

□ Ibex: Variational autoencoder for single-cell BCR sequencing.
>> https://www.biorxiv.org/content/10.1101/2022.11.09.515787v1
Ibex vectorizes the amino acid sequence of the complementarity-determining region 3 (cdr3) of the immunoglobulin heavy and light chains, allowing for unbiased dimensional reduction of B cells using their BCR repertoire.
Ibex was trained on 600,000 human cdr3 sequences of the respective Ig chain, w/ a 128-64-30-64-128 neuron structure. Ibex enables the reduction of cell-level quantifications to clonotype-level quantifications using minimal Euclidean distance across principal component dimensions.
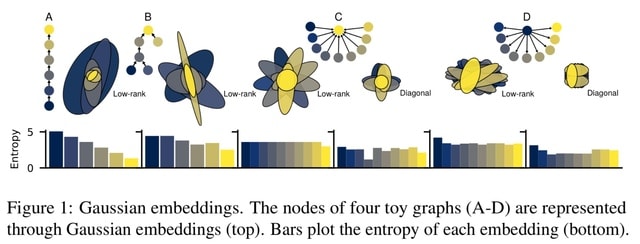
□ gGN: learning to represent graph nodes as low-rank Gaussian distributions
>> https://www.biorxiv.org/content/10.1101/2022.11.15.516704v1
gGN, a novel representation for graph nodes that uses Gaus- sian distributions to map nodes not only to point vectors (means) but also to ellipsoidal regions (covariances).
Besides the Kullback-Leibler divergence is well suited for capturing asymmetric local structures, the reverse KL additionally leads to Gaussian distributions whose entropies properly preserve the information contents of nodes.
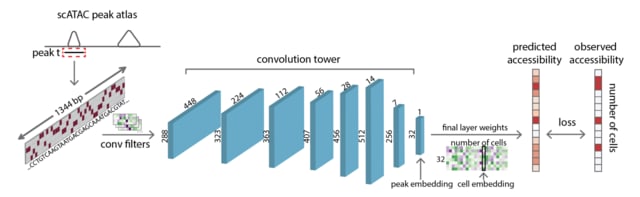
□ scBasset: sequence-based modeling of single-cell ATAC-seq using convolutional neural networks
>> https://www.nature.com/articles/s41592-022-01562-8
Extending the Basset architecture to predict single cell chromatin accessibility from sequences, using a bottleneck layer to learn low-dimensional representations of the single cells.
scBasset is based on a deep convolutional neural network to predict single cell chromatin accessibility from the DNA sequence underlying peak calls. scBasset takes as input a 1344 bp DNA sequence from each peak’s center and one-hot encodes it as a 4×1344 matrix.
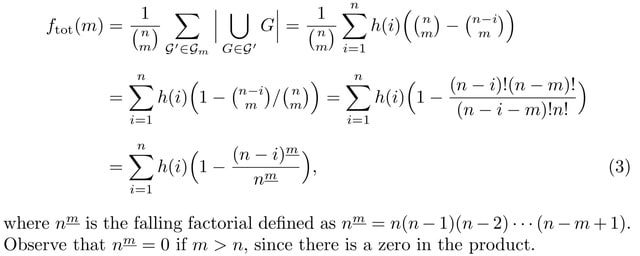
□ Revisiting pangenome openness with k-mers
>> https://www.biorxiv.org/content/10.1101/2022.11.15.516472v1
Defining a genome as a set of abstract items, which is entirely agnostic of the two approaches (gene-based, sequence-based). Genes are a viable option for items, but also other possibilities are feasible, e.g., genome sequence substrings of fixed length k .
Genome assemblies must be computed when using a gene-based approach, while k-mers can be extracted directly from sequencing reads. The pangenome is defined as the union of these sets. The estimation of the pangenome openness requires the computation of the pangenome growth.

□ Snapper: a high-sensitive algorithm to detect methylation motifs based on Oxford Nanopore reads
>> https://www.biorxiv.org/content/10.1101/2022.11.15.516621v1
Snapper, a new highly-sensitive approach to extract methylation motif sequences based on a greedy motif selection algorithm. Snapper has shown higher enrichment sensitivity compared with the MEME tool coupled with Tombo or Nanodisco instruments.
Snapper uses a k-mer approach, with k chosen to be 11 in order to cover all 6-mers that cover one particular base under the assumption that, in general, approximately 6 bases are located in the nanopore simultaneously.
All the extracted k-mers are merged by a greedy algorithm which generates the minimal set of potential modification motifs which can explain the most part of selected 11-mers, under the assumption that all selected 11-mers contain at least one modified base.

