


□ ORFeus: A Computational Method to Detect Programmed Ribosomal Frameshifts and Other Non-Canonical Translation Events
>> https://www.biorxiv.org/content/10.1101/2023.04.24.538127v1
ORFeus uses a hidden Markov model to infer translation patterns from ribo-seq data that is inherently noisy and sparse. The model identifies changes in reading frame and additional upstream or downstream reading frames, making it suitable for detection of many alternative translation events.
ORFeus can identify novel or extended ORFs (including uORFs and dORFs) with either canonical or non-canonical start codons, as well as programmed ribosomal frameshifts and stop codon readthrough events. For each transcript, ORFeus returns the most probable state path.

□ scGPT: Towards Building a Foundation Model for Single-Cell Multi-omics Using Generative AI
>> https://www.biorxiv.org/content/10.1101/2023.04.30.538439v1
scGPT, a single-cell foundation model by GPT on over 10 million cells. scGPT uses an in-memory data structure to store hundreds of datasets that allow fast access. The learned gene embedding maps decode known pathways by grouping together genes that are functionally relevant.
With zero-shot learning, the pre-trained model is able to reveal meaningful cell clusters on unseen datasets. With finetuning in a few-shot learning setting, the model achieves state-of-the-art performance on a wide range of downstream tasks.
scGPT employes the generative self-supervised objective to iteratively predict GE values of unknown tokens from known tokens in an auto-regressive manner. scGPT's embedding architecture can easily extend to multiple sequencing modalities, batches, and perturbation states.

□ REVNANO: Reverse Engineering DNA Origami Nanostructure Designs from Raw Scaffold and Staple Sequence Lists
>> https://www.biorxiv.org/content/10.1101/2023.05.03.539261v1
REVNANO, a constraint programming solver that recovers the (approximate) staple-scaffold contact map from origami sequences. REVNANO uses graph layout techniques to convert the topological contact map into an approximate geometric origami schematic.
REVNANO leverages the unique physical features of origami nanostructures as heuristics. DNA, RNA or hybrid scaffolded origami are all supported. The quality of the REVNANO solution is quantified by taking the base hamming distance between the ground truth contact map.

□ UnitedNet: Explainable multi-task learning for multi-modality biological data analysis
>> https://www.nature.com/articles/s41467-023-37477-x
UnitedNet has an encoder-decoder-discriminator structure and is trained by joint group identification / cross-modal prediction. Its structure does not presume that the data distributions are known - instead implicitly approximates the statistical characteristics of each modality.
UnitedNet uses SHapley Additive exPlanations algorithm and indicates the relevance relationship between gene expression and DNA accessibility with cell-type specificity. UnitedNet fuses these codes into shared latent codes using an adaptive weighting scheme.

□ AirLift: A Fast and Comprehensive Technique for Remapping Alignments between Reference Genomes
>> https://www.biorxiv.org/content/10.1101/2021.02.16.431517v2
AirLift, a methodology and tool for quickly, comprehensively, and accurately remapping a read data set that had previously been mapped to an older reference genome to a newer reference genome.
AirLift provides BAM-to-BAM remapping results on which downstream analysis can be immediately performed. AirLift Index exploits the similarity b/n two references to quickly identify candidate locations that a read should be remapped to based on its original mapping.

□ DELVE: Feature selection for preserving biological trajectories in single-cell data
>> https://www.biorxiv.org/content/10.1101/2023.05.09.540043v1
DELVE (dynamic selection of locally covarying features), an unsupervised feature selection method for identifying a representative subset of dynamically-expressed molecular features that recapitulates cellular trajectories.
DELVE uses a bottom-up approach to mitigate the effect of unwanted sources of variation confounding inference, and instead models cell states from dynamic feature modules that constitute core regulatory complexes.

