
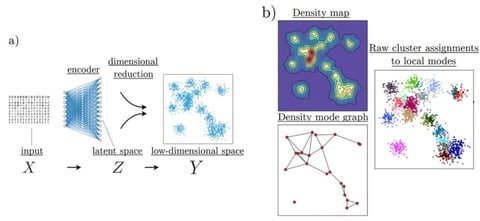
□ HAL-x: Scalable Clustering with Supervised Linkage Methods
>> https://www.biorxiv.org/content/10.1101/2021.08.01.454697v1.full.pdf
HAL-x, a novel hierarchical density clustering algorithm that uses supervised linkage methods to build a cluster hierarchy on raw single-cell data. HAL-x is designed to cluster datasets with up to 100 million points embedded in a 50+ dimensional space.
HAL-x can ensure that the predictive power is limited by the reproducibility of our clustering assignments and not by the choice of classifier. HAL-x defines an extended density neighborhood for each pure cluster, identifying spurious clusters that are representative of the same density maxima.

□ dynDeepDRIM: a dynamic deep learning model to infer direct regulatory interactions using single cell time-course gene expression data
>> https://www.biorxiv.org/content/10.1101/2021.08.28.458048v1.full.pdf
dynDeepDRIM integrated the primary image, neighbor images with time-course into a four-dimensional tensor and trained a convolutional neural network to predict the direct regulatory interactions between TFs and genes.
dynDeepDRIM structure consists of T subcomponents and 3 fully connected layers to produce the prediction values using Sigmoid function. The embeddings are transformed into another condensed embedding with 512 dimensions used to integrate w/ the results for the other time points.

□ β-VAE: Out-of-distribution prediction with disentangled representations for single-cell RNA sequencing data
>> https://www.biorxiv.org/content/10.1101/2021.09.01.458535v1.full.pdf
In disentanglement learning, a single latent dimension is linked to a single generative feature, while being relatively invariant to changes in other features.
β-VAE, a fully unsupervised model for disentanglement learning. The deviation of the KL divergence loss from C is penalized by β. β-VAE outperforms dHSIC in both disentanglement learning and OOD prediction.

□ BWA-MEME: BWA-MEM emulated with a machine learning approach
>> https://www.biorxiv.org/content/10.1101/2021.09.01.457579v1.full.pdf
BWA-MEME performs exact match search with O(1) memory accesses leveraging the learned index. BWA-MEME is based on a suffix array search algorithm that solves the challenges in utilizing learned indices for SMEM search which is extensively used in the seeding phase.
BWA-MEME achieves up to 3.45x speedup in seeding throughput over BWA-MEM2 by reducing the number of instructions by 4.60x, memory accesses by 8.77x, and LLC misses by 2.21x, while ensuring the identical SAM output to BWA-MEM2.
BWA-MEME uses a partially-3-layer recursive model index (P-RMI) which adapts well to the imbalanced distribution of suffixes and provides accurate prediction, and an algorithm that encodes the input substring or suffixes into a numerical key.

□ AMULET: a novel read count-based method for effective multiplet detection from single nucleus ATAC-seq data
>> https://genomebiology.biomedcentral.com/articles/10.1186/s13059-021-02469-x
AMULET (ATAC-seq MULtiplet Estimation Tool) enumerates regions with greater than two uniquely aligned reads across the genome to effectively detect multiplets. AMULET can detect multiplets with a runtime that scales near linearly with the number of cells/valid reads.
AMULET detected multiplets with high precision (assessed by sample multiplexing) and high recall (assessed by simulated multiplets), especially when samples are sequenced to a certain read depth, serving as an effective alternative to simulation-based ArchR.

□ GAMMA: a tool for the rapid identification, classification, and annotation of translated gene matches from sequencing data
>> https://academic.oup.com/bioinformatics/advance-article/doi/10.1093/bioinformatics/btab607/6355578
GAMMA is a command line tool that finds gene matches in microbial genomic data using protein coding (rather than nucleotide) identity, and then translates and annotates the match by providing the type (i.e., mutant, truncation, etc.) and a translated description.
GAMMA uses protein sequence similarity as the initial filter for determining calls, different calls occurred only when there were ambiguous, inexact matches at the protein level, which GAMMA resolves by using nucleotide similarity and then the least number of transversions.

