

□ STELLAR: Annotation of Spatially Resolved Single-cell Data
>> https://www.biorxiv.org/content/10.1101/2021.11.24.469947v1.full.pdf
STELLAR (SpaTial cELl LeARning), a geometric deep learning tool for cell-type discovery and identification in spatially resolved single-cell datasets. STELLAR uses a graph convolutional encoder to learn low-dimensional cell embeddings that capture cell topology.
STELLAR learns latent low-dimensional cell representations that jointly capture spatial and molecular similarities of cells that are transferable across different biological contexts.
STELLAR automatically assigns cells to cell types included in the reference set and also identifies cells with unique properties as belonging to a novel type that is not part of the reference set.
The encoder network in STELLAR consists of one fully-connected layer with ReLU activation and a graph convolutional layer with a hidden dimension of 128 in all layers. It uses the Adam optimizer with an initial learning rate of 10−3 and weight decay 0.
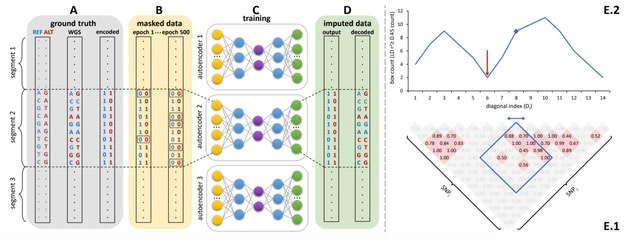
□ Sparse: Rapid, Reference-Free Human Genotype Imputation with Denoising Autoencoders
>> https://www.biorxiv.org/content/10.1101/2021.12.01.470739v1.full.pdf
Sparse, de-noising autoencoders spanning all bi-allelic SNPs observed in the Haplotype Reference Consortium were developed and optimized.
a generalized approach to unphased human genotype imputation using sparse, denoising autoencoders capable of highly accurate genotype imputation at genotype masking levels (98+%) appropriate for array-based genotyping and low-pass sequencing-based population genetics initiatives.
After merging the results from all genomic segments, the whole chromosome accuracy of autoencoder-based imputation remained superior to all HMM-based imputation tools, across all independent test datasets, and all genotyping array marker sets.
Inference time scales only with the number of variants to be imputed, whereas HMM-based inference time depends on both reference panel and the number of variants to be imputed.
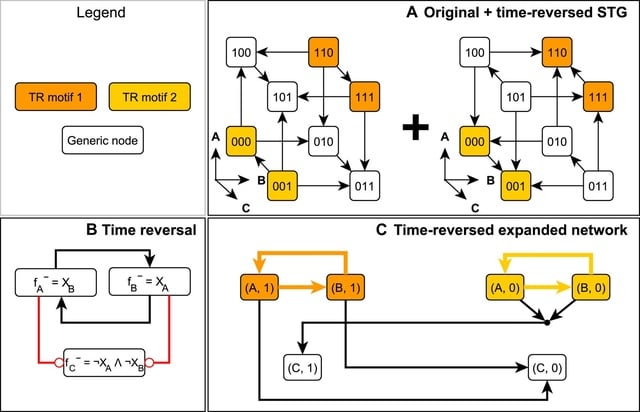
□ Parity and time reversal elucidate both decision-making in empirical models and attractor scaling in critical Boolean networks
>> https://www.science.org/doi/10.1126/sciadv.abf8124
New applications of parity inversion and time reversal to the emergence of complex behavior from simple dynamical rules in stochastic discrete models. These applications underpin a novel attractor identification algorithm implemented for Boolean networks under stochastic dynamics.
Its speed enables resolving a long-standing open question of how attractor count in critical random Boolean networks scales with network size and whether the scaling matches biological observations.
The parity-based encoding of causal relationships and time-reversal construction efficiently reveal discrete analogs of stable and unstable manifolds.
The time reversal of stochastically asynchronous Boolean systems identify subsets of the state space that cannot be reached from outside. Using parity and time-reversal transformations in tandem, This algorithm efficiently identifies all attractors of large-scale Boolean systems.
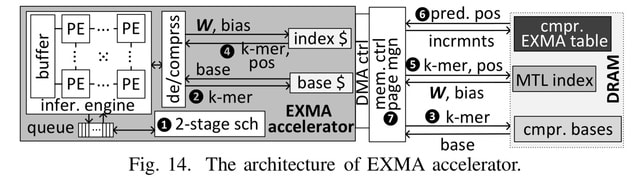
□ EXMA: A Genomics Accelerator for Exact-Matching
>> https://arxiv.org/pdf/2101.05314.pdf
EXMA enhances FM-Index search throughput. EXMA first creates a novel table with a multi-task-learning (MTL)-based index to process multiple DNA symbols with each DRAM row activation.
The EXMA accelerator connects to four DRAM channel, and improves search throughput by 4.9×, and enhances search throughput per Watt by 4.8×. EXMA adopts the state-of-the-art Tangram neural network accelerator as the inference engine.

