HFD to monitor the complexity of brain activity. There exists similarity between the whole and part of the protein sequence, so they can be represented by fractal curve.
FFP, it is a hybrid method for APPA. the primary amino acid sequence is converted into digital sequence using the pKa(COOH) value, which is critical for the dissociation constant. The feature vector of each protein is generated by integrating FFT and HFD.

□ BayesRCπ: Accounting for overlapping annotations in genomic prediction models of complex traits
>> https://bmcbioinformatics.biomedcentral.com/articles/10.1186/s12859-022-04914-5
BayesRCπ and BayesRC+ incorporate biological information in different ways, their performance is likely to be highly dependent on the underlying genetic architecture, the construction of annotation categories, and the biological relevance of the prior information.
The BayesRCπ model with a mixture of mixtures prior distribution on SNP effects, thus allowing multi-annotated SNPs to be assigned a posteriori to the most informative annotation. The BayesRC+ model assigns an additive impact of multiple annotation categories.
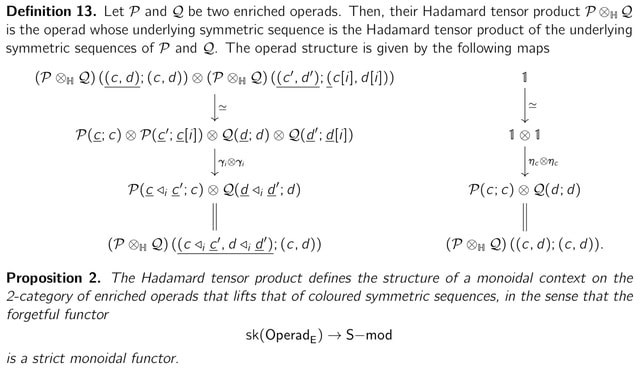
□ Mapping coalgebras II: Operads
>> https://arxiv.org/pdf/2208.14395v1.pdf
The Hadamard tensor product defines the structure of a monoidal context on the 2-category of enriched operads that lifts that of coloured symmetric sequences, in the sense that the forgetful functor sk(OperadE) → S−mod is a strict monoidal functor.
Monochromatic enriched operads are themselves algebras over a set-theoretical operad Op. Hence, the category AlgE (Op) of monochromatic enriched operads have the structure of a symmetric monoidal category ; this is the Hadamard tensor product.
Moreover, the category the category AlgE (Op) of Op-coalgebras endow a closed symmetric monoidal structure and operads are enriched-tensored-cotensored over Op-coalgebras.

□ Dual Fusion 2-Categories
>> https://arxiv.org/pdf/2208.08722v1.pdf
Given a fusion 2-category and a suitable module 2-category, the dual tensor 2-category is the associated 2-category of module 2-endofunctors. Proving the relative tensor product of modules over a separable algebra in a fusion 2-category exists.
Over a fusion 2-category, the 2-adjoint of a left module 2-functor carries a canonical left module structure. The dual tensor 2-category with respect to a separable module 2-category is a multifusion 2-category.

□ hCoCena: Horizontal integration and analysis of transcriptomics datasets
>> https://academic.oup.com/bioinformatics/advance-article/doi/10.1093/bioinformatics/btac589/6677225
Horizontal-CoCena (hCoCena: horizontal construction of co-expression networks and analysis) allows for the analysis of a single transcriptomic dataset, using a co-expression network for the identification of gene clusters and their subsequent functional analysis.
hCocena is a completely remastered, stand-alone. hCoCena’s ready-to-use workflow implementation is provided as an R markdown file utilizing the package functions with minimal code exposure and detailed descriptions of all in-and outputs as well as function parameters.

□ MMGraph: a multiple motif predictor based on graph neural network and coexisting probability for ATAC-seq data
>> https://academic.oup.com/bioinformatics/advance-article/doi/10.1093/bioinformatics/btac572/6673903
MMGraph is based on GNN and coexisting probability of k-mers, where the coexisting probability represents the degree of association between k-mers. MMGraph decomposes the heterogeneous graph into three sub-graphs, i.e. similarity graph, coexisting graph, and inclusive graph.
MMGraph consists of three components: a heterogeneous graph; a three-layer GNN model to get embeddings of k-mers and sequences; coexisting probability calculation for finding multiple motifs.

