

□ Fugue: Scalable batch-correction method for integrating large-scale single-cell transcriptomes
>> https://www.biorxiv.org/content/10.1101/2021.12.12.472307v1.full.pdf
Fugue extended the deep learning method at the heart of our recently published Miscell approach. Miscell learns representations of single-cell expression profiles through contrastive learning and achieves high performance on canonical single-cell analysis tasks.
Fugue encodes batch information of each cell as a trainable parameter and added to its expression profile; a contrastive learning approach is used to learn feature representation. Fugue can learn smooth embedding for time course trajectory and joint embedding space.

□ FIN: Bayesian Factor Analysis for Inference on Interactions
>> https://www.tandfonline.com/doi/full/10.1080/01621459.2020.1745813
Current methods for quadratic regression are not ideal in these applications due to the level of correlation in the predictors, the fact that strong sparsity assumptions are not appropriate, and the need for uncertainty quantification.
FIN exploits the correlation structure of the predictors, and estimates interaction effects in high dimensional settings. FIN uses a latent factor joint model, which incl. shared factors in both the predictor and response components while assuming conditional independence.
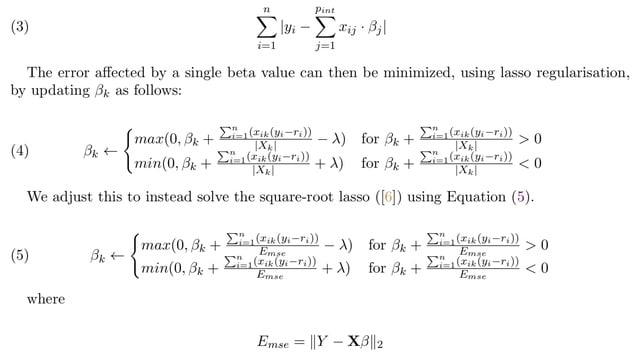
□ Pint: A Fast Lasso-Based Method for Inferring Higher-Order Interactions
>> https://www.biorxiv.org/content/10.1101/2021.12.13.471844v1.full.pdf
Pint performs square-root lasso regression on all pairwise interactions on a one thousand gene screen, using ten thousand siRNAs, in 15 seconds, and all three-way interactions on the same set in under ten minutes.
Pint is based on an existing fast algorithm, which adapts for use on binary matrices. The three components of the algorithm, pruning, active set calculation, and solving the sub-problem, can all be done in parallel.

□ TopHap: Rapid inference of key phylogenetic structures from common haplotypes in large genome collections with limited diversity
>> https://www.biorxiv.org/content/10.1101/2021.12.13.472454v1.full.pdf
TopHap determines spatiotemporally common haplotypes of common variants and builds their phylogeny at a fraction of the computational time of traditional methods.
In the TopHap approach, bootstrap branch support for the inferred phylogeny of common haplotypes is calculated by resampling genomes to build bootstrap replicate datasets.
This procedure assesses the robustness of the inferred phylogeny to the inclusion/exclusion of haplotypes likely created by sequencing errors and convergent changes that are expected to have relatively low frequencies spatiotemporally.

□ swCAM: estimation of subtype-specific expressions in individual samples with unsupervised sample-wise deconvolution
>> https://academic.oup.com/bioinformatics/advance-article/doi/10.1093/bioinformatics/btab839/6460803
a sample-wise Convex Analysis of Mixtures (swCAM) can accurately estimate subtype-specific expressions of major subtypes in individual samples and successfully extract co-expression networks in particular subtypes that are otherwise unobtainable using bulk expression data.
Fundamental to the success of swCAM solution is the nuclear-norm and l2,1-norm regularized low-rank latent variable modeling.
Determining hyperparameter values using cross-validation with random entry exclusion and obtain a swCAM solution using an efficient alternating direction method of multipliers.

□ Scalable, ultra-fast, and low-memory construction of compacted de Bruijn graphs with Cuttlefish 2
>> https://www.biorxiv.org/content/10.1101/2021.12.14.472718v1.full.pdf
The compacted de Bruijn graph forms a vertex-decomposition of the graph, while preserving the graph topology. However, for some applications, only the vertex-decomposition is sufficient, and preservation of the topology is redundant.
for applications such as performing presence-absence queries for k-mers or associating information to the con- stituent k-mers of the input, any set of strings that preserves the exact set of k-mers from the input sequences can be sufficient.
Relaxing the defining requirement of unit igs, that the paths be non-branching in the underlying graph, and seeking instead a set of maximal non-overlapping paths covering the de Bruijn graph, results in a more compact rep- resentation of the input data.
CUTTLE-FISH 2 can seamlessly extract such maximal path covers by simply constraining the algorithm to operate on some specific subgraph(s) of the original graph.