□ MIRA: Joint regulatory modeling of multimodal expression and chromatin accessibility in single cells
>> https://www.biorxiv.org/content/10.1101/2021.12.06.471401v1.full.pdf
MIRA: Probabilistic Multimodal Models for Integrated Regulatory Analysis, a comprehensive methodology that systematically contrasts transcription and accessibility to determine the regulatory circuitry driving cells along developmental continuums.
MIRA leverages joint topic modeling of cell states and regulatory potential modeling of individual gene loci.
MIRA represents cell states in an interpretable latent space, infers high fidelity lineage trees, determines key regulators of fate decisions at branch points, and exposes the variable influence of local accessibility on transcription at distinct loci.

□ scGTM: Single-cell generalized trend model: a flexible and interpretable model of gene expression trend along cell pseudotime
>> https://www.biorxiv.org/content/10.1101/2021.11.25.470059v1.full.pdf
scGTM can provide more informative and interpretable gene expression trends than the GAM and GLM when the count outcome comes from the Poisson, ZIP, NB or ZINB distributions.
scGTM robustly captures the hill-shaped trends for the four distributions and consistently estimates the change time around 0.75, which is where the MAOA gene reaches its expected maximum expression.
The scGTM parameters are estimated by the constrained maximum likelihood estimation via particle swarm optimization (PSO) metaheuristic algorithms.
scGTM is only applicable to a single pseudotime trajectory. A natural extension is to split a multiple-lineage cell trajectory into single lineages and fit the scGTM to each lineage separately. There is need to develop a variant algorithm of PSO or other metaheuristics algorithms.

□ ECLIPSER: identifying causal cell types and genes for complex traits through single cell enrichment of e/sQTL-mapped genes in GWAS loci
>> https://www.biorxiv.org/content/10.1101/2021.11.24.469720v1.full.pdf
ECLIPSER (Enrichment of Causal Loci and Identification of Pathogenic cells in Single Cell Expression and Regulation data) maps genes to GWAS loci for a given trait using s/eQTL data and other functional information.
ECLIPSER prioritizes causal genes in GWAS loci driving the enrichment signal in the specific cell types for experimental follow-up.
ECLIPSER is a computational framework that can be applied to single cell or single nucleus (sc/sn)RNA-seq data from multiple tissues and to multiple complex diseases and traits with discovered GWAS associations, and does not require genotype data from the e/sQTL.

