

□ UniAligner: a parameter-free framework for fast sequence alignment
>>
UniAligner—the parameter-free sequence alignment algorithm with sequence-dependent alignment scoring that automatically changes for any pair of compared sequences.
UniAligner prioritizes matches of rare substrings that are more likely to be relevant to the evolutionary relationship b/n two sequences. UniAligner estimates the mutation rates in human centromeres, and quantify the extremely high rate of large duplications and deletions.

□ LINGER: Continuous lifelong learning for modeling of gene regulation from single cell multiome data by leveraging atlas-scale external data
>> https://www.biorxiv.org/content/10.1101/2023.08.01.551575v1
LINGER (LIfelong neural Network for GEne Regulation), a novel deep learning-based method to infer GRNs from single-cell multiome data with paired gene expression and chromatin accessibility data from the same cell.
LINGER incorporates both atlas-scale external bulk data across diverse cellular contexts and the knowledge of TF motif matching to cis-regulatory elements as a manifold regularization to address the challenge of limited data and extensive parameter space in GRN inference.

□ Unsupervised removal of systematic background noise from droplet-based single-cell experiments using CellBender
>> https://www.nature.com/articles/s41592-023-01943-7
A deep generative model based on the phenomenology of noise generation in droplet-based assays. The proposed model accurately distinguishes cell-containing droplets from cell-free droplets, learns the background noise profile and provides noise-free quantification.
CellBender operates near the theoretically optimal denoising limit. Highlighting enhanced concordance b/n droplet-based single-cell data and established gene expression patterns, while the learned background noise profile provides evidence of degraded or uncaptured cell types.

□ Transmorph: a unifying computational framework for modular single-cell RNA-seq data integration
>> https://academic.oup.com/nargab/article/5/3/lqad069/7223068
Transmorph, a novel and ambitious data integration framework. It features a modular way to create data integration algorithms using basic algorithmic and structural blocks, as well as analysis tools including embedding quality assessment and plotting functions.
Transmorph provides annotated, high quality and ready-to-use datasets to benchmark algorithm. In this framework, data integration models can be assembled by combining four classes of algorithms: trans-formations, matchings, embeddings, and evaluators.

□ Dictys: dynamic gene regulatory network dissects developmental continuum with single-cell multiomics
>> https://www.nature.com/articles/s41592-023-01971-3
Dictys infers and analyzes (pseudo-)time-resolved dynamic GRNs to dissect gene regulation variations in continuous processes like development with a single snapshot experiment.
Along the provided trajectory, Dictys first defines a moving window to subset cells into overlapping small (~1000 cells) subpopulations, and then reconstructs a static GRN for each subpopulation and consequently the dynamic GRN with Gaussian kernel smoothing.
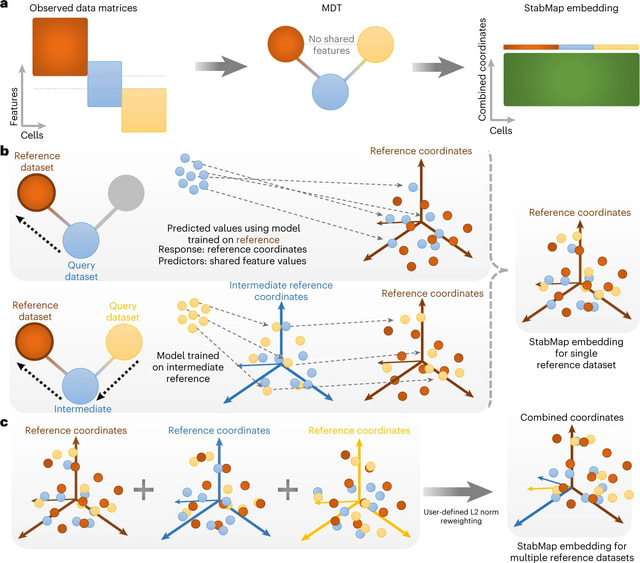
□ Stabilized mosaic single-cell data integration using unshared features
>> https://www.nature.com/articles/s41587-023-01766-z
StabMap projects all cells onto supervised or unsupervised reference coordinates using all available features regardless of overlap with other datasets, instead relying on traversal along the mosaic data topology (MDT).
Since StabMap results in a low-dimensional embedding common to all datasets, it can be combined with further downstream horizontal data integration tasks, such as mutual nearest neighbors, Seurat and scMerge, to adjust for any remaining batch effects.
StabMap requires only that the MDT be a connected network, and there be a way to draw a path from each node to every other node. StabMap performs multi-hop mosaic data integration, that is, integrating data where the intersection of features measured for all datasets is empty.

