
(Art by Dimitris Ladopoulos)



□ Chronocell: Trajectory inference from single-cell genomics data with a process time model
>> https://www.biorxiv.org/content/10.1101/2024.01.26.577510v1
Chronocell provides a biophysical formulation of trajectories built on cell state transitions. Chronocell interpolates between trajectory inference, when cell states lie on a continuum, and clustering, when cells cluster into discrete states.
By gradually changing sampling distributions from a uniform distribution to a Gaussian with a random mean, they generates dataset with sampling distributions that exhibit decreasing levels of uniformity, which was quantified using entropy.
The trajectory model of Chronocell is associated with a trajectory structure that specifies the states each lineage. A trajectory model degenerates into a Poisson mixtures in the fast dynamic limit where the dynamical timescale is much smaller that the cell sampling timescale.

□ scGND: Graph neural diffusion model enhances single-cell RNA-seq analysis
>> https://www.biorxiv.org/content/10.1101/2024.01.28.577667v1
scGND (Single Cell Graph Neural Diffusion), a physics-informed graph generative model that aims to represent the dynamics of information flow in a cell graph using the graph neural diffusion algorithm. sGND simulates a diffusion process that mirrors physical diffusion.
SCGND employs an attention mechanism to facilitate the diffusion process. In scGND, the attention matrix is given a physical interpretation of diffusivity, determining the rate of information spread on the cell graph.
scGND leverages two established concepts from diffusion theory: local and global equilibrium effects. The local equilibrium effect emphasizes the discreteness of ScRNA-seq data, by isolating each intrinsic cell cluster, making it more distinct from others.
Conversely, the global equilibrium effect focuses on the continuity of scRNA-seq data, enhancing the interconnections between all intrinsic cell clusters. Therefore, scGND offers both discrete and continuous perspectives in one diffusion process.

□ A Biophysical Model for ATAC-seq Data Analysis
>> https://www.biorxiv.org/content/10.1101/2024.01.25.577262v1
A model for chromatin dynamics, inspired by the Ising model from physics. Ising models have been used to analyze ChIP-chip data. A hidden Markov model (HMM) treats chromosomally consecutive probes in a microarray as neighbors in a 1-dimensional Ising chain.
The hidden state of the system is a specific configuration of enriched vs non-enriched probes in the chain.
In the Ising model, the external magnetic field is assumed to be constant for all spins in the lattice. However, inspection of the first order moments for chromatin accessibility from ATAC-seq data suggests that this feature of the model is not appropriate in this context.
Therefore, they allow the ratio of chromatin opening / closing rates to vary between sites, giving a separate field strength parameter per site, plus one correlation parameter e.g., a 7-parameter model to describe the chromatin aspect of the biological system for a 6-site locus.

□ PLIGHT: Assessing and mitigating privacy risks of sparse, noisy genotypes by local alignment to haplotype databases
>> https://www.ncbi.nlm.nih.gov/pmc/articles/PMC10760520/
PLIGHT (Privacy Leakage by Inference across Genotypic HMM Trajectories) uses population-genetics-based hidden Markov models (HMMs) of recombination and mutation to find piecewise alignment of small, noisy SNP sets to reference haplotype databases.
PLIGHT provides a visualization of all trajectories across the observed loci, and the logarithms of the joint probabilities of observing the query SNPs for: (a) the HMM, and models where (b) SNPs are independent and satisfy Hardy-Weinberg equilibrium.

□ DeepVelo: deep learning extends RNA velocity to multi-lineage systems with cell-specific kinetics
>> https://genomebiology.biomedcentral.com/articles/10.1186/s13059-023-03148-9
DeepVelo is optimized using a newly introduced continuity framework, resulting in an approach that is unbiased from pre-defined kinetic patterns. Empowered by graph convolutional networks (GCN), DeepVelo infers gene-specific and cell-specific RNA splicing and degradation rates.
DeepVelo enables accurate quantification of time-dependent and multifaceted gene dynamics. DeepVelo is able to model RNA velocity for differentiation dynamics of high complexity, particularly for cell populations with heterogeneous cell-types and multiple lineages.