□ Heron: Dynamic Pooling Improves Nanopore Base Calling Accuracy
>> https://ieeexplore.ieee.org/document/9616376/
Heron - high accuracy GPU nanopore basecaller. Heron is a dynamic pooling approach that continuous and differentiable almost everywhere.
Heron time-warps the signal using fractional distances in the pooling space.
• feature vector: fi = f(xi)∈(0,1)C
• point importance: wi = w(xi), wi∈(0, 1)
• length factor: mi = m(xi ), mi∈(0, 1)
Another intriguing goal is to extend dynamic pooling to multiple dimensions.
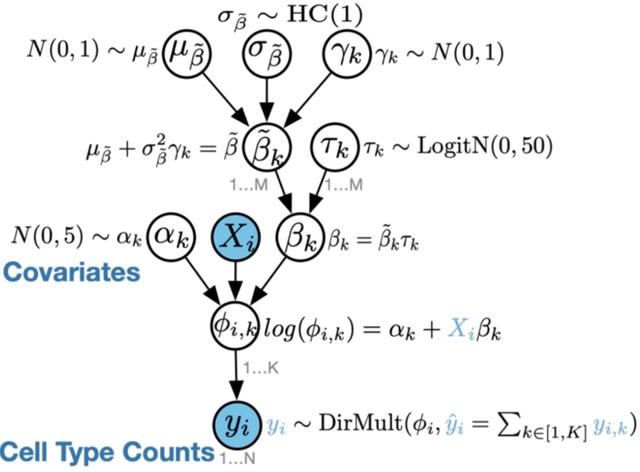
□ scCODA: a Bayesian model for compositional single-cell data analysis
>> https://www.nature.com/articles/s41467-021-27150-6
scCODA allows for identification of compositional changes in high-throughput sequencing count data, especially cell compositions from scRNA-seq. It also provides a framework for integration of cell-type annotated data directly from scanpy and other sources.
scCODA framework models cell-type counts with a hierarchical Dirichlet-Multinomial distribution that accounts for the uncertainty in cell-type proportions and the negative correlative bias via joint modeling of all measured cell-type proportions instead of individual ones.

□ Hubness reduction improves clustering and trajectory inference in single-cell transcriptomic data
>> https://academic.oup.com/bioinformatics/advance-article-abstract/doi/10.1093/bioinformatics/btab795/6433673
Considering a collection of datasets from the ARCHS4 repository, constructed the k-NN graphs with or without hubness reduction, then ran Louvain algorithm and calculated the modularity of the resulting clustering.
Reverse-Coverage approach, a method based on the size of the respective in-coming neighborhoods to retrieve hubs in a more robust way. Hubness reduction can be used instead of dimensionality reduction, in order to compensate for certain manifestations of the dimensionality curse.
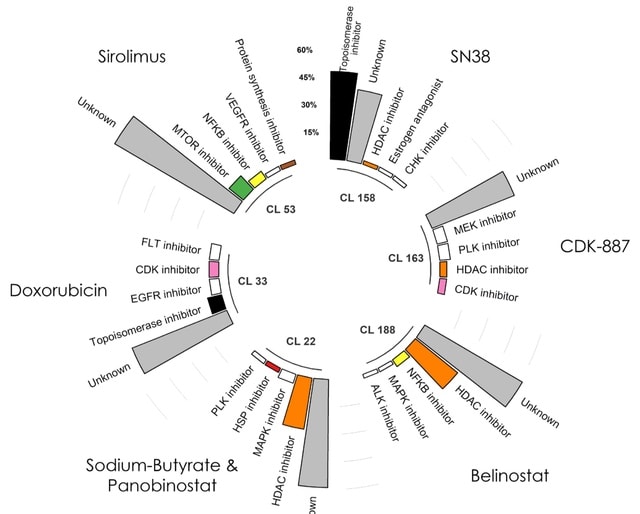
□ DeepSNEM: Deep Signaling Network Embeddings for compound mechanism of action identification
>> https://www.biorxiv.org/content/10.1101/2021.11.29.470365v1.full.pdf
deepSNEM, a novel unsupervised graph deep learning pipeline to encode the information in the compound-induced signaling networks in fixed-length high-dimensional representations.
The core of deepSNEM is a graph transformer network, trained to maximize the mutual information between whole- graph and sub-graph representations that belong to similar perturbations. the 256-dimensional deepSNEM-GT-MI embeddings were clustered using the k-means algorithm.

□ IReNA: integrated regulatory network analysis of single-cell transcriptomes
>> https://www.biorxiv.org/content/10.1101/2021.11.22.469628v1.full.pdf
IReNA integrates both bulk and single-cell RNA-seq data with bulk ATAC-seq data to reconstruct modular regulatory networks which provide key transcription factors and intermodular regulations.
IReNA uses Monocle to construct the trajectory and calculate the pseudotime of single cells. IReNA calculates the smoothed expression profiles based on pseudotime and divide DEGs into different modules using the K-means clustering of the smoothed expression profiles.
IReNA calculates expression correlation (Pearson’s correlation) for each pair of DEGs and select highly correlated gene pairs which contain at least one transcription factor from the TRANSFAC database as potential regulatory relationships.