□ DA-DSL-L2: A novel meta-analysis based on data augmentation and elastic data shared lasso regularization for gene expression
>> https://bmcbioinformatics.biomedcentral.com/articles/10.1186/s12859-022-04887-5
DA-DSL-L2 is based on a new data augmentation (DA) strategy and elastic data shared lasso method. Various CPN methods exist that can preserve original biological information of gene expression datasets from different angles and add different “perturbations” to the dataset.
DA-DSL-L2 transforms the DSL-L2 method to a standard Lasso problem. Even though the Lasso problem can be solved by some very efficient method, i.e., glmnet, to solve a big matrix such as a matrix size of over 40,000 * 40,000.

□ IsofunGO: Isoform function prediction by Gene Ontology embedding
>> https://academic.oup.com/bioinformatics/advance-article-abstract/doi/10.1093/bioinformatics/btac576/6673907
IsofunGO firstly introduces an attributed hierarchical network to model massive GO terms, and a GO network embedding strategy to learn compact representations of GO terms and project GO annotations of genes into compressed ones.
It develops an attention based multi-instance learning network to fuse genomics and transcriptomics data of isoforms and predict isoform functions by referring to compressed annotations.

□ scraps: an end-to-end pipeline for measuring alternative polyadenylation at high resolution using single-cell RNA-seq
>> https://www.biorxiv.org/content/10.1101/2022.08.22.504859v1
scraps (Single Cell RNA PolyA Site Discovery), a scalable, and reproducible end-to-end workflow, to identify polyadenylation sites at near-nucleotide resolution in single cells using 10X Genomics and other TVN-primed single-cell RNA-seq (scRNA-seq) libraries.
scraps performs best with long read 1 sequencing and paired alignment, is both unbiased relative to existing methods that utilize only read 2 and recovers more sites, despite the reduction in read quality observed on most modern DNA sequencers following homopolymer stretches.

□ CellDrift: inferring perturbation responses in temporally sampled single-cell data
>> https://academic.oup.com/bib/advance-article/doi/10.1093/bib/bbac324/6673850
CellDrift, a Generalized linear models (GLM)-based Functional data analysis model, to disentangle temporal patterns in perturbation responses in scRNA- seq data.
CellDrift first captures cell-type specific perturbation effects by adding an interaction term in the GLM and then utilizes predicted coefficients to calculate contrast coefficients, which represent perturbation effects.
Concatenated contrast coefficients over time are defined as functions, and Fuzzy C-mean clustering is used to identify temporal patterns, which is accompanied by FPCA to find the major components that account for the most temporal variance.

□ DeepGenePrior: A deep learning model to prioritize genes affected by copy number variants
>> https://www.biorxiv.org/content/10.1101/2022.08.22.504862v1
DeepGenePrior aims to uncover the genes contributing to the target disease and the underlying relationshio patterns. Based on the copy number variants of all cases and controls, they train a network, then use the model weights to calculate scores.
The model tries to encode the inputs into a Gaussian distribution with estimated mean and covariance. with DECIPHER data source, DeepGenePrior investigates how mutations in the detected genes influence other traits, and gene ontology analyses were also conducted.

□ PyWGCNA: A Python package for weighted gene co-expression network analysis
>> https://www.biorxiv.org/content/10.1101/2022.08.22.504852v1
PyWGCNA is designed to do Weighted correlation network analysis (WGCNA) can be used for finding clusters of highly correlated genes, for summarizing such clusters using the module eigengene for relating modules to one another and to external sample traits.
PyWGCNA can directly perform Gene Ontology enrichment on co-expression modules to characterize the functional activity of each module and supports addition or removal of data to allow for iterative improvement on network construction as new samples become available or defunct.

□ SPEX: A modular end-to-end analytics tool for spatially resolved omics of tissues
>> https://www.biorxiv.org/content/10.1101/2022.08.22.504841v1
SPEX (Spatial Expression Explorer), a comprehensive image analysis software implemented as a user- friendly web-based application with modules that can be put together by the user as pipelines conveniently through a graphical user interface.
SPEX introduced the novel application of the CLQ methodology. SPEX provides a clustering module that accommodates both proteomics and transcriptomics inputs. SPEX includes a modular pipeline to facilitate tissue-based single-cell segmentation.

