
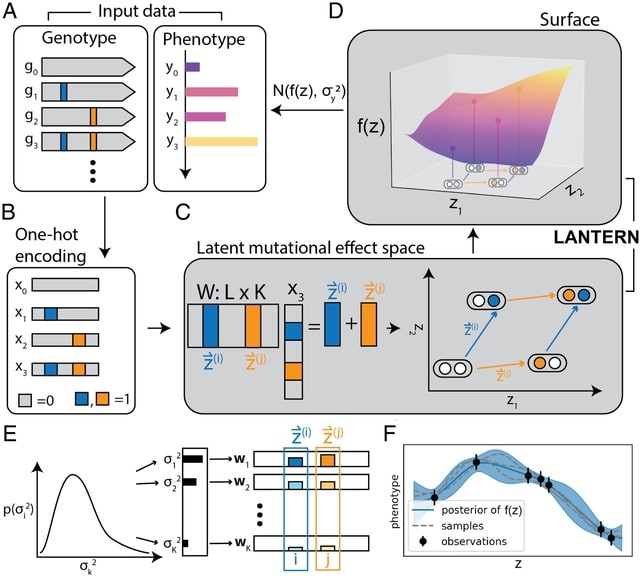
□ LANTERN: Interpretable modeling of genotype-phenotype landscapes with state-of-the-art predictive power
>> https://www.pnas.org/doi/10.1073/pnas.2114021119
LANTERN, a hierarchical Bayesian model that distills genotype–phenotype landscape (GPL) measurements into a low-dimensional feature space. LANTERN captures the nonlinear effects of epistasis through a multidimensional, nonparametric Gaussian Process model.
LANTERN predicts the position of variant in the latent mutational effect space as a linear combination of mutation effect vectors with an unknown matrix. LANTERN facilitates discovery of fundamental mechanisms in GPLs, while extrapolating to unexplored regions of genotypic space.

□ psupertime: supervised pseudotime analysis for time-series single-cell RNA-seq data
>> https://academic.oup.com/bioinformatics/article/38/Supplement_1/i290/6617492
psupertime, a supervised pseudotime approach based on a regression model. It identifies genes that vary coherently along a time series, in addition to pseudo-time values for individual cells, and a classifier that can be used to estimate labels for new data with unknown or differing labels.
psupertime is based on penalized ordinal regression, a statistical technique used where data have categorical labels that follow a sequence. A pseudotime value for each individual cell is obtained by multiplying the log gene expression values by the vector of coefficients.

□ scDREAMER: atlas-level integration of single-cell datasets using deep generative model paired with adversarial classifier
>> https://www.biorxiv.org/content/10.1101/2022.07.12.499846v1.full.pdf
scDREAMER can overcome critical challenges including the presence of skewed cell types among batches, nested batch effects, large number of batches and conservation of development trajectory across different batches.
scDREAMER employs a novel adversarial variational autoencoder for inferring the latent cellular embeddings from the high-dimensional gene expression matrices from different batches. scDREAMER is trained using evidence lower bound and Bhattacharyya loss.

□ scSTEM: clustering pseudotime ordered single-cell data
>> https://genomebiology.
biomedcentral.com/articles/10.1186/s13059-022-02716-9
scSTEM uses one of several metrics to summarize the expression of genes and assigns a p-value to clusters enabling the identification of significant profiles and comparison of profiles across different paths.
scSTEM generates summary time series data using several different approaches for each of the paths. This data is then used as input for STEM and clusters are determined for each path in the trajectory.