□ UNIFAN: Unsupervised cell functional annotation for single-cell RNA-Seq
>> https://www.biorxiv.org/content/10.1101/2021.11.20.469410v1.full.pdf
UNIFAN (Unsupervised Single-cell Functional Annotation) to simultaneously cluster and annotate cells with known biological processes including pathways.
UNIFAN uses an autoencoder that outputs a low-dimensional representation learned from the expression of all genes. UNIFAN combines both, the low dimension representation and the gene set activity scores to determine the cluster for each cell.
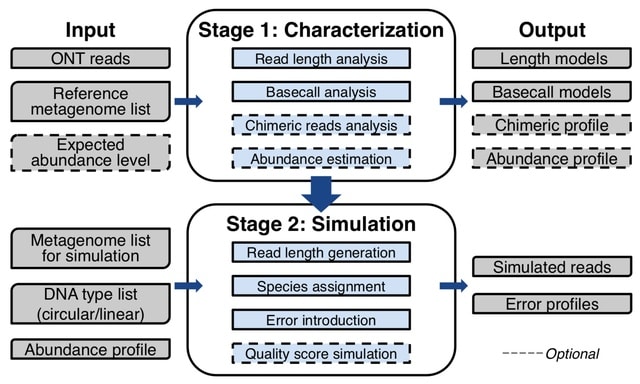
□ Meta-NanoSim: Characterization and simulation of metagenomic nanopore sequencing data
>> https://www.biorxiv.org/content/10.1101/2021.11.19.469328v1.full.pdf
Meta-NanoSim characterizes read length distributions, error profiles, and alignment ratio models. It also detects chimeric read artifacts and quantifies an abundance ptofile. Meta-NanoSim calculates the deviation between expected and estimated abundance levels.
Meta-NanoSim significantly reduced the length of the unaligned regions. Meta-NanoSim uses kernel density estimation learnt from empirical reads.
Meta-NanoSim records the aligned bases for each sub-alignment towards their source genome, and then uses EM algorithm to assign multi-aligned segments proportionally to their putative source genomes iteratively.

□ KCOSS: an ultra-fast k-mer counter for assembled genome analysis
>> https://academic.oup.com/bioinformatics/advance-article/doi/10.1093/bioinformatics/btab797/6443080
KCOSS fulfills k-mer counting mainly for assembled genomes with segmented Bloom filter, lock-free queue, lock-free thread pool, and cuckoo hash table.
KCOSS optimizes running time and memory consumption by recycling memory blocks, merging multiple consecutive first-occurrence k-mers into C-read, and writing a set of C-reads to disk asynchronously.
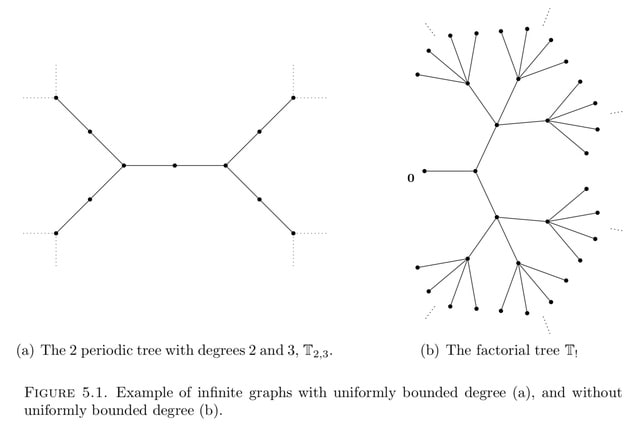
□ On Hilbert evolution algebras of a graph
>> https://arxiv.org/pdf/2111.07399v1.pdf
Hilbert evolution algebras generalize the concept through a framework of Hilbert spaces. This allows to deal with a wide class of infinite-dimensional spaces.
Hilbert evolution algebra associated to a given graph and the Hilbert evolution algebra associated to the symmetric random walk on a graph. These definitions with infinitely many vertices a similar theory developed for evolution algebras associated to finite graphs.