□ Identifying and correcting repeat-calling errors in nanopore sequencing of telomeres
>> https://genomebiology.biomedcentral.com/articles/10.1186/s13059-022-02751-6
Telomeric regions were frequently miscalled as other types of repeats in a strand-specific manner. Specifically, although human telomeres are typically represented by (TTAGGG)n repeats, these regions were frequently recorded as (TTAAAA)n repeats.
When examining the reverse complementary strand of the telomeres which are represented as (CCCTAA)n repeats, we instead observed frequent substitution of these regions by (CTTCTT)n and (CCCTGG)n repeats.
The examination of each telomeric long read also indicates that these error repeats frequently co-occur with telomeric repeats at the ends of each read, and are observed on all chromosomal arms of CHM13.

□ DeDoc2 identifies and characterizes the hierarchy and dynamics of chromatin TAD-like domains in the single cells
>> https://www.biorxiv.org/content/10.1101/2022.08.23.505046v1
deDoc2 is a TAD-like domain(TLD) prediction tool using structural information theory, it treats the Hi-C contact map as a weighted graph, and applys dynamic programming algorithm to globally optimize the two-dimensional structural entropy of the graph partiton.
The deDoc2.w minimizes the structural entropy in the whole Hi-C contact map, while the deDoc2.s minimizes the structural entropy in the matrices of sliding windows along the genome. deDoc2.binsize determines the optimal binsize with normalized decoding information.
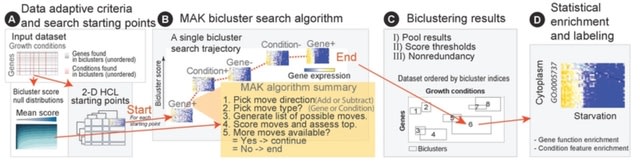
□ Deep surveys of transcriptional modules with Massive Associative Kbiclustering (MAK)
>> https://www.biorxiv.org/content/10.1101/2022.08.26.505372v1
Unsupervised Massive Associative K-biclustering (MAK) approach corrects this size bias while preserving high bicluster coherence both on simulated datasets with known ground truth and on real world data without, where we apply a new measure to evaluate biclustering.
MAK jointly maximizes bicluster coherence with biological enrichment and finds the most enriched biological functions. MAK reports the second-most enriched non-protein production functions, with higher bicluster coherence and arrayed across a large number of biclusters.

□ UltraSEQ: a universal bioinformatic platform for information-based clinical metagenomics and beyond
>> https://www.biorxiv.org/content/10.1101/2022.08.24.505213v1.full.pdf
UltraSEQ uses a novel, information-based approach that leverages a fast aligner that can handle both DNA and protein database to make sample-level predictions at the most specific taxonomic levels possible given the information in the sample and the database(s) used.
UltraSEQ was built from the ground up to make predictions for regions of sequences (including taxonomic binning), full sequences, and collections of sequences (i.e., a sample) without complicated user settings and the necessity for background subtraction.

□ SCOIT: Probabilistic tensor decomposition extracts better latent embeddings from single-cell multiomic data
>> https://www.biorxiv.org/content/10.1101/2022.08.26.505382v1
SCOIT is incorporated various distributions, including Gaussian, Poisson, and negative binomial distributions. SCOIT constructs a multiomic tensor with a union set of features. Second, it performs the probabilistic tensor decomposition.
SCOIT generates embedding matrices for omics, cells, and genes. SCOIT incorporates the global and local embeddings to capture global and local variability. SCOIT applies the Gaussian distribution for the continuous data type and the NBD for the count data with high variance.

