

□ Möbius Randomness Law for Frobenius Traces:
>> https://arxiv.org/abs/1909.00969
M ̈obius randomness law, and Sarnak’s conjecture which roughly asserts that for any bounded sequence s(n) of complex numbers.
Recall the following bound of exponential sums with M ̈obius function, which depends on the Diophantine properties of the exponent α.

□ Patterns
>> https://www.cell.com/patterns/home
Data are boundless. Big ideas deserve a big audience. Insights fuel action.
Patterns is domain agnostic and offers breadth and depth across the spectrum of research disciplines.
□ On Arithmetical Structures on Complete Graphs
>> https://arxiv.org/pdf/1909.02022.pdf
an arithmetical structure may be regarded as a generalization of the Laplacian matrix, which encodes many important properties of a graph.
An arithmetical structure on a finite, connected graph is an assignment of positive integers to the vertices.
At each vertex, the integer there is a divisor of the sum of the integers at adjacent vertices (counted with multiplicity if the graph is not simple), and the integers used have no nontrivial common factor.

□ MLTrigNer: Multiple-level biomedical event trigger recognition with transfer learning
>> https://bmcbioinformatics.biomedcentral.com/articles/10.1186/s12859-019-3030-z
a source domain with plentiful annotations of biomolecular event triggers (the BioNLP corpus) is used to improve performance on a target domain of multiple-level event triggers with fewer available annotations (the MLEE corpus).
Multiple-Level Trigger recogNizer (MLTrigNer), which is built based on the generalized cross-domain transfer learning BiLSTM-CRF model.

□ Unsupervised Clusterless Decoding using a Switching Poisson Hidden Markov Model
>> https://www.biorxiv.org/content/biorxiv/early/2019/09/08/760470.full.pdf
The iterative Baum–Welch parameter estimation procedure for the clusterless HMM. It makes use of the forward-backward algorithm to compute the posterior marginals of all hidden state variables given a sequence of observations.
a new computational model that is an extension of the standard (unsupervised) switching Poisson hidden Markov model, to a clusterless approximation in which is observed only a d-dimensional mark.
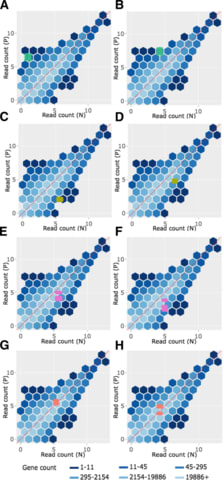
□ bigPint: Visualization methods for differential expression analysis
>> https://bmcbioinformatics.biomedcentral.com/articles/10.1186/s12859-019-2968-1
Methods for visualizing large multivariate datasets using static and interactive scatterplot matrices, parallel coordinate plots, volcano plots, and litre plots. Includes examples for visualizing RNA-sequencing datasets and differentially expressed genes.
these graphical tools allow researchers to quickly explore DEG lists that come out of models and ensure which ones make sense from an additional and arguably more intuitive vantage point.

□ KDML: a machine-learning framework for inference of multi-scale gene functions from genetic perturbation screens
>> https://www.biorxiv.org/content/biorxiv/early/2019/09/08/761106.full.pdf
Knowledge-Driven Machine Learning (KDML) systematically predicts multiple functions for a given gene based on the similarity of its perturbation phenotype to those with known function.
KDML is a novel framework for automated knowledge discovery from large-scale HT-GPS. KDML is designed to account for pleiotropic and partially penetrant phenotypic effects of gene loss.
□ Design and assembly of DNA molecules using multi-objective optimisation
>> https://www.biorxiv.org/content/biorxiv/early/2019/09/08/761320.full.pdf
DNA engineering as a multi-objective optimization problem aiming at finding the best tradeoff between design requirements and manufadturing constraints.
a new open-source algorithm for DNA engineering, called Multi-Objective Optimisation algorithm for DNA Design and Assembly (MOODA), provides near optimal constructs and scales linearly with design complexity.
□ hypeR: An R Package for Geneset Enrichment Workflows
>> https://academic.oup.com/bioinformatics/advance-article/doi/10.1093/bioinformatics/btz700/5566242
a comprehensive R package for geneset enrichment workflows that offers multiple enrichment, visualization, and sharing methods in addition to novel features such as hierarchical geneset analysis and built-in markdown reporting.
hypeR employs multiple types of enrichment analyses (e.g. hypergeometric, kstest, gsea). Depending on the type, different kinds of signatures are expected.


