
(Created with Midjourney v5.2)


□ Design Patterns of Biological Cells
>> https://arxiv.org/abs/2310.07880
Because design patterns exist at all levels of detail within biology, from the designs of specific molecules to the designs of multi-cellular organisms, they restrict this work to the chemical reaction networks that animate individual cells.
There are three dominant versions of this pattern, which are DNA replication, DNA transcription to RNA, and RNA translation to proteins.
Each is performed by complex biochemical machinery that moves along the template and catalyzes the production of the newly synthesized molecule, and each includes its own version of kinetic proofreading.

□ Deepurify: a multi-modal deep language model to remove contamination from metagenome-assembled genomes
>> https://www.biorxiv.org/content/10.1101/2023.09.27.559668v1
Deepurify developed two distinct encoders, a genomic sequence encoder (GseqFormer) and a taxonomic encoder (LSTM) to encode genomic sequences and their source genomes' taxonomic lineages.
Deepurify initially quantified the taxonomic similarities of contigs by assigning taxonomic lineages to them. It then used these lineages to construct a MAG-separated tree, partitioning the MAG into distinct sections, each containing contigs with the same lineage.
Deepurify optimized contig utilization within the MAG, avoiding immediate removal of contaminated contigs. A tree traversal algorithm was devised to maximize the count of medium- and high-quality MAGs within the MAG-separated tree.
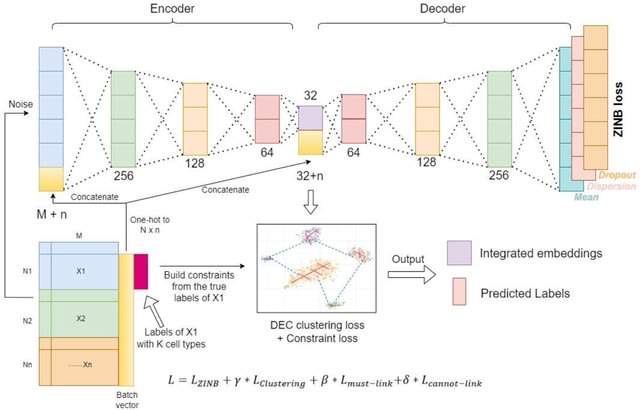
□ scDILT: a model-based and constrained deep learning framework for single-cell Data Integration, Label Transferring, and clustering
>> https://www.biorxiv.org/content/10.1101/2023.10.09.561605v1
scDILT (Single-Cell Deep Data Integration and Label Tranferring) leverages a conditional autoencoder (CAE). The CAE receives the concatenated count matrix of multiple datasets, along with a vector indicating the batch IDs.
scDILT generates an integrated latent space representing the input datasets along with predicted labels for all cells. The cell-to-cell constraints will be built based on the labels of these data and implemented on the bottle-neck layer Z of the autoencoder.

□ ProxyTyper: Generation of Proxy Panels for Privacy-aware Outsourcing of Genotype Imputation
>> https://www.biorxiv.org/content/10.1101/2023.10.01.560384v1
ProxyTyper, a framework for building proxy panels, i.e. panels that are similar in statistical properties to the original panel but are anonymized. ProxyTyper utilizes 3 mechanisms to protect haplotype datasets in terms of variant positions, genetic maps, and variant genotypes.
First mechanism protects the variant positions and genetic maps that can leak side-channel information. Second is resampling of original haplotype panels using a Li-Stephens Markov model with privacy parameters for tuning privacy level and utility.
ProxyTyper generates a mosaic of the original haplotypes so that each chromosome-wide haplotype is a mosaic of the haplotypes in the original panel. The third mechanism consists of encoding the alleles in resampled panels using locality-based hashing and permutation.

□ DiffDec: Structure-Aware Scaffold Decoration with an End-to-End Diffusion Model
>> https://www.biorxiv.org/content/10.1101/2023.10.08.561377v1
DiffDec optimizes molecules through molecular scaffold decoration conditioned on the 3D protein pocket by an E(3)-equivariant graph neural network and diffusion model. DiffDec could identify the growth anchors and generate R-groups well for the scaffolds without provided anchors.
The diffusion process iteratively adds Gaussian noise to the data, while the generative process gradually denoises the noise distribution under the condition of scaffold and protein pocket to recover the ground truth R-groups.


