


□ Long-read sequence and assembly of segmental duplications:
>> https://www.nature.com/articles/s41592-018-0236-3
a computational method based on polyploid phasing of long sequence reads to resolve collapsed regions of segmental duplications within genome assemblies. Segmental Duplication Assembler (SDA) constructs graphs in which paralogous sequence variants define the nodes and long-read sequences provide attraction and repulsion edges, enabling the partition and assembly of long reads corresponding to distinct paralogs.

□ scAlign: a tool for alignment, integration and rare cell identification from scRNA-seq data:
>> https://www.biorxiv.org/content/biorxiv/early/2018/12/22/504944.full.pdf
scAlign, a deep learning method for aligning and integrating scRNA-seq data collected across multiple conditions into a common, low-dimensional cell state space for downstream analysis such as clustering and trajectory inference across conditions. scAlign simultaneously aligns scRNA-seq from multiple conditions and performs a non-linear dimensionality reduction on the transcriptomes, and largely robust to the size of the architecture and network depth and width along with choice of hyper parameters.

□ High-dimensional Bayesian network inference from systems genetics data using genetic node ordering:
>> https://www.biorxiv.org/content/early/2018/12/24/501460
Bayesian network inference using pairwise node ordering is a highly efficient approach for reconstructing gene regulatory networks from High-Dimensional Systems data, which outperforms MCMC methods by assembling pairwise causal inference results in a global causal network.

□ nanoNOMe: Simultaneous profiling of chromatin accessibility and methylation on human cell lines with nanopore sequencing:
>> https://www.biorxiv.org/content/biorxiv/early/2018/12/22/504993.full.pdf
nanoNOMe combines the ability of NOMe-seq to simultaneously evaluate CpG and chromatin accessibility, with long-read nanopore sequencing technology. Using the bisulfite mode on IGV, we can view methylation over the length of long reads at single-read resolution.

□ SeQuiLa-cov: A fast and scalable library for depth of coverage calculations:
>> https://www.biorxiv.org/content/biorxiv/early/2018/12/13/494468.full.pdf
SeQuiLa-cov, an extension to the recently released SeQuiLa platform, runs a redesigned event-based algorithm for the distributed environment, which provides efficient depth of coverage calculations, reaching more than 100x speedup over the state-of-the-art tools. Performance and scalability of SeQuiLa-cov allows for exome and genome-wide calculations running locally or on a cluster while hiding the complexity of the distributed computing with Structured Query Language Application Programming Interface.

□ SVIM: Structural Variant Identification using Mapped Long Reads:
>> https://www.biorxiv.org/content/biorxiv/early/2018/12/13/494096.full.pdf
SVIM consists of three components for the collection, clustering and combination of structural variant signatures from read alignments. It discriminates 5 different variant classes including similar types, such as tandem and interspersed duplications and novel element insertions. SVIM is unique in its capability of extracting both the genomic origin and destination of duplications. It compares favorably with existing tools in evaluations on simulated data and real datasets from PacBio and Nanopore sequencing machines.
□ EXtrACtor, a tool for multiple queries and data extractions from the EXAC and gnomAD database:
>> https://www.biorxiv.org/content/biorxiv/early/2018/12/10/483909.full.pdf
It then queries the ExAC or gnomAD website for the data which would normally be returned in the browser (variation data, coverage information, etc) and after the data is retrieved quick selections can be made on which exact filtering steps can be executed, updating the data in EXtrACtor in real-time without the need to generate new queries to the ExAC database.