□ APSCALE: advanced pipeline for simple yet comprehensive analyses of DNA Meta-barcoding data
>> https://academic.oup.com/bioinformatics/advance-article/doi/10.1093/bioinformatics/btac588/6677653
Apscale is a metabarcoding pipeline that handles the most common tasks in metabarcoding pipelines like paired-end merging, primer trimming, quality filtering, otu clustering and denoising as well as an otu filtering step.
APSCALE offers an internal python-based version of the LULU (Frøslev et al. 2017), an algorithm for post-clustering curation that aims to provide more reliable biodiversity estimates. Both OTUs and ESVs are filtered using the LULU to reduce the number of erroneous OTUs and ESVs.

□ Aclust2.0: a revamped unsupervised R tool for Infinium methylation beadchips data analyses
>> https://academic.oup.com/bioinformatics/advance-article-abstract/doi/10.1093/bioinformatics/btac583/6677241
Aclust, one of the first unsupervised algorithms, was originally designed to analyze regional methylation of Infinium’s 27K and 450K arrays by clustering neighboring methylation sites prior to downstream analyses.
“aclust2.0.R” script provides all the necessary guidelines. “function GEE.clusters” runs GEE models with the identified clusters and takes as input “clusters.list”, betas data, exposure, covariates, “id” which is the column name of betas, and the correlation structure specification.

□ DeepBSA: A deep-learning algorithm improves bulked segregant analysis for dissecting complex traits
>> https://www.cell.com/molecular-plant/pdf/S1674-2052(22)00267-2.pdf
DeepBSA performs well in QTL mapping of multiple loci with marginal effects. DeepBSA usually requires shallower sequencing depth than alternative methods, making it more easily adoptable.
DeepBSA identifies the number of bulked pools automatically and integrates multiple algorithms. DeepBSA only requires pooled data; ΔSNP-index requires parental sequencing as a control. DeepBSA requires a simple input with standard VCF, whereas QTG-seq requires a gff annotation.

□ CoAtGIN: Marrying Convolution and Attention for Graph-based Molecule Property Prediction
>> https://www.biorxiv.org/content/10.1101/2022.08.26.505499v1
CoAtGIN uses the k-hop convolution in a graph convolution network for faster message aggregation within one iteration. CoAtGIN presents a new way to accomplish global message passing through the graph using the linear transformer.
CoAtGIN is composed of L layers. Each layer takes the Node Embedding (NE) and Graph Embedding (GE) as input, and then these two embeddings will be updated for layerwise iteration. CoAtGIN initializes the NE as the atom type of each node. And the GE are set to zeros.

□ SeQuiLa: Cloud-native distributed genomic pileup operations
>> https://www.biorxiv.org/content/10.1101/2022.08.27.475646v1
SeQuiLa, a scalable, distributed, and efficient implementation of a pileup algorithm that is suitable for deploying in cloud computing environments.
SeQuiLa is implemented a novel and unique approach to process alignment events from sequencing reads using the MD tags, the source code micro-optimizations for recurrent operations, and a modular structure of the algorithm.

□ Multiset partial least squares with rank order of groups for integrating multi-omics data
>> https://www.biorxiv.org/content/10.1101/2022.08.30.505949v1
Multiset partial least squares (PLS) is formulated as maximization of sum of covariance between scores for all combinations of each explanatory variables and between score for response and each explanatory variable.
multiset PLS-ROG is formulated as maximization of sum of covariance and almost the same constraint condition as PLS-ROG. multiset PLS-ROG loading is defined as the weighted correlation coefficient and could identify statistically significant compounds.

□ MELT: Metric learning for comparing genomic data with triplet network
>> https://academic.oup.com/bib/advance-article-abstract/doi/10.1093/bib/bbac345/6679451
MEtric Learning with Triplet network (MELT), which learns a nonlinear mapping from original space to the embedding space in order to keep similar data closer and dissimilar data far apart.
MELT is a weakly supervised and data-driven comparison framework that offers more adaptive and accurate dissimilarity learned in the absence of the label information when the supervised methods are not applicable.

□ genomicSimulation: fast R functions for stochastic simulation of breeding programs
>> https://academic.oup.com/g3journal/advance-article/doi/10.1093/g3journal/jkac216/6687129
genomicSimulation works as a scripting tool, with functions for performing targeted crosses, random crosses, doubled haploids and selfing. genomicSimulation’s inbuilt genotypic value calculator uses an additive model of marker effects.
Every genotype loaded or produced in genomicSimulation is allocated to a group. Mixing and separating groups allows for significant flexibility in regards to simulating multi-generational breeding pools, or having several interacting streams in the breeding program.