□ Matchtigs: minimum plain text of kmer sets
>> https://www.biorxiv.org/content/10.1101/2021.12.15.472871v1.full.pdf
Matchtigs, a polynomial algorithm computing a minimum representation (which was previously posed as a potentially NP-hard open problem), as well as an efficient near-minimum greedy heuristic.
Matchtigs finds an SPSS (spectrum preserving string set) of minimum size (CL). the SPSS problem allowing repeated kmers is polynomially solvable, based on a many-to-many min-cost path query and a min-cost perfect matching approach.

□ AliSim: A Fast and Versatile Phylogenetic Sequence Simulator For the Genomic Era
>> https://www.biorxiv.org/content/10.1101/2021.12.16.472905v1.full.pdf
AliSim integrates a wide range of evolutionary models, available in the IQ-TREE. AliSim can simulate MSAs that mimic the evolutionary processes underlying empirical alignments.
AliSim implements an adaptive approach that combines the commonly-used rate matrix and probability matrix approach. AliSim works by first generating a sequence at the root of the tree following the stationarity of the model.
AliSim then recursively traverses along the tree to generate sequences at each node of the tree based on the sequence of its ancestral node. AliSim completes this process once all the sequences at the tips are generated.

□ ortho2align: a sensitive approach for searching for orthologues of novel lncRNAs
>> https://www.biorxiv.org/content/10.1101/2021.12.16.472946v1.full.pdf
lncRNAs exhibit low sequence conservation, so specific methods for enhancing the signal-to-noise ratio were developed. Nevertheless, current methods such as transcriptomes comparison or searches for conserved secondary structures are not applicable to novel lncRNAs dy design.
ortho2align — a synteny-based approach for finding orthologues of novel lncRNAs with a statistical assessment of sequence conservation. ortho2align allows control of the specificity of the search process and optional annotation of found orthologues.

□ EmptyNN: A neural network based on positive and unlabeled learning to remove cell-free droplets and recover lost cells in scRNA-seq data
>> https://www.cell.com/patterns/fulltext/S2666-3899(21)00154-9
EmptyNN accurately removed cell-free droplets while recovering lost cell clusters, and achieved an area under the receiver operating characteristics of 94.73% and 96.30%, respectively.
EmptyNN takes the raw count matrix as input, where rows represent barcodes and columns represent genes. The output is a list, containing a Boolean vector indicating it is a cell-containing or cell-free droplet, as well as the probability of each droplet.

□ AMAW: automated gene annotation for non-model eukaryotic genomes
>> https://www.biorxiv.org/content/10.1101/2021.12.07.471566v1.full.pdf
Iterative runs of MAKER2 must also be coordinated to aim for accurate predictions, which includes intermediary specific training of different gene predictor models.
AMAW (Automated MAKER2 Annotation Wrapper), a program devised to annotate non-model unicellular eukaryotic genomes by automating the acquisition of evidence data.
□ Pak RT
Merge supply is decreasing.
Watch.
>> https://etherscan.io/token/0x27d270b7d58d15d455c85c02286413075f3c8a31

□ HolistIC: leveraging Hi-C and whole genome shotgun sequencing for double minute chromosome discovery
>> https://academic.oup.com/bioinformatics/advance-article-abstract/doi/10.1093/bioinformatics/btab816/6458320
HolistIC can enhance double minute chromosome predictions by predicting DMs with overlapping amplicon coordinates. HolistIC can uncover double minutes, even in the presence of DM segments with overlapping coordinates.
HolistIC is ideal for confirming the true association of amplicons to circular extrachromosomal DNA. it is modular in that the double minute prediction input can be from any program. This lends additional flexibility for future eccDNA discovery algorithms.

□ geneBasis: an iterative approach for unsupervised selection of targeted gene panels from scRNA-seq
>> https://genomebiology.biomedcentral.com/articles/10.1186/s13059-021-02548-z
geneBasis, an iterative approach for selecting an optimal gene panel, where each newly added gene captures the maximum distance between the true manifold and the manifold constructed using the currently selected gene panel.
geneBasis allows recovery of local and global variability. geneBasis accounts for batch effect and handles unbalanced cell type composition.
geneBasis constructs k-NN graphs within each batch, thereby assigning nearest neighbors only from the same batch and mitigating technical effects. Minkowski distances per genes are calculated across all cells from every batch thus resulting in a single scalar value for each gene.