□ ILIAD: A suite of automated Snakemake workflows for processing genomic data for downstream applications
>> https://www.biorxiv.org/content/10.1101/2023.10.11.561910v1
ILIAD, a suite of Snakemake workflows developed with several modules for automatic and reliable processing of raw or stored genomic data that lead to the output of ready-to-use genotypic information necessary to drive downstream applications.
ILIAD offers a containerized workflow with optional automatic downloads of desired files from file transfer protocol (FTP) sites coupled with the use of any genome reference assembly for variant calling using BCFtools.
Iliad features independent submodules for lifting over reference assembly genomic positions (GRCh37 to GRCh38 and vice versa) and merging multiple VCF files at once.

□ MSXFGP: combining improved sparrow search algorithm with XGBoost for enhanced genomic prediction
>> https://bmcbioinformatics.biomedcentral.com/articles/10.1186/s12859-023-05514-7
Chaos theory is a nonlinear theory and has good applications in random number generation. Many swarm intelligence optimization methods use chaos mapping as random number generators to initialize populations.
MSXFGP is based on a multi-strategy improved sparrow search algorithm (SSA) to optimize XGBoost parameters and feature selection. Firstly, logistic chaos mapping, elite learning, adaptive parameter adjustment, Levy flight, and an early stop strategy are incorporated into the SSA.

□ PhyGCN: Pre-trained Hypergraph Convolutional Neural Networks with Self-supervised Learning
>> https://www.biorxiv.org/content/10.1101/2023.10.01.560404v1
PhyGCN aims to enhance node representation learning in hypergraphs by effectively leveraging abundant unlabeled data. Hyperedge prediction is employed as a self-supervised task for model pre-training. The pre-trained embedding model is then used for downstream tasks.
To calculate the embedding for a target node, the hypergraph convolutional network aggregates information from neighboring nodes connected to it via hyperedges, and combines it with the target node embedding to output a final embedding.
PhyGCN employs two adapted strategies: DropHyperedge and Skip/Dense Connection. These strategies randomly mask the values of the adjacency matrix for the base hypergraph convolutional network during each iteration, which helps prevent overfitting and improves generalization.

□ Monopogen: Single-nucleotide variant calling in single-cell sequencing data
>> https://www.nature.com/articles/s41587-023-01873-x
Monopogen, a computational framework that enables researchers to detect single-nucleotide variants (SNVs) from a variety of single-cell transcriptomic and epigenomic sequencing data.
Monopogen uses high-quality haplotype and linkage disequilibrium (LD) data from an external reference panel to overcome uneven sequencing coverage, allelic dropout and sequencing errors in single-cell sequencing data.
Monopogen further conducts LD scoring at the cell population level within each sample, leveraging the expectation that most alleles are identical and in perfect LD with neighboring alleles across the genome, except for those that are somatically altered in a subpopulation of cells.

□ Ribotin: Automated assembly and phasing of rDNA morphs
>> https://www.biorxiv.org/content/10.1101/2023.09.29.560103v1
Ribotin uses the highly accurate long reads to build a graph which represents all variation within the rDNA. Then ultralong ONT reads are aligned to the graph and are used to detect rDNA repeat units. The ONT read paths are clustered to rDNA morphs..
Ribotin has integration with the assembly tool verkko to assemble rDNA morphs per chromosome. Ribotin also has a mode to run without a verkko assembly using only a related reference rDNA sequence. Ribotin detects the rDNA tangles using the reference k-mers and graph topology.

□ LMSRGC: Reference-based genome compression using the longest matched substrings with parallelization consideration
>> https://bmcbioinformatics.biomedcentral.com/articles/10.1186/s12859-023-05500-z
LMSRGC, an algorithm based on reference genome sequences, which uses the suffix array (SA) and the longest common prefix (LCP) array to find the longest matched substrings (LMS) for the compression of genome data in FASTA format.
The proposed algorithm utilizes the characteristics of SA and the LCP array to select all appropriate LMSs between the genome sequence to be compressed and the reference genome sequence and then utilizes LMSs to compress the target genome sequence.