□ InClust+: the deep generative framework with mask modules for multimodal data integration, imputation, and cross-modal generation
>> https://bmcbioinformatics.biomedcentral.com/articles/10.1186/s12859-024-05656-2
inClust+, a deep generative framework for the multi-omics. inClust+ is specific for transcriptome data, and augmented with two mask modules designed for multimodal data processing: an input-mask module in front of the encoder and an output-mask module behind the decoder.
InClust+ integrates scRNA-seq and MERFISH data from similar cell populations, and to impute MERFISH data based on scRNA-seq data. inClust+ integrates data from different modalities in the latent space. And the vector arithmetic further integrates data from different batches.

□ k-nonical space: sketching with reverse complements
>> https://www.biorxiv.org/content/10.1101/2024.01.25.577301v1
The canonicalization optimization problem that transforms an existing sketching method into one that is symmetric (k-mer and its reverse complement identically) while respecting the same window guarantee as the original method and not introducing any additional sketching deserts.
An integer linear programming (ILP) formulation for a variant of the MFVS problem that (a) accepts a maximum remaining path length constraint, (b) works with symmetries such as the reverse complement, and (c) minimizes the expected remaining path length after decycling.
There is an asymmetry between the sketching methods with a context used in practice (e.g., minimizers) and the context-free methods (e.g., syncmers).
Because minimizers always select a k-mer in every context, it has the same window guarantee before and after canonicalization and is therefore immune to the detrimental effects. Every context-free method is susceptible to not having any window guarantee in k-nonical space.

□ SGTCCA-Net: A Generalized Higher-order Correlation Analysis Framework for Multi-Omics Network Inference
>> https://www.biorxiv.org/content/10.1101/2024.01.22.576667v1
SGTCCA-Net (Sparse Generalized Tensor Canonical Correlation Analysis Network Inference) is adaptable for exploring diverse correlation structures within multi-omics data and is able to construct complex multi-omics networks in a two-dimensional space.
SGTCCA-Net achieves high signal feature identification accuracy even with only 100 subjects in the presence and absence of different phenotype-specific correlation structures and provides nearly-perfect prediction when the number of subjects doubles.

□ RGVP: Implicit Gaussian process representation of vector fields over arbitrary latent manifolds
>> https://arxiv.org/abs/2309.16746
RVGP (Riemannian manifold vector field GP), a generalisation of GPs for learning vector signals over latent Riemannian manifolds. RVGP encodes the manifold and vector field's smoothness as inductive biases, enabling out-of-sample predictions from sparse or obscured data.
RVGP uses positional encoding with eigenfunctions of the connection Laplacian, associated with the tangent bundle.RVGP possesses global regularity over the manifold, which allows it to super-resolve and inpaint vector fields while preserving singularities.

□ NEAR: Neural Embeddings for Amino acid Relationships
>> https://www.biorxiv.org/content/10.1101/2024.01.25.577287v1
NEAR's neural embedding model computes per-residue embeddings for target and query protein sequences, and identifies alignment candidates with a pipeline consisting of k-NN search, filtration, and neighbor aggregation.
NEAR's ResNet embedding model is trained using an N-pairs loss function guided by sequence alignments generated by the widely used HMMER3 tool.
NEAR is implemented as a 1D Residual Convolutional Neural Network. A batch of sequences is initially embedded as a [batch x 256 Xseq length tensor using a context-unaware residue embedding layer. The tensor is then passed through 8 residual blocks.
NEAR initiates search by computing residue embeddings for a set of target proteins. These embeddings are used to generate a search index with the FAISS library for efficient similarity search in high dimensions.

□ MetageNN: a memory-efficient neural network taxonomic classifier robust to sequencing errors and missing genomes
>> https://www.biorxiv.org/content/10.1101/2023.12.01.569515v1
MetageNN overcomes the limitation of not having long-read sequencing-based training data for all organisms by making predictions based on k-mer profiles of sequences collected from a large genome database.
MetageNN utilizes the extensive collection of reference genomes available to sample long sequences. MetageNN relies on computing short-k-mer profiles (6mers), which are more robust to sequencing errors and are used as input to the MetageNN architecture.