□ scMARK an 'MNIST' like benchmark to evaluate and optimize models for unifying scRNA data
>> https://www.biorxiv.org/content/10.1101/2021.12.08.471773v1.full.pdf
scMARK uses unsupervised models to reduce the complete set of single-cell gene expression matrices into a unified cell-type embedding space. And trains a collection of supervised models to predict author labels from all but one held-out dataset in this unified cell-type space.
scMARK show that scVI represents the only tested method that benefits from larger training datasets. Qualitative assessment of the unified cell-type space indicates that the scVI embedding is suitable for automatic cell-type labeling and discovery of new cell-types.

□ DISA tool: discriminative and informative subspace assessment with categorical and numerical outcomes
>> https://www.biorxiv.org/content/10.1101/2021.12.08.471785v1.full.pdf
DISA (Discriminative & Informative Subspace Assessment) is proposed to assess patterns in the presence of numerical outcomes using well-established measures together w/ a novel principle able to statistically assess the correlation gain of the subspace against the overall space.
If DISA receives a numerical outcome, a range of values in which samples are valid is determined. DISA accomplishes this by approximating two probability density functions (e.g. Gaussians), one for all the observed targets and the other with targets of the target subspace.

□ Improved Transcriptome Assembly Using a Hybrid of Long and Short Reads with StringTie
>> https://www.biorxiv.org/content/10.1101/2021.12.08.471868v1.full.pdf
a new release of StringTie which allows transcriptome assembly and quantification using a hybrid dataset containing both short and long reads.
Hybrid-read assembly with StringTie is more accurate than long-read only or short-read only assembly, and on some datasets it can more than double the number of correctly assembled transcripts, while obtaining substantially higher precision than the long-read data assembly alone.

□ scATAK: Efficient pre-processing of Single-cell ATAC-seq data
>> https://www.biorxiv.org/content/10.1101/2021.12.08.471788v1.full.pdf
The scATAK track module generated group ATAC signal tracks (normalized by the mapped group read counts) from cell barcode – cell group table and sample pseudo-bulk alignment file.
scATAK hic module utilizes a provided bulk HiC or HiChIP interactome map together with a single-cell accessible chromatin region matrix to infer potential chromatin looping events for individual cells and generate group HiC interaction tracks.

□ DeepPlnc: Discovering plant lncRNAs through multimodal deep learning on sequential data
>> https://www.biorxiv.org/content/10.1101/2021.12.10.472074v1.full.pdf
LncRNAs are supposed to act as a key modulator for various biological processes. Their involvement is reported in controlling transcription process through enhancers and providing regulatory binding sites is well reported
DeepPlnc can even accurately annotate the incomplete length transcripts also which are very common in de novo assembled transcriptomes. It has incorporated a bi-modal architecture of Convolution Neural Nets while extracting information from the sequences of nucleotides.
□ A mosaic bulk-solvent model improves density maps and the fit between model and data
>> https://www.biorxiv.org/content/10.1101/2021.12.09.471976v1
The mosaic bulk-solvent model considers solvent variation across the unit cell. The mosaic model is implemented in the computational crystallography toolbox and can be used in Phenix in most contexts where accounting for bulk-solvent is required.
Using the mosaic solvent model improves the overall fit of the model to the data and reduces artifacts in residual maps. The mosaic model algorithm was systematically exercised against a large subset of PDB entries to ensure its robustness and practical utility to improve maps.
□ Coalescent tree recording with selection for fast forward-in-time simulations
>> https://www.biorxiv.org/content/10.1101/2021.12.06.470918v1.full.pdf
The algorithm records the genetic history of a species, directly places the mutations on the tree and infers fitness of subsets of the genome from parental haplotypes. The algorithm explores the tree to reconstruct the genetic data at the recombining segment.
When reproducing, if a segment is transmitted without recombination, then the fitness contribution of this segment in the offspring individual is simply the fitness contribution of the parental segment multiplied by the effects of eventual new mutations.
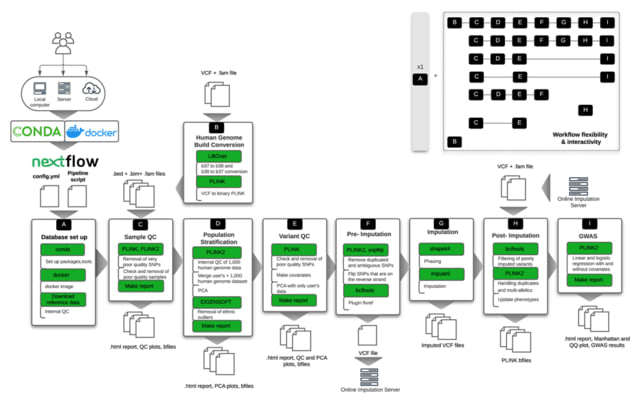
□ snpQT: flexible, reproducible, and comprehensive quality control and imputation of genomic data
>> https://f1000research.com/articles/10-567
snpQT: a scalable, stand-alone software pipeline using nextflow and BioContainers, for comprehensive, reproducible and interactive quality control of human genomic data.
snpQT offers some 36 discrete quality filters or correction steps in a complete standardised pipeline, producing graphical reports to demonstrate the state of data before and after each quality control procedure.