□ Higher rank graphs from cube complexes and their spectral theory
>> https://arxiv.org/pdf/2111.09120v1.pdf
There is a strong connection between geometry of CW-complexes, groups and semigroup actions, higher rank graphs and the theory of C∗-algebras.
The difficulty is that there are many ways to associate C∗-algebras to groups, semigroups and CW-complexes, and this can lead to both isomorphic and non-isomorphic C∗-algebras.
a generalisation of the Cuntz-Krieger algebras from topological Markov shifts. a combinatorial definition of a finite k-graph Λ which is decoupled from geometrical realisations.
The existence of an infinite family of combinatorial k-graphs constructed from k-cube complexes. Aperiodicity of a higher rank graph is an important property, because together with cofinality it implies pure infiniteness if every vertex can be reached from a loop with an entrance.

□ Theory of local k-mer selection with applications to long-read alignment
>> https://academic.oup.com/bioinformatics/advance-article/doi/10.1093/bioinformatics/btab790/6432031
os-minimap2: minimap2 with open syncmer capabilities. Investigating how different parameterizations lead to runtime and alignment quality trade-offs for ONT cDNA mapping.
the k-mers selected by more conserved methods are also more repetitive, leading to a runtime increase during alignment.
Deriving an exact expression for calculating the conservation of a k-mer selection method. This turns out to be tractable enough to prove closed-form expressions for a variety of methods, including (open and closed) syncmers, (a, b, n)-words, and an upper bound for minimizers.

□ CellVGAE: an unsupervised scRNA-seq analysis workflow with graph attention networks
>> https://academic.oup.com/bioinformatics/advance-article/doi/10.1093/bioinformatics/btab804/6448212
CellVGAE leverages the connectivity between cells as an inductive bias to perform convolutions on a non-Euclidean structure, thus subscribing to the geometric deep learning paradigm.
CellVGAE can intrinsically capture information such as pseudotime and NF-B activation dynamics, the latter being a property that is not generally shared by existing neural alternatives. CellVGAE learns to reconstruct the original graph from the lower-dimensional latent space.

□ Portal: Adversarial domain translation networks enable fast and accurate large-scale atlas-level single-cell data integration
>> https://www.biorxiv.org/content/10.1101/2021.11.16.468892v1.full.pdf
Portal, a unified framework of adversarial domain translation to learn harmonized representations of datasets. Portal preserves biological variation during integration, while having significantly reduced running time and memory, achieving integration of millions of cells.
Portal can accurately align cells from complex tissues profiled by scRNA-seq and single-nucleus RNA sequencing (snRNA-seq), and also perform cross-species alignment of the gradient of cells.
Portal can focus only on merging cells of high probability to be of domain-shared cell types, while it remains inactive on cells of domain-unique cell types.
Portal leverages three regularizers to help it find correct and consistent correspondence across domains, including the autoencoder regularizer, the latent alignment regularizer and the cosine similarity regularizer.
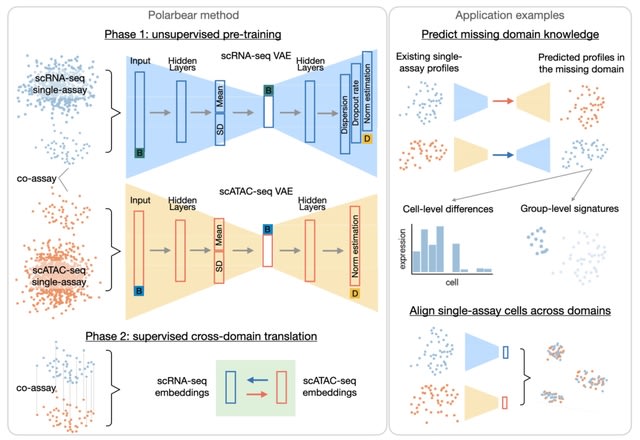
□ Polarbear: Semi-supervised single-cell cross-modality translation
>> https://www.biorxiv.org/content/10.1101/2021.11.18.467517v1.full.pdf
Polarbear uses single-assay and co-assay data to train an autoencoder for each modality and then uses just the co-assay data to train a translator between the embedded representations learned by the autoencoders.
Polarbear is able to translate between modalities with improved accuracy relative to BABEL. Polarbear trains one VAE for each type of data, while taking into consideration sequencing depth and batch factors.