□ Quantifying the tradeoff between sequencing depth and cell number in single-cell RNA-seq
>> https://www.biorxiv.org/content/biorxiv/early/2019/09/09/762773.full.pdf
an approach to quantifying the impact of sequencing depth and cell number on the estimation of a multivariate generative model for gene expression that is based on error analysis in the framework of a variational autoencoder.
at shallow depths, the marginal benefit of deeper sequencing per cell significantly outweighs the benefit of increased cell numbers.
The kallisto bustools workflow was used to obtain UMI gene count matrices at different sampled read depths to mimic datasets sequenced at varying depths.
Each of the scVI models were applied to the held-out data, and reconstruction error was calculated to give validation error values for each point in the sampling grid of cell numbers and reads per cell numbers.

□ Estimating information in time-varying signals
>> https://journals.plos.org/ploscompbiol/article?id=10.1371/journal.pcbi.1007290
these single-cell, decoding-based information estimates, rather than the commonly-used tests for significant differences between selected population response statistics, provide a proper and unbiased measure for the performance of biological signaling networks.
In contrast to the frequently-used k-nearest-neighbor estimator, decoding-based estimators robustly extract a large fraction of the available information from high-dimensional trajectories with a realistic number of data samples.

□ PK-DB: PharmacoKinetics DataBase for Individualized and Stratified Computational Modeling
>> https://www.biorxiv.org/content/biorxiv/early/2019/09/09/760884.full.pdf
PK-DB provides curated information on characteristics of studied patient cohorts and subjects; applied interventions; measured pharmacokinetic time-courses; pharmacokinetic parameters.
A special focus lies on meta-data relevant for individualized and stratified computational modeling with methods like physiologically based pharmacokinetic, pharmacokinetic/pharmacodynami, or population pharmacokinetic modeling.

□ Deep Equilibrium Models
>> https://arxiv.org/pdf/1909.01377.pdf
the Deep Equilibrium Model apploach (DEQ), which models temporal data by directly solving for the sequence-level fixed point and optimizing this equilibrium.
DEQ needs only O(1) memory at training time, is agnostic to the choice of the root solver in the forward pass, and is sufficiently versatile to subsume drastically different architectural choices.
Using the Deep Equilibrium Model, training and prediction in these networks require only constant memory, regardless of the effective “depth” of the network.