□ High performance of a GPU-accelerated variant calling tool in genome data analysis
>> https://www.biorxiv.org/content/10.1101/2021.12.12.472266v1.full.pdf
Sequencing data were analyzed on the GPU server using BaseNumber, the variant calling outputs of which were compared to the reference VCF or the results generated by the Burrows-Wheeler Aligner (BWA) + Genome Analysis Toolkit (GATK) pipeline on a generic CPU server.
BaseNumber demonstrated high precision (99.32%) and recall (99.86%) rates in variant calls compared to the standard reference. The variant calling outputs of the BaseNumber and GATK pipelines were very similar, with a mean F1 of 99.69%.
□ treedata.table: a wrapper for data.table that enables fast manipulation of large phylogenetic trees matched to data
>> https://peerj.com/articles/12450/
treedata.table, the first R package extending the functionality and syntax of data.table to explicitly deal with phylogenetic comparative datasets.
treedata.table significantly increases speed and reproducibility during the data manipulation involved in the phylogenetic comparative workflow. After an initial tree/data matching step, treedata.table continuously preserves the tree/data matching across data.table operations.

□ tRForest: a novel random forest-based algorithm for tRNA-derived fragment target prediction
>> https://www.biorxiv.org/content/10.1101/2021.12.13.472430v1.full.pdf
A significant advantage of using random forests is that they avoid overfitting, a common limitation of machine learning algorithms in which they become tailored specifically to the dataset they were trained on and thus become less predictive in independent datasets.
tRForest, a tRF target prediction algorithm built using the random forest machine learning algorithm. This algorithm predicts targets for all tRFs, including tRF-1s and includes a broad range of features to fully capture tRF-mRNA interaction.

□ Flimma: a federated and privacy-aware tool for differential gene expression analysis
>> https://genomebiology.biomedcentral.com/articles/10.1186/s13059-021-02553-2
Flimma - Fererated Limma Voom Tool preserves the privacy of the local data since the expression profiles never leave the local execution sites.
In contrast to meta-analysis approaches, Flimma is particularly robust against heterogeneous distributions of data across the different cohorts, which makes it a powerful alternative for multi-center studies where patient privacy matters.

□ GREPore-seq: A Robust Workflow to Detect Changes after Gene Editing through Long-range PCR and Nanopore Sequencing
>> https://www.biorxiv.org/content/10.1101/2021.12.13.472514v1.full.pdf
GREPore-seq captures the barcoded sequences by grepping reads of nanopore amplicon sequencing. GREPore-seq combines indel-correcting DNA barcodes with the sequencing of long amplicons on the ONT platforms.
GREPore-seq can detect NHEJ-mediated double-stranded oligodeoxynucleotide (dsODN) insertions with comparable accuracy to Illumina NGS. GREPore-seq also identifies HDR-mediated large gene knock-in, which excellently correlates with FACS analysis data.

□ CellOT: Learning Single-Cell Perturbation Responses using Neural Optimal Transport
>> https://www.biorxiv.org/content/10.1101/2021.12.15.472775v1.full.pdf
Leveraging the theory of optimal transport and the recent advents of convex neural architectures, they learn a coupling describing the response of cell populations upon perturbation, enabling us to predict state trajectories on a single-cell level.
CellOT, a novel approach to predict single-cell perturbation responses by uncovering couplings between control and perturbed cell states while accounting for heterogeneous subpopulation structures of molecular environments.
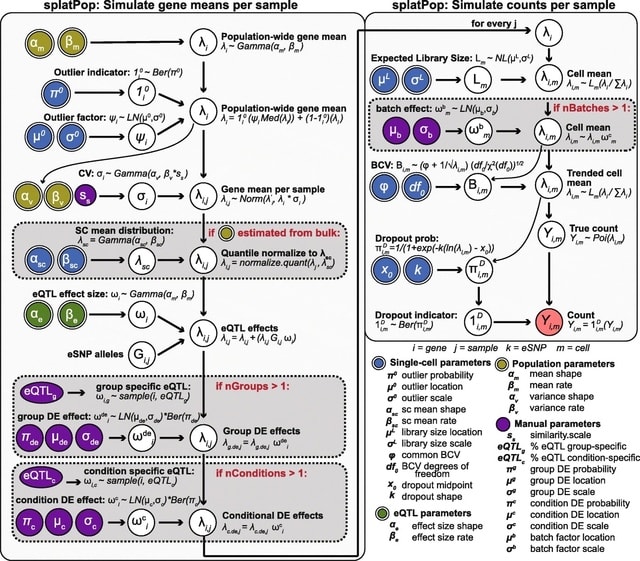
□ splatPop: simulating population scale single-cell RNA sequencing data
>> https://genomebiology.biomedcentral.com/articles/10.1186/s13059-021-02546-1
splatPop, a model for flexible, reproducible, and well-documented simulation of population-scale scRNA-seq data with known expression quantitative trait loci. splatPop can also be instructed to assign pairs of eGenes the same eSNP.
The splatPop model utilizes the flexible framework of Splatter, and can simulate complex batch, cell group, and conditional effects between individuals from different cohorts as well as genetically-driven co-expression.