□ ggcoverage: an R package to visualize and annotate genome coverage for various NGS data
>> https://bmcbioinformatics.biomedcentral.com/articles/10.1186/s12859-023-05438-2
ggcoverage, an R package dedicated to visualizing and annotating genome coverage of multi-groups and multi-omics. It allows users to visualize genome coverage with flexible input file formats, and annotate the genome coverage with various annotations.
ggcoverage provides reliable and efficient ways to perform data preprocessing, including parallel reads normalization per bin, consensus peaks generation from replicates and track data loading by extracting subsets.

□ VACmap: an accurate long-read aligner for unraveling complex structural variations
>> https://www.biorxiv.org/content/10.1101/2023.08.03.551566v1
VACmap incorporates a novel variant-aware chaining algorithm, which effectively identifies the globally optimal non-linear alignment for each long read.
VACmap connects anchors and assigns weights. Next, VACmap identifies the optimal variant-aware chaining by searching for the longest path. Finally, VACmap removes the variation edges within the optimal variant-aware chain and extracts collinear alignments.

□ TEQUILA-seq: a versatile and low-cost method for targeted long-read RNA sequencing
>> https://www.nature.com/articles/s41467-023-40083-6
TEQUILA-seq (Transcript Enrichment and Quantification Utilizing Isothermally Linear-Amplified probes in conjunction with long-read sequencing), versatile, easy-to-implement, and low-cost approach for generating large quantities of biotinylated capture oligos for any gene panel.
TEQUILA-seq probes are amplified from ssDNA oligo templates in a single pool using nickase-triggered SDA with universal primers and biotin-dUTPs. Full-length cDNAs are synthesized from poly(A)+ RNAs by reverse transcription. TEQUILA probes are then hybridized to cDNAs.
The setup cost of TEQUILA probe synthesis for the same 6000-probe panel is $3,086 ($1,820 for oligo pool), and this pool can potentially be used to synthesize TEQUILA probes for 6,250 to 25,000 reactions, at $0.31–$0.53/reaction.

□ scMD: cell type deconvolution using single-cell DNA methylation references
>> https://www.biorxiv.org/content/10.1101/2023.08.03.551733v1
scMD (single cell Methylation Deconvolution), a cellular deconvolution framework to reliably estimate cell type fractions from tissue-level DNAm data. scMD is successful in capturing useful signals from the original sparse scDNAm data.
scMD employs a statistical approach to aggregate scDNAm data at the cell cluster level, identify cell-type marker DNAm sites, and create a precise cell-type signature matrix that surpasses state-of-the-art sorted-cell or RNA-derived references.

□ PUMATAC: Systematic benchmarking of single-cell ATAC-sequencing protocols
>> https://www.nature.com/articles/s41587-023-01881-x
PUMATAC, a universal preprocessing pipeline. PUMATAC takes scATAC-seq data and applies a set of uniform preprocessing steps, incl. cell barcode error correction, adapter trimming, reference genome alignment and mapping quality filtering.
PUMATAC then records aligned chromatin fragments in the ubiquitous bed-like ‘fragments file’ format, a tab-separated text file providing the start and end positions of each fragment and its corresponding cell barcode.