□ CEN-DGCNN: Co-embedding of edges and nodes with deep graph convolutional neural networks
>> https://www.nature.com/articles/s41598-023-44224-1
CEN-DGCNN (Co-embedding of Edges and Nodes with Deep Graph Convolutional Neural Networks) introduces multi-dimensional edge embedding representation. It constructs a message passing framework which introduces the idea of residual connection and dense connection.
Based on CEN-DGCN, a deep graph convolution neural network can be designed to mine remote dependency relationships between nodes. Each layer can learn node features and edge features simultaneously, and can be updated iteratively across layers.
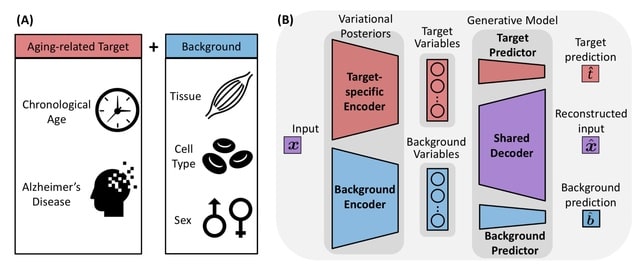
□ StrastiveVI: Isolating structured salient variations in single-cell transcriptomic data
>> https://www.biorxiv.org/content/10.1101/2023.10.06.561320v1
StrastiveVI (Structured Contrastive Variational Inference) leverages previous advances in conditionally invariant representation learning to model the variations underlying scRNA-seq data using two sets of latent variables.
Strastive VI separates the target variations and the dominant background variations. The background variables, are invariant to the given covariate of interest. The target variables, capture variations related to the covariate of interest.

□ HycDemux: a hybrid unsupervised approach for accurate barcoded sample demultiplexing in nanopore sequencing
>> https://genomebiology.biomedcentral.com/articles/10.1186/s13059-023-03053-1
HycDemux integrates an unsupervised hybrid approach to achieve accurate clustering, in which the nucleotides-based greedy algorithm is utilized to obtain initial clusters, and the raw signal information is measured to guide the continuously optimization of clustering.
HycDemux integrates a module that uses a voting mechanism to determine the final demultiplexing result. This module selects n representatives (5 by default) for each cluster and calculates the Dynamic Time Warping.

□ diVas: Digenic variant interpretation with hypothesis-driven explainable AI
>> https://www.biorxiv.org/content/10.1101/2023.10.02.560464v1
diVas, an ML-based approach for digenic variant interpretation aiming to overcome the limitations of the other tools described above. Unlike other tools, diVas leverages proband's phenotypic information to predict the probability of each pair to be causative.
diVas employs cutting-edge Explainable Artificial Intelligence (XAl) techniques for further subclassification into distinct digenic mechanisms: True Digenic /Composite and Dual Molecular Diagnosis.

□ Incorporating extrinsic noise into mechanistic modelling of single-cell transcriptomics
>> https://www.biorxiv.org/content/10.1101/2023.09.30.560282v1
A fully Bayesian framework for the mechanistic analysis of scRNAseq data based on the telegraph model of gene expression, building on single cell sequencing / Kinetics analysis and including cell size effects via a cell-specific scaling factor.
This framework is implemented in the probabilistic programming language Stan and relies on a state-of-the-art Hamiltonian Monte Carlo sampler. It uses Bayesian model selection to distinguish between modes of gene expression and evaluate the possible presence of zero-inflation.


□ MINI-AC: inference of plant gene regulatory networks using bulk or single-cell accessible chromatin profiles
>> https://onlinelibrary.wiley.com/doi/10.1111/tpj.16483
MINI-AC (Motif-Informed Network Inference based on Accessible Chromatin), a computational method that integrates TF motif information with bulk or single-cell derived chromatin accessibility data to perform motif enrichment analysis and GRN inference.
MINI-AC generates information about motifs showing enrichment on the ACRs, a network that is context-specific for a functional enrichment analysis. MINI-AC can be used in two alternative modes - genome-wide and locus-based - to select different non-coding genomic spaces.


□ MBE: model-based enrichment estimation and prediction for differential sequencing data
>> https://genomebiology.biomedcentral.com/articles/10.1186/s13059-023-03058-w
MBE can readily make use of modern-day neural network models in a plug-and-play manner, which also enables us to easily handle (possibly overlapping) reads of different lengths.
For example, fully convolutional neural network classifiers naturally handle variable-length sequences because the convolutional kernels and pooling operations in each layer are applied in the same manner across the input sequence, regardless of its length.
MBE trivially generalizes to settings with more than two conditions of interest by replacing the binary classifier with a multi-class classifier.
The multi-class classification model is trained to predict the condition from which each read arose; then, the density ratio for any pair of conditions can be estimated using the ratio of its corresponding predicted class probabilities.