□ Designing molecular RNA switches with Restricted Boltzmann machines
>> https://www.biorxiv.org/content/10.1101/2023.05.10.540155v1
Restricted Boltzmann machines (RBM), a simple two-layer machine learning model, capture intricate sequence dependencies induced by secondary and tertiary structure, as well as the switching mechanism, resulting in a model that can be used for the design of allosteric RNA.
The hidden units of the RBM must extract features shared by the data sequences and thus likely to be important for their biological function. Conservation of probability mass implies that regions of sequence space not populated by data sequences must be penalized.
The RBM is able to model complex interactions. After marginalizing over the hidden units configurations, effective interactions arise between the visible units. RBM can represent schematically a three-body interaction, arising from the three connections of the summed hidden unit.
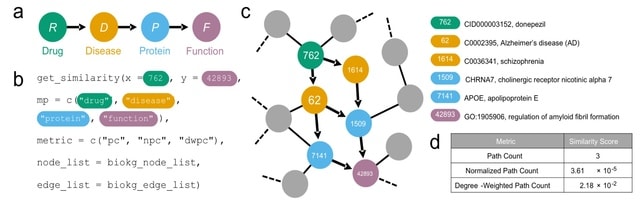
□ metapaths: similarity search in heterogeneous knowledge graphs via meta paths
>> https://academic.oup.com/bioinformatics/advance-article/doi/10.1093/bioinformatics/btad297/7152274
Once informative meta paths for a given KG have been defined, these meta paths define the semantics of the relationships between nodes in the KG, thereby enabling heterogeneous graph convolutional and graph attention networks for downstream machine learning analyses.
The primitives of the metapaths package identify the neighbors of a specified node with a given type by querying either an edge t or, for efficiency, an adjacency list precomputed from the edge list.
The meta path traversal function accepts an origin node, a destination node, and a specified meta path; then, via the neighbor identification functions, it starts at the origin node and recursively expounds the sequence of node types until the destination node is reached.


□ EvoAug: improving generalization and interpretability of genomic deep neural networks with evolution-inspired data augmentations
>> https://genomebiology.biomedcentral.com/articles/10.1186/s13059-023-02941-w
Random transformation of DNA sequences can potentially alter their function in unknown ways. EvoAug pretrains sequence-based deep learning models for regulatory genomics data w/ evolution-inspired augmentations followed by a finetuning on the original, unperturbed sequence data.
EvoAug data augmentations introduce a modeling bias to learn invariances of the (un)natural symmetries generated by the augmentations.
Random insertions and deletions assume that the distance between motifs is not critical, whereas random inversions and translocations promote invariances to motif strand orientation and the order of motifs.

□ ProteinSGM: Score-based generative modeling for de novo protein design
>> https://www.nature.com/articles/s43588-023-00440-3
ProteinSGM, a continuous-time score-based generative model that generates high-quality de novo proteins. ProteinSGM learns to generate four matrices that fully describes a protein's backbone, which are used as smoothed harmonic constraints in the Rosetta minimization protocol.
ProteinSGM generates variable-length structures with a mean < -3.9 REU per residue, indicative of native-like structures. It provides an alternative approach that uses MinMover for backbone minimization, and ProteinMPNN and OmegaFold for sequence design and structure prediction.

□ CEBRA: Learnable latent embeddings for joint behavioural and neural analysis
>> https://www.nature.com/articles/s41586-023-06031-6
CEBRA is a nonlinear dimensionality reduction method newly developed to explicitly leverage auxiliary (behaviour) labels and/or time to discover latent features in time series data—in this case, latent neural embeddings.
CEBRA can be used for supervised and self-supervised analysis, thereby directly facilitating hypothesis- and discovery-driven science. It produces both consistent embeddings across subjects and can find the dimensionality of neural spaces that are topologically robust.
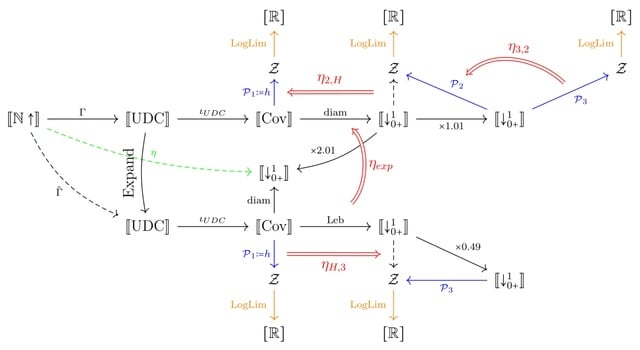
□ The categorical basis of dynamical entropy
>> https://arxiv.org/abs/2301.09205
The focus of topological Dynamical systems theory is to derive properties of the system. The objects that are usually in consideration are invariant behavior such as attractors, invariant sets and omega-limit sets, and asymptotic properties such as invariant measures and entropy.
A category-theoretic view of topological dynamical entropy, which reveals that the common limit is a consequence of the structural assumptions on these notions. One of the key tools developed is that of a qualifying pair of functors, which ensure a limit preserving property.
The diameter and Lebesgue number of open covers of a compact space, form a qualifying pair of functors. The various notions of complexity are expressed as functors, and natural transformations between these functors lead to their joint convergence to the common limit.