□ scMMGAN: Single-Cell Multi-Modal GAN architecture resolves the ambiguity created by only stating a distribution-level loss in learning a mapping.
>> https://www.biorxiv.org/content/10.1101/2022.07.04.498732v1.full.pdf
Single-Cell Multi-Modal GAN (scMMGAN) that integrates data from multiple modalities into a unified representation in the ambient data space for downstream analysis using a combination of adversarial learning and data geometry techniques.
scMMGAN achieves multi-modality and specify a generally applicable correspondence loss: the geometry preserving loss. It enforces the diffusion geometry, performed w/ a new kernel designed to pass gradients better than the Gaussian kernel, is preserved throughout the mapping.
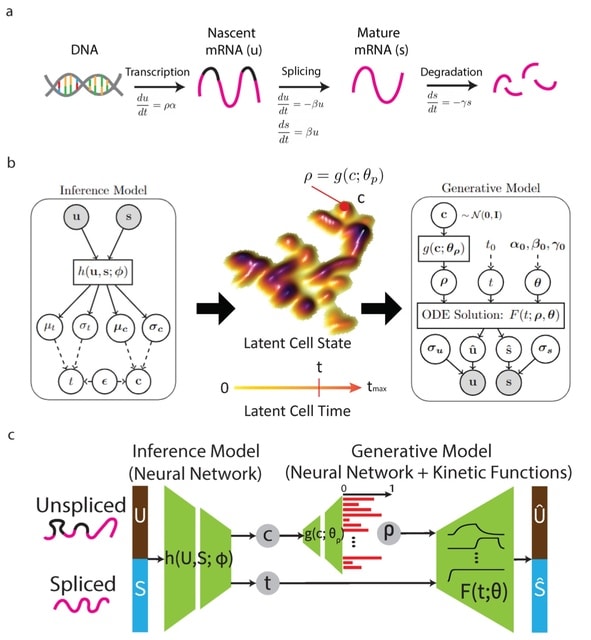
□ VeloVAE: Bayesian Inference of RNA Velocity from Multi-Lineage Single-Cell Data
>> https://www.biorxiv.org/content/10.1101/2022.07.08.499381v1.full.pdf
VeloVAE uses variational Bayesian inference to estimate the posterior distribution of latent time, latent cell state, and kinetic rate parameters for each cell.
VeloVAE addresses key limitations of previous methods by inferring a global time and cell state; modeling the emergence of multiple cell types; incorporating prior information such as time point labels; using scalable minibatch optimization; and quantifying parameter uncertainty.
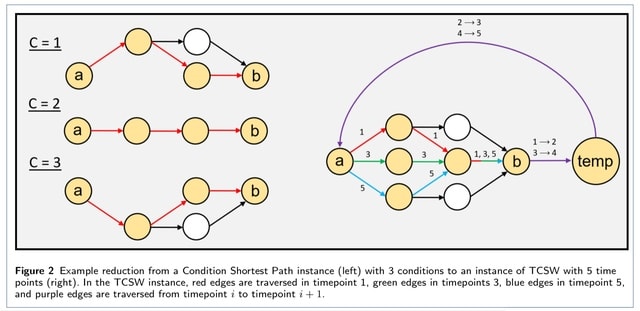
□ TCSW: Directed Shortest Walk on Temporal Graphs
>> https://www.biorxiv.org/content/10.1101/2022.07.08.499368v1.full.pdf
The Time Conditioned Shortest Walk (TCSW) problem, which takes on a similar flavor as Condition Shortest Path. It gives a series of ordered networks Gt and ordered conditions {1, ..., T} representing a discrete measurement of time, and as well as a pair of nodes (a∈G1,b∈GT).
Extending the Condition setting to TCSW, a singular global shortest path problem w/ the temporal walk constraint, becomes hard to solve. An integer linear program solves a generalized version of TCSW. It finds optimal solutions to the generalized k-TCSW problem in feasible time.