□ How to Pare a Pair: Topology Control and Pruning in Intertwined Complex Networks
>> https://www.biorxiv.org/content/biorxiv/early/2019/09/09/763649.full.pdf
an advanced version of the discrete Hu-Cai model, coupling two spatial networks in 3D.
The spatial coupling of two flow-adapting networks can control the onset of topological complexity given the system is exposed to short-term flow fluctuations.
The Lyapunov ansatz provides a generally applicable tool in network optimization, and should properly be tested for other boundary conditions or graph geometries which resemble realistic structures.
□ OmicsX: a web server for integrated OMICS analysis
>> https://www.biorxiv.org/content/biorxiv/early/2019/09/09/755918.full.pdf
OmicsX is a user-friendly web server for integration and comparison of different omic datasets with optional sample annotation information.
OmicsX includes modules for gene-wise correlation, sample-wise correlation, subtype clustering, and differential expression.
□ sn-m3C-seq: Single-cell multi-omic profiling of chromatin conformation and DNA methylation
>> https://protocolexchange.researchsquare.com/article/fbc07d40-d794-4cfc-9e7c-294aafbefc10/v1
single-nucleus methyl-3C sequencing to capture chromatin organization and DNA methylation information and robustly separate heterogeneous cell types.
sn-m3C-seq allows generates single cell chromatin conformation and DNA methylation profiles that are of equivalent quality as existing unimodal technologies.
□ Spectrum: Fast density-aware spectral clustering for single and multi-omic data
>> https://academic.oup.com/bioinformatics/advance-article/doi/10.1093/bioinformatics/btz704/5566508
Spectrum uses a tensor product graph data integration and diffusion procedure to reduce noise and reveal underlying structures.
Spectrum contains a new method for finding the optimal number of clusters (K) involving eigenvector distribution analysis, and can automatically find K for both Gaussian and non-Gaussian structures.
□ scBFA: modeling detection patterns to mitigate technical noise in large-scale single-cell genomics data
>> https://genomebiology.biomedcentral.com/articles/10.1186/s13059-019-1806-0
this technical variation in both scRNA-seq and scATAC-seq datasets can be mitigated by analyzing feature detection patterns alone and ignoring feature quantification measurements.
single-cell binary factor analysis (scBFA), leads to better cell type identification and trajectory inference, more accurate recovery of cell type-specific markers, and is much faster to perform compared to several quantification-based methods.
□ PARC: ultrafast and accurate clustering of phenotypic data of millions of single cells
>> https://www.biorxiv.org/content/biorxiv/early/2019/09/11/765628.full.pdf
a highly scalable graph-based clustering algorithm PARC - phenotyping by accelerated refined community-partitioning – for ultralarge-scale, high-dimensional single-cell data of 1 million cells.
PARC consistently outperforms state-of-the-art clustering algorithms without sub-sampling of cells, including Phenograph, FlowSOM, and Flock, in terms of both speed and ability to robustly detect rare cell populations.
□ An Open Source Mesh Generation Platform for Biophysical Modeling Using Realistic Cellular Geometries
>> https://www.biorxiv.org/content/biorxiv/early/2019/09/11/765453.full.pdf
Using GAMer 2 and associated tools PyGAMer and BlendGAMer, biologists can robustly generate computer and algorithm friendly geometric mesh representations informed by structural biology data.
The first step usually requires the generation of a geometric mesh over which the problem can be discretized using techniques such as finite difference, finite volume, finite element, or other methods to build the algebraic system that approximates the PDE.
The numerical approximation to the Partial Differential Equations (PDE) is then produced by solving the resulting linear or nonlinear algebraic equations using an appropriate fast solver.
□ QuartetScores: Quartet-based computations of internode certainty provide robust measures of phylogenetic incongruence
>> https://academic.oup.com/sysbio/advance-article-abstract/doi/10.1093/sysbio/syz058/5556115
three new Internode Certainty (IC) measures based on the frequencies of quartets, which naturally apply to both complete and partial trees.
on complete data sets, both quartet-based and bipartition-based measures yield very similar IC scores; IC scores of quartet-based measures on a given data set with and without missing taxa are more similar than the scores of bipartition-based measures;
and quartet-based measures are more robust to the absence of phylogenetic signal and errors in phylogenetic inference than bipartition-based measures.

□ ELMERI: Fast and Accurate Correction of Optical Mapping Data via Spaced Seeds
>> https://academic.oup.com/bioinformatics/advance-article/doi/10.1093/bioinformatics/btz663/5559485
ELMERI relies on the novel and nontrivial adaption and application of spaced seeds in the context of optical mapping, which allows for spurious and deleted cut sites to be accounted for. ELMERI improves upon the results of state-of-the-art correction methods but in a fraction of the time.
cOMet required 9.9 CPU days to error correct Rmap data generated from the human genome, whereas ELMERI required less than 15 CPU hours and improved the quality of the Rmaps by more than four times compared to cOMet.

□ Generation of Binary Tree-Child phylogenetic networks
>> https://journals.plos.org/ploscompbiol/article?id=10.1371/journal.pcbi.1007347
address the problem of generating all possible binary tree-child (BTC) networks with a given number of leaves in an efficient way via reduction/augmentation operations that extend and generalize analogous operations for phylogenetic trees, and are biologically relevant.
the operations can be employed to extend the evolutive history of a set of sequences, represented by a BTC network, to include a new sequence. And also obtain a recursive formula for a bound on the number of these networks.
□ Raven: Assembler for de novo DNA assembly of long uncorrected reads
>> https://github.com/lbcb-sci/raven
Raven is as an assembler for raw reads generated by third generation sequencing. It first finds overlaps between reads by chaining minimizer hits (submodule Ram which is minimap turned into a library).
Raven creates an assembly graph and simplifies it (code from Rala), and polishes the obtained contigs with partial order alignment (submodule Racon).


□ Optimal design of single-cell RNA sequencing experiments for cell-type-specific eQTL analysis
>> https://www.biorxiv.org/content/biorxiv/early/2019/09/12/766972.full.pdf
a practical guideline for designing ct-eQTL studies which maximizes statistical power.
by aggregating reads across cells within a cell type, it is possible to achieve a high average Pearson R^2 between the low-coverage estimates and the ground truth values of gene expression.