□ CoCoNat: a novel method based on deep-learning for coiled-coil prediction
>> https://academic.oup.com/bioinformatics/advance-article/doi/10.1093/bioinformatics/btad495/7237258
CoCoNat, a novel method for predicting coiled-coil helix boundaries, residue-level register annotation and oligomerization state.
CoCoNat encodes sequences with the combination of two state-of-the-art protein language models and implements a three-step deep learning procedure concatenated with a Grammatical-Restrained Hidden Conditional Random Field (GRHCRF) for CCD identification and refinement.

□ memerna: Sparse RNA Folding Including Coaxial Stacking
>> https://www.biorxiv.org/content/10.1101/2023.08.04.551958v1
memerna implements the Zuker-Stiegler algorithm with coaxial stacking, with some assumptions about the energy model. It assumes a Turner 04-like energy model, and is not as flexible as packages like RNAstructure or ViennaRNA as to what energy models can be specified.
This formulation considers branches separately when finding the optimal CD configuration, whereas normally coaxial stacks are optimized by trying every possible split point explicitly, in a loop.
A split point is an index which has a branch both to the left and right of it. For a particular split point, the existing algorithm looks at the branch to the left and the right and compute the free energy contribution of those two branches forming a coaxial stack.

□ ProtoCell4P: An Explainable Prototype-based Neural Network for Patient Classification Using Single-cell RNA-seq
>> https://academic.oup.com/bioinformatics/advance-article/doi/10.1093/bioinformatics/btad493/7237257
ProtoCell4P leverages the knowledge of scRNA-seq data to predict individual phenotypes, where the prototypes are representatives of cells. ProtoCell4P consists of a cell embedding module which encodes the cells into the latent space.
ProtoCell4P learns a group of cell prototypes that can be representatives of cell subpopulations, and a classification module that adaptively evaluates the relevance of prototypes and combines the prototype-related information from all cells to make the final prediction.

□ SHEPHARD: a modular and extensible software architecture for analyzing and annotating large protein datasets
>> https://academic.oup.com/bioinformatics/advance-article/doi/10.1093/bioinformatics/btad488/7237256
SHEPHARD is a Python-based general-purpose hierar- chical framework that facilitates reproducible, reliable, and high-throughput analysis of complex numerical and symbolic protein annotations at proteome-wide scales.
SHEPHARD stores data in an object-oriented hierarchical format where the base container is a Proteome. Proteomes contain one or more Proteins, and each Protein can be annotated with Domains, Sites, or Tracks.

□ Niche-DE: Niche differential gene expression analysis in spatial transcriptomics data identifies context-dependent cell-cell interactions
>> https://www.biorxiv.org/content/10.1101/2023.01.03.522646v1
Niche-DE identifies cell-type specific niche-associated genes, defined as genes whose expression within a specific cell type is significantly up / down regulated, in the context of specific spatial niches. Niche-DE is robust to technical issues such as over-dispersion and spot swapping.
Niche-DE can be applied to low-resolution spot- and ROI-based spatial transcriptomics data as well as data that is single-cell or subcellular in resolution. niche-DE reveals the ligand-receptor signaling mechanisms that underlie niche-differential gene expression patterns.

□ SEUSS: Interface-guided phenotyping of coding variants in the transcription factor RUNX1
>> https://www.biorxiv.org/content/10.1101/2023.08.03.551876v1
SEUSS (ScalablE fUnctional Screening by Sequencing), a Perturb-seq like approach, to generate and assay mutations at physical interfaces of the RUNX1 Runt domain.
SEUSS vector is designed to improve signal in their screens to eliminate issues with barcode shuffling they positioned the variant and variant barcode in direct proximity.

□ Multi-representation DeepInsight: an improvement on tabular data analysis
>> https://www.biorxiv.org/content/10.1101/2023.08.02.551620v1
Multi-representation DeepInsight (abbreviated as MRep-DeepInsight), an innovative extension of the DeepInsight method, specifically designed to enhance the analysis of tabular data.
By generating multiple representations of samples using diverse feature extraction techniques, MRep-DeepInsight aims to capture a broader range of features and reveal deeper insights.
In the transformation phase, tabular data is converted to image samples using a multi-representation strategy. Multi-representation samples are processed by a CNN for training. A novel test sample is analyzed to one of the defined classes.