□ SCOOTR: Jointly aligning cells and genomic features of single-cell multi-omics data with co-optimal transport
>> https://www.biorxiv.org/content/10.1101/2022.11.09.515883v1
SCOOTR provides quality alignments for unsupervised cell- level and feature-level integration of datasets with sparse feature correspondences. It returns the feature-feature coupling matrix for the user to investigate the correspondence probabilities.
SCOOTR uses the cell-cell coupling matrix to align the samples in the same space via barycentric projection or co-embedding via tSNE. Its unique joint alignment formulation provides the ability to perform the weak supervision at both sample and feature level.
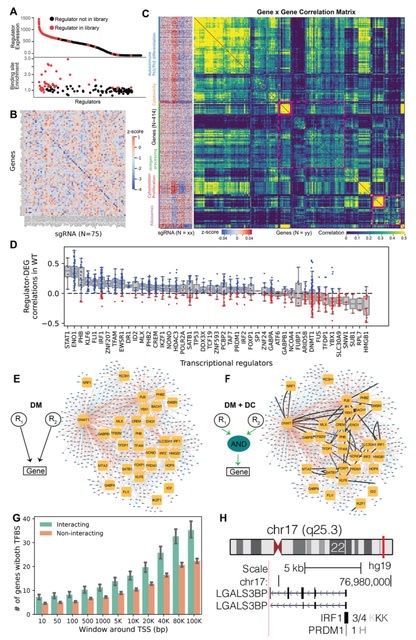
□ memento: Generalized differential expression analysis of single-cell RNA-seq with method of moments estimation and efficient resampling
>> https://www.biorxiv.org/content/10.1101/2022.11.09.515836v1
memento, an end-to-end method that implements a hierarchical model for estimating the mean, residual variance, and gene correlation from scRNA-seq data and a statistical framework for hypothesis testing of differences in these parameters between groups of cells.
memento models scRNA-seq using a novel multivariate hypergeometric sampling process while making no assumptions about the true distributional form of gene expression within cells.
memento implements an innovative bootstrapping strategy for efficient statistical comparisons of the estimated parameters between groups of cells that can also incorporate biological and technical replicates.
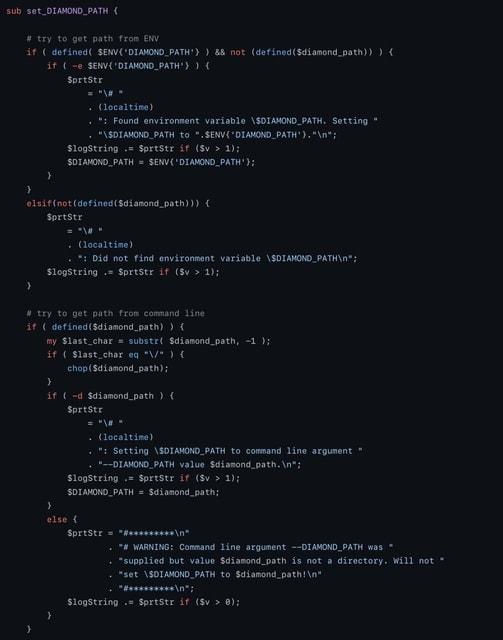
□ GALBA: a pipeline for fully automated prediction of protein coding gene structures with AUGUSTUS
>> https://github.com/Gaius-Augustus/GALBA
GALBA code was derived from BRAKER, a fully automated pipeline for predicting genes in the genomes of novel species with RNA-Seq data and a large-scale database of protein sequences with GeneMark-ES/ET/EP/ETP and AUGUSTUS.
GALBA is a fully automated gene pipeline that trains AUGUSTUS, for a novel species and subsequently predicts genes with AUGUSTUS. GALBA uses the protein sequences of one closely related species to generate a training gene set for AUGUSTUS with either miniprot, or GenomeThreader.
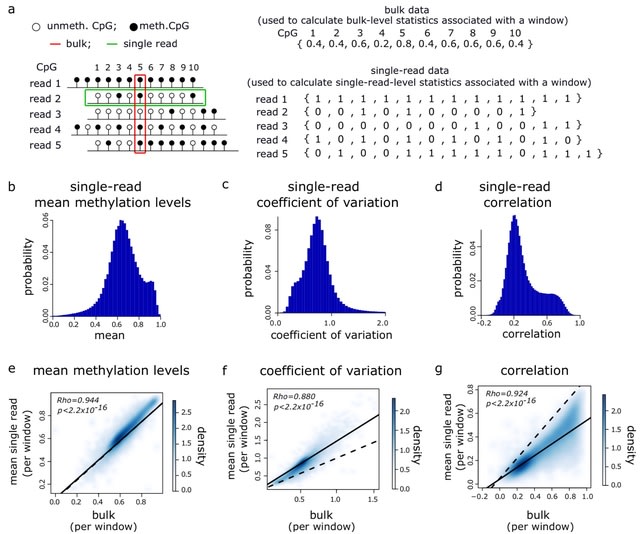
□ Genome-wide single-molecule analysis of long-read DNA methylation reveals heterogeneous patterns at heterochromatin
>> https://www.biorxiv.org/content/10.1101/2022.11.15.516549v1
Conducting a genome-wide analysis of single-molecule DNA methy- lation patterns in long reads derived from Nanopore sequencing in order to understand the nature of large-scale intra-molecular DNA methylation heterogeneity in the human genome.
Like mean methylation levels, the mean single-read and bulk measurements of the coefficient of variation and correlation were significantly correlated. Oscillatory DNA patterns are observed in single reads with a high heterogeneity.