□ GRASP: a Bayesian network structure learning method using adaptive sequential Monte Carlo
>> https://www.biorxiv.org/content/biorxiv/early/2019/09/12/767327.full.pdf
GRowth-based Approach with Staged Pruning (GRASP) is a sequential Monte Carlo (SMC) based three-stage approach.
on categorical variables (nodes) with multinomial distribution, one may extend our approach to other types of variables including Gaussian ones, as long as all the nodes have the same distribution and the local conditional distribution can be estimated.
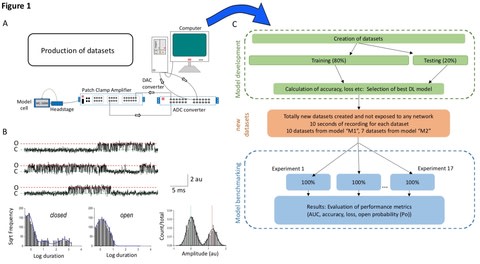
□ Deep-Channel: A Deep Convolution and Recurrent Neural Network for Detection of Single Molecule Events
>> https://www.biorxiv.org/content/biorxiv/early/2019/09/12/767418.full.pdf
a hybrid recurrent convolutional neural network (RCNN) model to idealise ion channel records, with up to 5 ion channel events occurring simultaneously.
an analogue synthetic ion channel record generator system and find that this “Deep-Channel” model, involving LSTM and CNN layers, rapidly and accurately idealises/detects experimentally observed single molecule events.
□ A new local covariance matrix estimation for the classification of gene expression profiles in RNA-Seq data
>> https://www.biorxiv.org/content/biorxiv/early/2019/09/12/766402.full.pdf
a new type of covariance matrix estimate, which is called local covariance matrix, that can be implemented in qtQDA classifier. Integrating this new local covariance matrix into the qtQDA classifier improves the performance of the classifier.
since the local covariance is updated for each new sample observation with a newly proposed method, the classifier, qtQDA, becomes an adaptive algorithm and we call it Local-quantile transformed Quadratic Discriminant Analysis (L-qtQDA).
□ Tree congruence: quantifying similarity between dendrogram topologies
>> https://www.biorxiv.org/content/biorxiv/early/2019/09/12/766840.full.pdf
this article describes and tests two metrics the Clade Retention Index (CRI) and the MASTxCF which is derived from the combined information available from a maximum agreement subtree and a strict consensus.
□ 2D-HELS-AA MS Seq: Direct sequencing of tRNA reveals its different isoforms and multiple dynamic base modifications
>> https://www.biorxiv.org/content/biorxiv/early/2019/09/12/767129.full.pdf
a direct method for sequencing tRNAPhe without cDNA by combining 2- dimensional hydrophobic RNA end-labeling with an anchor-based algorithm in mass spectrometry-based sequencing (2D-HELS-AA MS Seq).
a two-dimensional (2D) LC-MS-based RNA sequencing method was established to produce easily-identifiable mass- retention time (tR) ladders, allowing de novo sequencing of short single-stranded RNAs.
the results of the 2D-HELS-AA MS Seq revealed new isoforms, RNA base modifications and editing as well as their relative abundance in the tRNA that can’t be determined by cDNA-based methods, opening new opportunities in the field of epitranscriptomics.
□ wenda: Weighted elastic net for unsupervised domain adaptation with application to age prediction from DNA methylation data
>> https://academic.oup.com/bioinformatics/article/35/14/i154/5529259
A common additional obstacle in computational biology is scarce data with many more features than samples.
wenda (weighted elastic net for unsupervised domain adaptation) compares the dependency structure between inputs in source and target domain to measure how similar features behave.
□ Creating Artificial Human Genomes Using Generative Models
>> https://www.biorxiv.org/content/biorxiv/early/2019/09/14/769091.full.pdf
A restricted Boltzmann machine, initially called Harmonium is another generative model which is a type of neural network capable of learning probability distributions through input data.
train deep generative adversarial networks (GANs) and restricted Boltzmann machines (RBMs) to learn the high dimensional distributions of real genomic datasets and create artificial genomes (AGs).