□ Compound models and Pearson residuals for normalization of single-cell RNA-seq data without UMIs
>> https://www.biorxiv.org/content/10.1101/2023.08.02.551637v1
Compound Pearson residuals, a new theoretically motivated method for normalization of non-UMI data that explicitlv accounts for the amplification noise. This vields a generative model for read counts that reproduces characteristic patterns of non-UMI data.
The compound NB model with amplification modeled by broken zeta yields a generative model reproducing zero-inflation and overdispersion patterns similar to what is observed in read count data.
Compared to the ZINB model with three per-gene parameters, this model contains only one free per-gene parameter, and the varying zero-inflation and overdispersion naturally emerge as a function of a gene's mean expression.
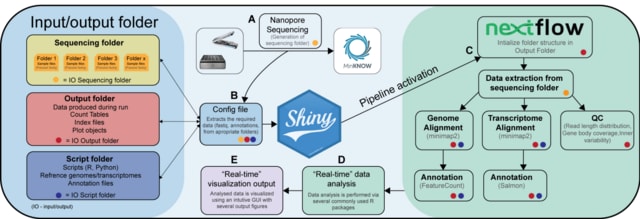
□ NanopoReaTA: a user-friendly tool for nanopore-seq real-time transcriptional analysis
>> https://academic.oup.com/bioinformatics/advance-article/doi/10.1093/bioinformatics/btad492/7238212
NanopoReaTA focuses on the analysis of (direct) cDNA and RNA-sequencing (cDNA, DRS) reads and guides you through the different steps up to final visualizations of results from i.e. differential expression or gene body coverage.
NanopoReaTA can be run in real-time right after starting a run via MinKNOW, the sequencing application of ONT. NanopoReaTA provides visual snapshots of a sequencing run in progress, thus enabling interactive sequencing and rapid decision-making.

□ HEARTSVG: a fast and accurate method for spatially variable gene identification in large-scale spatial transcriptomic data
>> https://www.biorxiv.org/content/10.1101/2023.08.06.552154v1
HEARTS G, a distribution-free, test-based method for fast and accurately identifying spatially variable genes in large-scale spatial transcriptomic data. HEARTSVG identifies non-SVGs by testing the serial autocorrelations in the marginal expressions across global space.
By excluding non-SVGs, the remaining genes are considered as SVGs. As a test-based method without assuming underlying spatial patterns, HEARTSVG detects SVGs with arbitrary spatial expression shapes and is suitable for diverse types of large-scale ST data.

□ LAST-seq: single-cell RNA sequencing by direct amplification of single-stranded RNA without prior reverse transcription and second-strand synthesis
>> https://genomebiology.biomedcentral.com/articles/10.1186/s13059-023-03025-5
LAST-seq directly and linearly amplifies the original ssRNA molecules in single cells in a linear fashion, achieving a high single-molecule capture efficiency and a low level of technical noise compared to existing scRNA-seq methods.
LAST-seq characterizes cell-to-cell variation in human cells, quantify gene expression noise of individual genes, and derive transcriptional bursting kinetics for further investigation in the context of 3D chromatin organization.

□ Revealing Structural Information about Complex Systems from Minimal Data
>> https://pubs.aip.org/aip/sci/article/2023/29/291112/2903004/Revealing-Structural-Information-about-Complex
Although for high-dimensional systems, dimension inference from a single variable may not yet be practical due to the limited precision of recorded data, it may serve as a starting point for studying dimension inference from a very small number of variables or even just one.
In principle, this technique can reconstruct arbitrarily high state space dimensions using only data from a single variable. In practice, the technique is limited due to unavoidable measurement errors, noise, and inaccuracies in processing the recorded dynamics.

