
“Nemlich es reichen Die Sterblichen eh'an den Abgrund.Also wendet es sich,das Echo Mit diesen.”

環境-生態系の相互作用、あるいは種間の共生関係に対して、人間の社会的尺度における『合理性』を捉えてしまうことはアナロジーとしては遡行しており、物理的計算過程にある『状態』に対するシミュラークル現象である。

□ Infinitely Deep Bayesian Neural Networks with Stochastic Differential Equations
>> https://arxiv.org/pdf/2102.06559.pdf
Gradient-based stochastic variational inference in this infinite-parameter setting, producing arbitrarily-flexible approximate posteriors. A novel gradient estimator that approaches zero variance as the approximate posterior over weights approaches the true posterior.
SDE-BNNs, an alternative construction of Bayesian continuous-depth neural networks. Considering the limit of infinite-depth Bayesian neural networks w/ separate unknown weights at each layer. It allows non-factorized approximate posteriors implicitly defined through neural SDEs.
□ ON ∞-COSMOI OF BICATEGORIES:
>> https://arxiv.org/pdf/2108.11786v1.pdf
There are various ∞-cosmoi whose “∞-categories” are 2-categories or bicategories and whose “∞-functors” and “∞-natural transformations” define some variety of functor and natural transformation.
∞-cosmological definitions of adjunctions between ∞-categories or limits inside ∞-categories compile out to in the 2-quasi-categories model.
There is an ∞-cosmos in which the “∞-categories” are the (∞, n)- categories in that particular model. This suggests the tantalizing possibility that it might be possible to develop (∞,2)-category theory or (∞,n)-category theory “model-independently” by adapting ∞-cosmological methods.

□ Ergodicity and Convergence of Markov chain Monte Carlo Estimators
>> https://arxiv.org/pdf/2110.07032.pdf
A Short Review of the basic theory for quantifying both the asymptotic and preasymptotic convergence of Markov chain Monte Carlo estimators.
Geometric ergodicity in the total variation metric guarantees the existence of a Markov chain Monte Carlo central limit theorem that allows us to empirically quantify preasymptotic convergence of Markov chain Monte Carlo estimators for any sufficiently integrable function.
A Markov transition is periodic whenever there is a sequence of disjoint, π-non-null sets that trap Markov chains into cyclic transitions.
Once a Markov chain wanders into any of these sets it will be forever doomed to cycle between the three sets and unable to explore the rest of the ambient space.
Letting N grow to infinity the normal approximation given by the central limit theorem continues to narrow until it finally converges to a Dirac distribution in the asymptotic limit.

□ FoldHSphere: deep hyperspherical embeddings for protein fold recognition
>> https://bmcbioinformatics.biomedcentral.com/articles/10.1186/s12859-021-04419-7
To ensure maximum angular separation between prototypes, we draw inspiration from the well-known Thomson problem. Its goal is to determine the minimum energy configuration of K charged particles on the surface of a unit sphere.
By minimizing a Thomson-based loss function, extended to a hypersphere of arbitrary number of dimensions, FoldHSphere optimizes the angular distribution of our prototype vectors for each fold class that are maximally separated in hyperspherical space.
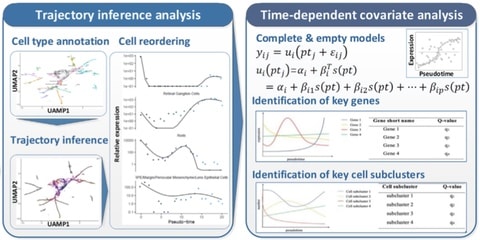
□ scTITANS: Identify differential genes and cell subclusters from time-series scRNA-seq data
>> https://www.sciencedirect.com/science/article/pii/S2001037021003068
scTITANS, a method that takes full advantage of individual cells from all time points at the same time by correcting cell asynchrony using pseudotime from trajectory inference analysis.
scTITANS reconstructs the true gene expression trends in time-series data. After correcting the asynchrony of single cells based on TI analysis, a time-dependent covariate is introduced to identify the DEGs and cell subclusters in dynamic processes.

□ scTriangulate: Decision-level integration of multimodal single-cell data
>> https://www.biorxiv.org/content/10.1101/2021.10.16.464640v1.full.pdf
Different from other multimodal methods that integrate at the data-level, through either a low-dimensional latent space, or through geometric graph, scTriangulate integrates results at a decision-level to reconcile conflicting cluster label assignments.
scTriangulate leverages cooperative game theory in conjunction w/ stability metrics (reassign / TFIDF / SCCAF) to intelligently integrate clustering from unlimited sources. Applied to multimodal datasets, scTriangulate highlights new cell mechanisms underlying lineage diversity.