□ cloudrnaSPAdes: Isoform assembly using bulk barcoded RNA sequencing data
>> https://academic.oup.com/bioinformatics/advance-article/doi/10.1093/bioinformatics/btad781/7585775
cloudraSPAdes, a novel tool for de novo assembly of full-length isoforms from barcoded RNA-seq data. It constructs a single assembly graph using the entire set of input reads and further derives paths for each read cloud, closing gaps and fixing sequencing errors in the process.
The cloudraSPAdes algorithm processes each read cloud individually and exploits barcode-specific edge coverage, while using the assembly graph constructed from all read clouds combined.

□ scDisInFact: disentangled learning for integration and prediction of multi-batch multi-condition single-cell RNA-sequencing data
>> https://www.nature.com/articles/s41467-024-45227-w
scDisInFact (single cell disentangled Integration preserving condition-specific Factors) can perform all three tasks: batch effect removal, condition-associated key genes (CKGs) detection, and perturbation prediction on multi-batch multi-condition scRNA-seq dataset.
scDisInFact is designed based on a variational autoencoder (VAE) framework. The encoder networks encode the high dimensional gene expression data of each cell into a disentangled set of latent factors, and the decoder network reconstructs GE data from the latent factors.
scDisInFact has multiple encoder networks, where each encoder learns independent latent factors from the data. scDisInFact disentangles the gene expression data into the shared biological factors, unshared biological factors, and technical batch effect.

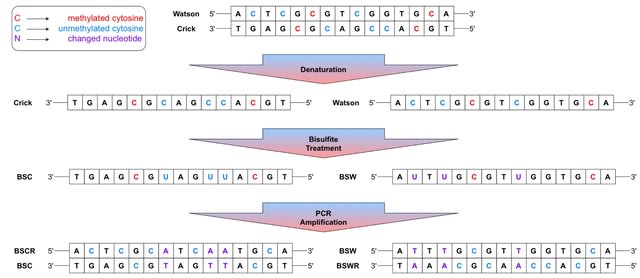
□ ARYANA-BS: Context-Aware Alignment of Bisulfite-Sequencing Reads
>> https://www.biorxiv.org/content/10.1101/2024.01.20.576080v1
ARYANA uses a seed-and-extend paradigm for aligning short reads of genomic DNA. It creates a Burrows-Wheeler Transform (BWT) index of the genome using the BWA engine, partitions the reference genome into equal-sized windows, and finds maximal substrings.
ARYANA-BS departs from conventional DNA aligners by considering base alterations in BS reads within its alignment engine. ARYANA-BS generates five indexes from the reference, aligns each read to all indexes, and selects the hit with the minimum penalty.

□ Jointly benchmarking small and structural variant calls with vcfdist
>> https://www.biorxiv.org/content/10.1101/2024.01.23.575922v1
Extending vefdist to be the first tool to jointly evaluate phased SNP, INDEL, and SV calls in whole genomes. Doing so required major internal restructuring and improvements to vefdist to overcome scalability issues relating to memory and compute requirements.
vedist's alignment-based analysis obtains similar accuracy results to Truvari-MAFFT and Truvari-WFA, but is able to scale to evaluating whole-genome datasets.
Differing variant representations cause variants to appear incorrectly phased, though they are not. These false positive flip errors then lead to false positive switch errors. vefdist is able to avoid these errors in phasing analysis by using alignment-based variant comparison.

□ scPerturb: harmonized single-cell perturbation data
>> https://www.nature.com/articles/s41592-023-02144-y
scPerturb uses E-statistics for perturbation effect quantification and significance testing. E-distance is a general distance measure for single cell data.
The E-distance relates the distance between cells across the groups ("signal"), to the width of each distribution ("noise"). If this distance is large, distributions are distinguishable, and the corresponding perturbation has a strong effect.
A low E-distance indicates that a perturbation did not induce a large shift in expression profiles, reflecting either technical problems in the experiment, ineffectiveness of the perturbation, or perturbation resistance.
This work provides an information resource and guide for researchers working with single-cell perturbation data, highlights conceptual considerations for new experiments, and makes concrete recommendations for optimal cell counts and read depth.

□ COMEBin: Effective binning of metagenomic contigs using contrastive multi-view representation learning
>> https://www.nature.com/articles/s41467-023-44290-z
COMEBin utilizes data augmentation to generate multiple fragments (views) of each contig and obtains high-quality embeddings of heterogeneous features (sequence coverage and k-mer distribution) through contrastive learning.
COMEBin incorporates a “Coverage module” to obtain fixed-dimensional coverage embeddings, which enhances its performance across datasets with varying numbers of sequencing samples.