□ iCpG-Pos: An Accurate Computational Approach for Identification of CpG Sites Using Positional Features on Single-Cell Whole Genome Sequence Data
>> https://academic.oup.com/bioinformatics/advance-article/doi/10.1093/bioinformatics/btad474/7239862
iCpG-Pos uses positional features extracted from the single-cell whole-genome sequencing data. iCpG-Pos presents two techniques which are CatBoost-based and stacking-based.
All the classification algorithms used in this study are optimized using the OPTUNA framework. This work can be used to uncover the direct linkage between methylation and diseases by comprehending the complicated biological mechanisms that enable methylation.

□ HiBrowser: an interactive and dynamic browser for synchronous Hi-C data visualization
>> https://academic.oup.com/bib/advance-article/doi/10.1093/bib/bbad283/7237943
The advantages of HiBrowser are flexible multi-omics navigation, novel multidimensional synchronization comparisons and dynamic interaction system.
In particular, HiBrowser first provides an out of the box web service and allows flexible and dynamic reconstruction of custom annotation tracks on demand during running.

□ Maast: genotyping thousands of microbial strains efficiently
>> https://genomebiology.biomedcentral.com/articles/10.1186/s13059-023-03030-8
Maast (Microbial agile accurate SNP Typer) for accurate genotyping of orders of magnitude more microbial strains than other state-of-the-art methods. The key innovation is an algorithm to pick a minimal set of maximally diverse genomes.
Maast uses a hybrid method combining whole-genome alignment and optimized k-mer exact match for genotyping SNPs in either assembled genomes or unassembled whole-genome sequencing (WGS) libraries.

□ The CUT&RUN suspect list of problematic regions of the genome
>> https://genomebiology.biomedcentral.com/articles/10.1186/s13059-023-03027-3
Using publicly available C&R negative control data, they have compiled suspect lists (for the hg38 and T2T human genomes, and mm10 and mm39 mouse genomes) containing artifact regions that are consistently and spuriously enriched across experiments.
Some artifact regions are unique to C&R, indicating the need for technique-specific suspect lists and implying a partially biological origin to the signal enrichment, while the reduction in number of regions for the improved genomic assemblies implies a computational nature.
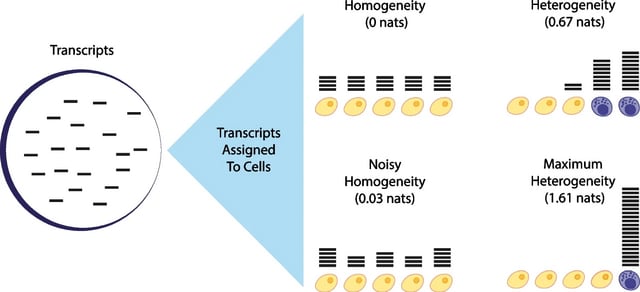
□ An information-theoretic approach to single cell sequencing analysis
>> https://bmcbioinformatics.biomedcentral.com/articles/10.1186/s12859-023-05424-8
Demonstrating a natural relation between this notion of heterogeneity and that of cell type, decomposing heterogeneity into that component attributable to differential expression between cell types (inter-cluster heterogeneity) and that remaining (intra-cluster heterogeneity).
A definition of gene heterogeneity leads to a biologically meaningful notion of cell type, as groups of cells that are statistically equivalent with respect to their patterns of gene expression.
A method for the automatic unsupervised clustering of cells from sc-Seq data is developed. A measure of heterogeneity, and its decomposition into inter- and intra-cluster, is non-parametric, intrinsic, unbiased, and requires no additional assumptions about expression patterns.

□ spatiAlign: An Unsupervised Contrastive Learning Model for Data Integration of Spatially Resolved Transcriptomics
>> https://www.biorxiv.org/content/10.1101/2023.08.08.552402v1
spatiAlign, an unsupervised contrastive learning model that employs the expression of all measured genes and spatial location of cells, to integrate multiple tissue sections.
spatiAlign enables the joint downstream analysis of multiple datasets not only in low-dimensional embeddings, but also in the reconstructed full expression space.

