
(Created with Midjourney v6.0 ALPHA)

□ Stellarscope: A single-cell transposable element atlas of human cell identity
>> https://www.biorxiv.org/content/10.1101/2023.12.28.573568v1
Stellarscope (Single cell Transposable Element Locus Level Analysis of scRNA Sequencing), a scRNA-seq-based computational pipeline for characterizing cell identity. Stellarscope reassigns multi-mapped reads to specific genomic loci using an expectation-maximization algorithm.
Stellarscope provides a variety of reassignment strategies incl. filtering based on a threshold, excluding fragments with multiple optimal alignments, and randomly selecting from multiple optimal alignments; these criteria result in a different number of excluded alignments.
Stellarscope implements a generative model of single cell RNA-seq that rescales alignment probabilities for independently aligned reads based on the cumulative weights of all alignments, and uses the posterior probability matrix to reassign ambiguous fragments.

□ FinaleMe: Predicting DNA methylation by the fragmentation patterns of plasma cell-free DNA
>> https://www.biorxiv.org/content/10.1101/2024.01.02.573710v1
FinaleMe (FragmentatIoN AnaLysis of cEll-free DNA Methylation), to predict the DNA methylation status in each CpG at each cfDNA fragment and obtain the continuous DNA methylation level at CpG sites, mostly accurate in CpG rich regions.
FinaleMe is a non-homogeneous Hidden Markov Model. It incorporates the distance between CpG sites into the model and utilizes the following three features: fragment length, normalized coverage, and the distance of each CpG site to the center of the DNA fragment.

□ ECOLE: Learning to call copy number variants on whole exome sequencing data
>> https://www.nature.com/articles/s41467-023-44116-y
ECOLE (Exome-based COpy number variation calling LEarner) is based on a variant of the transformer model. ECOLE processes the read-depth signal over each exon. It learns which parts of the signal need to be focused on and in which context (i.e., chromosome) to call a CNV.
ECOLE uses the high-confidence calls obtained on the matched WGS samples as the semi-ground truth. ECOLE employs a multi-head attention mechanism which means multiple attentions are calculated over the signal which is concatenated and transformed into the 192 x 1001 dimensions.

□ Probabilistic Modeling for Sequences of Sets in Continuous-Time
>> https://arxiv.org/abs/2312.15045
A general framework for modeling set-valued data in continuous-time, compatible with any intensity-based recurrent neural point process model, where event types are subsets of a discrete set.
Their simplest baseline uses a homogeneous Poisson model as the temporal component and a static Bernoulli model for the set distribution (where the Bernoulli probabilities correspond to the marginal probabilities in the dataset), referred to below as the StaticB-Poisson model.
This simple baseline provides useful context for evaluating the effectiveness of more complex models for set-valued data over time. For the temporal component they use the Neural Hawkes (NH) model as a specific instantiation of the recurrent MTPP component.
In the Bernoulli variants of this model this is coupled with the Dynamic Bernoulli model for the set-component or the marginal Bernoulli option as a baseline (same model for sets as the Poisson baseline), referred as DynamicB-NH and StaticB-NH.

□ Gradient Flossing: Improving Gradient Descent through Dynamic Control of Jacobians
>> https://arxiv.org/abs/2312.17306
Gradient Flossing is based on a recently described link between the gradients of backpropagation through time and Lyapunov exponents, which are the time-averaged logarithms of the singular values of the long-term Jacobian.
Gradient flossing regularizes one or several Lyapunov exponents to keep them close to zero. This improves not only the error gradient norm but also the condition number of the long-term Jacobian. As a result, error signals can be propagated back over longer time horizons.

□ UVAE: Integration of Heterogeneous Unpaired Data with Imbalanced Classes
>> https://www.biorxiv.org/content/10.1101/2023.12.18.572157v1
UVAE (Unbiasing Variational Autoencoder), a VAE-based method capable of integrating and normalising unpaired, partially annotated data streams, thus addressing these challenges.
UVAE separates the confounding factor variability from the shared latent space, transforming heterogeneous datasets into a unified, homogeneous data stream, while performing simulatenous normalisation, merging, and class inference using stable non-adversarial learning objectives.