□ singleCellHaystack: A universal differential expression prediction tool for single-cell and spatial genomics data
>> https://www.biorxiv.org/content/10.1101/2022.11.13.516355v1
singleCellHaystack, a method that predicts DEGs based on the distribution of cells in which they are active within an input space. Previously, singleCellHaystack was not able to handle sparse matrices, limiting its applicability to the ever-increasing dataset sizes.
singleCellHaystack now accepts continuous features that can be RNA or protein expression, chromatin accessibility or module scores from single cell, spatial and even bulk genomics data, and it can handle 1D trajectories, 2-3D spatial coordinates, as well as higher-dimensional latent spaces.
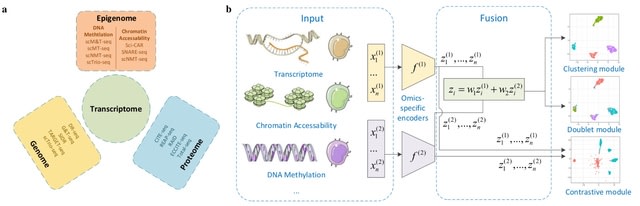
□ MoClust: Clustering single-cell multi-omics data
>> https://academic.oup.com/bioinformatics/advance-article/doi/10.1093/bioinformatics/btac736/6831092
MoClust uses a selective automatic doublet detection module that can identify and filter out doublets is introduced in the pretraining stage to improve data quality. Omics-specific autoencoders are introduced to characterize the multi-omics data.
A contrastive learning way of distribution alignment is adopted to adaptively fuse omics representations into an omics-invariant representation.
This novel way of alignment boosts the compactness and separableness of clusters, while accurately weighting the contribution of each omics to the clustering object.
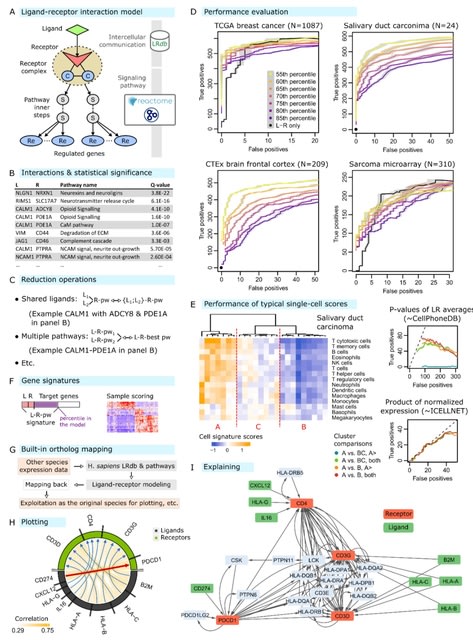
□ BulkSignalR: Inferring ligand-receptor cellular networks from bulk and spatial transcriptomic datasets
>> https://www.biorxiv.org/content/10.1101/2022.11.17.516911v1
BulkSignalR exploits reference databases of known ligand-receptor interactions (LRIs), gene or protein interactions, and biological pathways to assess the significance of correlation patterns between a ligand, its putative receptor, and the targets of the downstream pathway.
There is an obvious parallel with enrichment analysis of gene sets versus the analysis of individual differentially expressed genes. This infrastructure allows network visualization for relating LRIs to target genes.