□ A draft human pangenome reference
>> https://www.nature.com/articles/s41586-023-05896-x
Flagger detects different types of misassemblies within a phased diploid assembly. The pipeline works by mapping the HiFi reads to the combined maternal and paternal assembly in a haplotype-aware manner.
Flagger identifies coverage inconsistencies within these read mappings. Coverage is calculated across the genome and a mixture model is fit to account for reliably assembled haploid sequence and various classes of unreliably assembled sequence.

□ Squigulator: simulation of nanopore sequencing signal data with tunable noise parameters
>> https://www.biorxiv.org/content/10.1101/2023.05.09.539953v1
Squigulator generates simulated nanopore signal data based on an input reference genome or transcriptome sequence, or directly from a set of basecalled reads.
Squigulator uses an idealised 'pore model' that specifies the predicted current signal reading associated with every possible DNA or RNA k-mer, as appropriate to the specific nanopore protocol being emulated.
Squigulator generates sequential signal values corresponding to sequential k-mers in the provided reference sequence. squigulator transforms the data using Gaussian noise functions in both the time and amplitude domains to produce realistic, rather than ideal, signal reads.

□ Ariadne: Synthetic Long Read Deconvolution Using Assembly Graphs
>> https://www.biorxiv.org/content/10.1101/2021.05.09.443255v3
Ariadne, a novel assembly graph-based SLR deconvolution algorithm, that can be used to extract single-species read-clouds from SLR datasets to improve the taxonomic classification and de novo assembly of complex populations, such as metagenomes.
Ariadne leverages the linkage information encoded in the full de Bruin-based assembly graph generated by a de novo assembly tool such as cloudSPAdes to generate up to 37.5-fold more read clouds containing only reads from a single fragment.

□ Merizo: a rapid and accurate domain segmentation method using invariant point attention
>> https://www.biorxiv.org/content/10.1101/2023.02.19.529114v2
Network inputs to the IPA encoder are the single and pairwise representations and backbone frames in the style of AlphaFold2. The IPA encoder comprises six weight-shared blocks, each containing a single IPA block with RoPE positional encoding, and a bi-GRU transition block.
In the Masked transformer decoder, learnable domain mask embeddings dare concatenated to the single representation and passed through a 10-layer MHA stack with ALiBi positional encoding.
The predicted domain mask tensor is split according to the predicted domain and is passed through a two-layer biGRU, followed by projection into one dimension to produce a single ploU value for each domain. ndom represents the number of predicted domains.

□ Evolutionary graph theory on rugged fitness landscapes
>> https://www.biorxiv.org/content/10.1101/2023.05.04.539435v1
A unifying theory of how heterogenous structure shapes evolutionary dynamics. Even a simple extension to a two-mutational landscape can exhibit evolutionary dynamics not observed in deme-based models and that cannot be predicted using single-mutation results.
This model can be applied to understand the evolutionary trajectory of cellular systems with complex architectures. Heterogenous structure can affect fitness landscape crossing by allowing intermediate mutants to persist for longer, until the final beneficial mutation occurs.

□ The Compositional Structure of Bayesian Inference
>> https://arxiv.org/abs/2305.06112
A compositional Bayesian inversion of Markov kernels in isolation, using a suitable axiomatisation of a category of Markov kernels. It builds categories whose morphisms are pairs of a Markov kernel and an associated 'Bayesian inverter', which is itself built compositionally.
Symmetric monoidal categories with compatible families of copy and delete morphisms have been identified as an expressive language for synthetically representing concepts from probability theory.
A categorical translation of Bayes allows for a general definition of a Bayesian inverse to a morphism in a Markov category. The category of Bayesian lenses is constructed as a fired category that is closely related to the families fibration, in the semantics of dependent types.

□ CoCoNat: a novel method based on deep-learning for coiled-coil prediction
>> https://www.biorxiv.org/content/10.1101/2023.05.08.539816v1
CoCoNat encodes sequences with the combination of two state-of-the- art protein language models and implements a three-step deep learning procedure concatenated with a Grammatical-Restrained Hidden Conditional Random Field (GRHCRF) for CCD identification and refinement.
CoCoNat makes use of residue embeddings obtained with large-scale protein Language Models (pLMs) to represent proteins in training and testing sets. CoCoNat adopts a 15 residue long sliding window, takes as input, where each residue is represented with a 2304-feature vector.