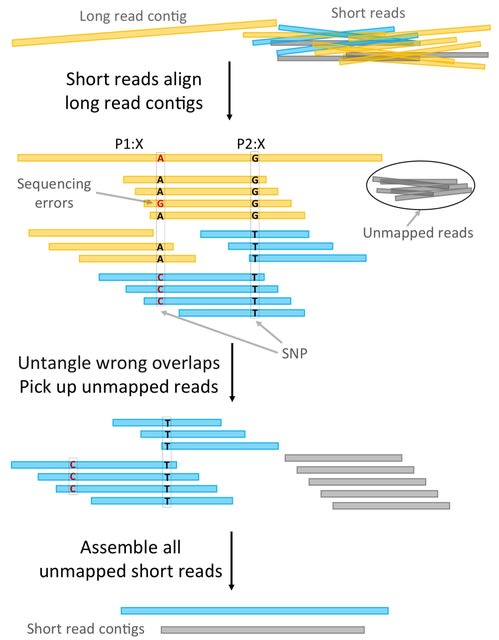
□ HyLight: Strain aware assembly of low coverage metagenomes
>> https://www.biorxiv.org/content/10.1101/2023.12.22.572963v1
HyLight, a novel approach to push the limits of strain-aware metagenome assembly in a substantial manner. HyLight is based on de novo hybrid assembly, characterized by integrating both long / short, and next-generation sequencing reads during the assembly process.
HyLight is rooted in a "cross hybrid" strategy: it assembles long reads using short reads as auxiliary source of data, and vice versa assembles short reads assisted by long read information. HyLight employs overlap graphs as the driving underlying data structure.
HyLight realizes that the presence of long reads renders usage of de Bruijn graphs obsolete. While this is understood for long read assemblies as overlap graphs have regained a prominent role when processing long reads this may be somewhat surprising when considering short reads.
HyLight incorporates a filtering step that identifies mistaken (strain-unaware) overlaps and removes them from the graphs. The filtering step prevents the incorrect compression of strain-specific variation into contigs that mistakenly connect sequence from different strains.


□ BATH: Sensitive and error-tolerant annotation of protein-coding DNA
>> https://www.biorxiv.org/content/10.1101/2023.12.31.573773v1
BATH, a tool for highly sensitive annotation of protein-coding DNA based on direct alignment of that DNA to a database of protein sequences or profile hidden Markov models (pHMMs).
BATH is built on top of the HMMER3 code base, and its core functionality is to provide full HMMER3 sensitivity w/ automatic management of 6-frame codon translation. BATH introduces novel frameshift-aware algorithms to detect frameshift-inducing nucleotide insertions / deletions.

□ GCNFORMER: graph convolutional network and transformer for predicting lncRNA-disease associations
>> https://bmcbioinformatics.biomedcentral.com/articles/10.1186/s12859-023-05625-1
GCNFORMER, a novel convolutional network and transformer-based LDA prediction model that constructs a graph relationship adjacency matrix based on the intraclass and interclass relationships between lncRNA, miRNA and disease.
In GCNFORMER model, graph convolutional network can effectively capture the topology and interactions in lncRNA-disease association network, while transformer can extract the contextual information under the complex relationships.

□ scLANE: Interpretable trajectory inference with single-cell Linear Adaptive Negative-binomial Expression testing
>> https://www.biorxiv.org/content/10.1101/2023.12.19.572477v1
scLANE testing, a negative-binomial generalized linear model (GLM) framework for modeling nonlinear relationships while accounting for correlation structures inherent to multi-sample scRNA-seq experiments.
The scLANE framework is an extension of the Multivariate Adapative Regression Splines (MARS) method, which builds nonlinear models out of piecewise linear components. scLANE can be used downstream of any pseudotemporal ordering or RNA velocity estimation method.
Truncated power basis splines are chosen empirically per-gene, per-lineage, providing results that are specific to each gene's dynamics across each biological subprocess - an improvement on methods that use a common number of equidistant knots for all genes.
The coefficients generated by scLANE carry the same multiplicative interpretation as any GLM, providing a quantitative measure and significance test of the relationship of pseudotime with gene expression over empirically selected pseudotime intervals from each lineage.

□ GSDensity: Pathway centric analysis for single-cell RNA-seq and spatial transcriptomics data
>> https://www.nature.com/articles/s41467-023-44206-x
GSDensity uses multiple correspondence analysis (MCA) to co-embed cells and genes into a latent space and quantifies the overall variation of pathway activity levels across cells by estimating the density of the pathway genes in the latent space.
GSDensity calculates pathway activity for each cell using network propagation in a nearest-neighbor cell-gene graph, with pathway genes used as seeds for random walks.