□ STGATE: Deciphering spatial domains from spatially resolved transcriptomics with adaptive graph attention auto-encoder
>> https://www.biorxiv.org/content/10.1101/2021.08.21.457240v1.full.pdf
STGATE first constructs a spatial neighbor network (SNN) based on a pre-defined radius, and another optional one by pruning it according to the pre-clustering of gene expressions to better characterize the spatial similarity at the boundary of spatial domains.
STGATE learns low-dimensional latent representations with both spatial information and GE via a graph attention auto-encoder. The input of auto-encoder is the normalized expression matrix, and the graph attention layer is adopted in the middle of the encoder and decoder.

□ Supermeasured: Violating Statistical Independence without violating statistical independence
>> https://arxiv.org/pdf/2108.07292.pdf
Violations of Statistical Independence are commonly in- terpreted as correlations between the measurement settings and the hidden variables (which determine the mea- surement outcomes). Such correlations have been discarded as “finetuning” or a “conspiracy”.
The problem with the common interpretation is that Statistical Independence might be violated because of a non-trivial measure in state space, a possibility called “supermeasured”.
“supermeasured” is not under the control of the experimenter. ρBell contains information both about the intrinsic properties of the space and the distribution over the space. Interpretations of Bell’s theorem run afoul of physics whenever one is dealing with a theory μ(λ,X) ̸=μ0.

□ AENET: Interfaces for accurate and efficient molecular dynamics simulations with machine learning potentials
>> https://aip.scitation.org/doi/10.1063/5.0063880
ænet enables accurate simulations of large and complex systems with low computational cost that scales linearly with the number of atoms.
The ænet achieves excellent parallel efficiency on highly parallel distributed-memory systems and benefits from the highly optimized neighbor list. ænet make it possible to simulate atomic structures w/ millions of atoms w/ an accuracy close to first-principles calculations.

□ SiGMoiD: A super-statistical generative model for binary data
>> https://journals.plos.org/ploscompbiol/article?id=10.1371/journal.pcbi.1009275
Super-statistical Generative Model for binary Data (SiGMoiD) is a maximum entropy-based framework where we imagine the data as arising from super-statistical system.
SiGMoiD characterizes each binary variable using a K dimensional vector of features. SiGMoiD is significantly faster than typical max ent models, allowing us to analyze very high dimensional data sets (over 1000 dimensions) that remain well out of the reach of current max ent.

□ Regulus: a transcriptional regulatory networks inference tool based on Semantic Web technologies
>> https://www.biorxiv.org/content/10.1101/2021.08.02.454721v1.full.pdf
Regulus has been developed to be stringent and to limit the space of the candidates TF-genes relations highlighting the candidate relations which are the most likely to occur.
Regulus uses the system dynamics to decipher the inhibition and activation roles of regulators. Regulus relies on a principle of consistency between genomic landscape, genes and TF expressions to decide if a relation is susceptible to exist.

□ MegaLMM: Mega-scale linear mixed models for genomic predictions with thousands of traits
>> https://genomebiology.biomedcentral.com/articles/10.1186/s13059-021-02416-w
MegaLMM (linear mixed models for millions of observations), a novel statistical method for fitting massive-scale MvLMMs. MegaLMM dramatically improves upon existing methods that fit low-rank MvLMMs, allowing multiple random effects with large amounts of missing data.
MegaLMM decomposes a typical MvLMM into a two-level hierarchical model. MegaLMM is inherently a linear model and cannot effectively model trait relationships that are non-linear. MegaLMM estimates genetic values for all traits (both observed and missing) in a single step.

□ METAMVGL: a multi-view graph-based metagenomic contig binning algorithm by integrating assembly and paired-end graphs
>> https://bmcbioinformatics.biomedcentral.com/articles/10.1186/s12859-021-04284-4
METAMVGL applies the auto-weighted multi-view graph-based algorithm to optimize the weights of the two graphs and predict binning groups for the unlabeled contigs.
METAMVGL learns the two graphs’ weights automatically and predicts the contig labels in a uniform multi-view label propagation framework. METAMVGL made use of significantly more high-confidence edges from the combined graph and linked dead ends to the main graph.