□ GeneTrajectory: Gene Trajectory Inference for Single-cell Data by Optimal Transport Metrics
>> https://www.biorxiv.org/content/10.1101/2022.07.08.499404v1.full.pdf
GeneTrajectory unravels gene trajectories associated with distinct biological processes. GeneTrajectory computes a cell-cell graph that preserves the manifold structure of the cells.
GeneTrajectory construct a gene-gene graph where the affinities between genes are based on the Wasserstein distances between their distributions on the cell graph. Each trajectory is associated with a specific biological process and reveals the pseudo-temporal order.
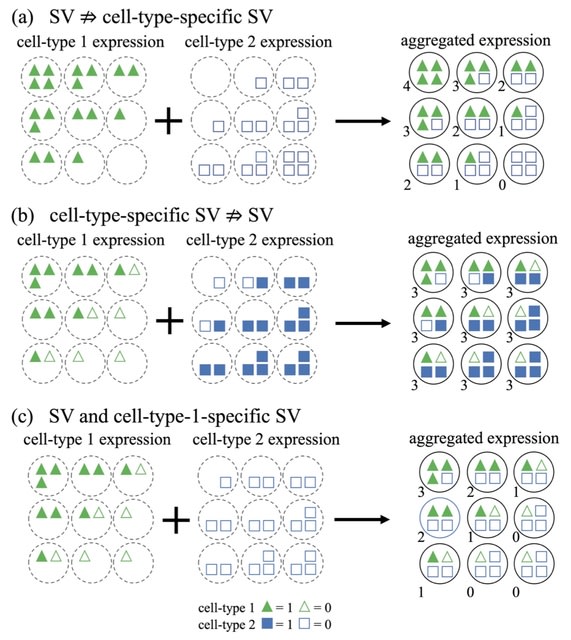
□ CTSV: Identification of Cell-Type-Specific Spatially Variable Genes Accounting for Excess Zeros
>> https://academic.oup.com/bioinformatics/advance-article/doi/10.1093/bioinformatics/btac457/6632658
In fact, the spatial information can be incorporated into the Gaussian process in two ways—the spatial effect on the mean vector or the spatial dependency induced by the covariance matrix.
CTSV explicitly incorporates the cell type proportions of spots into a zero-inflated negative binomial distribution and models the spatial effects through the mean vector, whereas existing SV gene detection approaches either do not directly utilize cellular compositions or do not account for excess zeros.
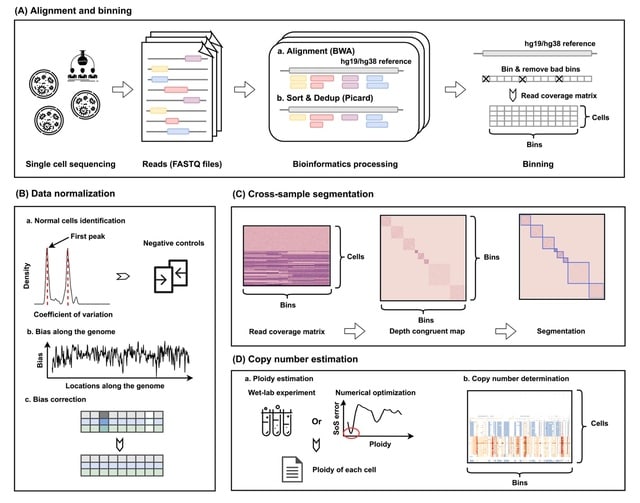
□ SeCNV: Resolving single-cell copy number profiling for large datasets
>> https://academic.oup.com/bib/advance-article-abstract/doi/10.1093/bib/bbac264/6633647
SeCNV successfully processes large datasets (>50 000 cells) within 4 min, while other tools fail to finish within the time limit, i.e. 120 h.
SeCNV adopts a local Gaussian kernel to construct a matrix, depth congruent map (DCM), capturing the similarities between any two bins along the genome. Then, SeCNV partitions the genome into segments by minimizing the structural entropy.

□ BubbleGun: Enumerating Bubbles and Superbubbles in Genome Graphs
>> https://academic.oup.com/bioinformatics/advance-article/doi/10.1093/bioinformatics/btac448/6633304
BubbleGun is considerably faster than vg especially in bigger graphs, where it reports all bubbles in less than 30 minutes on a human sample de Bruijn graph of around 2 million nodes.
BubbleGun detects superbubbles in a given input graph by implementing the algorithm, which is an average-case linear time algorithm. The algorithm iterates over all nodes s in the graph and determines whether there is another node t that satisfies the superbubble rules.

□ treeArches: Single-cell reference mapping to construct and extend cell type hierarchies
>> https://www.biorxiv.org/content/10.1101/2022.07.07.499109v1.full.pdf
treeArches, a framework to automatically build and extend reference atlases while enriching them with an updatable hierarchy of cell type annotations across different datasets. treeArches enables data-driven construction of consensus, atlas-level cell type hierarchies.
treeArches builds on scArches and single-cell Hierarchical Progressive Learning (scHPL). treeArches maps new query datasets to the latent space learned from the reference datasets using architectural surgery.

□ Detection of cell markers from single cell RNA-seq with sc2marker
>> https://bmcbioinformatics.biomedcentral.com/articles/10.1186/s12859-022-04817-5
sc2marker is based on the maximum margin to select markers for flow cytometry. sc2marker finds an optimal threshold α (or margin) with maximal distances to true positives (TP) and true negatives (TN) and low distances to false positives (FP) and false negatives (FN).
Hypergate uses a non-parametric score statistic to find markers in scRNA-seq data that distinguish different cell types. sc2marker reimplements the Hypergate criteria to rank all markers. sc2marker allows users to explore the COMET database using the option “category=FlowComet”.