□ sc-SynO: Automated annotation of rare-cell types from single-cell RNA-sequencing data through synthetic oversampling
>> https://bmcbioinformatics.biomedcentral.com/articles/10.1186/s12859-021-04469-x
sc-SynO, which is based on LoRAS (Localized Random Affine Shadowsampling) algorithm applied to single-cell data. The algorithm corrects for the overall imbalance ratio of the minority and majority class.
The LoRAS algorithm generates synthetic samples from convex combinations of multiple shadowsamples generated from the rare cell types. The shadowsamples are obtained by adding Gaussian noise to features representing the rare cells.
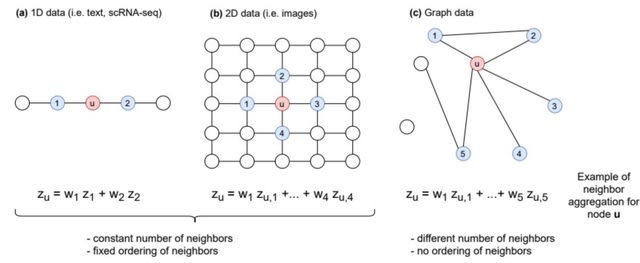
□ Graph-sc: GNN-based embedding for clustering scRNA-seq data
>> https://academic.oup.com/bioinformatics/advance-article-abstract/doi/10.1093/bioinformatics/btab787/6432030
Graph-sc, a method modeling scRNA-seq data as a graph, processed with a graph autoencoder network to create representations (embeddings) for each cell. The resulting embeddings are clustered with a general clustering algorithm to produce cell class assignments.
Graph-sc is stable across consecutive runs, robust to input down-sampling, generally insensitive to changes in the network architecture or training parameters and more computationally efficient than other competing methods based on neural networks.

□ Asc-Seurat: analytical single-cell Seurat-based web application
>> https://bmcbioinformatics.biomedcentral.com/articles/10.1186/s12859-021-04472-2
Asc-Seurat provides: quality control, by the exclusion of low-quality cells & potential doublets; data normalization, incl. log normalization and the SCTransform, dimension reduction, clustering of the cell populations, incl. selection or exclusion of clusters and re-clustering.
Asc-Seurat is built on three analytical cores. Using Seurat, users explore scRNA-seq data to identify cell types, markers, and DEGs. Dynverse allows the evaluation and visualization of developmental trajectories and identifies DEGs on these trajectories.

□ sc-CGconv: A copula based topology preserving graph convolution network for clustering of single-cell RNA-seq data
>> https://www.biorxiv.org/content/10.1101/2021.11.15.468695v1.full.pdf
sc-CGconv, a new robust-equitable copula correlation (Ccor) measure for constructing cell-cell graph leveraging the scale-invariant property of Copula while reducing the computational cost of processing large datasets due to the use of structure-aware using LSH.
sc-CGconv preserves the cell-to-cell variability within the selected gene set by constructing a cell-cell graph through copula correlation measure. And provides a topology-preserving embedding of cells in low dimensional space.