□ trans-PCO: Trans-eQTL mapping in gene sets identifies network effects of genetic variants
>> https://www.biorxiv.org/content/10.1101/2022.11.11.516189v1
trans-PCO, a flexible approach that uses the PCA-based omnibus test combine multiple PCs and improve power to detect trans-eQTLs. trans-PCO filters sequencing reads and genes based on mappability across different regions of the genome to avoid false positives due to mis-mapping.
trans-PCO uses a novel multivariate association test to detect genetic variants with effects on multiple genes in predefined sets and captures genetic effects on multiple PCs. By default, trans-PCO defines sets of genes based on co-expression gene modules as identified by WGCNA.

□ Accurate Detection of Incomplete Lineage Sorting via Supervised Machine Learning
>> https://www.biorxiv.org/content/10.1101/2022.11.09.515828v1
A model to infer important properties of a particular internal branch of the species tree via genome-scale summary statistics extracted from individual alignments and inferred gene trees.
The model predicts the presence/absence of discordance, estimate the probability of discordance, and infer the correct species tree topology. A variety of SML algorithms can distinguish biological discordance from gene tree inference error across a wide range of parameter space.

□ STREAK: A Supervised Cell Surface Receptor Abundance Estimation Strategy for Single Cell RNA-Sequencing Data using Feature Selection and Thresholded Gene Set Scoring
>> https://www.biorxiv.org/content/10.1101/2022.11.10.516050v1
STREAK estimates receptor abundance levels by leveraging associations between gene expression and protein abundance to enable receptor gene set scoring of scRNA-seq target data.
STREAK generates weighted receptor gene sets using joint scRNA-seq/CITE-seq training data with the gene set for each receptor containing the genes whose normalized and reconstructed scRNA-seq expression values are most strongly correlated with CITE-seq receptor protein abundance.
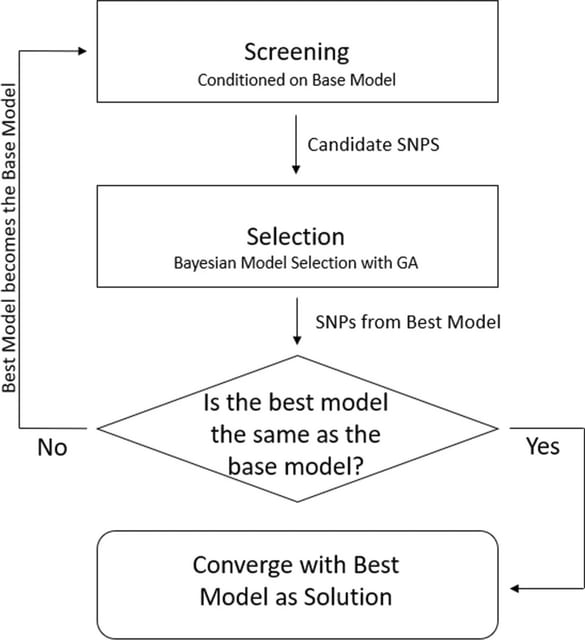
□ BICOSS: Bayesian iterative conditional stochastic search for GWAS
>> https://bmcbioinformatics.biomedcentral.com/articles/10.1186/s12859-022-05030-0
BICOSS is an iterative procedure where each iteration is comprised of two steps: a screening and a model selection step. BICOSS is initialized with a base model fitted as a linear mixed model with no SNPs in the model.
Then the screening step fits as many models as there are SNPs, each model containing one SNP and regressed against the residuals of the base model. The screening step identifies a set of candidate SNPs using Bayesian FDR control applied to the posterior probabilities of the SNPs.
BICOSS performs Bayesian model selection where the possible models contain any combination of the base model and SNPs from the candidate set. If the model space is too large to perform complete enumeration, a genetic algorithm is used to perform stochastic model search.
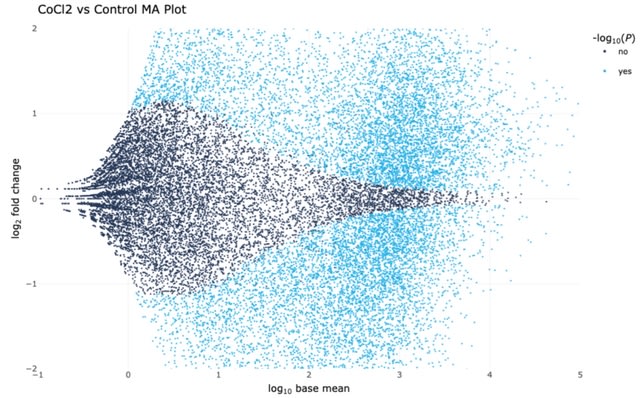
□ LVBRS: Latch Verified Bulk-RNA Seq toolkit: a cloud-based suite of workflows for bulk RNA-seq quality control, analysis, and functional enrichment
>> https://www.biorxiv.org/content/10.1101/2022.11.10.516016v1
The LVBRS toolkit supports three databases—Gene Ontology, KEGG Pathway, and Molecular Signatures database—capturing diverse functional information. The LVBRS workflow also conducts differential intron excision analysis.
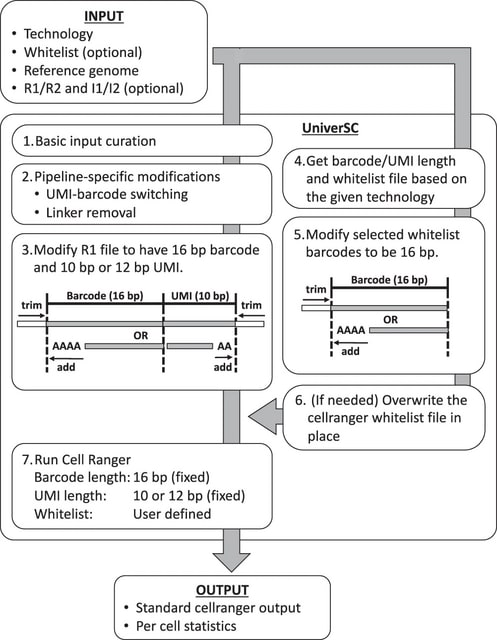
□ UniverSC: A flexible cross-platform single-cell data processing pipeline
>> https://www.nature.com/articles/s41467-022-34681-z
UniverSC; a shell utility that operates as a wrapper for Cell Ranger. Cell Ranger has been optimised further by adapting open-source techniques, such as the third-party EmptyDrops algorithm for cell calling or filtering, which does not assume thresholds specific for the Chromium platform.
In principle, UniverSC can be run on any droplet-based or well-based technology. UniverSC provides a file with summary statistics, including the mapping rate, assigned/mapped read counts and UMI counts for each barcode, and averages for the filtered cells.

