
"non-ergodic."

□ Strelka2: Fast and accurate variant calling for clinical sequencing applications:
>> https://www.biorxiv.org/content/biorxiv/early/2017/09/23/192872.full.pdf
In germline and somatic variant probability model, supplemented by a final empirical variant scoring, motivated in part by machine learning. Strelka2 demonstrated an average runtime advantage of 3.2x over TNhaplotyper, which itself is over 10x faster than the MuTect2.

□ Predictive-State Decoders: Encoding the Future into Recurrent Networks:
>> https://arxiv.org/pdf/1709.08520.pdf
Predictive-State methods do not parameterize a specific representation of the internal state but use certain assumptions to construct a mathematical structure in terms of the observations to find a globally optimal representation.

□ Extracting Evidence Fragments for Distant Supervision of Molecular Interactions using INTACT database:
>> https://www.biorxiv.org/content/biorxiv/early/2017/09/23/192856.full.pdf
an OWL-based implementation of the existing BioC formulation, extended the SciDT pipeline system to export linked data conforming. and applied an open-source event extraction for signaling pathway events (REACH) to develop a baseline for detailed semantic extraction.
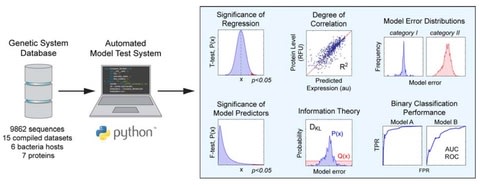
□ An automated model test system for systematic development and improvement of gene expression models:
>> https://www.biorxiv.org/content/biorxiv/early/2017/09/25/193367.full.pdf
leveraged the compiled genetic system database, confirmed mechanistic hypotheses, and identified sources of model error to develop and validate a new version of the RBS Calculator free energy model (v2.1) with a significant improvement in accuracy.
□ WHOLE GENOME BISULPHITE SEQUENCING USING THE ILLUMINA HISEQ X SYSTEM:
>> https://www.biorxiv.org/content/biorxiv/early/2017/09/24/193193.full.pdf
The X-WGBS results interrogate at least one CG in the majority of these loci at 5 or 10X coverage, whereas RRBS represents only a small proportion of these loci, with the Infinium HumanMethylation450 microarray the SeqCap Epi CpGiant system representing similar proportions, with the expanded Infinium MethylationEPIC microarray representing a higher proportion of ATAC-seq peaks.
□ EpgntxEinstein:
Completely open protocol, data and software, credit to @masako_suzuki for inventing BS-tagging idea, great analytics Will Liao #epigenetics
□ Interphase Human Chromosome Exhibits Out of Equilibrium Glassy Dynamics:
>> https://www.biorxiv.org/content/biorxiv/early/2017/09/24/193375.full.pdf
the glass-like landscape might also be beneficial in chromosome functions because only a certain minimum needs to be accessed to carry out a specific function, which will minimize large scale structural fluctuations.
□ bjoerngruening:
200+ packages migrated to #condaforge; 700+ packages build for #rstats 3.4; all available in #bioconda and #biocontainers now!
□ Graphtyper enables population-scale genotyping using pangenome graphs:
>> http://www.nature.com/ng/journal/vaop/ncurrent/full/ng.3964.html
Graphtyper genotyped 89.4 million sequence variants in the whole genomes of 28,075 Icelanders using less than 100,000 CPU days, Graphtyper realigns short-read sequence data to a pangenome, a variation-aware graph structure that encodes sequence variation within a population by representing possible haplotypes as graph paths.
□ Mash Screen: what's in my sequencing run?
>> https://genomeinformatics.github.io/mash-screen/
The new screen operation is compatible with the existing RefSeq sketch DB, or a custom DB can be created for any collection of sequences. A set of sequencing reads can then be streamed against this database requiring just a few minutes per thread per gigabase of reads:
□ OWallerman:
Trying out guppy -> minimap2 -> miniasm (-s 400) for draft assemblies. 7.5G basecalled in 12h, assembly ~20 min., 1.3Mb contig n50.

□ HiDRA: Building on Sharpr-MPRA+STARR-Seq+ATAC-Seq to dissect millions of human regulatory regions in one experiment
>> https://www.biorxiv.org/content/early/2017/09/27/193136
construct a HiDRA library w/ 9.7 million total unique mapping fragments, of which 4 mln had a frequency greater than 0.1 reads per million. More than 99% of fragments
had lengths between 169 nt and 477nt (median: 337nt), with the fragment length distribution showing two peaks spaced by ~147nt, corresponding to the length of DNA wrapped around each nucleosome.