□ Verkko: telomere-to-telomere assembly of diploid chromosomes
> https://www.biorxiv.org/content/10.1101/2022.06.24.497523v1.full.pdf
To resolve the most complex repeats, this project relied on manual integration of ultra-long Oxford Nanopore sequencing reads with a high-resolution assembly graph built from long, accurate PacBio HiFi reads.
Verkko, an iterative, graph-based pipeline for assembling complete, diploid genomes. Verkko begins with a multiplex de Bruijn graph built from long, accurate reads and progressively simplifies this graph via the integration of ultra-long reads and haplotype paths.


□ ResMiCo: increasing the quality of metagenome-assembled genomes with deep learning
>> https://www.biorxiv.org/content/10.1101/2022.06.23.497335v1.full.pdf
Accuracy for the state of the art in reference-free misassembly prediction does not exceed an AUPRC of 0.57, and it is not clear how well these models generalize to real-world data.
the Residual neural network for Misassembled Contig identification (ResMiCo) is a deep convolutional neural network with skip connections between non-adjacent layers. ResMiCo is substantially accurate, and the model is robust to novel taxonomic diversity and varying assembly.

□ Bookend: precise transcript reconstruction with end-guided assembly
>> https://genomebiology.biomedcentral.com/articles/10.1186/s13059-022-02700-3
Bookend is a generalized framework for identifying RNA ends in sequencing data and using this information to assemble transcript isoforms as paths through a network accounting for splice sites, transcription start sites (TSS), and polyadenylation sites (PAS).
Bookend takes RNA-seq reads from any method as input and after alignment to a reference genome, reads are stored in an ELR format that records all RNA boundary features. The Overlap Graph is iteratively traversed to resolve an optimal set of Greedy Paths from TSSs to PASs.


□ Lokatt: A hybrid DNA nanopore basecaller with an explicit duration hidden Markov model and a residual LSTM network
>> https://www.biorxiv.org/content/10.1101/2022.07.13.499873v1.full.pdf
The duration of any state with a self transition in a Bayesian state-space model is always geometrically distributed. This is inconsistent with the dwell-times reported for both polymers and helicase, two popular candidates for ratcheting enzymes.
Lokatt: explicit duration Markov model and residual-LSTM network. Lokatt uses an explicit duration HMM (EDHMM) model with an additional duration state that models the dwell-time of the dominating k-mer.

□ scDART: integrating unmatched scRNA-seq and scATAC-seq data and learning cross-modality relationship simultaneously
>> https://genomebiology.biomedcentral.com/articles/10.1186/s13059-022-02706-x
scDART (single cell Deep learning model for ATAC-Seq and RNA-Seq Trajectory integration), a scalable deep learning framework that embeds data modalities into a shared low-dimensional latent space that preserves cell trajectory structures in the original datasets.
scDART learns a joint latent space for both data modalities that well preserve the cell developmental trajectories. Even though scDART-anchor were designed for cells that form continuous trajectories, they can also work for cells that form discrete clusters.

□ Duet: SNP-Assisted Structural Variant Calling and Phasing Using Oxford Nanopore Sequencing
>> https://www.biorxiv.org/content/10.1101/2022.07.04.498779v1.full.pdf
Duet, an SV detection tool optimized for SV calling and phasing using ONT data. The tool uses novel features integrated from both SV signatures and single-nucleotide polymorphism (SNP) signatures, which can accurately distinguish SV haplotype from a false signal.
Duet can perform accurate SV calling, SV genotyping and SV phasing using low-coverage ONT data. Duet will use the haplotype and the prediction confidence of the reads. Duet employs GNU Parallel to allow parallel processing of all chromosomes.

□ MSRCall: A Multi-scale Deep Neural Network to Basecall Oxford Nanopore Sequences
>> https://academic.oup.com/bioinformatics/advance-article-abstract/doi/10.1093/bioinformatics/btac435/6619554
MSRCall comprises a multi-scale structure, recurrent layers, a fusion block, and a CTC decoder. To better identify both short-range and long-range dependencies, the recurrent layer is redesigned to capture various time-scale features with a multi-scale structure.
MSRCall fuses convolutional layers to manipulate multi-scale downsampling. These back-to-back convolutional layers aim to capture features with receptive fields at different levels of complexity.