□ VarSCAT: A computational tool for sequence context annotations of genomic variants
>> https://www.biorxiv.org/content/10.1101/2022.11.11.516085v1
Breakpoint ambiguities may cause potential problems for downstream annotations, such as the Human Genome Variation Society (HGVS) nomenclature of variants, which recommends a 3’-aligned position but may lead to redundancies of indels.
VarSCAT, a variant sequence context annotation tool with various functions for studying the sequence contexts around variants and annotating variants with breakpoint ambiguities, flanking sequences, HGVS nomenclature, distances b/n adjacent variants, and tandem repeat regions.

□ AGouTI - flexible Annotation of Genomic and Transcriptomic Intervals
>> https://www.biorxiv.org/content/10.1101/2022.11.13.516331v1
AGouTI – a universal tool for flexible annotation of any genomic or transcriptomic coordinates using known genomic features deposited in different publicly available data- bases in the form of GTF or GFF files.
AGouTI is designed to provide a flexible selection of genomic features overlapping or adjacent to annotated intervals, can be used on custom column- based text files obtained from different data analysis pipelines, and supports operations on transcriptomic coordinate systems.

□ SEGCOND predicts putative transcriptional condensate-associated genomic regions by integrating multi-omics data
>> https://academic.oup.com/bioinformatics/advance-article/doi/10.1093/bioinformatics/btac742/6832039
SEGCOND, a computational framework aiming to highlight genomic regions involved in the formation of transcriptional condensates. SEGCOND is flexible in combining multiple genomic datasets related to enhancer activity and chromatin accessibility, to perform a genome segmentation.
SEGCOND uses this segmentation for the detection of highly transcriptionally active regions of the genome. And through the integration of Hi-C data, it identifies regions of PTC as genomic domains where multiple enhancer elements coalesce in three-dimensional space.