□ Fast and Accurate Genomic Analyses using Genome Graphs:
>> https://www.biorxiv.org/content/biorxiv/early/2017/09/27/194530.full.pdf
a graph genome implementation that enables read alignment across 2,800 diploid genomes encompassing 12.6 million SNPs & 4.0 million indels. graph genome aligner and variant calling pipeline consume around 5.5 and 2 hours per high coverage whole-genome-sequenced sample, this graph genome implementation is able to overcome the practical accuracy limitation of linear reference genomes.
□ ESPRIT-Forest: Subquadratic-time parallel sequence clustering of massive amplicon seq data:
>> http://journals.plos.org/ploscompbiol/article?id=10.1371/journal.pcbi.1005518
a pseudo-metric based partitioning (PBP) enables fast searching of the closest pairs by making only a small number of distance comparisons. A PBP tree is an ordered, equal-depth tree that partitions a sequence space with a series of hyper-spheres.

□ Fractal stock markets: International evidence of dynamical (in)efficiency based on the Hölder pointwise regularity.
>> http://aip.scitation.org/doi/figure/10.1063/1.4987150
Empirical evidence based on the local Gaussian behavior of financial time series along with the good rate of convergence (O(δ−12log−1N) of Hˆkδ,q,N,K(t) suggest to choose values of δ in the range 20–30 datapoints.
□ Exploration of the Boolean-model space of a developmental switch:
>> http://journals.plos.org/ploscompbiol/article?id=10.1371/journal.pcbi.1005744
Genetic algorithms are known to be efficient at exploring high-dimensional spaces, such as the space of all Boolean models. Genetic programming has the added benefit of being able to generate Boolean equations directly, which makes it easier to target models involving simpler, more plausible regulatory interactions.
□ U of T Engineering spinoff Deep Genomics raises US$13 million to fund expansion:
>> http://news.engineering.utoronto.ca/u-t-engineering-spinoff-deep-genomics-raises-us13-million-fund-expansion/

□ Reconstruction of developmental landscapes by optimal-transport analysis sheds light on cellular reprogramming.
>> https://www.biorxiv.org/content/biorxiv/early/2017/09/27/191056.full.pdf
The diffusion pseudo-time method orders cells using an appropriately weighted distance in diffusion component space: This rescaling is derived by considering a random walk according to a transition matrix T that specifies the probability of transitioning from any single cell to another in an infinitesimal amount of time.
□ Constructing an autonomous system with infinitely many chaotic attractors:
>> http://aip.scitation.org/doi/10.1063/1.4986356
a Lorenz-type system and a Rössler-type system with infinitely many chaotic attractors are constructed with bifurcation analysis. {(x1,x2,x3):|x1|≤C/R1,|x2|≤C/R2}; (iv) ẋ =Φ(ϕ1(x1),ϕ2(x2),ϕ3(x3)) with attractors “almost everywhere.”

□ Mixed model association for biobank-scale data sets:
>> https://www.biorxiv.org/content/biorxiv/early/2017/09/27/194944.full.pdf
Conditioning on BOLT-LMM’s polygenic predictions—which attain accuracy (r2BOLT-LMM) approaching SNP-heritability (hg2) for some traits—achieves effective sample sizes as high as ∼700K.
□ Enter the matrix: Interpreting unsupervised learning with matrix decomposition to discover hidden knowledge in high-dimensional omics data
>> https://www.biorxiv.org/content/biorxiv/early/2017/10/02/196915.1.full.pdf
“inner dimension” corresponds to the number of CBPs learned and is often called the “rank” or “dimensionality” of the input data matrix. In linear NMF, the factors are additive and many biological pathways can be combined in a linear fashion to form a molecular phenotype.

□ Using neural networks for reducing the dimensions of single-cell RNA-Seq data:
>> https://academic.oup.com/nar/article/doi/10.1093/nar/gkx681/4056711/Using-neural-networks-for-reducing-the-dimensions
The models using stochastic gradient descent with Nesterov accelerated gradient with a learning rate of 0.1, decay 10^−6, momentum 0.9. The learning rate for epoch K is the original learning rate multiplied by 1/(1+decay*K).

□ Learning causal networks with latent variables from multivariate information in genomic data:
>> http://journals.plos.org/ploscompbiol/article?id=10.1371/journal.pcbi.1005662
MIIC (Multivariate Information Inductive Causation) learns a large class of causal or non-causal graphical models from purely observational data, while including the effects of unobserved latent variables, commonly found in many datasets.
□ Introducing prescribed biases in out of equilibrium Markov models:
>> https://www.biorxiv.org/content/biorxiv/early/2017/10/04/198697.full.pdf
a maximum path entropy based framework to update prior non-equilibrium Markov models to state and dynamical trajectory-based constraints. the framework using a biochemical model network of growth factors-based signaling, state space topology has a significant impact on the stationary distribution.
□ Apple's CoreML tools are now on GitHub & open to contributions! Run models from Keras/sklearn/XGBoost/LibSVM on iOS
>> https://github.com/apple/coremltools
Construct a builder that builds a neural network classifier with a 299x299x3
dimensional input and 1000 dimensional output
Set the neural network spec inputs to be 3 dimensional vector data1 and 4 dimensional vector data2.