□ Var I Decrypt: a novel and user-friendly tool to explore and prioritize variants in whole-exome sequencing data
>> https://www.biorxiv.org/content/10.1101/2022.09.02.506346v1
Var | Decrypt offers a wide range of gene and variant filtering possibilities, clustering and enrichment tools, providing an efficient way to derive patient-specific functional information and to prioritize gene variants for functional analyses.
Var | Decrypt imports the output results from the Exome-seq pipeline and provides many built-in enrichment analyses options. Var | Decrypt contains different disease ontology, gene ontology, and Reactome/Kegg pathway enrichment tab.

□ MacSyFinder v2: Improved modelling and search engine to identify molecular systems in genomes
>> https://www.biorxiv.org/content/10.1101/2022.09.02.506364v1
MacSyFinder version 2 (v2) was improved and rationalized to facilitate future maintainability. The novel v2 search engine explores the space of possible solutions more thoroughly. It provides optimal solutions with an explicit scoring system favouring complete but concise systems.
The systems are now searched one by one: the identified components are filtered by type of system and assembled in clusters if relevant. Using a system-by-system approach prevents the spurious elimination of relevant candidate systems.

□ MVsim is a toolset for quantifying and designing multivalent interactions
>> https://www.nature.com/articles/s41467-022-32496-6
MVsim, an interactive toolset with a simple graphical user interface (GUI) for the design, prediction, multidimensional parameter exploration, and quantification of multivalent binding phenomena.
MVsim accurately simulates both monospecific multivalent interactions (i.e., a single repeated ligand domain on one binding partner and a single repeated target domain on the other) and multispecific multivalent interactions.

□ Bridging The Evolving Semantics: A Data Driven Approach to Knowledge Discovery In Biomedicine
>> https://www.biorxiv.org/content/10.1101/2022.09.05.506661v1
Dynamic MeSH Embeddings: MeSH embeddings is a powerful diachronic tool, which is capable of capturing the semantic evolution. In the B-Med framework, MeSH embeddings with an augmented notion of time component to captures the evolutionary properties of medical concepts.
In the dynamic embedding space, the semantic change of a MeSH term can be easily mod- eled as the location shift of this term. Hence, MeSH terms are projected into the vector space based on their medical properties and gradually drift over time as they evolve.

□ muSignAl: An algorithm to search for multiple omic signatures with similar predictive performance
>> https://analyticalsciencejournals.onlinelibrary.wiley.com/doi/10.1002/pmic.202200252
muSignAl (multiple signature algorithm) selects multiple signatures with similar predictive performance while systematically bypassing the requirement of exploring all the combinations of features.
muSignAl is applicable in various bioinformatics driven explorations, such as understanding the relationship between multiple biological feature sets and phenotypes, and development of biomarker panels while providing the opportunity of optimising their development cost.

□ Multi-agent Feature Selection for Integrative Multi-omics Analysis
>> https://ieeexplore.ieee.org/document/9871758/
MAgentOmics extends the ant colony optimization algorithm to multi-omics data, which iteratively builds candidate solutions and evaluates them.
Moreover, a new fitness function is introduced to assess the candidate feature subsets without using prediction target such as survival time of patients.

□ ScanExitronLR: characterization and quantification of exitron splicing events in long-read RNA-seq data
>> https://academic.oup.com/bioinformatics/advance-article-abstract/doi/10.1093/bioinformatics/btac626/6696711
ScanExitronLR, an application for the characterization and quantification of exitron splicing events in long-reads. From a BAM alignment file, reference genome and reference gene annotation, ScanExitronLR outputs exitron events at the individual transcript level.
Outputs of ScanExitronLR can be used in downstream analyses of differential exitron splicing. In addition, ScanExitronLR optionally reports exitron annotations such as truncation or frameshift type, nonsense-mediated decay status, and Pfam domain interruptions.
※コメント投稿者のブログIDはブログ作成者のみに通知されます