□ lmerSeq: an R package for analyzing transformed RNA-Seq data with linear mixed effects models
>> https://bmcbioinformatics.biomedcentral.com/articles/10.1186/s12859-022-05019-9
lmerSeq can fit models incl. multiple random effects, implement the correlation structures, constructing contrasts and simultaneous tests of multiple regression coefficients, and utilize multiple methods for calculating denominator degrees of freedom for F- and t-tests.
In models with a misspecified random effects structure (incl. a random intercept only), FDR is increased relative to the models with correctly specified random effects for both lmerSeq and DREAM.
Since DREAM and lmerSeq are capable of fitting similar LMMs, it appears that the driving force behind the differential behavior b/n lmerSeq and DREAM is the choice of transformation, with lmerSeq utilizing DESeq2’s VST and DREAM using their own modification of VOOM.
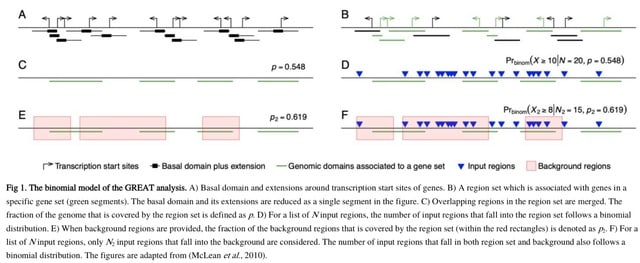
□ rGREAT: an R/Bioconductor package for functional enrichment on genomic regions
>> https://academic.oup.com/bioinformatics/advance-article/doi/10.1093/bioinformatics/btac745/6832038
GREAT is a widely used tool for functional enrichment on genomic regions. However, as an online tool, it has limitations of outdated annotation data, small numbers of supported organisms and gene set collections, and not being extensible for users.
rGREAT integrates a large number of gene set collections for many organisms. First it serves as a client to directly interact with the GREAT web service in the R environment. It automatically submits the imput regions to GREAT and retrieves results from there.

□ Modeling and cleaning RNA-seq data significantly improve detection of differentially expressed genes
>> https://bmcbioinformatics.biomedcentral.com/articles/10.1186/s12859-022-05023-z
A program RNAdeNoise for cleaning RNA-seq data, which improves the detection of differentially expressed genes and specifically genes with a low to moderate absolute level of transcription.
This cleaning method has a single variable parameter – the filtering strength, which is a removed quantile of the exponentially distributed counts. It computes the dependency between this parameter and the number of detected DEGs.
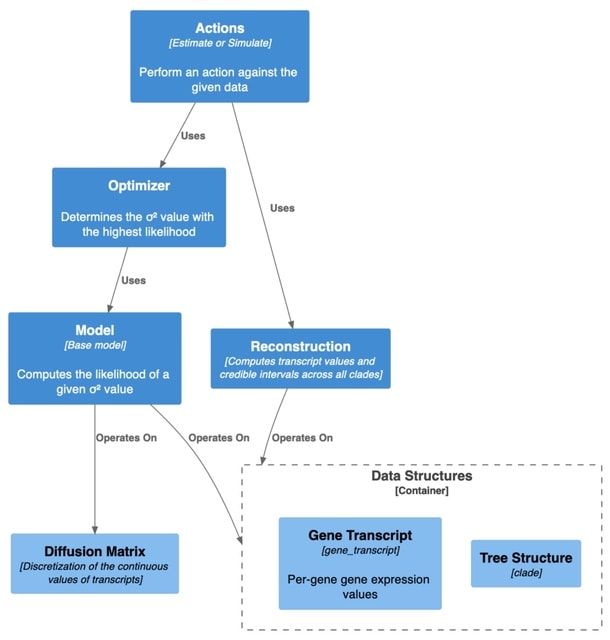
□ CAGEE: computational analysis of gene expression evolution
>> https://www.biorxiv.org/content/10.1101/2022.11.18.517074v1
CAGEE analyzes changes in global or sample- or clade-specific gene expression taking into account phylogenetic history, and provides a statistical foundation for evolutionary inferences. CAGEE uses Brownian motion to model GE changes across a user-specified phylogenetic tree.
The reconstructed distribution of counts and their inferred evolutionary rate σ2 generated under this model provides a basis for assessing the significance of the observed differences among taxa.

