
□ Aperiodic tidal data resource assessment and LCOE analysis of selected areas in Nigeria:
>> https://academic.oup.com/ce/advance-article/doi/10.1093/ce/zky007/5001502
The objective of this research is to examine the tidal stream resource potential of seven coastal sites using the modified continuous wavelet transform (CWT) method with a levelized cost of energy (LCOE) analysis.
□ scEpath: energy landscape-based inference of transition probabilities and cellular trajectories from single-cell transcriptomic data:
>> https://academic.oup.com/bioinformatics/article/34/12/2077/4838235
scEpath ("single-cell Energy path") allows us to identify common and specific temporal dynamics and transcriptional factor programs along branched lineages, as well as the transition probabilities that control cell fates. scEpath also performs downstream analyses including identification of marker genes and transcription factors important for specific cell clusters over pseudotime.

□ diffcyt: Differential discovery in high-dimensional cytometry via high-resolution clustering:
>> https://www.biorxiv.org/content/biorxiv/early/2018/06/18/349738.full.pdf
By default, this implementation uses the FlowSOM clustering algorithm, given its strong performance and fast runtimes (other high-resolution clustering algorithms could also be substituted). This high-resolution clustering approach instead provides a tractable ‘middle ground’ between discrete clustering and a continuum of cell populations and return results directly at the level of high-resolution clusters. A related limitation concerns the identification of cell population phenotypes: while our approach relies on visualizations, improved methods for automatic annotation and labeling of clusters may allow cell populations to be identified in a more automated manner.

□ Multi‐Omics Factor Analysis—a framework for unsupervised integration of multi‐omics data sets
>> http://msb.embopress.org/content/14/6/e8124
MOFA infers a set of (hidden) factors that capture biological and technical sources of variability. It disentangles axes of heterogeneity that are shared across multiple modalities and those specific to individual data modalities. The model is linear, which means that it can miss strongly non‐linear relationships between features within and across assays. Non‐linear extensions of MOFA may address this, although, as with any models in high‐dimensional spaces, there will be trade‐offs between model complexity, computational efficiency and interpretability.

□ htsget: a protocol for securely streaming genomic data:
>> https://academic.oup.com/bioinformatics/advance-article/doi/10.1093/bioinformatics/bty492/5040320
htsget allows a client to retrieve data overlapping a specific genomic interval and uses existing community standards such as SAM/BAM/CRAM/VCF/BCF as the on-the-wire format. Direct access to genomic loci in read or variant data over HTTP is a major improvement, but this is achieved by the means of auxiliary index files and requires that the server expose file semantics for all the data that it serves.
□ DoubletFinder: Doublet detection in single-cell RNA sequencing data using artificial nearest neighbors:
>> https://www.biorxiv.org/content/biorxiv/early/2018/06/20/352484.full.pdf
Neighborhood detection in gene expression space then identifies sequenced cells with increased probability of being doublets based on their proximity to artificial doublets. DoubletFinder robustly identifies doublets across scRNA-seq datasets with variable numbers of cells and sequencing depth, and predicts false-negative and false-positive doublets defined using conventional barcoding approaches.
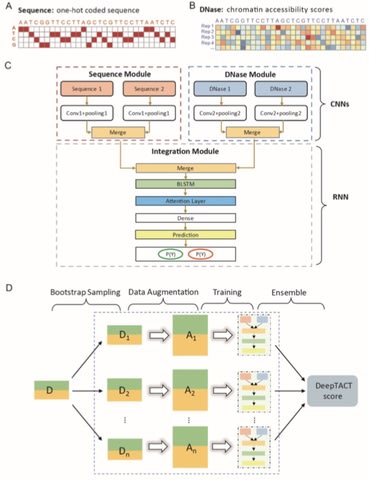
□ DeepTACT: predicting high-resolution chromatin contacts via bootstrapping deep learning:
>> https://www.biorxiv.org/content/biorxiv/early/2018/06/22/353284.full.pdf
DeepTACT improves the resolution of the high-quality promoter capture Hi-C (PCHi-C) data from multiple regulatory elements level (5-20kb) to individual regulatory element level (1kb).