□ Many-core algorithms for high-dimensional gradients on phylogenetic trees
>> https://academic.oup.com/bioinformatics/advance-article/doi/10.1093/bioinformatics/btae030/7577857
Hamiltonian Monte Carlo (HMC) requires repeated calculation of the gradient of the data log-likelihood with respect to (wrt) all branch-length-specific (BLS) parameters that traditionally takes O(N2) operations using the standard pruning algorithm.
The CPU-GPU implementation of this approach makes the calculation of the gradient computationally tractable for nucleotide-based models but falls short in performance for larger state-space size models, such as Markov-modulated and codon models.

□ GRAPHDeep: Assembling spatial clustering framework for heterogeneous spatial transcriptomics data
>> https://academic.oup.com/bioinformatics/advance-article/doi/10.1093/bioinformatics/btae023/7577854
GRAPHDeep, is presented to aggregate two graph deep learning modules (i.e., Variational Graph Auto-Encoder and Deep Graph Infomax) and twenty graph neural networks for spatial domains discrimination.
GRAPHDeep integrates two robust graph deep learning (GDL) modules, VGAE and DGI, utilizing twenty GNNs as encoders and decoders. This encompasses a total of forty distinct GNN-based frameworks, each contributing to the spatial clustering objective.

□ A graph clustering algorithm for detection and genotyping of structural variants from long reads
>> https://academic.oup.com/gigascience/article/doi/10.1093/gigascience/giad112/7516265
An accurate and efficient algorithm to predict germline SVs from long-read sequencing data. The algorithm starts collecting evidence of SVs from read alignments. Signatures are clustered based on a Euclidean graph with coordinates calculated from lengths and genomic positions.
Clustering is performed by the DBSCAN algorithm, which provides the advantage of delimiting clusters with high resolution. Clusters are transformed into SVs and a Bayesian model allows to precisely genotype SVs based on their supporting evidence.

□ Modes and motifs in multicellular communication
>> https://www.sciencedirect.com/science/article/pii/S2405471223003617
Key signaling pathways only use a limited number of all possible expression profiles, suggesting that they operate in specific modes. In analogy to musical modes, while thousands of note combinations are possible, chords are selected from a given scale.
Chords from different scales can be independently combined to generate a composition, similar to the use of pathway modes and motifs in different cell states.

□ FateNet: an integration of dynamical systems and deep learning for cell fate prediction
>> https://www.biorxiv.org/content/10.1101/2024.01.16.575913v1
FateNet leams to predict and distinguish different bifurcations in pseudotime simulations of a 'universe' of different dynamical systems.
FateNet takes in all preceding data and assigns a probability for a fold, transcritical and pitchfork bifurcation, and a probability for no bifurcation (null). FateNet successfully signals the approach of a fold and a pitchfork bifurcation in the gene regulatory network.

□ SURGE: uncovering context-specific genetic-regulation of gene expression from single-cell RNA sequencing using latent-factor models
>> https://genomebiology.biomedcentral.com/articles/10.1186/s13059-023-03152-z
SURGE (Single-cell Unsupervised Regulation of Gene Expression), a novel probabilistic model that uses matrix factorization to learn a continuous representation of the cellular contexts that modulate genetic effects.
SURGE leverages information across genome-wide variant-gene pairs to jointly learn both a continuous representation of the latent cellular contexts defining each measurement.
SURGE allows for any individual measurement to be defined by multiple, overlapping contexts. From an alternative but equivalent lens, SURGE discovers the latent contexts whose linear interaction with genotype explains the most variation in gene expression levels.

□ STAR+WASP reduces reference bias in the allele-specific mapping of RNA-seq reads
>> https://www.biorxiv.org/content/10.1101/2024.01.21.576391v1
The main bottleneck of the WASP's original implementation is its multistep nature, which requires writing and reading BAM files twice. To mitigate this issue, they reimplemented the WASP algorithm inside their RNA-seq aligner STAR.
STAR+WASP alignments were considerably faster (6.5 to 10.5 times) than WASP. While STAR+WASP and WASP both use STAR for the read alignment to the genome, the on-the-fly implementation of the WASP algorithm in STAR+WASP allows for much faster re-mapping and filtering of the reads.