□ snATAK: Assessing the multimodal tradeoff
>> https://www.biorxiv.org/content/10.1101/2021.12.08.471788v2
snATAK incorporates kallisto and other tools in a workflow that facilitates the preprocessing of snATAC-seq data from numerous technologies in minimal computing environments. snATAK can be used for allele-specific analysis of multimodal data, even in the absence of genotype data.
snATACK consists of first mapping reads to a reference genome using Minimap2. snATAK identifies putative open chromatin regions with Genrich. A kallisto pseudoalignment index is made and reads are remapped using kalisto. The snATAK output is compatible with the Signac and ArchR.

□ GenPhys: From Physical Processes to Generative Models
>> https://arxiv.org/abs/2304.02637
GenPhys (Generative Models from Physical Processes), a frame-work that can convert physical Partial differential equations (PDEs) to generative models. Diffusion models and Poisson flow generative models leverage the diffusion equation and the Poisson equation.
There exists non s-generative model which can also provide useful generative modeling, such as the case in quantum machine learning with dynamics based on the Schrödinger equation and quantum circuits.

□ Learning Decision Trees with Gradient Descent
>> https://arxiv.org/abs/2305.03515
Gradient-based decision trees (GDTs), a novel approach for learning hard, axis-aligned Decision Trees (DTs) with gradient descent. The proposed method uses backpropagation with a straight-through operator on a dense DT representation to jointly optimize all tree parameters.
GDTs are less prone to overfitting. GDT optimizes the gradient descent algorithm by exploiting common stochastic gradient descent techniques, including mini-batch calculation and momentum using the Adam optimizer with weight averaging.

□ LatentDiff: A Latent Diffusion Model for Protein Structure Generation
>> https://arxiv.org/abs/2305.04120
Latent Diff generates a novel protein backbone structure. They first sample multivariate Gaussian noise and use the learned latent diffusion model to generate 3D positions and node embeddings in the latent space.
Latent Diff uses a pre-trained equivariant 3D autoencoder to transform protein backbones into a more compact latent space, and models the latent distribution with an equivariant latent diffusion model.
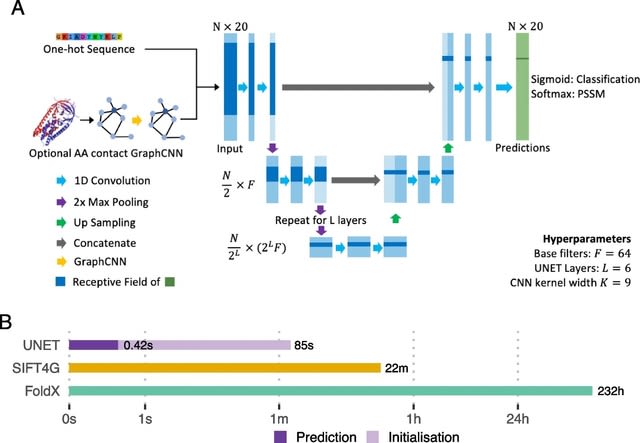
□ Sequence UNET: High-throughput deep learning variant effect prediction
>> https://genomebiology.biomedcentral.com/articles/10.1186/s13059-023-02948-3
Sequence UNET is trained to directly predict variant frequency or to classify low frequency variants, as a proxy for deleteriousness, and then fine-tuned for pathogenicity prediction.
Sequence UNET uses a fully convolutional architecture. Convolutional kernels also naturally integrate information from nearby amino acids. The model outputs a matrix of per position features and can therefore be trained to predict various positional properties.

□ aaHash: recursive amino acid sequence hashing
>> https://www.biorxiv.org/content/10.1101/2023.05.08.539909v1
aaHash, a recursive hashing algorithm tailored for amino acid sequences. This algorithm utilizes multiple hash levels to represent biochemical similarities between amino acids. aaHash performs ~10X faster than generic string hashing algorithms in hashing adjacent k-mers.
aaHlash builds on ntHash, a rolling hash algorithm for DNA/RNA sequences, and adapts it for amino acid sequences. aaHash also supports using different levels of hashes together to create a multi-level pattern, mimicking the functionality of spaced seeds.