□ uLTRA: Accurate spliced alignment of long RNA sequencing reads
>> https://academic.oup.com/bioinformatics/advance-article/doi/10.1093/bioinformatics/btab540/6327681
uLTRA, an alignment method for long RNA sequencing reads based on a novel two- pass collinear chaining algorithm. uLTRA achieves an accuracy of about 60% for exons of length 10 nucleotides or smaller and close to 90% accuracy for exons of length between 11 to 20 nucleotides.
uLTRA uses minimap2’s primary alignments for reads aligned outside the regions indexed by uLTRA and chooses the best alignment of the two aligners for reads aligned in gene regions.
uLTRA uses a novel two-pass collinear chaining algorithm. In the first pass, uLTRA uses maximal exact matches (MEMs) between reads and the transcriptome as seeds. uLTRA solves the chaining instances by highest upper bound on coverage.

□ A divide and conquer metacell algorithm for scalable scRNA-seq analysis
>> https://www.biorxiv.org/content/10.1101/2021.08.08.453314v1.full.pdf
Metacell-2, a recursive divide and conquer algorithm allowing efficient decomposition of scRNA-seq datasets of any size into small and cohesive groups of cells denoted as metacells.
Metacell-2 uses a new graph partition score to avoid time-consuming resampling and directly control metacell sizes, implements a new adaptive outlier detection module, and employs a rare-gene- module detector ensuring high sensitivity for detecting transcriptional states.

□ SIRV: Spatial inference of RNA velocity at the single-cell resolution
>> https://www.biorxiv.org/content/10.1101/2021.07.26.453774v1.full.pdf
The SIRV (Spatially Inferred RNA Velocity) algorithm consists of four major parts: (i) integration of the spatial transcriptomics and scRNA-seq datasets, (ii) predictions of un/spliced expressions, (iii) label/metadata transfer (optional), and (iv) estimation of RNA velocities within the spatial context.
SIRV calculates RNA velocity vectors for each cell that are then projected onto the two-dimensional spatial coordinates, which are then used to derive flow fields by averaging dynamics of spatially neighboring cells.

□ TraSig: Inferring cell-cell interactions from pseudotime ordering of scRNA-Seq data
>> https://www.biorxiv.org/content/10.1101/2021.07.28.454054v1.full.pdf
TraSig (Trajectory-based Signalling genes inference) identifies interacting cell types pairs and significant ligand-receptors based on the expression of genes as well as the pseudo-time ordering of cells.
TraSig uses continuous state Hidden Markov model (CSHMM). It learns a generative model on the expression data using transition states and emission probabilities, and assumes a tree structure for the trajectory and assigns cells to specific locations on its edges.

□ Bi-Directional PBWT: Efficient Haplotype Block Matching
>> https://drops.dagstuhl.de/opus/volltexte/2021/14372/pdf/LIPIcs-WABI-2021-19.pdf
Bi-directional PBWT finds blocks of matches around each variant site and the changes of matching blocks using forward and reverse PBWT at each variant site at the same time.
The time complexity of the algorithms to find matching blocks using bi-PBWT is linear to the input. It provides an efficient solution that can tolerate genotyping errors. The divergence values in the forward PBWT can be updated using the block information in the reverse PBWT.