□ LIANA: Comparison of methods and resources for cell-cell communication inference from single-cell RNA-Seq data
>> https://www.nature.com/articles/s41467-022-30755-0
CCC events are typically represented as a one-to-one interaction between a transmitter and receiver protein, accordingly expressed by the source and target cell clusters. The information about which transmitter binds to which receiver is extracted from diverse sources.
LIANA (a LIgand-receptor ANalysis frAmework) takes any annotated single-cell RNA (scRNA) dataset as input and establishes a common interface to all the resources and methods in any combination. LIANA provides a consensus ranking for the method’s predictions.

□ Benchmarking long-read RNA-sequencing analysis tools using in silico mixtures
>> https://www.nature.com/articles/s41592-023-02026-3
For long-read RNA-seq, This study is the first to compare differential transcript expression (DTE) and differential transcript usage (DTU) methods on a controlled dataset with a tens of millions of reads per sample, as is typically available in short-read studies.
DTU analysis calculates the proportion of transcript expression relative to all transcripts, which can be impacted more readily by changes in quantification of any transcript from a gene. Therefore, the difference of quantification in ONT and Illumina data had a larger impact.

□ happi: a hierarchical approach to pangenomics inference
>> https://genomebiology.biomedcentral.com/articles/10.1186/s13059-023-03040-6
happi is a method for modeling gene presence in pangenomics that leverages information about genome quality. happi models the association between an experimental condition and gene presence where the experimental condition is the primary predictor of interest.
happi provides sensible results in an analysis of metagenome-assembled genome data, improves statistical inference under simulation. The latent variable structure of the model makes the expectation-maximization algorithm an appealing choice for estimating unknown parameters.

□ PaGeSearch: A Tool for Identifying Genes within Pathways in Unannotated Genomes
>> https://www.biorxiv.org/content/10.1101/2023.09.26.559665v1
PaGeSearch identifies a list of genes within a genome, with a focus on genes associated with specific pathways. By identifying candidate regions through a sequence similarity search and performing gene prediction within them, PaGeSearch significantly reduces the search space.
PaGeSearch uses a neural network model to provide candidates that are the most likely orthologs of the query genes.

□ GenArk: towards a million UCSC genome browsers
>> https://genomebiology.biomedcentral.com/articles/10.1186/s13059-023-03057-x
GenArk (Genome Archive), a collection of UCSC Genome Browsers from NCBI assemblies. Built on our established track hub system, this enables fast visualization of annotations. Assemblies come with gene models, repeat masks, BLAT, and in silico PCR.
The GenArk genome browsers cover multiple clades: 159 primates, 409 mammals, 270 birds, 271 fishes, 115 other vertebrates, 598 invertebrates, 554 fungi, and 230 plants. It also includes 446 assemblies from the Vertebrate Genome Project (VGP) and 336 legacy assemblies.

□ scRANK: Ranking of cell clusters in a single-cell RNA-sequencing analysis framework using prior knowledge
>> https://www.biorxiv.org/content/10.1101/2023.10.02.560416v1
A novel methodology that exploits prior knowledge for a disease in combination with expert-user information to accentuate cell types from a scRNA-seq analysis that are most closely related to the molecular mechanism of a disease of interest.
The methodology is fully automated and a ranking is generated for all cell types. This provides a ranking which is based on topology information obtained from the CellChat networks.

□ Accelerated identification of disease-causing variants with ultra-rapid nanopore genome sequencing
>> https://www.nature.com/articles/s41587-022-01221-5
An approach for ultra-rapid nanopore WGS that combines an optimized sample preparation protocol, distributing sequencing over 48 flow cells, near real-time base calling and alignment, accelerated variant calling and fast variant filtration for efficient manual review.
The cloud-based pipeline scales compute-intensive base calling and alignment across 16 instances with 4× Tesla V100 GPUs each and runs concurrently with sequencing.
The instances aim for maximum resource utilization, where base calling using Guppy runs on GPU and alignment using Minimap2 runs on 42 virtual CPUs in parallel. Small-variant calling performed using GPU-accelerated PEPPER–Margin–DeepVariant.