□ Hamiltonian truncation tensor networks for quantum field theories
>> https://scirate.com/arxiv/2312.12506
Hamiltonian truncation tensor networks uses matrix product operator representations of interactions in momentum space, thus avoiding the issues of lattice discretisation and reducing significantly the computational cost of simulation compared to exact diagonalisation.
Hamiltonian truncation defines the Hilbert space basis and construct the interacting part. For the mS model the free part is a massive boson model, which in momentum space reduces to an infinite set of independent harmonic oscillator modes.

□ Boolean TQFTs with accumulating defects, sofic systems, and automata for infinite words
>> https://arxiv.org/abs/2312.17033
They established a relationship between automata and one-dimensional Boolean Topological Quantum Field Theories (TQFTs), as well as the universal construction for Boolean topological theories in one dimension.
It is clear that it has a well-defined evaluation, independent of how the word is chopped into several intervals with finitely-many defects and one interval with infinitely-many defects, when presenting the floating interval as the composition of elementary morphisms.
To define a TQFT valued in the category of free B-modules, one needs suitable versions of automata and infinite words (w-automata) to account for various types of boundary behaviour at inner endpoints of cobordisms.
A Z-invariant subset of Σ^Z is called an infinite language (a language of infinite words). An infinite language is called closed if the corresponding subset is closed in Σ^Z. Closed infinite languages are in a bijection with shift spaces.

□ Quantification of cell phenotype transition manifolds with information geometry
>> https://www.biorxiv.org/content/10.1101/2023.12.28.573500v1
A novel approach to quantitatively analyze low-dimensional manifolds from single cell data. Transform each single cell's sequencing data into a multivariate Gaussian distribution, calculate the Fisher information of each cell and quantify the manifold of Cell Phenotype Transition.
Using a vector field learning method that is trained with sparse vector data pairs to learn a vector value function in a function Hilbert space.
We can define the Fisher metric on pre-defined variables such as eigengenes, using the reproducing kernel Hilbert space method (RKHS) or neural networks with backward propagation.
As RNA velocity reflects the direction of single cell along the path of CPT in the gene expression space, the information velocity of single cell represents the speed of information variation along the transition path of Cell Phenotype Transition.


□ Four-Dimensional-Spacetime Atomistic Artificial Intelligence Models
>> https://pubs.acs.org/doi/10.1021/acs.jpclett.3c01592
The 4D-spacetime GICnet model, which for the given initial conditions (nuclear positions and velocities at time zero) can predict nuclear positions and velocities as a continuous function of time up to the distant future.
Such models of molecules can be unrolled in the time dimension to yield longtime high-resolution molecular dynamics trajectories with high efficiency and accuracy.
4D-spacetime models can make predictions for different times in any order and do not need a stepwise evaluation of forces and integration of the equations of motions at discretized time steps, which is a major advance over traditional, cost-inefficient molecular dynamics.

□ Complexity And Ergodicity In Chaos Game Representation Of Genomic Sequences
>> https://www.biorxiv.org/content/10.1101/2023.12.30.573653v1
The Chaos Game Representation (CGR) transforms a DNA sequence into a visual representation that exhibits personalized characteristics unique to that specific sequence.
An ergodic system explores all accessible states and, in the long run, provides a representative sample of its entire state space. In the analysis of biological sequences like DNA or protein sequences, ergodic theory facilitates the exploration of the distribution of elements.
A DNA sequence can be transformed into a sequence of Bernoulli trials, specifically, a sequence composed of two symbols Xy and X2, where each nucleotide corresponds to an element of the transformed sequence.
CGR visually represents DNA sequences in a fractal-like pattern. In the chaos game representation of genomic sequences, each nucleotide is associated with a specific position in a coordinate system. The algorithm proceeds by iteratively plotting points based on the sequence.