□ BGWAS: Bayesian variable selection in linear mixed models with nonlocal priors for genome-wide association studies
>> https://bmcbioinformatics.biomedcentral.com/articles/10.1186/s12859-023-05316-x
BGWAS uses a novel nonlocal prior for linear mixed models (LMMs). The screening step fits as many LMMs as the number of SNPs using a mixture of a Dirac delta at zero and a nonlocal prior, and estimates the probability of the Dirac delta component.
BGWAS uses a pMOM nonlocal prior for LMMs that uses the full Fisher information matrix. BGWAS either uses complete enumeration or searches the model space with a genetic algorithm.

□ AIONER: All-in-one scheme-based biomedical named entity recognition using deep learning
>> https://academic.oup.com/bioinformatics/advance-article/doi/10.1093/bioinformatics/btad310/7160912
AIONER, a new NER tagger that takes full advantage of various existing datasets for recognizing multiple entities simultaneously, despite their inherent differences in scope and quality, through a novel all-in-one (AIO) scheme.
The AIO scheme utilizes a small dataset recently annotated with multiple Entity types as a bridge to integrate multiple datasets annotated with a subset of entity types, thereby recognizing multiple entities at once, resulting in improved accuracy and robustness.

□ NanoPack2: Population scale evaluation of long-read sequencing data
>> https://academic.oup.com/bioinformatics/advance-article-abstract/doi/10.1093/bioinformatics/btad311/7160911
The cramino, chopper, kyber, and phasius tools are written in Rust and available as executable binaries without requiring installation or managing dependencies. Binaries build on musl are available for broad compatibility.
Phasius is developed to visualize the results of read phasing, which shows in a dynamic genome browser style the length and interruptions between contiguously phased blocks from a large number of individuals together with genome annotation, for example, segmental duplications.

□ copMEM2: Robust and scalable maximum exact match finding
>> https://academic.oup.com/bioinformatics/advance-article/doi/10.1093/bioinformatics/btad313/7160910
copMEM2, a multi-threaded MEM finding tool, targeting the execution speed and reducing the memory, as well as incorporating an improvement to speed up its processing by orders of magnitude when the pair of genomes is highly similar.
copMEM2 allows to compute all MEMs of minimum length 50 between the human and mouse genomes in 59s, using 10.40 GB of RAM and 12 threads, being at least a few times faster than its main contenders. On a pair of human genomes, hg18 and hg19, the results are 324s and 16.57 GB.

□ Integration of a multi-omics stem cell differentiation dataset using a dynamical model
>> https://journals.plos.org/plosgenetics/article?id=10.1371/journal.pgen.1010744
A hierarchical dynamical model that allowed us to integrate all data sets. This model was able to explain mRNA-protein discordance for most genes and identified instances of potential microRNA-mediated regulation.
Overexpression or depletion of microRNAs identified by the model, followed by RNA sequencing and protein quantification, were used to follow up on the predictions of the model.

□ Improving variant calling using population data and deep learning
>> https://bmcbioinformatics.biomedcentral.com/articles/10.1186/s12859-023-05294-0
A population-aware DeepVariant models with a new channel encoding allele frequencies. This model reduces variant calling errors, improving both precision and recall in single samples, and reduces rare homozygous and pathogenic clinvar calls cohort-wide.
The relative advantage of the population-aware models increase at lower coverage, suggesting that population information is most valuable in difficult examples, where read-level information alone may not be sufficient for confident calling.

□ DeSide: A unified deep learning approach for cellular decomposition of bulk tumors based on limited scRNA-seq data
>> https://www.biorxiv.org/content/10.1101/2023.05.11.540466v1
The DeSide architecture considers only non-cancerous cells during the training process, indirectly calculating the proportion of cancerous cells.
DeSide avoids directly handling the often more variable heterogeneity of cancerous cells, and instead leverages scRNA-seq data from three different cancer types to empower the DNN model with a robust generalization capability across diverse cancers.

□ A Superior Thumb Drive: Optimizing DNA Stability for DNA Data Storage
>> https://www.biorxiv.org/content/10.1101/2023.05.11.540302v1
While methods to achieve DNA stability for hundreds or even millennia are possible, they call for completely enclosing DNA inside a silica matrix.
For instance, for an Archival Storage system whose DNA is enclosed in silica, the probability of strand loss or breakage is much lower, thereby enabling the use of longer DNA strands and higher information densities.
Conversely, for Working or Short-Term Storage systems, shorter strand lengths and lower information density requirements would be more appropriate due to the higher likelihood of strand loss.