□ IBAS: Interaction-bridged association studies discovering novel genes underlying complex traits
>> https://www.biorxiv.org/content/10.1101/2023.08.08.552376v1
IBAS, Interaction-Bridged Association Study, a new model using statistical learning techniques to extract representations of interaction patterns in transcriptome data, which act as a mediator for the next genotype-phenotype association test.
IBAS is more robust to noise than similar mediation-based protocols replying on single-genes, i.e., TWAS. By applying IBAS to real genotype-phenotype and expression data, they reported additional genes underlying complex traits as well as their biological annotations.

□ MetaCerberus: distributed highly parallelized scalable HMM-based implementation for robust functional annotation across the tree of life
>> https://www.biorxiv.org/content/10.1101/2023.08.10.552700v1
MetaCerberus transforms raw shotgun metaomics sequencing data into knowledge. It is a start to finish python code for versatile analysis of the Functional Ontology Assignments for Metagenomes (FOAM), KEGG, CAZy, VOG/pVOG, PHROG, and COG databases via Hidden Markov Models.
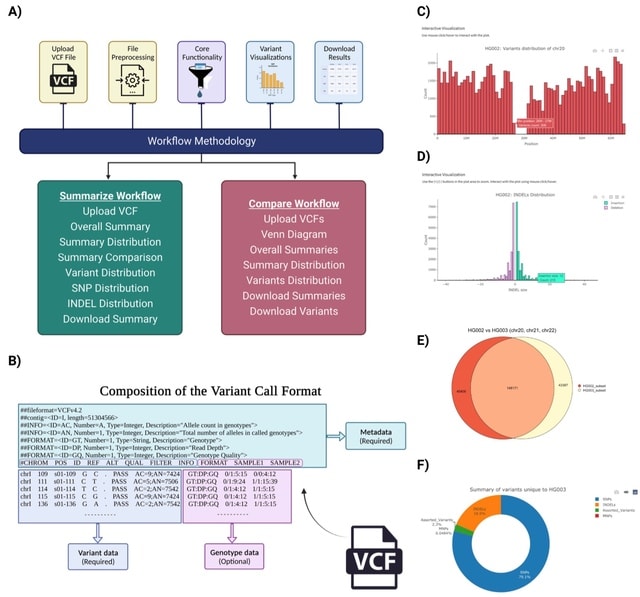
□ SCI-VCF: A cross-platform application to summarise, compare and design interactive visualisations of the variant call format
>> https://www.biorxiv.org/content/10.1101/2023.08.09.552664v1
SCI-VCF, a comprehensive toolkit with an intuitive Graphical User Interface (GUI) that lets users summarise, interpret, and compare genomic variants from VCF files. It also equips users to design interactive visualisations of the VCF in numerous ways.
SCI-VCF is platform-agnostic and works seamlessly across any operating system. SCI-VCF provides a well-founded framework that simplifies the core components of VCF analyses, thus increasing the approachability of genomics to novices.

□ Ulisse: Cross-talk quantification in molecular networks with application to pathway-pathway and cell-cell interactions.
>> https://www.biorxiv.org/content/10.1101/2023.08.10.552776v1
Ulisse, a method to (1) quantify cross-talks between gene sets, with application to pathways and intercellular cross-talks; (2) investigate the role of the genes involved in cross-talks, via functional relevance analysis, in terms of regulated processes/cell types.
Ulisse and PathNet use two different empirical nulls, which is probably the main factor that determined different results on the same input. Ulisse focuses on interactions between pairs of gene sets and the empirical null models the expected interactions between the two sets.

□ MELISSA: Semi-Supervised Embedding for Protein Function Prediction Across Multiple Networks
>> https://www.biorxiv.org/content/10.1101/2023.08.09.552672v1
MELISSA (MultiNetwork Embedding with Label Integrated Semi- Supervised Augmentation) which incorporates functional labels in the embedding stage.
The function labels induce sets of “must link" and “cannot link" constraints which guide a further semi-supervised dimension reduction to yield an embedding that captures both the network topology and the information contained in the annotations.
※コメント投稿者のブログIDはブログ作成者のみに通知されます