□ PHONI: Streamed Matching Statistics with Multi-Genome References
>> https://ieeexplore.ieee.org/document/9418770/
PHONI, Practical Heuristic ON Incremental matching statistics computation uses longest-common-extension (LCE) queries to compute the len values at the same time that computes the pos values.
The matching statistics MS of a pattern P [0..m − 1] with respect to a text T [0..n − 1] are an array of (position, length)-pairs MS[0..m − 1] such that
•P[i..i+MS[i].len−1]=T[MS[i].pos..MS[i].pos+MS[i].len−1],
• P [i..i + MS[i].len] does not occur in T.
Two-pass algorithm for quickly computing MS using only an O(r)-space data structure during the first pass, from right to left in O(m log log n) time.
• φ−1(p) = SA[ISA[p] + 1] (or NULL if ISA[p] = n − 1), • PLCP[p] = LCP[ISA[p]] (or 0 if ISA[p] = 0),
and SA, ISA, LCP and PLCP are the suffix array, inverse suffix array, longest-common-prefix array and permuted longest-common-prefix array.
PHONI uses Rossi et al.’s construction algorithm for MONI to build the RLBWT and the SLP. PHONI’s query times become faster as the number of reducible positions increases, making the time-expensive LCE queries less frequent.
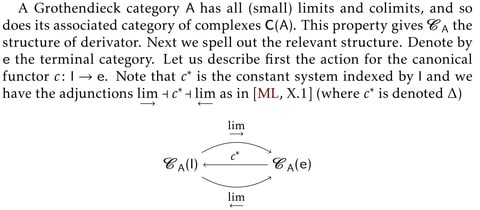
□ UNBOUNDED ALGEBRAIC DERIVATORS
>> https://arxiv.org/pdf/2111.05918v1.pdf
Proving the derived category of a Grothendieck category with enough projective objects is the base category of a derivator. Therefore all such categories possess all co/limits and can be organized in a representable derivator.
This derivator is the base for constructing the derivator associated to the derived category by deriving the relevant functors. the framework provides a more general - arbitrary base ring, complexes as coefficients and simpler approach to some basic theorems of group cohomology.

□ Duesselpore: a full-stack local web server for rapid and simple analysis of Oxford Nanopore Sequencing data
>> https://www.biorxiv.org/content/10.1101/2021.11.15.468670v1.full.pdf
Duesselpore, a deep sequencing workflow that runs as a local webserver and allows the analysis of ONT data everywhere without requiring additional bioinformatic tools or internet connection.
Duesselpore performs differential gene expression (DGE) analysis. DuesselporeTM will also conduct gene set enrichment analyses (GSEA), enrichment analysis based on the DisGeNET and pathway-based data integration and visualization focusing on KEGG.

□ discover: Optimization algorithm for omic data subspace clustering
>> https://www.biorxiv.org/content/10.1101/2021.11.12.468415v1.full.pdf
the ground truth subspace is rarely the most compact one, and other subspaces may provide biologically relevant information.
discover, an optimization algorithm performing bottom-up subspace clustering on tabular high dimensional data. And identifies the corresponding sample clusters, such that the partitioning of the subspace has maximal internal clustering score of feature subspaces.
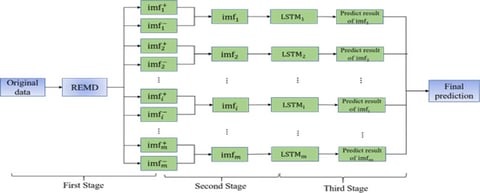
□ REMD-LSTM:A novel general-purpose hybrid model for time series forecasting
>> https://link.springer.com/article/10.1007/s10489-021-02442-y
Empirical Mode Decomposition (EMD) is a typical algorithm for decomposing data according to its time scale characteristics. The core of the EMD algorithm is empirical mode decomposition, which can decompose complex signals into a finite number of Intrinsic Mode Functions.
The REMD-LSTM algorithm can solve the problem of marginal effect and mode confusion in EMD. Decomposing time series data into multiple components through REMD can reveal the specific influence of hidden variables in time series data to a certain extent.

□ smBEVO: A computer vision approach to rapid baseline correction of single-molecule time series
>> https://www.biorxiv.org/content/10.1101/2021.11.12.468397v1.full.pdf
Current approaches for drift correction primarily involve either tedious manual assignment of the baseline or unsupervised frameworks such as infinite HMMs coupled with baseline nodes that are computationally expensive and unreliable.
smBEVO estimates the time-varying baseline drift that can in practice be difficult to eliminate in single-molecule experimental modalities. smBEVO provides visually and quantitatively compelling baseline estimation for simulated data w/ multiple types of mild to aggressive drift.
□ FMAlign: A novel fast multiple nucleotide sequence alignment method based on FM-index
>> https://academic.oup.com/bib/advance-article-abstract/doi/10.1093/bib/bbab519/6458932
FMAlign, a novel algorithm to improve the performance of multiple nucleotide sequence alignment. FM-index uses FM-index to extract long common segments at a low cost rather than using a space-consuming hash table.