□ USAT: a bioinformatic toolkit to facilitate interpretation and comparative visualization of tandem repeat sequences
>> https://bmcbioinformatics.biomedcentral.com/articles/10.1186/s12859-022-05021-1
A Universal STR Allele Toolkit (USAT) for TR haplotype analysis, which takes TR haplotype output from existing tools to perform allele size conversion, sequence comparison of haplotypes, figure plotting, comparison for allele distribution, and interactive visualization.
USAT takes the TR sequences in a plain text file and TR loci configure information in a BED formatted plain text file as input to calculate the length of each haplotype sequence in nucleotide base pairs (bps) and the number of repeats.
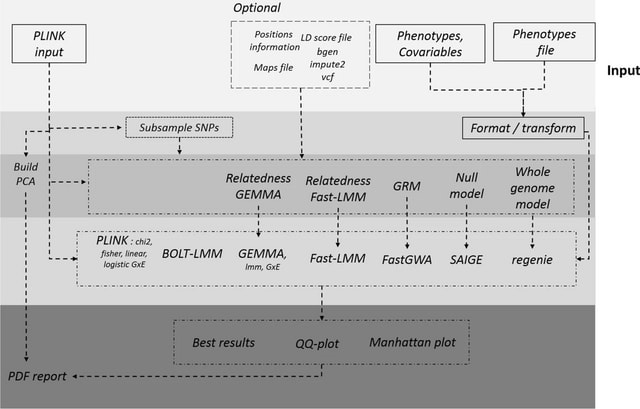
□ H3AGWAS: a portable workflow for genome wide association studies
>> https://bmcbioinformatics.biomedcentral.com/articles/10.1186/s12859-022-05034-w
H3Agwas is a simple human GWAS analysis workflow for data quality control and basic association testing developed by H3ABioNet. It is an extension of the witsGWAS pipeline for human genome-wide association studies built at the Sydney Brenner Institute for Molecular Bioscience.
H3Agwas uses Nextflow for workflow managment and has been dockerised to facilitate portability. And split into several independent sub-workflows mapping to separate phases. Independent workflows allow to execute parts that are only relevant to them at those different phases.
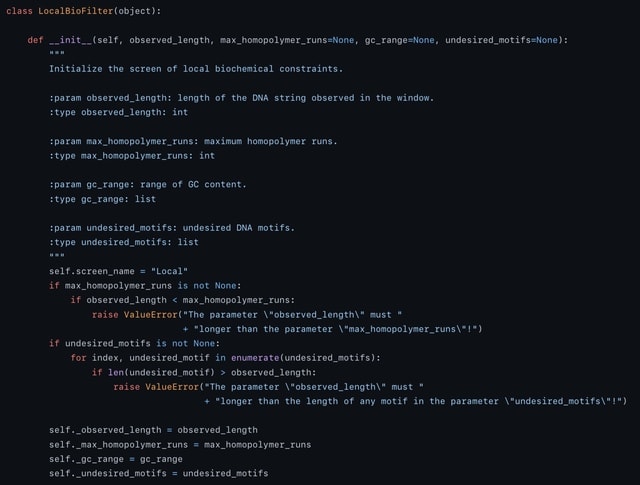
□ DNA-LC: Multiple errors correction for position-limited DNA sequences with GC balance and no homopolymer for DNA-based data storage
>> https://academic.oup.com/bib/advance-article-abstract/doi/10.1093/bib/bbac484/6835379
DNA-LC, a novel coding schema which converts binary sequences into DNA base sequences that satisfy both the GC balance and run-length constraints.
The DNA-LC coding mode enables detect and correct multiple errors with a higher error correction capability than the other methods targeting single error correction within a single strand.