□ scaDA: A Novel Statistical Method for Differential Analysis of Single-Cell Chromatin Accessibility Sequencing Data
>> https://www.biorxiv.org/content/10.1101/2024.01.21.576570v1
scaDA (Single-Cell ATAC-seq Differential Chromatin Analysis) is based on ZINB model for scATAC-seq DA analysis. scaDA focuses on testing distribution difference in a composite hypothesis, while most existing methods only focus on testing mean difference.
scaDA improves the parameter estimation by leveraging an empirical Bayes approach for dispersion shrinkage and iterative estimation. scaDA is superior to both ZINB-based likelihood ratio tests and published methods by achieving the highest power and best FDR control.

□ MAGE: Metafounders assisted genomic estimation of breeding value, a novel Additive-Dominance Single-Step model in crossbreeding systems
>> https://academic.oup.com/bioinformatics/advance-article/doi/10.1093/bioinformatics/btae044/7588872
MAGE is a genomic relationship matrix calculation tool designed for livestock and poultry populations. It can perform integrated calculations for the kinship relationships of multiple unrelated populations and their hybrid offspring.

□ HiPhase: Jointly phasing small, structural, and tandem repeat variants from HiFi sequencing
>> https://academic.oup.com/bioinformatics/advance-article/doi/10.1093/bioinformatics/btae042/7588891
HiPhase uses two novel approaches to solve the phasing problem: dual mode allele assignment and a phasing algorithm based on the A* search algorithm.
HiPhase breaks the phasing problem into: phase block generation, allele assignment, and diplotype solving. HiPhase collapses mappings with the same read name into a single entry. This allows HiPhase to cross deletion events and reference gaps bridged by split read mappings.

□ A simple refined DNA minimizer operator enables twofold faster computation
>> https://academic.oup.com/bioinformatics/advance-article/doi/10.1093/bioinformatics/btae045/7588893
A simple minimizer operator as a refinement of the standard canonical minimizer. It takes only a few operations to compute. It can improve the k-mer repetitiveness, especially for the lexicographic order. It applies to other selection schemes of total orders (e.g. random orders).

□ Fast computation of the eigensystem of genomic similarity matrices
>> https://bmcbioinformatics.biomedcentral.com/articles/10.1186/s12859-024-05650-8
A unified way to express the covariance matrix, the weighted Jaccard matrix, and the genomic relationship matrix which allows one to efficiently compute their eigenvectors in sparse matrix algebra using an adaptation of a fast SVD algorithm.
Notably, the only requirement for the proposed Algorithm to work efficiently is the existence of efficient row-wise and column-wise subtraction and multiplication operations of a vector with a sparse matrix.

□ GeneSelectR: An R Package Workflow for Enhanced Feature Selection from RNA Sequencing Data
>> https://www.biorxiv.org/content/10.1101/2024.01.22.576646v1
With GeneSelectR, features can be selected from a normalized RNAseq dataset with a variety of ML methods and user-defined parameters. This is followed by an assessment of their biological relevance with Gene Ontology (GO) enrichment analysis, along with a semantic similarity.
Similarity coefficients and fractions of the GO terms of interest are calculated. With this, GeneSelectR optimizes ML performance and rigorously assesses the biological relevance of the various lists, offering a means to prioritize feature lists with regard to the biological question.

□ Intrinsic-Dimension analysis for guiding dimensionality reduction and data fusion in multi-omics data processing
>> https://www.biorxiv.org/content/10.1101/2024.01.23.576822v1
Leveraging the intrinsic dimensionality of each view in a multi-modal dataset to define the dimensionality of the lower-dimensional space where the view is transformed by dimensionality reduction algorithms.
A novel application of block-analysis leverages any of the most promising id estimators and obtain an unbiased id-estimate of the views in a multi-modal dataset.
An automatic analysis of the block-id distribution computed by the block-analysis to detect feature noise and redundancy contributing to the curse of dimensionality and evidence the need to apply a view-specific dimensionality reduction phase prior to any subsequent analysis.