□ DeepSE: Detecting super-enhancers among typical enhancers using only sequence feature embeddings
>> https://www.sciencedirect.com/science/article/pii/S0888754321003700
DeepSE is based on a deep convolutional neural network model, to distinguish the SEs from TEs. DeepSE can be generalized well across different cell lines, which implied that cell-type specific SEs may share hidden sequence patterns across different cell lines.
DeepSE uses the whole genome sequences as learning corpus to train dna2vec for generating k-mer embeddings with a fixed number of dimensions. The Parameter dk indicates that every k-mer was represented as a 100-dimension vector.

□ scINSIGHT for interpreting single-cell gene expression from biologically heterogeneous data
>> https://www.biorxiv.org/content/10.1101/2021.10.13.464306v1.full.pdf
Based on a novel matrix factorization model, scINSIGHT learns coordinated gene expression patterns that are common among or specific to different biological conditions, offering a unique chance to jointly identify heterogeneous biological processes and diverse cell types.
scINSIGHT achieves sparse, interpretable, and biologically meaningful decomposition. scINSIGHT simultaneously identifies common and condition-specific gene modules and quantify their expression levels in each sample in a lower-dimensional space.

□ Airpart: Interpretable statistical models for analyzing allelic imbalance in single-cell datasets https://www.biorxiv.org/content/10.1101/2021.10.15.464546v1.full.pdf
Airpart, a statistical method airpart for identifying differential CTS allelic imbalance (AI) from scRNA-seq data, or other spatially- or time-resolved datasets. airpart outputs discrete partitions of data, pointing to groups of genes and cells under common mechanisms.
Airpart uses a Generalized Fused Lasso w/ Binomial likelihood for partitioning groups of cells by AI signal, and a hierarchical Bayesian model. Airpart identifies differential AI patterns across cell states and could be used to define trends of AI signal over spatial / time axes.
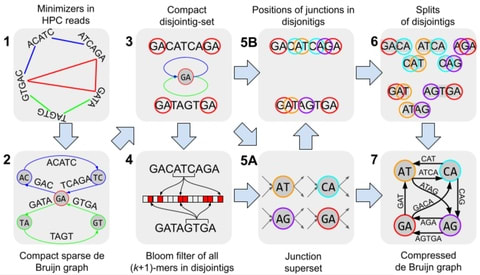
□ La Jolla Assembler (LJA): Assembling Long Accurate Reads Using Multiplex de Bruijn Graphs
>> https://www.biorxiv.org/content/10.1101/2020.12.10.420448v2.full.pdf
La Jolla Assembler (LJA) includes three modules addressing all three challenges in assembling long and accurate reads: jumboDBG (constructing large de Bruijn graphs), mowerDBG (error-correcting reads), and multiplexDBG (utilizing the entire read-length for resolving repeats).
a fast LJA algorithm reduces the error rate by 3 orders of magnitude and constructs the de Bruijn graph for large k-mer sizes. Since the de Bruijn graph constructed for a fixed k-mer size is typically either too tangled or too fragmented, LJA uses a multiplex de Bruijn graph.

□ HiLoop: Identification, visualization, statistical analysis and mathematical modeling of high-feedback loops in gene regulatory networks
>> https://bmcbioinformatics.biomedcentral.com/articles/10.1186/s12859-021-04405-z
HiLoop quantifies the enrichment of high-feedback loops in the given networks and automatically generates parameterized mathematical models that describe characteristic dynamical systems based on the network topologies.
HiLoop visualizes multiple attractors in the state space of specific genes or axes of reduced dimensions. HiLoop can be extended to facilitate the analysis of diverse transient dynamics and spatial (e.g. Turing) patterns generated from individual spatiotemporal models.

□ VLMCs: Fast parallel construction of variable-length Markov chains
>> https://bmcbioinformatics.biomedcentral.com/articles/10.1186/s12859-021-04387-y
The methods range from probability distributions of sequence composition to first and higher-order Markov chains, where a k-th order Markov chain over DNA has 4^k formal parameters.
VLMCs (variable-length Markov chains) adapt the depth depending on sequence context and curtail excesses in the number of parameters. The scarcity of available fast prompted the development of a parallel implementation using lazy suffix trees and a hash-based alternative.