□ A Bayesian implementation of the multispecies coalescent model with introgression for comparative genomic analysis
>> https://www.biorxiv.org/content/biorxiv/early/2019/09/14/766741.full.pdf
the multispecies-coalescent-with-introgression (MSci) model, an extension of the multispecies-coalescent (MSC) model to incorporate introgression, in our Bayesian Markov chain Monte Carlo (MCMC) program BPP.
The MSci model accommodates deep coalescence (or incomplete lineage sorting) and introgression and provides a natural framework for inference using genomic sequence data.
□ Complete characterization of the human immune cell transcriptome using accurate full-length cDNA sequencing
>> https://www.biorxiv.org/content/10.1101/761437v1
Rolling Circle to Concatemeric Consensus (R2C2) protocol9 to generate over 10,000,000 full-length cDNA sequences at a median accuracy of 97.9%.
□ QUBIC2: A novel and robust biclustering algorithm for analyses and interpretation of large-scale RNA-Seq data
>> https://academic.oup.com/bioinformatics/advance-article-abstract/doi/10.1093/bioinformatics/btz692/5567116
QUBIC2 is a fast and efficient dropouts-saving expansion strategy for functional gene modules optimization using information divergency,
and a rigorous statistical test for the significance of all the identified biclusters in any organism, including those without substantial functional annotations.
QUalitative BIClustering algorithm Version 2 (QUBIC2), which is empowered by: a novel left-truncated mixture of Gaussian model for an accurate assessment of multimodality in zero-enriched expression data.
□ The mass-energy-information equivalence principle
>> https://aip.scitation.org/doi/10.1063/1.5123794
all the missing dark matter is in fact information mass, the initial estimates indicate that ∼10^93 bits would be sufficient to explain all the missing dark matter in the visible Universe.
information has mass and could account for universe’s dark matter. and could be developing a sensitive interferometer similar to LIGO or an ultra-sensitive Kibble balance.
□ Entropy Production as the Origin of Information Encoding in RNA and DNA
>> https://www.preprints.org/manuscript/201909.0146/v1
There is a non-equilibrium thermodynamic imperative which favors the amplification of fluctuations which lead to stationary states (disipative structures) with greater dissipation efficacy.
Information related to which nucleic acid – amino acid complexes provided most efficient photon dissipation would thus gradually have begun to be incorporated into the primitive genetic code.

□ dnAQET: a framework to compute a consolidated metric for benchmarking quality of de novo assemblies
>> https://link.springer.com/article/10.1186/s12864-019-6070-x
The dnAQET framework comprises of two main steps: (i) aligning assembled scaffolds (contigs) to a trusted reference genome and then (ii) calculating quality scores for the scaffolds and the whole assembly.
Using this strategy, dnAQET achieves a high level of parallelization for the alignment step that enables the tool to scale the quality evaluation processes for large de novo assemblies.

□ Deep Augmented Multiview Clustering
>> https://www.biorxiv.org/content/biorxiv/early/2019/09/16/770016.full.pdf
Deep Collective Matrix Factorization (dCMF), a neural architecture for collective matrix factorization where shared latent representations of each entity are obtained through deep autoencoders.

□ Valid Post-clustering Differential Analysis for Single-Cell RNA-Seq
>> https://www.cell.com/cell-systems/fulltext/S2405-4712(19)30269-8
clustering “forces” separation, reusing the same dataset generates artificially low p values and hence false discoveries. a valid framework and test to correct for the selection bias, this framework finds more relevant genes on single-cell datasets.


□ Galapagos: Straightforward clustering of single-cell RNA-Seq data with t-SNE and DBSCAN
>> https://www.biorxiv.org/content/biorxiv/early/2019/09/16/770388.full.pdf
Galapagos (Generally applicable low-complexity approach for the aggregation of similar cells), a simple and effective clustering workflow based on t-SNE and DBSCAN that does not require a gene selection step.

□ A Bayesian approach to accurate and robust signature detection on LINCS L1000 data
>> https://www.biorxiv.org/content/biorxiv/early/2019/09/16/769620.full.pdf
a novel Bayes’ theory based deconvolution algorithm that gives unbiased likelihood estimations for peak positions and characterizes the peak with a probability based z-scores.
The gene expression profiles deconvoluted from this Bayesian method achieve higher similarity between bio-replicates and drugs with shared targets than those generated from the existing methods.
※コメント投稿者のブログIDはブログ作成者のみに通知されます