□ AutoClass: A universal deep neural network for in-depth cleaning of single-cell RNA-Seq data
>> https://www.nature.com/articles/s41467-022-29576-y
AutoClass integrates two DNN components, an autoencoder and a classifier, as to maximize both noise removal and signal retention. AutoClass is distribution agnostic as it makes no assumption on specific data distributions, hence can effectively clean a wide range of noise and artifacts.
AutoClass effectively models and cleans a wide range of noises and artifacts in scRNA-Seq data including dropouts, random uniform, Gaussian, Gamma, Poisson, and negative binomial noises, as well as batch effects.

□ Mabs: a suite of tools for gene-informed genome assembly
>> https://bmcbioinformatics.biomedcentral.com/articles/10.1186/s12859-023-05499-3
Mabs is a genome assembly tool which optimizes parameters of genome assemblers Hifiasm and Flye. Mabs optimizes parameters of a genome assembler to make an assembly where protein-coding genes are assembled more accurately.
Mabs is able to distinguish true multicopy orthogroups from false multicopy orthogroups, because genes originating from haplotypic duplications have two times lower coverage than correctly assembled genes.

□ The longest intron rule
>> https://www.biorxiv.org/content/10.1101/2023.10.02.560625v1
The presence of introns substantially increases the complexity of ribosomal protein gene expression as they variably slow the expression cycle, and in addition, many introns can contain non-coding RNA involved in other layers of regulation.
The localization of the longest intron in the second or third third is significantly more frequent for certain functionally related groups of genes, e.g. for DNA repair genes.

□ DAESC: Single-cell allele-specific expression analysis reveals dynamic and cell-type-specific reg- ulatory effects
>> https://www.nature.com/articles/s41467-023-42016-9
DAESC (Differential Allelic Expression using Single-Cell data) accounts for haplotype switching using latent variables and handles sample repeat structure of single-cell data using random effects.
DAESC is based on a beta-binomial regression model and can be used for differential ASE against any independent variable, such as cell type, continuous developmental trajectories, genotype (eQTLs), or disease status.
The baseline model DAESC-BB is a beta-binomial model with individual-specific random effects that account for the sample repeat structure arising from multiple cells measured per individual inherent to single-cell data.
DAESC-BB can be used generally for differential ASE regardless of sample size (number of individuals, N). When sample size is reasonably large (e.g., N ≥ 20), a full model DAESC-Mix that accounts for both sample repeat structure and implicit haplotype phasing.

□ KmerSV: a visualization and annotation tool for structural variants using Human Pangenome derived k-mers
>> https://www.biorxiv.org/content/10.1101/2023.10.11.561941v1
KmerSV, a new tool for SV visualization and annotation. To mediate these functions, KmerSV uses a reference sequence deconstructed into its component k-mers, each having a length of 31 bp. These reference-derived k-mers are compared to the sequence of interest.
The program maps the Pangenome or other reference 31-mers against one or multiple target sequences which can include either contigs or sequence reads.
Initially, they retrieve these k-mers via a sliding window across a segment of the reference with its coordinate information. Then, the retrieved k-mers are systematically mapped against the target.
Unique 31-mers (as defined by the reference) serve as "anchor" points in the target sequence to facilitate using k-mers with multiple coordinates. This anchoring process eliminates ambiguous k-mers and improves the visualization of complex SVs such as duplications.

□ PanKmer: k-mer based and reference-free pangenome analysis
>> https://academic.oup.com/bioinformatics/advance-article/doi/10.1093/bioinformatics/btad621/7319363
PanKmer, a non-graphical k-mer decomposition method designed to efficiently represent and analyze many forms of variation in large pangenomic datasets, with no reliance on a reference genome and no assumption of annotation.
PanKmer includes a function to calculate the number of shared k-mers between all pairs of input genomes and return them as an adjacency matrix. Subsequently, the adjacency values can be used to perform a hierarchical clustering of input genomes.

□ Oxford Nanopore
>> https://nanoporetech.com/about/events/community-meetings/ncm-2023-houston
This week is #WorldSpaceWeek! At #nanoporeconf, Sarah Castro-Wallace will share @NASA’s project to take the MinION device to Mars — which will prove invaluable if we are to discover life beyond Earth.