□ DeepImpute: an accurate, fast and scalable deep neural network method to impute single-cell RNA-Seq data:
>> https://www.biorxiv.org/content/biorxiv/early/2018/06/22/353607.full.pdf
The architecture of DeepImpute efficiently uses dropout layers and loss functions to learn patterns in the data, allowing for accurate imputation. Overall DeepImpute yields better accuracy than other publicly available scRNA-Seq imputation methods on experimental data, as measured by mean squared error or Pearson's correlation coefficient.

□ Reparametrizing the Sigmoid Model of Gene Regulation for Bayesian Inference⋆:
>> https://www.biorxiv.org/content/biorxiv/early/2018/06/22/352070.full.pdf
The proposed reparametrization of the model implemented in the Stan probabilistic programming language makes inference over the model stable and amenable to fully Bayesian treatment with state of the art Hamiltonian Monte Carlo methods. This model aims at modelling and identifying transcriptional regulations from time series data of gene expression. While the model aims to be more general, and on determining for which genes does a known sigma factor initiate transcription.

□ A deep learning approach to pattern recognition for short DNA sequences:
>> https://www.biorxiv.org/content/biorxiv/early/2018/06/22/353474.full.pdf
DNN can predict the species of origin of individual reads more accurately than existing machine learning baselines and alignment-based methods like BWA or BLAST, achieving absolute performance within 2.0% of perfect memorization of the training inputs.
□ Deep SNP: An End-to-end Deep Neural Network with Attention-based Localization for Break-point Detection in SNP Array Genomic data:
>> https://www.biorxiv.org/content/biorxiv/early/2018/06/24/354423.full.pdf
Highly fragmented segments often occur in noisy profiles as artifacts predicted by Rawcopy and need to be merged. In case of false negative segment predictions, additional segments have to be generated and added to the final segmentation. using a bidirectional Gated Recurrent layer (GRU) and apply it on the hidden space that feature learning module learned, and apply the GRUs on the sequence of hidden activations formed by the dimension in the hidden space that represents the genomic position.

>> https://github.com/ryanlayer/samplot
SAMPLOT now supports simultanious visualization of short reads, long reads (@PacBio and @nanopore), linked and phased reads (@10xgenomics), and gene and annotation tracks. Long read (Oxford nanopore and PacBio) and linked read support: Any alignment that is longer than 1000 bp are treated as a longread, and the plot design will focus on aligned regions and gaps. Aligned regions are in orange, and gaps follow the same DEL/DUP/INV color code used for short reads. The height of the alignment is based on the size of its largest gap.