□ Single-cell generalized trend model (scGTM): a flexible and interpretable model of gene expression trend along cell pseudotime
>> https://academic.oup.com/bioinformatics/advance-article-abstract/doi/10.1093/bioinformatics/btac423/6618524
the single-cell generalized trend model (scGTM) for capturing a gene’s expression trend, which may be monotone, hill-shaped, or valley-shaped, along cell pseudotime.
scGTM uses the particle swarm optimization algorithm to find the constrained maximum likelihood estimates. A natural extension is to split a multiple-lineage cell trajectory into single lineages and fit the scGTM to each lineage separately.

□ scGET-seq: Dimensionality reduction and statistical modeling
>> https://www.biorxiv.org/content/10.1101/2022.06.29.498092v1.full.pdf
scGET-seq, a technique that exploits a Hybrid Transposase (tnH) along with the canonical enzyme (tn5), which is able to profile altogether closed and open chromatin in a single experiment.
scGET-seq uses Tensor Train Decomposition. It allows to represent data using a single tensor which can be factorized to obtain a low-dimensional embedding. scGET-seq overcomes the limitations of chromatin velocity and allows robust identification of cell trajectories.

□ GAVISUNK: Genome assembly validation via inter-SUNK distances in Oxford Nanopore reads
>> https://www.biorxiv.org/content/10.1101/2022.06.17.496619v1.full.pdf
GAVISUNK is a method of validating HiFi-driven assemblies with orthogonal ONT sequence. It specifically assesses the contiguity of regions, flagging potential haplotype switches or misassemblies.
GAVISUNK may be applied to any region or genome assembly to identify misassemblies and potential collapses, and is valuable for validating the integrity of regions. It can be applied at fine scale to closely examine regions of interest across multiple haplotype assemblies.

□ Bcmap: fast alignment-free barcode mapping for linked-read sequencing data
>> https://www.biorxiv.org/content/10.1101/2022.06.20.496811v1.full.pdf
Bcmap is accurate and an order of magnitude faster than full read alignment. Bcmap uses k-mer hash tables and window minimizers to swiftly map barcodes to the reference whilst calculating a mapping score.
Bcmap calculates all minimizers of the reads labeled with the same barcode and look them up in the k-mer reference index. It is constructed in a way that allows one to look up the frequency of a minimizer before accessing all associated positions.

□ CCC: An efficient not-only-linear correlation coefficient based on machine learning
>> https://www.biorxiv.org/content/10.1101/2022.06.15.496326v1.full.pdf
the Clustermatch Correlation Coefficient (CCC) reveals biologically meaningful linear and nonlinear patterns missed by standard, linear-only correlation coefficients.
CCC has a single parameter that limits the maximum complexity of relationships found. CCC captures general patterns in data by comparing clustering solutions while being much faster than state-of-the-art coefficients such as the Maximal Information Coefficient.

□ JASPER: a fast genome polishing tool that improves accuracy and creates population-specific reference genomes
>> https://www.biorxiv.org/content/10.1101/2022.06.14.496115v1.full.pdf
JASPER (Jellyfish-based Assembly Sequence Polisher for Error Reduction) gains efficiency by avoiding the alignment of reads to the assembly. Instead, JASPER uses a database of k-mer counts that it creates from the reads to detect and correct errors in the consensus.
JASPER can use these k-mer counts to “correct” a human genome assembly so that it contains all homozygous variants that are common in the population from which the reads were drawn.

□ Uncertainty quantification of reference based cellular deconvolution algorithms
>> https://www.biorxiv.org/content/10.1101/2022.06.15.496235v1.full.pdf
An accuracy metric that quantifies the CEll TYpe deconvolution GOodness (CETYGO) score of a set of cellular heterogeneity variables derived from a genome-wide DNA methylation profile for an individual sample.
While theorhetically the CETYGO score can be used in conjunction with any reference based deconvolution method, this package only contains code to calculate it in combination with Houseman's algorithm.

□ SAE-IBS: Hybrid Autoencoder with Orthogonal Latent Space for Robust Population Structure Inference
>> https://www.biorxiv.org/content/10.1101/2022.06.16.496401v1.full.pdf
SAE-IBS combines the strengths of traditional matrix decomposition-based (e.g., principal component analysis) and more recent neural network-based (e.g., autoencoders) solutions.
SAE-IBS generates a robust ancestry space in the presence of relatedness. SAE-IBS yields an orthogonal latent space enhancing dimensionality selection while learning non-linear transformations.