□ B-LORE: Bayesian multiple logistic regression for meta-analyses of GWAS:
>> https://www.biorxiv.org/content/biorxiv/early/2017/10/05/198911.full.pdf
Meta-analysis using summary statistics. B-LORE optimizes the hyperparameters θ by maximizing the marginal likelihood combining. a novel quasi-Laplace approximation which makes the Bayesian treatment of the multiple logistic regression case analytically tractable.
□ VASC: dimension reduction and visualization of single cell RNA sequencing data by deep variational autoencoder:
>> https://www.biorxiv.org/content/biorxiv/early/2017/10/06/199315.full.pdf
a multi-dimensional Gaussian distribution Q(z|X) of latent variables z given the expression values X, whose mean and variance parameters could be generated by the encoder network. Loss(X, Y )=binary entropy(X, Y )+KL( Q(z|X)||P (z) )

□ Ontology-based similarity calculations with an improved annotation model (SEBASTIAN KÖHLER)
>> https://www.biorxiv.org/content/biorxiv/early/2017/10/06/199554.full.pdf
The hypothesis is that standard semantic similarity measures can be improved by extending the standard “Merged” annotation model that can be extracted from the accompanying full text description in OMIM and the meta-information available in the GO annotation data set.
□ Attractor dynamics in networks with learning rules inferred from in vivo data (Ulises Pereira and Nicolas Brunel)
>> https://www.biorxiv.org/content/biorxiv/early/2017/10/06/199521.full.pdf
this rule produces a storage capacity that is close to the maximal capacity, in the space of unsupervised Hebbian learning rules with sigmoidal dependence on both pre and post-synaptic firing rates.

□ Bratwurst: Deciphering programs of transcriptional regulation by combined deconvolution of multiple omics layers:
>> https://www.biorxiv.org/content/biorxiv/early/2017/10/08/199547.full.pdf
Bratwurst determines the optimal factorization rank by iterating over different factorization ranks, evaluating quality metrics for any one of them and selecting the rank which complies best with the quality metrics.
□ A parametric interpretation of Bayesian Nonparametric Inference from Gene Genealogies: linking ecological, population genetics and evolutionary processes.
>> https://www.biorxiv.org/content/biorxiv/early/2017/10/09/200469.full.pdf
In the context of Bayesian statistics, the Nonparametrics labeling refers to placing priors to a potentially infinite number of parameters. the difficulty imposed by having to specify an infinite dimensional object like a function of time as a prior is nicely overcome with GPs.

□ Bistability, non-ergodicity, and inhibition in pairwise maximum-entropy models:
>> http://journals.plos.org/ploscompbiol/article?id=10.1371/journal.pcbi.1005762
Boltzmann-learning based on asynchronous Glauber dynamics, used to find the Lagrange multipliers and distributions of these models, becomes practically non-ergodic. The distribution is therefore difficult or impossible to find. This problem is known in the statistical mechanics of finite-size systems. This non-ergodicity can go undetected.

□ Time-Consistent Reconciliation Maps and Forbidden Time Travel:
>> https://www.biorxiv.org/content/biorxiv/early/2017/10/10/201053.full.pdf
an O(|V(T)|log(|V(S)|))-time algorithm to decide whether a time-consistent reconciliation map exists.
ReconMap::write_nexus_recon(std::ostream& os) {
os << "BEGIN RECONCILIATION;" << std::endl;
os << "[SIGMA represents the species a gene resides in]" << std::endl;
os << "[Syntax is: gene_leaf_name species_leaf_name]" << std::endl;
os << "\tSIGMA" << std::endl;

□ Evolutionary rescue over a fitness landscape:
>> https://www.biorxiv.org/content/biorxiv/early/2017/10/13/135780.full.pdf
The volume in phenotype space occupied by the subset is roughly constant across stress & determines the ER (evolutionary rescue) probability, The cost of complexity is more important in this regime, as it strongly determines the volume of the resistance subspace.
□ The language of music: Common neural codes for structured sequences in music and natural language:
>> https://www.biorxiv.org/content/biorxiv/early/2017/10/12/202382.full.pdf
□ How to cite the GTEx project
>> https://liorpachter.wordpress.com/2017/10/12/how-to-cite-the-gtex-project/

□ Robust Gaussian Processes in Stan: Bayesian Inference of Gaussian Processes Hyperparameters:
>> https://betanalpha.github.io/assets/case_studies/gp_part3/part3.html
the only way to maintain similar performance for higher-dimensional covariate spaces is to add exponentially for information into this model.
matrix[N, N] cov = cov_exp_quad(x, alpha, rho)
+ diag_matrix(rep_vector(square(sigma), N));
matrix[N, N] L_cov = cholesky_decompose(cov);
※コメント投稿者のブログIDはブログ作成者のみに通知されます