□ Nfeature: A platform for computing features of nucleotide sequences
>> https://www.biorxiv.org/content/10.1101/2021.12.14.472723v1.full.pdf
Nfeature comprises of three major modules namely Composition, Correlation, and Binary profiles. Composition module allow to compute different type of compositions that includes mono-/di-tri-nucleotide composition, reverse complement composition, pseudo composition.
Correlation module allow to compute various type of correlations that includes auto-correlation, cross-correlation, pseudo-correlation. Similarly, binary profile is developed for computing binary profile based on nucleotides, di-nucleotides, di-/tri-nucleotide properties.
Nfeature also allow to compute entropy of sequences, repeats in sequences and distribution of nucleotides in sequences. This tool computes a total of 29217 and 14385 features for DNA and RNA sequence, respectively.

□ GENPPI: standalone software for creating protein interaction networks from genomes
>> https://bmcbioinformatics.biomedcentral.com/articles/10.1186/s12859-021-04501-0
GENPPI can help fill the gap concerning the considerable number of novel genomes assembled monthly and our ability to process interaction networks considering the noncore genes for all completed genome versions.
GENPPI transfers the question of topological annotation from the centralized databases to the final user, the researcher, at the initial point of research. the GENPPI topological annotation information is directly proportional to the number of genomes used to create an annotation.

□ Sim-it: A benchmark of structural variation detection by long reads through a realistic simulated model
>> https://genomebiology.biomedcentral.com/articles/10.1186/s13059-021-02551-4
Sim-it, a straightforward tool for the simulation of both structural variation and long-read data. These simulations from Sim-it reveal the strengths and weaknesses for current available structural variation callers and long-read sequencing platforms.
combiSV is a new method that can combine the results from structural variation callers into a superior call set with increased recall and precision, which is also observed for the latest structural variation benchmark set.

□ seGMM: a new tool to infer sex from massively parallel sequencing data
>> https://www.biorxiv.org/content/10.1101/2021.12.16.472877v1.full.pdf
seGMM, a new sex inference tool that determines the gender of a sample from called genotype data integrated aligned reads and jointly considers information on the X and Y chromosomes in diverse genomic data, including TGS panel data.
seGMM applies Gaussian Mixture Model (GMM) clustering to classify the samples into different clusters. seGMM provides a reproducible framework to infer sex from massively parallel sequencing data and has great promise in clinical genetics.

□ FourierDist: HarmonicNet: Fully Automatic Cell Segmentation with Fourier Descriptors
>> https://www.biorxiv.org/content/10.1101/2021.12.17.472408v1.full.pdf
FourierDist, a network, which is a modification of the popular StarDist and SplineDist architectures. FourierDist utilizes Fourier descriptors, predicting a coefficient vector for every pixel on the image, which implicitly define the resulting segmentation.
FourierDist is also capable of accurately segmenting objects that are not star-shaped, a case where StarDist performs suboptimally.

□ Analyzing transfer learning impact in biomedical cross-lingual named entity recognition and normalization
>> https://bmcbioinformatics.biomedcentral.com/articles/10.1186/s12859-021-04247-9
Firstly, for entity identification and classification, they implemented two bidirectional Long Short Memory (Bi-LSTM) layers with a CRF layer based on the NeuroNER model. The architecture of this model consists of a first Bi-LSTM layer for character embeddings.
In the second layer, they concatenate the output of the first layer with the word embeddings and sense-disambiguate embeddings for the second Bi-LSTM layer. Finally, the last layer uses a CRF to obtain the most suitable labels for each token.
※コメント投稿者のブログIDはブログ作成者のみに通知されます