□ Ouija: A descriptive marker gene approach to single-cell pseudotime inference:
>> https://academic.oup.com/bioinformatics/advance-article-abstract/doi/10.1093/bioinformatics/bty498/5043298
this method can detect differences in the regulation timings between two genes and identify “metastable” states - discrete cell types along the continuous trajectories - that recapitulate known cell types. ouijaflow implements the probabilistic single-cell pseudotime model Ouija in Edward and Tensorflow, allowing scalable inference on large single-cell datasets. Inference is performed using reparametrization gradient variational inference.
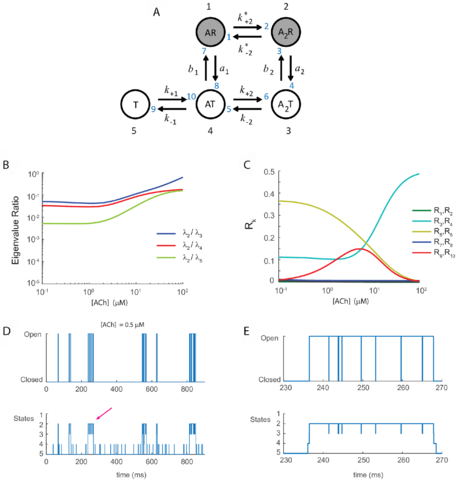
□ Stochastic shielding and edge importance for Markov chains with timescale separation:
>> http://journals.plos.org/ploscompbiol/article?id=10.1371/journal.pcbi.1006206
Stochastic shielding provides an alternative approach by simplifying the description of the noise driving the process, while preserving the Markov property, by removing from the model those fluctuations that are not directly observable. When edge importance reversal occurs, current fluctuations are dominated by a slow noise component arising from the hidden transitions.
□ The multifurcating skyline plot:
>> https://www.biorxiv.org/content/biorxiv/early/2018/06/26/356097.full.pdf
using the theory of Λ-coalescents (Beta(2α, α)-coalescents) to develop the “multifurcating skyline plot”, which estimates a piecewise constant function of effective population size through time, conditional on a time-scaled multifurcating phylogeny. if the probability measure Λ is concentrated solely at 0, then the Λ-coalescent process is strictly binary and identical to the Kingman coalescent. However, the paintbox construction scheme does not apply to this case, since ∮δ0(dx)/x2 = ∞.
□ genomeview – an extensible python-based genomics visualization engine:
>> https://www.biorxiv.org/content/biorxiv/early/2018/06/26/355636.full.pdf
genomeview includes several features intended to improve the visualization of noisy long-fragment sequencing data such as PacBio and Oxford Nanopore, most notably a quick-consensus mode that hides putative sequencing errors while retaining likely variants.

□ SAVER: gene expression recovery for single-cell RNA sequencing:
>> https://www.nature.com/articles/s41592-018-0033-z
SAVER (single-cell analysis via expression recovery), an expression recovery method for unique molecule index (UMI)-based scRNA-seq data that borrows information across genes and cells to provide accurate expression estimates for all genes. the variance is estimated through maximum likelihood assuming constant variance, Fano factor, or coefficient of variation variance structure for each gene. The posterior distribution is calculated and the posterior mean is reported as the SAVER estimate.

□ EnsembleCNV: An ensemble machine learning algorithm to identify and genotype copy number variation using SNP array data:
>> https://www.biorxiv.org/content/biorxiv/early/2018/06/27/356667.full.pdf
EnsembleCNV identifies and eliminates batch effects at raw data level; assembles individual CNV calls into CNV regions from multiple existing callers with complementary strengths by a heuristic algorithm; re-genotypes each CNVR with local likelihood model adjusted by global information across multiple CNVRs; refines CNVR boundaries by local correlation structure in copy number intensities;
□ DataRemix: a universal data transformation for optimal inference from gene expression datasets:
>> https://www.biorxiv.org/content/biorxiv/early/2018/06/27/357467.full.pdf
optimizing the transformation with respect to the downstream biological objective, this parametric framework reweighs the contribution of each hidden factor and make the biological signals visible. optimizing for trans-eQTL discovery also improves the correlation network as measured by guilt-by-association pathway prediction.
□ A deep proteome and transcriptome abundance atlas of 29 healthy human tissues:
>> https://www.biorxiv.org/content/biorxiv/early/2018/06/27/357137.full.pdf
□ StarSeeker: an automated tool for mature duplex microRNA sequence identification based on secondary structure modeling of precursor molecule:
>> https://link.springer.com/article/10.1186/s40709-018-0081-7
StarSeeker predicts the sequence of miRNA* given the precursor and the corresponding mature miRNA sequences. Using RNAfold from Vienna RNA package, StarSeeker assigns the bracket notation of the precursor to each pair and returns the corresponding miRNA* sequence, in accordance to the theory about microRNA biogenesis.
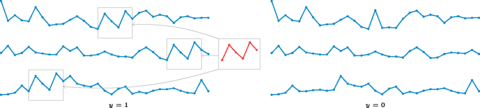
□ Association mapping in biomedical time series via statistically significant shapelet mining:
>> https://academic.oup.com/bioinformatics/article/34/13/i438/5045733
The significance of a shapelet is evaluated while considering the problem of multiple hypothesis testing and mitigating it by efficiently pruning untestable shapelet candidates with Tarone’s method.