□ DeepNano-coral: Nanopore Base Calling on the Edge
>>
DeepNano-coral, a new base caller for nanopore sequencing, which is optimized to run on the Coral Edge Tensor Processing Unit, a small USB-attached hardware accelerator.
A new design of the residual block, which is a fundamental building block of the QuartzNet speech recognition architecture and was also deployed for base calling in Bonito.
DeepNano-coral provides real-time base calling that is energy efficient. The k-blueprint-separable convolution factorizes the convolution into the two parts differently, in effect reducing the depthwise operation at the cost of increasing computation in the pointwise operation.
□ New strategies to improve minimap2 alignment accuracy
>> https://arxiv.org/pdf/2108.03515.pdf
A new heuristic to additional minimizers. If |x1 − x2| ≥ 500, minimap2 v2.22 selects ⌊|x1 − x2|/500⌋ minimizers of the lowest occurrence among minimizers between x1 and x2. And use a binary heap data structure to select minimizers of the lowest occurrence in this interval.
To see if minimap2 v2.22 could improve long INDEL alignment, running dipcall on contig-to-reference alignments and focused on INDELs longer than 1kb (real-sv-1k). v2.22 is more sensitive at comparable specificity, confirming its advantage in more contiguous alignment.
□ Co-evolutionary Distance Predictions Contain Flexibility Information
>> https://academic.oup.com/bioinformatics/advance-article-abstract/doi/10.1093/bioinformatics/btab562/6349220
The predicted distance distribution of each residue pair was analysed for local maxima of probability indicating the most likely distance or distances between a pair of residues.
Rigid residue pairs tended to have only a single local maximum in their predicted distance distributions while flexible residue pairs more often had multiple local maxima.

□ Learning Invariant Representations using Inverse Contrastive Loss
>> https://www.ncbi.nlm.nih.gov/pmc/articles/PMC8366266/
If the extraneous variable is binary, then optimizing ICL is equivalent to optimizing a regularized Maximum Mean Discrepancy divergence. The formulation of ICL can be decomposed into a sum of convex functions of the given distance metric.
These models obtained by optimizing ICL achieve significantly better invariance to the extraneous variable for a fixed desired level of accuracy. Applicability of ICL for learning invariant representations for both continuous and discrete extraneous variables.

□ A scalable algorithm for clonal reconstruction from sparse time course genomic sequencing data
>> https://www.biorxiv.org/content/10.1101/2021.08.19.457037v1.full.pdf
a novel scalable algorithm for clonal reconstruction from sparse time course data containing hundreds of novel mutations occurring at each sampled time point.
It employs a statistical method to estimate the sampling variance of VAFs derived from low coverage sequencing data and incorporated it into the maximum likelihood framework for clonal reconstruction.

□ MultiVI: deep generative model for the integration of multi-modal data
>> https://www.biorxiv.org/content/10.1101/2021.08.20.457057v1.full.pdf
MultiVI, a deep generative model probabilistic framework that leverages deep neural networks to jointly analyze scRNA, scATAC and multiomic (scRNA + scATAC) data.
MultiVI creates an informative low-dimensional latent space that reflects both chromatin and transcriptional properties even when one of the modalities is missing. MultiVI provides a batch- corrected view of the high-dimensional data, along with quantification of uncertainty.

□ MultiK: an automated tool to determine optimal cluster numbers in single-cell RNA sequencing data
>> https://genomebiology.biomedcentral.com/articles/10.1186/s13059-021-02445-5
there exist different levels of cluster resolution (i.e., multi-resolution) that are biologically relevant in the data: some clusters are more distinct (e.g., cell types), and others are less distinct but still different (such as related subtypes within a common cell type).
MultiK presents multiple diagnostic plots to assist in the determination of meaningful Ks in the data and makes objective optimal K suggestions, which encompasses both high- and low-resolution parameters.
MultiK aggregates all the clustering runs that give rise to the same K groups regardless of the resolution parameter and computes a consensus matrix. To determine several multi-scale optimal K candidates, MultiK applies a convex hull approach.
MultiK first constructs a dendrogram of the cluster centroids using hierarchical clustering and then runs SigClust on each pair of terminal clusters to determine classes and subclasses.
□ Hierarchical Bayesian models of transcriptional and translational regulation processes with delays
>> https://academic.oup.com/bioinformatics/advance-article-abstract/doi/10.1093/bioinformatics/btab618/6358716
Inferring the variability of parameters that determine gene dynamics. However, It’s complicated by the fact that the effects of many reactions are not observable directly. Unobserved reactions can be replaced w/ time delays to reduce model dimensionality and simplify inference.
a non-Markovian, hierarchical Bayesian inference framework for quantifying the variability of cellular processes within and across cells in a population. This hierarchical framework is robust and leads to improved estimates compared to its non-hierarchical counterpart.