□ scCapsNet: a deep learning classifier with the capability of interpretable feature extraction, applicable for single cell RNA data analysis:
>> https://www.biorxiv.org/content/biorxiv/early/2018/12/27/506642.full.pdf
The parallel fully connected neural networks could function like a feature extractor as convolutional neural networks in the original CapsNet model. scCapsNet provides the precise contribution of each extracted feature to the cell type recognition, and could be used in the classification scenario where multiple information sources are available such as -omic datasets with data generated across different biological layers.
□ elPrep 4: A multithreaded framework for sequence analysis:
>> https://www.biorxiv.org/content/biorxiv/early/2018/12/10/492249.full.pdf
elPrep 4, a reimplementation from scratch of the elPrep framework for processing sequence alignment map files in the Go programming language. elPrep executes the preparation steps of the GATK Best Practices up to 13x faster on WES data, and up to 7.4x faster for WGS data compared to running the same pipeline with GATK 4, while utilizing fewer compute resources.
□ Scavager: a versatile postsearch validation algorithm for shotgun proteomics based on gradient boosting:
>> https://onlinelibrary.wiley.com/doi/abs/10.1002/pmic.201800280
Scavager employing CatBoost, an open‐source gradient boosting library, which shows improved efficiency compared with the other machine learning algorithms, such as Percolator, PeptideProphet, and Q‐ranker. Scavager - a proteomics post-search validation tool, currently supported search engines: Identipy, X!Tandem, Comet, MSFragger, msgf+, Morpheus.
□ npGraph - Resolve assembly graph in real-time using nanopore data:
>> https://github.com/hsnguyen/assembly
npGraph is another real-time scaffolder beside npScarf. Instead of using contig sequences as pre-assemblies, this tool is able to work on assembly graph (from SPAdes). If the sequences are given, then it's mandatory to have either BWA-MEM or minimap2 installed in your system to do the alignment between long reads and the pre-assemblies.
□ m-pCMF / ZINBayes: Scalable probabilistic matrix factorization for single-cell RNA-seq analysis:
>> https://www.biorxiv.org/content/biorxiv/early/2018/12/14/496810.full.pdf
Two novel generative models for dimensionality reduction: modified probabilistic count matrix factorization (m-pCMF) and Bayesian zero-inflated negative binomial factorization (ZINBayes). In terms of cell type separability in the reduced spaces, m-pCMF and ZINBayes yield higher ASW scores and moderately lower ARI and NMI scores than all competing methods, except pCMF, in the ZEISEL data set.
□ CDSeq: A novel computational complete deconvolution method using RNA-seq data:
>> https://www.biorxiv.org/content/biorxiv/early/2018/12/14/496596.full.pdf
CDSeq is a complete deconvolution algorithm that takes RNA-seq data (raw read counts) from a collection of possibly heterogeneous samples as input and returns estimates of GEPs for each constituent cell type as well as the proportional representation of those cell types.
□ Hera-EM: A revisit of RSEM generative model and its EM algorithm for quantifying transcript abundances.
>> https://www.biorxiv.org/content/early/2018/12/21/503672
100x faster than RSEM with better accuracy. Hera-EM identified and removed early converged parameters to significantly reduce the model complexity in further iterations, and used SQUAREM method to speed up the convergence. On a data set with 60 million of reads, RSEM takes about an hour (3432 seconds) for EM step only, while Hera-EM needs half a minute (24 seconds); for an other data set with 75 million of reads, RSEM takes about 1.5 hours (5044 seconds), while Hera-EM takes 39 seconds.
□ Real-Time Point Process Filter for Multidimensional Decoding Problems Using Mixture Models:
>> https://www.biorxiv.org/content/biorxiv/early/2018/12/23/505289.full.pdf
the posterior distribution on each filtering time-step can be approximated using a Gaussian Mixture Model. The algorithm provides a real-time solution for multi-dimensional point-process filter problem and attains accuracy comparable to the exact solution.

□ SNIPER: Revealing Hi-C subcompartments by imputing high-resolution inter-chromosomal chromatin interactions
>> https://www.biorxiv.org/content/biorxiv/early/2018/12/23/505503.full.pdf
a new computational approach, named SNIPER, based on an autoencoder and multilayer perceptron classifier to infer subcompartments using typical Hi-C datasets with moderate coverage.

□ Stochastic diffusion framework determines the free-energy landscape and rate from single-molecule trajectory:
>> https://www.ncbi.nlm.nih.gov/pubmed/30579309
This manuscript reports a general theoretical/computational methodology that characterises D(Q) [and by consequence, F(Q) and τf] by only giving as an input one single-molecule time-dependent trajectory [Q(t)]. The stochastic approach recovered v and F, in which the lattice model simulations were subjected to by simply imposing Gaussian distributions diffusing in one-dimension reaction coordinates.
□ Cryfa: a secure encryption tool for genomic data:
>> https://academic.oup.com/bioinformatics/article/35/1/146/5055587
Cryfa, a fast secure encryption tool for genomic data, namely in Fasta, Fastq, VCF, SAM and BAM formats, which is also capable of reducing the storage size of Fasta and Fastq files. Cryfa uses advanced encryption standard (AES) encryption combined with a shuffling mechanism, which leads to a substantial enhancement of the security against low data complexity attacks.
□ RGAAT: A Reference-based Genome Assembly and Annotation Tool for New Genomes and Upgrade of Known Genomes:
>> https://www.sciencedirect.com/science/article/pii/S1672022918304376
RGAAT can detect sequence variants with comparable precision, specificity, and sensitivity to GATK and with higher precision and specificity than Freebayes & SAMtools on four DNA-seq, and can identify sequence variants based on cross-cultivar or cross-version genomic alignments.

□ sn-m3C-seq: Single-cell multi-omic profiling of chromatin conformation and DNA methylome:
>> https://www.biorxiv.org/content/biorxiv/early/2018/12/26/503235.full.pdf
The sn-m3C-seq method allows unequivocal clustering of cell types using two orthogonal types of epigenomic information and the reconstruction of cell-type specific chromatin conformation maps.

□ ORGaNICs: A Canonical Neural Circuit Computation:
>> https://www.biorxiv.org/content/biorxiv/early/2018/12/26/506337.full.pdf
The ORGaNICs (Oscillatory Recurrent GAted Neural Integrator Circuits) theory provides a means for reading out information from the dynamically varying responses at any point in time, in spite of the complex dynamics.