□ SCuPhr: A Probabilistic Framework for Cell Lineage Tree Reconstruction:
>> https://www.biorxiv.org/content/biorxiv/early/2018/06/29/357442.full.pdf
The model is well suited for full Bayesian analysis by introducing prior probabilities for key parameters as well as maximum a posteriori estimation using expectation maximization algorithm. the model contains variables associated with pairs of loci, of which one is homozygous and the other heterozygous, and has the capacity to perform Bayesian probabilistic read phasing.
□ Nanopore sequencing of long ribosomal DNA amplicons enables portable and simple biodiversity assessments with high phylogenetic resolution across broad taxonomic scale:
>> https://www.biorxiv.org/content/early/2018/06/29/358572
□ "Gene activation precedes DNA demethylation in response to infection in human dendritic cells". We show that the losses in DNA methylation observed in response to infection are a downstream consequence of TF binding.
>> https://goo.gl/xnLUBV
□ Self-similarity in the Foundations (incl. set theory and category theory):
>> https://arxiv.org/pdf/1806.11310.pdf
The work in foundational category theory consists in the formulation of a novel algebraic set theory which is proved to be equiconsistent to New Foundations (NF), and which can be modulated to correspond to intuitionistic or classical NF, with or without atoms.
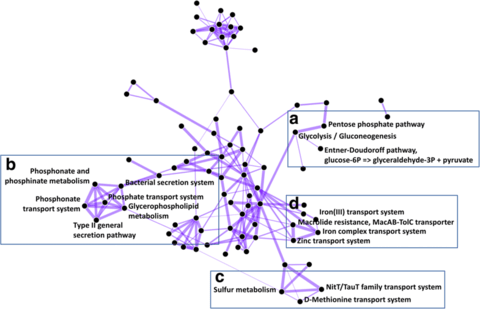
□ PathCORE-T: identifying and visualizing globally co-occurring pathways in large transcriptomic compendia:
>> https://biodatamining.biomedcentral.com/articles/10.1186/s13040-018-0175-7
PathCORE-T creates and displays the network of globally co-occurring pathways based on features observed in a machine learning analysis of gene expression data.

□ A graph-based approach to diploid genome assembly:
>> https://academic.oup.com/bioinformatics/article/34/13/i105/5045759
the effectiveness of this method on a pseudo-diploid genome and show that require 50× coverage Illumina data and 10× PacBio data to generate accurate and complete assemblies.
□ Mango: Distributed Visualization for Genomic Analysis:
>> https://www.biorxiv.org/content/biorxiv/early/2018/07/03/360842.1.full.pdf
Mango is a genomic sequence visualization and analysis platform that removes these constraints regarding scalability and staticity by leveraging the power of multi-node compute clusters in the cloud to allow interactive analysis over terabytes of sequencing data.
□ A new model explaining the origin of different topologies in interaction networks:
>> https://www.biorxiv.org/content/biorxiv/early/2018/07/05/362871.full.pdf
the intensity of trade-offs in the performance of each consumer species on different resource species is the main factor driving network topology. the model generates predictions consistent with ecological and evolutionary theories and real-world observations. Therefore, it supports the IHS as a useful conceptual framework to study the architecture of interaction networks.
□ McImpute: Matrix completion based imputation for single cell RNA-seq data:
>> https://www.biorxiv.org/content/biorxiv/early/2018/07/05/361980.full.pdf
The transcript which is not detected because of failing to get amplified in sequencing step, essentially corresponds to a “false zero” in the finally observed count data and needs to be imputed. all imputation strategies successfully impute the “true zeros” while, as the gene expression amplifies, un-imputed matrix still exhibits large fraction of zeros, which essentially correspond to dropouts and only mcImpute and scImpute are able to curtail the fraction of zeros.
※コメント投稿者のブログIDはブログ作成者のみに通知されます