□ A Converse Sum of Squares Lyapunov Function for Outer Approximation of Minimal Attractor Sets of Nonlinear Systems https://arxiv.org/pdf/2110.03093v1.pdf
a new Lyapunov characterization of attractor sets that is well suited to the problem of finding the minimal attractor set. This Lyapunov characterization is non-conservative even when restricted to Sum-of-Squares (SOS) Lyapunov functions.
a SOS programming problem based on determinant maximization that yields an SOS Lyapunov function whose 1-sublevel set has minimal volume, is an attractor set itself, and provides an optimal outer approximation of the minimal attractor set of the ODE.

□ A Bayesian neural network predicts the dissolution of compact planetary systems
>> https://www.pnas.org/content/118/40/e2026053118
a Bayesian neural network (BNN) naturally incorporates confidence intervals into its instability time predictions, accounting for model uncertainty as well as the intrinsic uncertainty due to the chaotic dynamics.
The gradient information can significantly speed up parameter estimation using Hamiltonian Monte Carlo. The model numerically integrates 10,000 orbits for a compact three-planet system (top) and records orbital elements.

□ Axioms for the category of Hilbert spaces
>> https://arxiv.org/pdf/2109.07418v1.pdf
The latter uses the framework of category theory, and emphasises operators more than their underlying Hilbert spaces. It postulates a category with structure that models physical features of quantum theory.
Which axioms guarantee that a category is equivalent to that of continuous linear functions between Hilbert spaces? The approach is similar to Lawvere’s categorical characterisation of the theory of sets. the finite-dimensional Hilbert spaces can be categorically axiomatised.
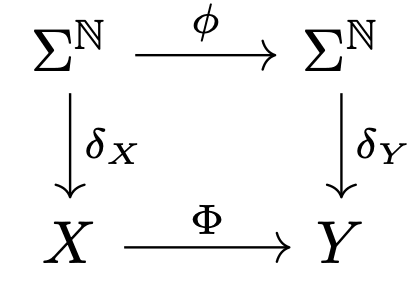
□ Robustness of non-computability
>> https://arxiv.org/pdf/2109.15080v1.pdf
a framework for analyzing whether a non-computability result is robust over continuous spaces. the notion of computability is extended to continuous spaces - i.e., non-discrete topological spaces.
There exists a computable C∞ function h : R2 → R2, h ∈ V(K), such that h has a unique computable equilibrium point s - a sink - and the basin of attraction Ws of s is non-computable, where K is the disk centered at the origin with radius 3.

□ SVAT: Secure Outsourcing of Variant Annotation and Genotype Aggregation
>> https://www.biorxiv.org/content/10.1101/2021.09.28.462259v1.full.pdf
SVAT can decrease the time and memory usage for the annotation of deletions by making use of an annotation vector that contains the 1-bp deletions and making use of this to translate the impact of deletions that span multiple nucleotides.
SVAT utilizes proxy re-encryption to securely re-code the genotype matrices. SVAT can perform counting at the allele count or variant existence level. SVAT makes use of a novel vectorized representation of the variant loci to protect the variant loci information.

□ PEAK2VEC ENABLES INFERRENCE OF TRANSCRIPTIONAL REGULATION FROM ATAC-SEQ
>> https://www.biorxiv.org/content/10.1101/2021.09.29.462455v1.full.pdf
Peak2vec, a novel algorithm that can identify ATAC-seq peaks regulated with the same TF, while providing the corresponding signature motif. Peak2vec is also easier to interpret since a multinomial convolution kernel directly represents a position weight matrix.
Peak2vec performes Gaussian mixture on the embedding vector. peak2vec may also be applied to TF ChIP-seq experiment in case multiple motifs exists for cofactors.

□ TRIPOD: Nonparametric Interrogation of Transcriptional Regulation in Single-Cell RNA and Chromatin Accessibility Multiomic Data
>> https://www.biorxiv.org/content/10.1101/2021.09.22.461437v1.full.pdf
TRIPOD, a nonparametric approach to detect and characterize three-way relationships between a TF, its target gene, and the accessibility of the TF’s binding site, using single-cell RNA and ATAC multiomic data.
TRIPOD matches metacells by either their TF expressions or peak accessibilities. For each matched metacell pair, the variable being matched is controlled for, and differences between the pair in the other two variables are computed.