□ SyBLaRS: A web service for laying out, rendering and mining biological maps in SBGN, SBML and more
>> https://journals.plos.org/ploscompbiol/article?id=10.1371/journal.pcbi.1010635
SyBLaRS (Systems Biology Layout and Rendering Service) accommodates a number of novel methods as well as widely known and used ones on automatic layout of pathways, calculating graph-theoretic properties in pathways and mining pathways for subgraphs of interest.
SyBLaRS exposes the shortest paths algorithm of Dijkstra. It finds one of many potentially available shortest paths from a single dedicated node to another one, whereas algorithms such as Paths-between and Paths-from-to find all such paths b/n a group of source and target nodes.
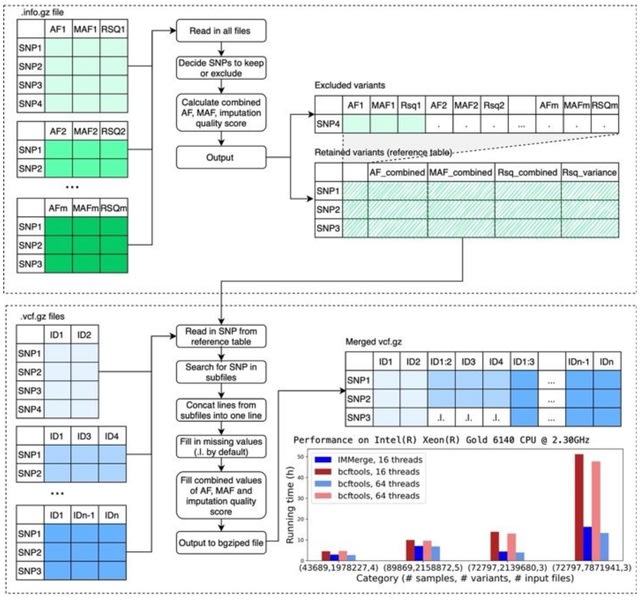
□ IMMerge: Merging imputation data at scale
>> https://academic.oup.com/bioinformatics/advance-article/doi/10.1093/bioinformatics/btac750/6839927
IMMerge, a Python-based tool that takes advantage of multiprocessing to reduce running time. For the first time in a publicly available tool, imputation quality scores are correctly combined with Fisher’s z transformation.
IMMerge is designed to: (i) rapidly combine sets of imputed data through multiprocessing to accelerate the decompression of inputs, compression of outputs, and merging of files; (ii) preserve variants not shared by all subsets;
(iii) combine imputation quality statistics and detect significant variation in SNP-level imputation quality; (iv) manage samples duplicated across subsets; (v) output relevant combined summary information incl. allele frequency (AF) and minor AF as weighed means, maximum, and minimum values.

□ Improving dynamic predictions with ensembles of observable models
>> https://academic.oup.com/bioinformatics/advance-article/doi/10.1093/bioinformatics/btac755/6842325
The procedure starts by analysing structural identifiability and observability; if the analysis of these properties reveals deficiencies in the model structure that prevent it from inferring key parameters or state variables, the method then searches for a suitable reparameterization.
Once a fully identifiable and observable model structure is obtained, it is calibrated using a global optimization procedure, that yields not only an optimal parameter vector but also an ensemble of other possible solutions.
This method exploits the information in these additional vectors to build an ensemble of models with different parameterizations.
The hybrid global optimization approach used here performs a balanced sampling of the parameter space; as a consequence, the median of the ensemble is a good approximation of the median of the model given parameter uncertainty.
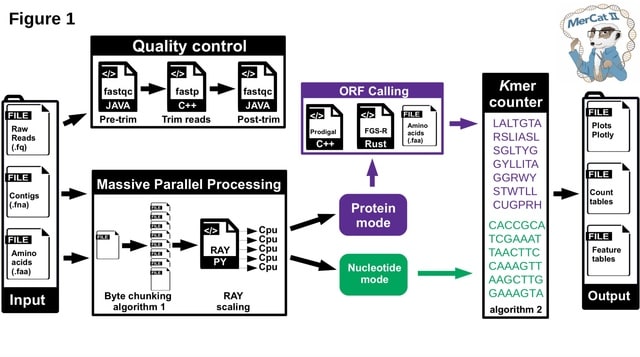
□ MerCat2: a versatile k-mer counter and diversity estimator for database-independent property analysis obtained from omics data
>> https://www.biorxiv.org/content/10.1101/2022.11.22.517562v1
MerCat2 (“Mer - Catenate2") allows for direct analysis of data properties in a database-independent manner that initializes all data, which other profilers and assembly- based methods cannot perform.
For massive parallel processing (MPP) and scaling, MerCat2 uses a byte chunking algorithm to split files for MPP and utilization in RAY, a massive open-source parallel computing framework.
□ k2v: A Containerized Workflow for Creating VCF Files from Kintelligence Targeted Sequencing Data
>> https://www.biorxiv.org/content/10.1101/2022.11.21.517402v1
k2v, a containerized workflow for creating standard specification-compliant variant call format (VCF) files from the custom output data produced by the Kintelligence Universal Analysis Software.
k2v enables the rapid conversion of Kintelligence variant data. VCF files produced with k2v enable the use of many pre-existing, widely used, community-developed tools for manipulating and analyzing genetic data in the standard VCF format.
※コメント投稿者のブログIDはブログ作成者のみに通知されます