□ A Darwinian Uncertainty Principle:
>> https://www.biorxiv.org/content/biorxiv/early/2018/12/26/506535.full.pdf
the simulation results have a similar flavor to a fundamental principle in quantum physics – Heisenberg’s uncertainty principle – which provides an absolute lower bound on the precision of simultaneously estimating both the position and the momentum of a particle. the phylogenetic analogue of ‘position’ as ‘ancestral state’, and thus ‘momentum’ (closely related to velocity) corresponds to the rates at which ancestral states change into different alternative states.
□ HiCluster: A Robust Single-Cell Hi-C Clustering Method Based on Convolution and Random Walk:
>> https://www.biorxiv.org/content/biorxiv/early/2018/12/27/506717.full.pdf
HiCluster, a single-cell clustering algorithm for Hi-C contact matrices that is based on imputations using linear convolution and random walk. Using both simulated and real data as benchmarks.

□ S3norm: simultaneous normalization of sequencing depth and signal-to-noise ratio in epigenomic data:
>> https://www.biorxiv.org/content/biorxiv/early/2018/12/26/506634.full.pdf
S3norm is a robust way to normalize both SDs and SNRs across multiple data sets. S3norm can become increasingly useful to normalize signals across these diverse and heterogeneous epigenomic data sets and better highlight true epigenetic changes against technical bias.

□ Time-resolved mapping of genetic interactions to model rewiring of signaling pathways:
>> https://elifesciences.org/articles/40174
the genetic interactions form in different trajectories and developed an algorithm, termed MODIFI, to analyze how genetic interactions rewire over time.
□ EMEP: Single-cell RNA-seq Interpretations using Evolutionary Multiobjective Ensemble Pruning:
>> https://academic.oup.com/bioinformatics/advance-article-abstract/doi/10.1093/bioinformatics/bty1056/5265329
EMEP is designed to dynamically select the suitable clustering results from the ensembles. This algorithm firstly applies the unsupervised dimensionality reduction to project data from the original high dimensions to low-dimensional sub-spaces.
□ FINDOR: Leveraging Polygenic Functional Enrichment to Improve GWAS Power
>> https://www.cell.com/ajhg/fulltext/S0002-9297(18)30411-7
□ TIGAR: An Improved Bayesian Tool for Transcriptomic Data Imputation Enhances Gene Mapping of Complex Traits:
>> https://www.biorxiv.org/content/biorxiv/early/2018/12/28/507525.full.pdf
TIGAR (Transcriptome-Integrated Genetic Association Resource) integrates both data-driven nonparametric Bayesian and Elastic-Net models for transcriptomic data imputation, along with TWAS and summary-level GWAS data for univariate and multi-variate phenotypes.

□ LATE / TRANSLATE: Imputation of single-cell gene expression with an autoencoder neural network:
>> https://www.biorxiv.org/content/biorxiv/early/2018/12/29/504977.full.pdf
TRANSLATE builds on LATE and further incorporates a reference gene expression data set (bulk gene expression, larger scRNA-seq data set through transfer learning.
□ R/qtl2: Software for Mapping Quantitative Trait Loci with High-Dimensional Data and Multi-parent Populations
>> http://www.genetics.org/content/early/2018/12/26/genetics.118.301595
R/qtl2 is designed to handle modern high-density genotyping data and high-dimensional molecular phenotypes including gene expression and proteomics.

□ Janggu - Deep learning for Genomics:
>> https://github.com/BIMSBbioinfo/janggu
Janggu provides special Genomics datasets that allow you to access raw data in FASTA, BAM, BIGWIG, BED and GFF file format.
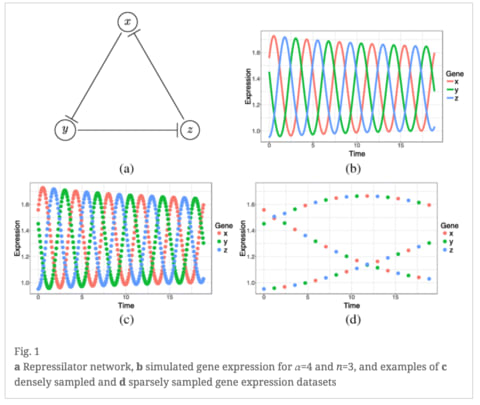
□ Time-lagged Ordered Lasso for network inference
>> https://bmcbioinformatics.biomedcentral.com/articles/10.1186/s12859-018-2558-7
a regularized regression method with temporal monotonicity constraints, for de novo reconstruction.
□ Libra: scalable k-mer based tool for massive all-vs-all metagenome comparisons:
>> https://academic.oup.com/gigascience/advance-article/doi/10.1093/gigascience/giy165/5266304
Libra uses a scalable Hadoop framework for massive metagenome comparisons, Cosine Similarity for calculating the distance using sequence composition and abundance while normalizing for sequencing depth.
※コメント投稿者のブログIDはブログ作成者のみに通知されます