□ Wavelet Screening: a novel approach to analyzing GWAS data
>> https://bmcbioinformatics.biomedcentral.com/articles/10.1186/s12859-021-04356-5
Wavelets are oscillatory functions that are useful for analyzing the local frequency and time behavior of signals. The signals can then be divided into different scale components and analyzed separately.
Haar Wavelet transforms the raw genotype data similarly to the widely used ‘Gene- or Region-Based Aggregation Tests of Multiple Variants’ method.

□ BlockPolish: accurate polishing of long-read assembly via block divide-and-conquer https://academic.oup.com/bib/advance-article/doi/10.1093/bib/bbab405/6383560
BlockPolish couples four Bidirectional LSTM layers with a compressed projection layer and a flip-flop projection layer to predict the consensus sequence according to the reads-to-assembly alignment.
The Bi-LSTM layers take both left and right alignment features when making decisions. The compressed projection layer converts the alignment features to the DNA sequence without continuously repeated nucleotides.
The flip-flop projection layer converts the alignment features into the DNA sequence in which the continuous repeated nucleotides are flip-flopped.
BlockPolish divides contigs into blocks with low complexity and high complexity according to statistics of reads aligned to the assembly. Dividing contigs and generating feature matrix is done in the BPFGM.

□ scAAnet: Non-linear Archetypal Analysis of Single-cell RNA-seq Data by Deep Autoencoders
>> https://www.biorxiv.org/content/10.1101/2021.09.17.460824v1.full.pdf
Non-linear archetypal analysis methods have been proposed based on kernelization, such as kernel principal convex hull analysis. However, there is no guarantee that kernel-based transformation makes data well-approximated by a simplex.
scAAnet decomposes an expression profile into a usage matrix and a GEP/archetype matrix. The role of the encoder part is to perform a non-linear decomposition of the data by mapping data from a high-dimensional space to a much latent space.

□ Modelling the bioinformatics tertiary analysis research process
>> https://bmcbioinformatics.biomedcentral.com/articles/10.1186/s12859-021-04310-5
a conceptual model that captures the salient characteristics of the research methods and human tasks involved in Bioinformatics Tertiary Analysis.
a Conversational Agent guides the user step by step in the data extraction. The final hierarchical task tree was then converted into an ontological representation using an ontology standard formalism.

□ CVODE: Reverse engineering gene regulatory network based on complex-valued ordinary differential equation model
>> https://bmcbioinformatics.biomedcentral.com/articles/10.1186/s12859-021-04367-2
Grammar-guided genetic programming (GGGP) is utilized to evolve the structure of CVODE and complex-valued firefly algorithm (CFA) is proposed to search the optimal complex-valued parameters of model.
CVODE has the complex-valued structures, constants and coefficients, which could improve the modeling ability. GGGP overcomes the shortcomings of GP and CFA has more population diversity and faster convergence.

□ MM-Deacon: Multimodal molecular domain embedding analysis via contrastive learning
>> https://www.biorxiv.org/content/10.1101/2021.09.17.460864v1.full.pdf
MM-Deacon is trained using SMILES and IUPAC molecule representations as two different modalities. First, SMILES and IUPAC strings are encoded by using two different transformer-based language models independently.
Then the contrastive loss is utilized to bring these encoded representations from different modalities closer to each other if they belong to the same molecule, and to push embeddings farther from each other if they belong to different molecules.
PubChem cross-modal molecule search serves as a way to test the learned agreement across SMILES and IUPAC representations in the joint embedding space. Specifically, molecules in the PubChem test set are all embedded into 512-dimensional vectors in the joint embedding space.

□ STAT: a fast, scalable, MinHash-based k-mer tool to assess Sequence Read Archive next-generation sequence submissions
>> https://genomebiology.biomedcentral.com/articles/10.1186/s13059-021-02490-0
Sequence Taxonomic Analysis Tool (STAT), a scalable k-mer-based tool for fast assessment of taxonomic diversity intrinsic to submissions, independent of metadata.
Based on MinHash, and inspired by Mash, STAT employs a reference k-mer database built from available sequenced organisms to allow mapping of query reads to the NCBI taxonomic hierarchy.
STAT uses the MinHash principle to compress the representative taxonomic sequences by orders of magnitude into a k-mer database, a process that yields a set of diagnostic k-mers for each organism. This allows for significant coverage of taxa w/ a minimal set of diagnostic k-mers.
□ SEDIM: High-throughput single-cell RNA-seq data imputation and characterization with surrogate-assisted automated deep learning
>> https://academic.oup.com/bib/advance-article/doi/10.1093/bib/bbab368/6374131
Deep imputation architectures are difficult to design and tune for those without rich knowledge of deep neural networks and scRNA-seq.
Surrogate-assisted Evolutionary Deep Imputation Model (SEDIM) automatically designs the architectures of deep neural networks for imputing GE levels. SEDIM constructs an offline surrogate model, which can accelerate the computational efficiency of the architectural search.
□ scHiCStackL: a stacking ensemble learning-based method for single-cell Hi-C classification using cell embedding
>> https://academic.oup.com/bib/advance-article-abstract/doi/10.1093/bib/bbab396/6374065
scHiCStackL contains a two-layer stacking learning-based ensemble model. the cell embedding generated by its data preprocessing method increases by 0.23, 1.22, 1.46 and 1.61% comparing with the cell embedding generated by scHiCluster.
The stacking ensemble learning-based model is comprised of Ridge Regression (RR) classifier and Logistic Regression (LR) classifier as the base-classifiers (i.e., first-level) and Gaussian Naive Bayes (GaussianNB) classifier as the meta-classifier.