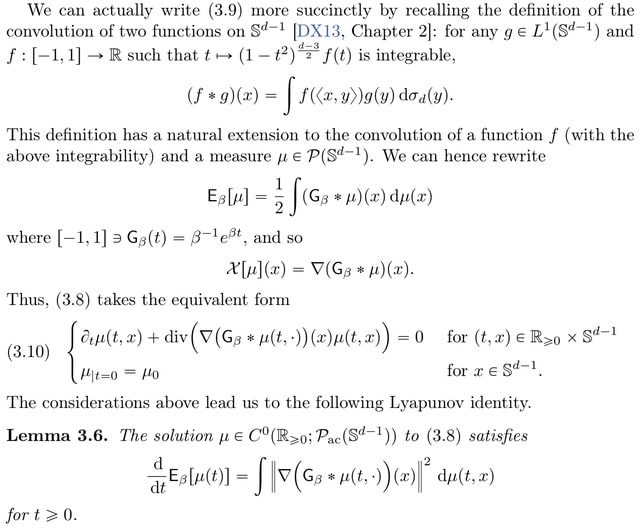
□ A mathematical perspective on Transformers
>> https://arxiv.org/abs/2312.10794
Transformers are in fact flow maps on P(R^d), the space of probability measures over R^d. Transformers evolve a mean-field interacting particle system. Every particle follows the flow of a vector field which depends on the empirical measure of all particles.
The structure of these interacting particle systems allows one to draw concrete connections to established topics in mathematics, including nonlinear transport equations, Wasserstein gradient flows, collective behavior mod-els, and optimal configurations of points on spheres.

□ Time Vectors: Time is Encoded in the Weights of Finetuned Language Models
>> https://arxiv.org/abs/2312.13401
Time vectors, a simple tool to customize language models to new time periods.
Time vectors are created by finetuning a language model on data from a single time, and then subtracting the weights of the original pretrained model.
Time vectors specify a direction in weight space that, as our experiments show, improves performance on text from that time period. Time vectors specialized to adjacent time periods appear to be positioned closer together in a manifold.

□ SECE: accurate identification of spatial domain by incorporating global spatial proximity and local expression proximity
>> https://www.biorxiv.org/content/10.1101/2023.12.26.573377v1
SECE, an accurate spatial domain identification method for ST data. In contrast to the existing approaches, SECE incorporates global spatial proximity and local expression proximity of data to derive spatial domains.
The spatial embedding (SE) obtained by SECE enables downstream analysis including low-dimensional visualization and trajectory inference.
SECE utilizes Partition-based Graph Abstraction (PAGA) at the domain level and Monocle3 at the single-cell level. Moreover, when applied to ST data with single-cell resolution, SECE can accurately assign cell type labels by clustering cell type-related embedding.

□ SOAPy: a Python package to dissect spatial architecture, dynamics and communication
>> https://www.biorxiv.org/content/10.1101/2023.12.21.572725v1
SOAPy (Spatial Omics Analysis in Python) performs multiple tasks for dissecting spatial organization, incl. spatial domain, spatial expression tendency, spatiotemporal expression pattern, co-localization of paired cell types, multi-cellular niches, and cell-cell communication.
SOAPy employs tensor decomposition to extract components from the three-order expression tensor ("Time-Space-Gene"), revealing hidden patterns and reducing the complexity of data explanation.

□ scPML: pathway-based multi-view learning for cell type annotation from single-cell RNA-seq data
>> https://www.nature.com/articles/s42003-023-05634-z
scPML, utilizing well-labeled gene expression data, learns latent cell-type-specific patterns for annotating cells in test data. scPML initially employs various pathway datasets to model multiple cell-cell graphs to learn kinds of relationships among cells for a training dataset.
Pathway datasets divide genes into various gene sets based on specific biological processes, which reflect cell heterogeneity on the level of biological functions and minimize the impact of dropout events as a gene has limited effect on the entire gene set.
Structural information is learned from cell-cell graphs using self-supervised convolutional neural networks in scPML to produce denoised low-dimensional representations for cells.
scPML attempts to find a common representation which can be reconstructed to according embeddings and has the quality of separability. After obtaining the common latent representations, scPML uses a classifier to assign labels.


□ Pair-EGRET: enhancing the prediction of protein-protein interaction sites through graph attention networks and protein language models
>> https://www.biorxiv.org/content/10.1101/2023.12.25.572648v1
Pair-EGRET, an edge-aggregated graph attention network that leverages the features extracted from pre-trained transformer-like models to accurately predict PPI sites.
Pair-EGRET works on a k-nearest neighbor graph, representing the three-dimensional structure of a protein, and utilizes the cross-attention mechanism for accurate identification of interfacial residues of a pair of proteins.