□ Analyzing single-cell bisulfite sequencing data with scbs
>> https://www.biorxiv.org/content/10.1101/2022.06.15.496318v1.full.pdf
scbs prepare parses methylation files produced by common bisulfite sequencing mappers and stores their contents in a compressed format optimised for efficient access to genomic intervals.
To obtain a methylation matrix, similar to the count matrices used in scRNA-seq, the user must first decide in which genomic intervals methylation should be quantified. The methylation matrix can be used for downstream analysis such as cell clustering / dimensionality reduction.
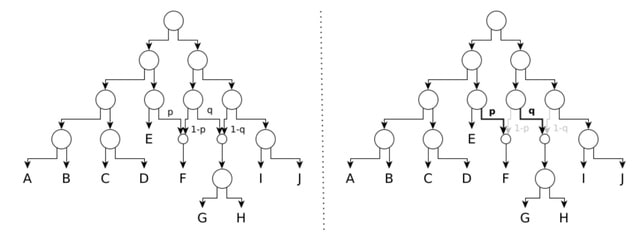
□ NetRAX: Accurate and Fast Maximum Likelihood Phylogenetic Network Inference
>> https://academic.oup.com/bioinformatics/advance-article/doi/10.1093/bioinformatics/btac396/6609768
NetRAX can infer maximum likelihood phylogenetic networks from partitioned multiple sequence alignments and returns the inferred networks in Extended Newick format.
NetRAX uses a greedy hill climbing approach to search for network topologies. It deploys an outer search loop to iterate over different move types and an inner search loop to search for the best- scoring network using a specific move type.

□ PolyAtailor: measuring poly(A) tail length from short-read and long-read sequencing data
>> https://academic.oup.com/bib/advance-article-abstract/doi/10.1093/bib/bbac271/6620877
PolyAtailor provides two core functions for measuring poly(A) tails, namely Tail_map and Tail_scan, which can be used for profiling tails with or without using a reference genome.
PolyAtailor can identify all potential tails in a read, providing users with detailed information such as tail position, tail length, tail sequence and tail type.
PolyAtailor integrates rich functions for poly(A) tail and poly(A) site analyses, such as differential poly(A) length analysis, poly(A) site identification and annotation, and statistics and visualization of base composition in tails.

□ Patchwork: alignment-based retrieval and concatenation of phylogenetic markers from genomic data
>> https://www.biorxiv.org/content/10.1101/2022.07.03.498606v1.full.pdf
Patchwork, a new method for mining phylogenetic markers directly from an assembled genome. Homologous regions are obtained via an alignment search, followed by a “hit-stitching” phase, in which adjacent or overlapping regions are concatenated together.
Patchwork utilizes the sequence aligner DIAMOND, and is written in the programming language Julia. A novel sliding window technique is used to trim non-coding regions from the alignments.

□ A Draft Human Pangenome Reference
>> https://www.biorxiv.org/content/10.1101/2022.07.09.499321v1.full.pdf
A draft pangenome that captures known variants and haplotypes, reveals novel alleles at structurally complex loci, and adds 119 million base pairs of euchromatic polymorphic sequence and 1,529 gene duplications relative to the existing reference, GRCh38.

□ UnpairReg: Integration of single-cell multi-omics data by regression analysis on unpaired observations
>> https://genomebiology.biomedcentral.com/articles/10.1186/s13059-022-02726-7
UnpairReg attempts to perform linear regression on the unpaired data. UnpairReg provides an accurate estimation of cell gene expression where only chromatin accessibility data is available. The cis-regulatory network inferred from UnpairReg is highly consistent with eQTL mapping.
UnpairReg uses a fast linear approximation algorithm. UnpairReg transfers the linear regression problem into a regression on covariance matrix. It is based on the assumption that the expression of different genes is independent under the condition of REs accessibility given.

□ On the importance of data transformation for data integration in single-cell RNA sequencing analysis
>> https://www.biorxiv.org/content/10.1101/2022.07.19.500522v1.full.pdf
A re-investigation employing different data transformation methods for preprocessing revealed that large performance gains can be achieved by a properly chosen optimal data transformation method. Transfer learning might not have significant benefits when preprocessing steps are well optimized.