□ Deep GONet: self-explainable deep neural network based on Gene Ontology for phenotype prediction from gene expression data
>> https://bmcbioinformatics.biomedcentral.com/articles/10.1186/s12859-021-04370-7
Deep GONet architecture represents different levels of the ontology preserving the hierarchical relationships between the GO terms by using sparse regularization.
Deep GONet is based on a MLP constrained by the GO structure. GO gathers three ontologies that respectively describe the following categories: biological process (GO-BP), molecular function, and cellular component.

□ XENet: Using a new graph convolution to accelerate the timeline for protein design on quantum computers
>> https://journals.plos.org/ploscompbiol/article?id=10.1371/journal.pcbi.1009037
XENet, a GNN model that addresses both concerns while also avoid the computational issues introduced by FGNs.
XENet is a message-passing GNN that simultaneously accounts for both the incoming and outgoing neighbors of each node, such that a node’s representation is based on the messages it receives as well as those it sends.
XENet can model residue-level environments better than existing methods ECC and CrystalConv. Not only does the usage of XENet result in lower validation losses, XENet can withstand deeper architectures.
□ RLM: Fast and simplified extraction of Read-Level Methylation metrics from bisulfite sequencing data
>> https://academic.oup.com/bioinformatics/advance-article/doi/10.1093/bioinformatics/btab663/6380544
RLM, a fast and scalable tool that implements established and frequently used inter- and intramolecular metrics of DNA methylation at the read level from bisulfite sequencing experiments.
RLM is applicable for any reference genome, a wide range of library protocols w/ input alignment files from multiple commonly used alignment tools. RLM automatically accounts for potential errors / biases caused by sequencing artifacts, mapping quality and overlapping read pairs.

□ HyINDEL – A Hybrid approach for Detection of Insertions and Deletions
>> https://www.biorxiv.org/content/10.1101/2021.10.08.463662v1.full.pdf
HyINDEL integrates clustering, split-mapping and assembly-based approaches, for the detection of INDELs of all sizes (from small to large) and also identifies the insertion sequences.
HyINDEL starts with identifying clusters of discordant and soft-clip reads which are validated by depth-of-coverage and alignment of soft-clip reads to identify candidate INDELs, while the assembly -based approach is used in identifying the insertion sequence.
□ SFt: Improved Unsupervised Representation Learning of Spatial Transcriptomic Data with Sparse Filtering
>> https://www.biorxiv.org/content/10.1101/2021.10.11.464002v1.full.pdf
Sparse filtering (SFt), uses principles of sparsity and mutual information to build representations from both global and local features from a minimal list of samples. Critically, the samples that comprise each representation are listed and ranked by informativeness.
SFt, implemented with the PyTorch machine learning libraries for Python, returned the most accurate reconstruction of anatomical ground truth of any method tested.
Sparse learning is a powerful, but underexplored means to derive biologically meaningful representations from complex datasets and a quantitative basis for compressed sensing of classifiable phenomena.
SFt should be considered as an alternative to PCA or manifold learning for any high dimensional dataset and the basis for future spatial learning algorithms.

□ Modular assembly of dynamic models in systems biology
>> https://journals.plos.org/ploscompbiol/article?id=10.1371/journal.pcbi.1009513
a model of the Mos/MAPK cascade in a modular fashion using bond graphs. This enabled a principled approach for benchmarking and comparing models of glycolysis with different levels of complexity.
In conjunction with the programmatic approach, bond graphs provide a useful framework for updating models and recording their provenance. MAPK cascade incremental changes were made to incorporate feedback.