□ ChimericFragments: Computation, analysis, and visualization of global RNA networks
>> https://www.biorxiv.org/content/10.1101/2023.12.21.572723v1
ChimericFragments, a computational platform for the analysis and interpretation of RNA-RNA interaction datasets starting from raw sequencing files. ChimericFragments enables rapid computation of RNA-RNA pairs, RNA duplex prediction, and a graph-based, interactive visualization of the results.
ChimericFragments employs a new algorithm based on the complementarity of chimeric fragments around the ligation site, which boosts the identification of bona fide RNA duplexes.
ChimericFragments shows the aggregate of all detected ligation sites for each interacting transcript, allowing for the identification of preferred base-pairing sequences in regulatory RNAs and their targets.

□ GAPS: Geometric Attention-based Networks for Peptide Binding Sites Identification by the Transfer Learning Approach
>> https://www.biorxiv.org/content/10.1101/2023.12.26.573336v1
GAPS employs a transfer learning strategy, leveraging pre-trained information on protein-protein binding sites to enhance the training for recognizing protein-peptide binding sites, while considering the similarity between proteins and peptides.
The atom-based geometric information makes the GAPS model granularity smaller, increasing the likelihood of capturing inherent biological information among amino acid residues, and it also ensures the model's translation-invariance and rotation-equivariance.

□ Optimal distance metrics for single-cell RNA-seq populations
>> https://www.biorxiv.org/content/10.1101/2023.12.26.572833v1
A reusable framework for evaluating distance metrics for single-cell gene expression data. To mimic how distance metrics would be used in model evaluation or dataset analysis, they quantify their sensitivity and robustness when identifying differences between populations.
The control relative percentile (CRP) is defined as the percentage of perturbed conditions with a larger distance to the reference control set than the control sets to each other, averaged across five control sets.

□ COBRA: Higher-order correction of persistent batch effects in correlation networks
>> https://www.biorxiv.org/content/co10.1101/2023.12.28.573533v1
COBRA (Co-expression Batch Reduction Adjustment), a method for computing a batch-corrected gene co-expression matrix based on estimating a conditional covariance matrix.
COBRA estimates a reduced set of parameters expressing the co-expression matrix as a function of the sample covariates, allowing control for continuous and categorical covariates.

□ Multidimensional Soliton Systems
>> https://arxiv.org/abs/2312.17096
A remarkable feature of multidimensional solitons is their ability to carry vorticity; however, 2D vortex rings and 3D vortex tori are subject to strong splitting instability.
Therefore, it is natural to categorize the basic results according to physically relevant settings which make it possible to maintain stability of fundamental (non-topological) and vortex solitons against the collapse and splitting, respectively.
The present review is focused on schemes that were recently elaborated in terms of Bose-Einstein condensates and similar photonic setups.
These are two-component systems with spin-orbit coupling, and ones stabilized by the beyond-mean-field Lee-Huang-Yang effect.The latter setting has been implemented experimentally, giving rise to stable self-trapped quasi-2D and 3D "quantum droplets".

□ Node Features of Chromosome Structure Network and Their Connections to Genome Annotation
>> https://www.biorxiv.org/content/10.1101/2023.12.29.573476v1
Constructing chromosome structure networks (CSNs) from bulk Hi-C data and calculated a set of site-resolved (node-based) network properties of these CSNs. These network properties are useful for characterizing chromosome structure features.
Semi-local network properties are more capable of characterizing genome annotations than diffusive or ultra-local node features.
For example, local square clustering coefficient can be a strong classifier of lamina-associated domains (LADs), whereas a path-based network property, closeness centrality, does not vary concordantly with LAD status.

□ RepeatOBserver: tandem repeat visualization and centromere detection
>> https://www.biorxiv.org/content/10.1101/2023.12.30.573697v1
RepeatOBserver, a new tool for visualizing tandem repeats and clustered transposable elements and for identifying potential natural centromere locations, using a Fourier transform of DNA walks.
RepeatOBserver can identify a broad range of repeats (3-20,000bp long) in genome assemblies without any a priori knowledge of repeat sequences or the need for optimizing parameters.

□ AntiNoise: Genomic background sequences systematically outperform synthetic ones in de novo motif discovery for ChIP-seq data
>> https://www.biorxiv.org/content/10.1101/2023.12.30.573742v1
The synthetic approach performs nucleotides shuffling that abolishes the enrichment of any motifs. This procedure radically destroys in the foreground sequences the enrichment of k-mers of any length.
These k-mers represent either specific or non-specific motifs; they compete between each other at the next step of de novo motifs search.
Maximal number of attempts NA to find matching background sequences in the genome. If a given number NA of last attempts to find any at least one more background sequence are unsuccessful, the algorithm terminates.