□ scProject: Identifying Gene-wise Differences in Latent Space Projections Across Cell Types and Species in Single Cell Data
>> https://www.biorxiv.org/content/10.1101/2021.08.25.457650v1.full.pdf
scProject with projectionDrivers, a new framework to quantitatively examine latent space usage across single-cell exper- imental systems while concurrently extracting the genes driving the differential usage of the latent space across the defined testing parameters.
scProject uses unconstrained elastic net regression allowing for the use of latent spaces containing negative weights. The elastic net regression in scProject both encourages sparsity, a known feature of single-cell data, while also handling the potential for collinearity.
□ Tensor-decomposition--based unsupervised feature extraction in single-cell multiomics data analysis
>> https://www.biorxiv.org/content/10.1101/2021.08.25.457731v1.full.pdf
Singular value decomposition (SVD) was applied to individual omics profiles such that 34 individual omics profiles have common L singular value vectors.
Then, K omics profiles are formatted as an L × M × K dimensional tensor, where M is the number of single cells. Then, higher-order singular value decomposition (HOSVD), which is a type of TD, is applied to the tensor.
UMAP applied to singular value vectors attributed to single cells by HOSVD successfully generated two dimensional embedding, coincident with known classification of single cells.

□ ION: Inferring causality in biological oscillators
>> https://academic.oup.com/bioinformatics/advance-article/doi/10.1093/bioinformatics/btab623/6360457
Conventional methods manipulate one or more components experimentally to investigate the effect on others in the system. However, these are time-consuming and costly, particularly as the number of components increases.
ION infers regulations within various network structures such as a cycle, multiple cycles, and a cycle with outputs from in silico oscillatory time-series data. ION predicts hidden regulations for the pS2 promoter after estradiol treatment, guiding experimental investigation.

□ NetRAX: Accurate and Fast Maximum Likelihood Phylogenetic Network Inference
>> https://www.biorxiv.org/content/10.1101/2021.08.30.458194v1.full.pdf
NetRAX can infer maximum likelihood phylogenetic networks from partitioned multiple sequence alignments and returns the inferred networks in Extended Newick format.
NetRAX uses a greedy hill climbing approach to search for network topologies. It deploys an outer search loop to iterate over different move types and an inner search loop to search for the best-scoring network using a specific move type.

□ Ultrafast homomorphic encryption models enable secure outsourcing of genotype imputation
>> https://www.cell.com/cell-systems/fulltext/S2405-4712(21)00288-X
Homomorphic Encryption -based imputation methods enable a general modular approach. The first step is imputation model building, where imputation models are trained using the reference genotype panel w/ a set of tag variants to impute the genotypes for a set of target variants.
The second step is the secure imputation step, where the encrypted tag variant genotypes are used to predict the target genotypes by using the imputation models. Imputation model evaluation using the encrypted tag variant genotypes, is where the HE-based methods are deployed.

□ RcppML NMF: Fast and robust non-negative matrix factorization for single-cell experiments
>> https://www.biorxiv.org/content/10.1101/2021.09.01.458620v1.full.pdf
RcppML NMF, an accessible NMF implementation that is much faster than PCA and rivals the runtimes of state-of-the-art Singular Value Decomposition (SVD).
RcppML NMF uses random initialization. NMF models learned with this implementation from raw count matrices yield intuitive summaries of complex biological processes, capturing coordinated gene activity and enrichment of sample metadata.

□ Semantics in High-Dimensional Space
>> https://www.frontiersin.org/articles/10.3389/frai.2021.698809/full
If we are in a 128-dimensional, 1,000-dimensional, or 10-dimensional space, the natural sense of space, direction, or distance we have acquired poking around over our lifetime on the 2-dimensional surface of a 3-dimensional sphere do not quite cut it and risk leading us astray.
An increasing majority of the points in a hypercube lies far from the surface of the hypersphere, and any projected structure that depends on the differences in distance from the origin is lost. The structures in the vector space are partially shadowed onto the hypersphere cave.
※コメント投稿者のブログIDはブログ作成者のみに通知されます